the Creative Commons Attribution 4.0 License.
the Creative Commons Attribution 4.0 License.
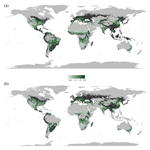
Global meta-analysis of the relationship between soil organic matter and crop yields
Emily E. Oldfield
Mark A. Bradford
Stephen A. Wood
Resilient, productive soils are necessary to sustainably intensify agriculture to increase yields while minimizing environmental harm. To conserve and regenerate productive soils, the need to maintain and build soil organic matter (SOM) has received considerable attention. Although SOM is considered key to soil health, its relationship with yield is contested because of local-scale differences in soils, climate, and farming systems. There is a need to quantify this relationship to set a general framework for how soil management could potentially contribute to the goals of sustainable intensification. We developed a quantitative model exploring how SOM relates to crop yield potential of maize and wheat in light of co-varying factors of management, soil type, and climate. We found that yields of these two crops are on average greater with higher concentrations of SOC (soil organic carbon). However, yield increases level off at ∼2 % SOC. Nevertheless, approximately two-thirds of the world's cultivated maize and wheat lands currently have SOC contents of less than 2 %. Using this regression relationship developed from published empirical data, we then estimated how an increase in SOC concentrations up to regionally specific targets could potentially help reduce reliance on nitrogen (N) fertilizer and help close global yield gaps. Potential N fertilizer reductions associated with increasing SOC amount to 7 % and 5 % of global N fertilizer inputs across maize and wheat fields, respectively. Potential yield increases of 10±11 % (mean ± SD) for maize and 23±37 % for wheat amount to 32 % of the projected yield gap for maize and 60 % of that for wheat. Our analysis provides a global-level prediction for relating SOC to crop yields. Further work employing similar approaches to regional and local data, coupled with experimental work to disentangle causative effects of SOC on yield and vice versa, is needed to provide practical prescriptions to incentivize soil management for sustainable intensification.
- Article
(2581 KB) - Full-text XML
-
Supplement
(1573 KB) - BibTeX
- EndNote
The pressure to increase crop production has resulted in the expansion of land area dedicated to agriculture and the intensification of cropland management through practices such as irrigation and fertilization. These practices have led to degradation of land and waters, prompting sustainable intensification initiatives to increase yields on existing farmland while decreasing the environmental impact of agriculture (Foley et al., 2011; Godfray et al., 2010; Mueller et al., 2012). One sign of land degradation is the loss of soil organic matter (SOM) (Reeves, 1997). Rebuilding SOM in agricultural lands holds the promise of improving soil fertility, as SOM affects many properties of soils, including their ability to retain water and nutrients, to provide structure promoting efficient drainage and aeration, and to minimize loss of topsoil via erosion (Reeves et al., 1997; Robertson et al., 2014). As such, managing SOM to ensure stable and long-lasting crop productivity and to decrease reliance on external inputs such as mineral fertilizers and irrigation has been identified as a critical component of sustainable intensification (Foley et al., 2011). Yet the emphasis on soil management has remained qualitative, meaning that the potential contribution of building SOM as a means to increase crop production and minimize the environmental impact of agriculture has not yet been broadly quantified (Adhikari and Hartemink, 2016; Chabbi et al., 2017; Hatfield et al., 2017).
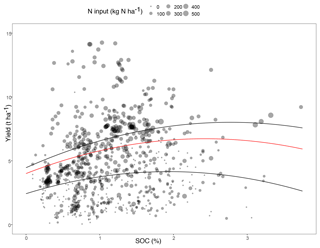
Figure 1Relationship between SOC and yield of maize for published studies. The regression lines are modeled yields (i.e., effect sizes) for rain-fed (i.e., non-irrigated) maize using observed means of our meta-dataset for aridity, pH, texture, and latitude at different N input rates. We varied SOC (x axis) across the range of values extracted from the literature. The red line represents the mean N input rate (118 kg N ha−1 yr−1) across all studies, with the bottom line representing 0 inputs of N and the top line representing 200 kg N ha−1 yr−1. For the raw data points, N input is mapped as a continuous variable across its range from 0 (smallest circles) to 500 kg N ha−1 yr−1 (largest circles). Note that the observed scatter of the individual observations is an outcome of the fact that yield is controlled by multiple factors (Table 1), and therefore the regression lines isolate just the potential effect of SOC with all other factors held constant.
A primary hurdle to managing SOM for sustainable intensification is the lack of predictive, quantitative targets of SOM for specific agricultural and environmental objectives (Herrick, 2000; NRC, 2010). While several studies show correlations between SOM and yield (Culman et al., 2013; de Moraes Sa et al., 2014; Lucas and Weil, 2012; Stine and Weil, 2002), it remains unclear how much yield could be expected to increase per unit change in organic matter (Herrick, 2000; NRC, 2010). Establishing these quantitative metrics is challenging because research shows increases (Bauer and Black, 1992), decreases (Bhardwaj et al., 2011), and no change (Hijbeek et al., 2017) in yields with increased SOM. This lack of a general relationship is likely the result of a number of interacting factors related to management, climate, and soil type that can confound the SOM–yield relationship. This confusion has led some to claim that the amount of SOM is unnecessary for crop yields, so long as there is sufficient N fertilizer (Hijbeek et al., 2017; Loveland and Webb, 2003; Oelofse et al., 2015), whereas others highlight the need to build SOM to increase crop yields while minimizing environmental harm (Lal, 2004). The growing momentum to launch global-scale initiatives to manage SOM (Banwart et al., 2014; Lal, 2004; Minasny et al., 2017; Zomer et al., 2017) suggests the need to test competing claims about the effects of SOM on these agricultural and environmental outcomes.
One could critique the effort to establish a global-level understanding of the SOM–yield relationship on the grounds that farm-level responses are necessarily heterogeneous and poorly predicted by global assessments. Yet, global initiatives for managing SOM could create policy environments that stimulate regional and local prescriptions for SOM levels that inform practice (Chabbi et al., 2017; Minasny et al., 2017; Zomer et al., 2017). Whereas it is difficult to disentangle the extent to which SOM–yield relationships are driven by SOM effects on yield, as opposed to yield (i.e., higher plant carbon inputs) effects on SOM, there is nevertheless experimental evidence showing that building SOM positively affects yield (Bauer and Black, 1994; Majumder et al., 2008; Oldfield et al., 2017). In addition, numerous soil properties that relate to soil fertility, such as water holding capacity, respond positively to increasing SOM and in turn are expected to increase yields (Williams et al., 2016). As such, correlative SOM–yield relationships suggest the potential – but likely not the true – effect of SOM on yield.
We developed a quantitative model exploring how SOM relates to crop yield potential in light of co-varying factors of management, soil type, and climate. The aim is that this model can then be used to establish relationships at broad scales between SOM and yield to provide better quantification of this relationship for policy initiatives. We quantified the relationship between SOM (measured as soil organic carbon, SOC, which is a common proxy for SOM) and yield at a global level using data from published studies. We focused our analyses on wheat and maize, two common staple crops that (along with rice) constitute two-thirds of the energy in human diets (Cassman, 1999). Along with SOC, we modeled the effects on crop yields of several factors widely reported in yield studies: N input rate, irrigation, pH, soil texture (% clay), aridity, crop type (i.e., wheat or maize), and latitude (as a proxy for growing-season day length). The data informing our model came from empirical studies that capture local-scale variation in these variables, and hence we interpret our results in light of the correlative nature of the database we assembled. Using the resulting multiple-regression relationship, we then estimated how an increase in SOC concentrations up to regionally specific target thresholds might affect global yields. Our overarching aim was to estimate the potential extent to which restoring SOC in global agricultural lands could help close global yield gaps and potentially help reduce reliance on – and the negative effects of – N fertilizer.
2.1 The relationship between SOC and yield
At the global level and focusing specifically on the potential effect size of SOC on yield, we found that the largest gains in yield occur between SOC concentrations of 0.1 % and 2.0 %. For instance, yields are 1.2 times higher at 1.0 % SOC than 0.5 % SOC (Fig. 1). Gains in yield leveled off at a concentration of approximately 2 % SOC (Fig. 1). Two percent SOC has previously been suggested as a critical threshold, with values below this concentration threatening the structure and, ultimately, the ability of a soil to function (Kemper and Koch, 1966). Importantly, the asymptotic relationship between SOC and yield lends support to the idea that building SOC will increase yields – at least to a certain extent – as opposed to simply being an outcome of higher yields. That is, if yield was an explanatory variable for SOC, we would expect greater yields to keep driving greater levels of SOC (i.e., the relationship would appear more linear) since we know that soils can accumulate concentrations much greater than 2 % (Castellano et al., 2015). However, our data do not display a linear pattern, suggesting that higher yields are not driving higher levels of SOC.
It has been suggested that there is no evidence for 2 % SOC being a critical threshold for productivity, as long as there is sufficient mineral fertilizer to support crop production (Edmeades, 2003; Loveland and Webb, 2003; Oelofse et al., 2015). Such conclusions deem the amount of SOM as substitutable by mineral fertilizers (at least for crop growth), but are inconsistent with the motivation for sustainable intensification to minimize environmental harm caused by mineral fertilizers in relation to emissions of greenhouse gases and eutrophication of waters (Vitousek et al., 2009). The same logic about substitutability also does not account for the other co-benefits associated with building SOM in agricultural lands, such as reductions in nutrient runoff, drought resistance, and yield stability (Robertson et al., 2014). Field- and regional-scale studies have shown a similar pattern as that observed from our global analysis: there exists a positive relationship between SOC and yield that starts to level off at ∼2 % SOC (Kravchenko and Bullock, 2000; Pan et al., 2009; Zvomuya et al., 2008). Our analysis suggests that this relationship holds on average at the global scale and when N fertilization is controlled for.
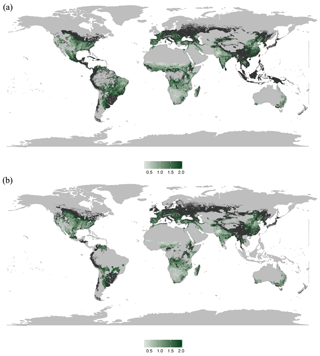
Figure 2Global maize and wheat lands with less than 2 % SOC. Cultivated (a) maize lands and (b) wheat lands on soils with SOC contents less than 2 %. Approximately two-thirds of all maize (61 %) and of all wheat (64 %) producing areas are on soils with less than 2 % SOC. Black areas on the maps are cultivated maize and wheat lands that have concentrations over 2 % SOC. Yield data are taken from EarthStat and SOC data are taken from ISRIC SoilGrids.
Ninety-one percent of the published studies used for our analysis were carried out in fields with less than 2 % SOC, with a mean of 1.1 %. To see whether these observations in SOC distribution reflected global patterns, we used globally gridded data on crop yield and SOC (to a depth of 15 cm) (Hengl et al., 2014; Monfreda et al., 2008). We found that, by both area and production, two-thirds of maize and wheat cultivation takes place on soils with less than 2 % SOC (Fig. 2). Indeed, a recent analysis estimates that agricultural land uses (including cropland and grazing) have resulted in a loss of 133 Pg of carbon over the past 12 000 years of human land use (Sanderman et al., 2017). There appears to be, therefore, significant opportunity to increase SOC on maize and wheat lands to improve crop yields.
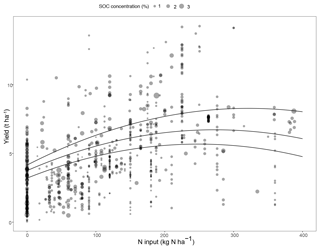
Figure 3Potential reductions in nitrogen fertilizer with an increase in SOC concentration. The lines on the graph represent varying SOC concentrations – 2.0 %, 1.0 %, and 0.5 % SOC – from top to bottom for rain-fed maize. These lines are plotted on top of the observations from our dataset with SOC mapped as a continuous variable across its range from 0.1 % (smallest circles) to 3.0 % (largest circles). Our model shows that keeping yield constant by increasing SOC contents allows for potentially significant reductions in N input (e.g., the same yield is achievable with 0 N input and 2 % SOC as with 50 kg N ha−1 yr−1 and 0.5 % SOC). Recognizing that the 0 N input values may influence the modeled relationship, we analyzed data excluding these values. The qualitative patterns remain the same if the 0 N input values are excluded from the analysis; and while the absolute quantitative patterns shift slightly, the general trends remain intact.
Table 1Modeled regression coefficients with standard errors, standardized coefficients, and P values for our regression model.
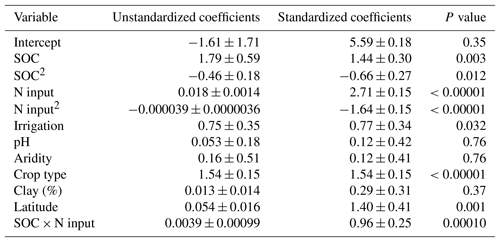
The output of our linear mixed effect model (n=834). The full model explained 83 % of observed variability within the dataset with fixed effects (included in the table) accounting for 42 % of the variability. Standardized coefficients allow for direct comparison of the relative effect size of each modeled variable despite different scales on which the variables are measured. For example, crop type's effect on yield is 2 times greater than that of irrigation. Crop type was coded as a binary variable with 0 for wheat and 1 for maize. Irrigation was also coded as a binary variable with 0 for no irrigation and 1 for irrigation.
2.2 The interaction between SOC and N fertilizer on yield
One of the key goals of sustainable intensification is to reduce the environmental impacts of agriculture (Foley et al., 2011; Mueller et al., 2012). Nitrogen fertilization, while a boon to yields, can cause environmental damages, such as eutrophication of waters and increased soil emissions of nitrous oxide, a potent greenhouse gas (Vitousek et al., 2009). Using our regression model, we asked whether there might be target N fertilizer addition rates that suggest the possibility of maximizing yield per unit N applied by building SOC and reducing inorganic N inputs. We wanted to see if yields converge at higher levels of SOC, suggesting that crops are obtaining sufficient nutrients through SOM and excess mineral N is not necessary. Our analysis suggests that SOC is not directly substitutable for mineral fertilizer (Fig. 1); however, at lower rates of N input (≤50 kg N ha−1), we found that increasing SOC from 0.5 % to 1.0 % could potentially maintain current yields and reduce fertilizer inputs by approximately half (50 %). At higher rates of N input (≥200 kg N ha−1), an increase from 0.5 % to 2.0 % SOC could potentially reduce synthetic N inputs by up to 70 % per hectare (Fig. 3). Building SOC from 0.5 % to 2.0 % represents a very large increase, which would require a significant amount of inputs that may not be feasible due to inherent and logistical difficulties related to soil properties, climate, and farmer access to inputs. Furthermore, such an increase could take several years or decades to accomplish. For example, results from long-term field trials show a range of annual increases in SOC for temperate agricultural soils, which were as low as 0.3 % and as high as 18 % (Poulton et al., 2018). At the low end of this range, and starting at 0.5% SOC, it would take ∼47 years to build to 2 % SOC if the annual relative rate of increase was constant, and ∼9 years at the high end of the range. Admittedly, the range emerged as a result of a number of different inputs ranging from farmyard manure to sewage sludge to mineral fertilization, some of which may not be available to farmers given cost and/or access (Poulton et al., 2018). Feasibility aside, however, our results suggest that building SOM in agricultural lands may supply enough plant available nutrients to sustain crop yields while drastically cutting back on N fertilizer inputs.
Table 2Scenarios for increases in yield and reductions in N input with an increase in SOC concentration to target values.
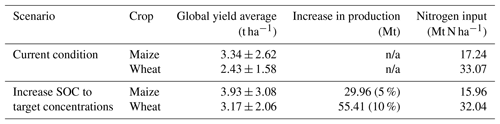
Values (mean ± SD) represent current EarthStat yields and projected gains in yield and production (with % increase in parentheses) resulting from an increase in SOC concentration to target values for each agroecological zone (AEZ; targets ranged from 1.0 % to 2.0 %). We used our regression model to determine potential gains in EarthStat yield and reductions in EarthStat N input. Global yield averages represent tonnes produced per unit land area, whereas production represents tonnes of maize and wheat produced globally.
There was an interaction between SOC and N input, where at higher SOC concentrations N input had a greater impact on yield (Fig. 1, Table 1). This may be because higher SOC improves soil structure and water holding properties, resulting in improved crop growth at a given level of N input (Powlson et al., 2011). Higher levels of SOM could also provide more essential macro- and micronutrients that are limiting in soils with lower SOC concentrations. Additionally, soils receiving more N may have greater SOC because N increases crop yields, which can increase the return of plant residues into the soil and potentially build SOC (Powlson et al., 2011). However, if the relationship was simply an effect of greater inputs building SOC, we should not have seen an interaction between SOC and N on yields (because SOC should then just have been additively related to yield). Whatever the specific explanation, the SOC by N interaction we detect suggests that a combination of both building SOM and using targeted N applications could lead to potential increases in yield (Fig. 3). Practices such as cover cropping represent a strategy that can both increase N supply and build SOM through biological N fixation and the return of high-quality residues (narrow C:N ratios) to the soil (Drinkwater et al., 1998). Building SOM and reducing fertilizer N input would require a balance where SOM N mineralization accounts for any limitations in N supply that arise from reducing mineral fertilizer applications. The balance required will depend on the amount and C:N ratios of inputs used in specific agricultural systems and could prove challenging to achieve in some smallholder systems where low SOC concentrations might be compounded by a lack of access to and insufficient quality of organic inputs (Giller et al., 2009; Palm et al., 2001). As such, the combination of both SOM improvement and targeted fertilizer input will likely be especially important for degraded soils, which require a suite of organic and inorganic nutrients to help build SOM and improve crop yields (Palm et al., 1997).
Gains in yield from fertilizer input leveled off at about 200 kg N ha−1 yr−1 (Fig. 3), meaning that optimum yields appear achievable, at least on average, with this fertilizer input level and an SOC target concentration of 2 %. Using this target N input rate, we explored potential fertilizer reductions on agricultural lands using more than 200 kg N ha−1 yr−1. We found that for lands receiving more than 200 kg N ha−1 yr−1, current yields could be maintained while decreasing global N fertilizer inputs by 7 % for maize and 5 % for wheat. It is estimated that 25 % to 30 % of fertilizer N is exported to streams and rivers, resulting in eutrophication (Raymond et al., 2012). Targeted reductions in the application of fertilizer N on the order of magnitude our analysis suggests could then prevent the annual export of as much as 3.73 million tonnes of N into inland waters, which amounts to 10 % of mineral fertilizer applied to maize and wheat lands (see Methods for an explanation of how this percentage was obtained).
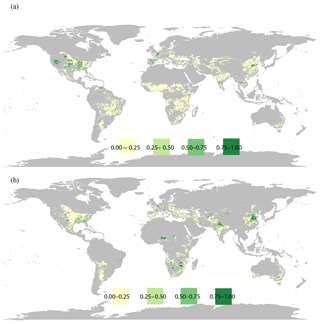
Figure 4Proportion closure of yield gap for (a) maize and (b) wheat given an increase in SOC concentration to target values for each AEZ (ranging from 1 % to 2 %). Modeled gains come from our regression relationship between SOC and yield and applying it to EarthStat yield gap data. Doing so determines the potential increase in yield and therefore projected reductions in yield gaps for maize and wheat.
2.3 Exploring potential reductions in global yield gaps of maize and wheat
With a majority of cultivated lands containing less than 2 % SOC and a growing imperative to build, restore, and protect SOC in agricultural soils (NSTC, 2016; FAO, 2008; NRCS, 2012), we used global gridded datasets coupled with our regression model (Table 1) to examine the potential gains in yield and production if opportunities to increase SOC are realized (Table 2). We then calculated how these gains in production would impact global yield gaps of maize and wheat, the difference between observed and attainable yields (Mueller et al., 2012). Although our model identified 2 % as a global target for SOC, we created regionally specific SOC targets given the fact that achieving 2 % SOC in some soils (e.g., those of drylands) may be unachievable due to inherent constraints of physical soil properties and climate (see Methods). We found that increasing SOC concentrations to the defined targets has the potential capacity to increase average yields on a per hectare basis by 10±11 % (mean ± SD) for maize and 23±37 % for wheat. These gains in yield translate to a 5 % and 10 % increase in the global annual tonnes produced of maize and wheat, respectively (Table 2). These increases in production would close 32 % of the global yield gap for maize and 60 % of the gap for wheat (Fig. 4a, b).
These yield gap results represent an exploration of potential best-case impacts of increasing SOC concentrations. We recognize there are inherent and logistical challenges to building SOM in agricultural soils; and when managing for and building SOM, it is important to account for its dynamic nature. For instance, to derive some of the nutrient benefits of SOM, it must be mineralized and used (Janzen, 2006), and so frequent additions of organic inputs may be necessary to sustain SOM levels. Furthermore, soil characteristics such as texture can have a large effect on SOC content because sandier (rather than more clay rich) soils have less surface area to stabilize SOC (Rasmussen et al., 2018) and so hold much less water and nutrients than clay-rich soils (Johnston et al., 2009). Maintaining SOC contents in sandy soils may require more frequent additions of organic amendments because these soils do not have the surface area to retain nutrients, moisture, and to stabilize SOC (Lehmann and Kleber, 2015).
Different regions and climate types also face different imperatives for building SOM. In the midwestern United States, for instance, building SOM may be a good strategy to reduce fertilizer inputs and irrigation needs, whereas in sub-Saharan Africa, building SOM may be critical for drought protection and nutrient provision. Notably, high SOM values are not common in dryland environments (for our dataset, mean SOC = 0.9 % for dryland climates versus 1.4 % SOC for mesic soils), and building and maintaining SOM in arid zones is typically hindered by the lack of organic matter to return to soils (Rasmussen et al., 1980). On a positive note, however, our analysis suggests that increases in SOC in drylands, for example, from 0.5 % to 0.8 %, could potentially increase yields by 10 %, likely due to impacts on water retention as well as improved nutrient supply.
The goal of our analysis was to establish a global, average relationship between SOC and yield. Whereas we did use lower SOC targets (ranging from 1.0 % to 1.5 %) for the arid agroecological zones (AEZs) in our analysis, the majority of data used for our analysis are from the more temperate and tropical humid zones (Fig. S2 in the Supplement) and a large proportion of our data comes from China (Fig. 5). We recognize that the distribution of our data could potentially bias our results. As such, we explored the SOM–yield relationship in the absence of data from China and also for Chinese observations only. While the effect size of SOC changes depending on the subset of data analyzed (Table S2 in the Supplement), the qualitative patterns of this relationship remain the same. That is, SOC leads to gains in yield that are most pronounced at lower SOC concentrations and decline in their magnitude as ∼2 % SOC is reached (Fig. S3). Notably, when exploring the subset of data from China, the effect size of SOC was higher than that from the entire dataset (Table S2). However, China only had 10 observations above 2 % SOC, and so the modeled relationship for China captures the part of the SOM–yield relationship where an increase in SOC leads to the largest gains in yield (i.e., where the modeled slope is the steepest). Our analysis then highlights both the need for studies to come evenly from systems where maize and wheat are grown and also the importance of analyzing regional datasets that capture the observed range of SOC values in order to quantify a regionally specific relationship between SOC and yield to more directly inform practice.
Moving from the global relationship presented in our paper to bolstering and/or refining SOC targets, our correlative analysis needs to be supplemented with well-replicated experimental studies incorporating different management strategies across multiple soil and climate types to develop SOC–yield relationships that can be applied to the specific set of local farm conditions. Further, these studies should ideally report data related to soil texture and mineralogy, nutrient management, and paired SOC–yield observations with SOC taken to meaningful depths, such as those that represent plant-rooting depth. These experimental studies will help generate information that practitioners can use to inform management by taking into account the potential benefits of SOC, compared against the inherent and logistical challenges to building SOC to target levels.
Despite uncertainties and calls for further research into how SOM affects agricultural performance (Cassman, 1999; Herrick, 2000; Oldfield et al., 2015), policy for sustainable intensification already widely supports the merits of increasing SOM in agricultural lands (FAO, 2008; NRCS, 2012). The purported benefits include improved yields, increased resilience, and decreased inputs of fertilizer and irrigation water. However, although consensus exists around the importance of SOM to soil health, translating SOM policy to practice is hindered by the lack of a predictive capacity for SOM target setting to inform management efforts focused on yield and reducing fertilizer and irrigation (Chabbi et al., 2017; Herrick, 2000; NRC, 2010). Our analysis helps establish a quantitative framework for SOC targets that achieve measurable agricultural outcomes as part of sustainable intensification efforts. It quantifies the potential effect size of SOC on yield while also accounting for climate, soil, and management variables that influence crop yield. We find that greater concentrations of SOC are associated with greater yields up to an SOC concentration of 2 %. With two-thirds of global maize and wheat lands having SOC concentrations of less than 2%, there seems to be significant opportunity to increase SOC to reduce N inputs and potentially help close global yield gaps.
Our approach consisted of a two-stage process. In the first stage, we assembled published empirical data from studies that reported both SOC and yield data for maize and wheat. From this meta-dataset, we then quantified how both SOC concentrations and N input rates are related to yields, in the context of spatial variation in climatic, management, and soil co-variables. In the second stage, we used globally gridded datasets to extract values for the factors we investigated in the first stage for global lands where maize and wheat is produced. Using the regression relationship developed from the published empirical data compiled under the first stage, we then estimated how an increase in SOC concentrations up to target thresholds we identified (ranging from 1 % to 2 % depending on agroecological zoning) affected global yield potentials. Finally, we used an N input threshold identified through our regression analysis (200 kg N ha−1 yr−1) to calculate potential N reductions on global maize and wheat lands.
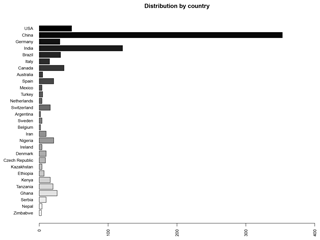
Figure 5Distribution of data points by country. Countries are ordered by gross domestic product (GDP) in order from largest (top) to smallest (bottom). The dataset used for this study contains a total of 840 individual observations from 29 different countries.
4.1 Data collection
In the first stage of our approach, we searched the database Web of Science (Thomson Reuters) in January 2016 and again in October 2016 using the following topic search terms: soil organic matter, soil organic carbon, soil carbon, or soil c; and yield, crop yield, productivity, and agricult*. We restricted the initial search to articles published in English between 1980 and December 2015 and excluded conference proceedings; the second search captured articles published in 2016. The initial search resulted in 1384 articles and the second in 169 articles (Fig. S1). For each citation, we reviewed titles and abstracts to select articles that met the following criteria: experimental field studies whose abstract included information on yield and SOC for systems growing wheat and/or maize. This initial screening resulted in 523 records for which we assessed the full text. We assessed these records for eligibility based on inclusion of data on crop yield, SOC, and N fertilizer rates for each observation. For inclusion within our analysis, it was essential that studies reported paired SOC and yield data. Furthermore, we required SOC concentrations (as opposed to stocks). Studies did not meet our criteria for inclusion if they reported SOC stocks with no corresponding data on bulk density to convert into concentrations and also if they reported baseline SOC concentrations as opposed to experimental SOC concentrations that we could pair with yield data. In addition to our literature search, we also contacted authors to see if they were willing to include raw data within our database. This resulted in three datasets (Adiku et al., 2009; Birkhofer et al., 2008; Kautz et al., 2010). Finally, we consulted the recently published database by the Swedish Board of Agriculture that is a key repository of peer-reviewed literature focusing specifically on studies (735 in total) related to the effects of agricultural management on soil organic carbon (Haddaway et al., 2015). We explored this database to find studies from regions that were underrepresented within our literature search (e.g., the Southern Hemisphere). This resulted in a search of 55 studies to see if they met our criteria for inclusion. We scanned each paper to see if they included SOC data paired with matching yield data. From these papers, we extracted data from 12 studies, which resulted in an additional 52 data points. We encountered limitations similar to our initial search: namely, SOC and yield data were not paired, studies included only baseline SOC concentrations, or SOC stocks were reported without any corresponding bulk density data to convert into concentrations. Overall, our dataset included 840 individual observations from 90 articles covering sites across the globe (Adiku et al., 2009; Agegnehu et al., 2016; Albizua et al., 2015; Alijani et al., 2012; Araya et al., 2012; Atreya et al., 2006; Bai et al., 2009; Bedada et al., 2014; Bhardwaj et al., 2011; Bhattacharyya et al., 2015; Birkhofer et al., 2008; Boddey et al., 2010; Boulal et al., 2012; Bremer et al., 1994; Calegari et al., 2008; Campbell et al., 2007; Castellanos-Navarrete et al., 2012; Celik et al., 2010; Chen et al., 2015; Chirinda et al., 2010; Cid et al., 2014; Costa et al., 2010; D'Hose et al., 2014; Datta et al., 2010; DeMaria et al., 1999; Diacono et al., 2012; Grandy et al., 2006; Guo et al., 2012, 2009; He et al., 2011; Hossain et al., 2016; Hu et al., 2015, 2014; Kaihura et al., 1999; Karbozova Saljnikov et al., 2004; Kautz et al., 2010; Kazemeini et al., 2014; Kucharik et al., 2001; Larsen et al., 2014; Lebbink et al., 1994; Leogrande et al., 2016; Li et al., 2015; E. K. Liu et al., 2014; X. E. Liu et al., 2014; X. Y. Liu et al., 2014; Liu et al., 2016; López-Garrido et al., 2014; Lu et al., 2016; Ma et al., 2012, 2016; Madejón et al., 2001; Mandal et al., 2013; Masto et al., 2007; Mikanová et al., 2012; Mishra et al., 2015; Mupangwa et al., 2013; N'Dayegamiye, 2006; Niu et al., 2011; Njoku and Mbah, 2012; Paul et al., 2013; Qin et al., 2015; Quiroga et al., 2009; Sadeghi and Bahrani, 2009; Saikia et al., 2015; Scalise et al., 2015; Seremesic et al., 2011; Singh and Dwivedi, 2006; Singh et al., 2016; Sisti et al., 2004; Soldevilla-Martinez et al., 2013; Spargo et al., 2011; Šimon et al., 2015; Tejada et al., 2016; Tiecher et al., 2012; van Groenigen et al., 2011; Vieira et al., 2007, 2009; Wang et al., 2015; Q. J. Wang et al., 2014; Z. G. Wang et al., 2014; Wortman et al., 2012; Wu et al., 2015; Yang et al., 2013; J. Yang et al., 2015; Z. C. Yang et al., 2015; Yeboah et al., 2016; Zhang et al., 2015, 2009, 2016; Zhao et al., 2016). Where necessary, we extracted data from manuscript figures using GraphClick software (version 3.0.3, http://www.arizona-software.ch/graphclick/, last access: January, 2018).
Studies that presented individual data points recorded over multiple years were included as well as studies that averaged both yield and SOC data over multiple years. To avoid overrepresentation of studies that included data points recorded for both yield and SOC over multiple years (>10 years), we took observations from the beginning, middle, and last year of the study.
4.2 Data compilation
For each extracted observation, we compiled the following information: latitude, longitude, year of data collection, crop type, yield, SOC or SOM, depth of SOC or SOM measurement, N fertilization rate, P fertilization rate, soil pH, texture, and whether or not crops were irrigated. We used SOC (as opposed to SOM) for our analysis given that SOC is a common proxy for SOM. Carbon, as an element that is easily identified and measured within soil, is thought to comprise ∼50 %–60 % of SOM and is commonly reported in the literature (Pribyl, 2010). When SOM was reported, we converted it to SOC by dividing the value by 1.724 (Cambardella et al., 2001). Different studies reported SOC concentrations to different depths, which ranged from 0–5 to 0–30 cm, with the majority of studies reporting SOC to 0–20 cm. When studies reported SOC to multiple depths, we averaged SOC values across depths to 30 cm. If no information on irrigation was provided, we scored the observation as rain fed. Soil texture and pH were not reported for every study; 79 % of included studies reported pH, and so we used the study's latitude and longitude to extract these data using ISRIC SoilGrids (Hengl et al., 2014) to fill in the missing pH values. Texture was reported for about half (49 %) of included studies, and so we used coordinates to pull these data from SoilGrids as well (Hengl et al., 2014). We also used latitude and longitude to obtain an aridity index through the CGIAR-CSI database (Zomer et al., 2008). We chose to use aridity as our primary climatic variable since it is expressed as a function of precipitation, temperature, and potential evapotranspiration (Trabucco, 2009).
4.3 Data analysis
We used a linear mixed model (LMM) to analyze the observations we extracted from the literature. Our model included SOC, N fertilizer rate, crop type (maize or wheat, coded as a binary variable), irrigation (coded as a binary variable), aridity index, latitude, pH, and texture (% clay) as fixed effects. The differences in soil carbon observed in our dataset are from experimental plots capturing long-term differences in SOC within a given site. Specifically, our data capture differences within SOC largely driven by management interventions related to inputs (e.g., compost, fertilizer, manure, crop residues) and tillage (e.g., no till versus till). Site-specific differences in management as well as spatial and temporal correlation among the studies were accounted for by nesting year within study as random effects (Bolker et al., 2009). The LMMs were fit with a Gaussian error distribution in the “lme4” package for the R statistical program (version 3.3.1), using the “lmer” function. The first stage of our data analysis was to test the data distributions. We removed data points with N fertilization rates >600 kg N ha−1 (four data points) and yields >18 t ha−1 (two data points) since these represented outliers for our dataset (being beyond 3 times the interquartile range of the meta-dataset) and are not representative of on-farm management practices or outcomes. Our final model was based on 834 observations across 90 studies. We added quadratic terms for both SOC and N input rate since these variables exhibited a nonlinear relationship with yield. The square root of the variance inflation factors (vif) was <2 for all factors when included as main effects, indicating that collinearity was low among all variables. As would be expected, there was a correlation between SOC and its quadratic term and between N input rate and its quadratic term. We reran our regression after removing four seemingly influential data points (those that had high SOC concentrations with low yields; see Fig. 1) and model coefficients remained essentially the same. We calculated the r2 values for our model following Nakagawa and Schielzeth (Nakagawa and Schielzeth, 2013) to retain the random effects structure. The r2 of our model was 83 % for the full model, with the fixed effects explaining 42 % of observed variance within our dataset.
We based the choice of factors for inclusion in our model on the approach of Hobbs and Hilborn (2006), by only investigating factors where biological mechanism as to their influence on yield is firmly established and where we were interested in their effect sizes relative to one another. Also following Hobbs and Hilborn (2006), we did not carry out model selection. Operationally, there is substantial subjectivity and lack of agreement in model selection approaches, with different decisions leading to markedly different conclusions as to the influence of different factors. Instead, coefficients are generally most robust when all terms are retained in a model, assuming that the inclusion of each is biologically justified. We decided to include an SOC by N interaction to explore potential reductions in N fertilizer with increased SOC concentrations. This was an effort to see if there is a level of SOC that can compensate for N input. We acknowledge that there are a number of interactions we could have included within our statistical model, and we did run our regression model with additional interactions to include SOC by irrigation, SOC by clay, and SOC by aridity. Including these interactions, however, did not offer any additional explanatory power and our main results between SOC, N inputs, and yield were essentially unchanged with these additional interactions (Table S3). As such, we chose to present our analysis including only the SOC × N interaction.
To examine the effect sizes of the factors on yield, we took two approaches. First, we compared the size of the standardized coefficients, where standardizing involved subtracting the mean of the factor from each observed value and dividing by 2 standard deviations (Gelman, 2008). Dividing by 2 standard deviations is useful when binary predictors are included within regression models (in our case, crop type and irrigation are coded as binary predictors). This way, continuous and binary variables all have a mean of 0 and a standard deviation of 0.5 (Gelman, 2008). This accounts for the fact that the factors were measured on different unit scales (Table 1). Second, we examined the influence of changing SOC concentration or N fertilization rates on yield. To do this, we used the regression relationship derived from our statistical model, held all other factors at a constant value (e.g., the mean of all observations for that factor), and systematically varied SOC or N fertilization across the range of values we extracted from the literature. For SOC, this meant varying SOC values from 0.1 % to 3.5 % to estimate changing yield of rain-fed maize or wheat as SOC concentrations were increased (Fig. 1). For N fertilization, we varied N input rates from 0 to 300 kg N ha−1 for rain-fed maize or wheat at different SOC concentrations (Fig. 3). When these factor–yield relationships were plotted, we identified threshold values where yield became minimally responsive to SOC or N fertilization as the point where the slope of the relationship became <0.25 (for SOC) and <0.002 (for N fertilization).
4.4 Global extrapolations
We used the regression relationship developed in the first stage of our approach to predict how building SOC concentrations would potentially affect global crop yield averages. To obtain values for each of the factors in our regression model at a global scale, we used globally gridded data products. Global SOC, pH, and texture data were taken from ISRIC SoilGrids (Hengl et al., 2014) at a 10 km grid cell resolution to match the spatial grain for maize and wheat yields and N fertilization data, which we obtained from the EarthStat product (Monfreda et al., 2008; Mueller et al., 2012). SoilGrids has multiple layers for SOC concentrations, so we used the 0–15 cm layer because the average depth to which SOC was reported for our dataset was 0–20 cm. The aridity index was obtained from the CGIAR-CSI database (Zomer et al., 2008). We used the resulting global dataset to explore the potential impact of increasing SOC (up to regionally identified threshold levels ranging from 1 % to 2 %) on yield for lands across the globe where maize and wheat are produced.
To establish regionally appropriate SOC targets, we classified maize- and wheat-producing areas by their agroecological zones. The Food and Agricultural Organization has 18 zones defined on the basis of combinations of soil, landform, and climatic characteristics (Ramankutty et al., 2007). For each AEZ, we examined the distribution of SOC in areas classified as naturally vegetated (e.g., not in urban or agricultural land uses). We did this by stacking two GIS raster layers of SOC (SoilGrids) and land use (Friedl et al., 2010), excluding agricultural and urban land use classifications. We then extracted SOC data for each AEZ using a shape file outlining the geographical extent of each AEZ (Ramankutty et al., 2007). Examining the distribution of SOC across each AEZ, we identified targets based on the mean SOC value within each zone. All but four zones had means greater than 2 % SOC, so we set target values for those zones at 2 %. Mean SOC concentrations were lower for the more arid zones and so we set those targets to 1 % for AEZ 1 and 1.5 % for AEZ zones 2, 3, and 7. These targets were in line with recent quantitative assessments based on similar climatic classifications. For instance, recent analysis of global SOC concentrations across globally defined ecoregions shows mean values of SOC at or greater than 2 % for all regions except land classified as desert and xeric shrubland (Stockmann et al., 2015).
Prior to our global extrapolations, we performed a suite of data checks. We wanted to ensure that global yields predicted using our regression model were comparable to those from EarthStat. These checks helped validate the strength of our extrapolations. Firstly, we explored the range of variation in variables from experimental data used to generate our model as well as the range of global variation in variables we project across. The range of our regressors encompasses the range of global variation, except for aridity, in which case 4.6 % percent of our projections fall in grids that have axis conditions outside of our range of measurements. These values fall in extremely arid systems, with aridity values of less than 0.1. In these extremely arid zones, we do make a point to use lower target SOC values, recognizing that achieving 2 % SOC in these very arid areas is not very likely. Secondly, using our regression model to predict global yields for both maize and wheat (separately), we first removed all values from the analysis that had predicted yields of less than 0 because negative yields are not possible. This amounted to 0.004 % of the total predictions for maize and 0.15 % for wheat. For clarification, we refer to predictions from our regression model as predicted or model predicted. We then calculated the proportional difference between model-predicted and globally gridded yield data from EarthStat. We dropped all cells for which the proportional difference between predicted and gridded data was >3 times. This threshold represents the mean ± half of the standard deviation for the distribution of the proportional difference between predicted and EarthStat yield data. This amounted to 14 % of cells for maize and 7 % for wheat. The mean proportional difference between predicted and gridded data was 0.85±0.91 for maize (Fig. S4b) and 0.45±0.87 for wheat (Fig. S5b). The correlation between predicted and gridded data was r=0.73 for maize (Fig. S4c) and r=0.38 for wheat (Fig. S5c). We also visualized an overlap in the distribution of model-predicted and gridded data. Model-predicted maize yield had a global mean of 4.66±1.84 t ha−1 and EarthStat had a global mean of 3.34±2.62 t ha−1 (Fig. S4a). Model-predicted wheat yield had a global mean of 3.18±1.66 t ha−1 and EarthStat had a global mean of 2.43±1.58 t ha−1 (Fig. S5a).
We also compared the distribution of EarthStat yield data with observed yield data from the studies included in our analysis. We found that the correlation (r values) between the gridded and collected data was 0.56 for maize and 0.39 for wheat. Average observed maize yield was 5.61±3.32 t ha−1 and wheat yield was 4.02±2.11 t ha−1 (mean ± SD). EarthStat maize yield, again, was 3.34±2.62 t ha−1 and wheat yield was 2.43±1.58 t ha−1. These differences between predicted and EarthStat yield averages are likely due to the fact that EarthStat data are based on regional census data, incorporating much more variability in terms of management practices and skill than experimental field studies.
After the data checks, we then used our model to extrapolate global yield potentials of maize and wheat given increases in SOC. We masked EarthStat production and cultivated area data layers for maize and wheat for cells that had SoilGrids SOC concentrations of >2 %. We compared the subsetted data (i.e., cultivated lands with <2 % SOC) with the original data layers to determine the fraction of global maize and wheat production and cropland that is on soils with less than 2 % SOC. We used this subsetted data along with our regression model to predict yields at current SOC levels. As stated above, we used EarthStat, ISRIC SoilGrids, and CGIAR-CSI data layers to fill in the values for each of the factors in our regression model. This new data layer was used as a baseline with which to compare to potential gains in yield with an increase to SOC target values. This created a second data layer with model-predicted yields given an increase in SOC. We calculated the percentage increase in yield between these two layers (the baseline and the improved-SOC layer) and multiplied this by EarthStat yield and production data to determine potential gains in maize and wheat yields and production (Table 2). We then used EarthStat yield gap data to see how such an increase in SOC would reduce projected yields gaps. Using the new yield data layer (with yields at SOC target values), we calculated the proportion of EarthStat yield gaps that was reduced for both maize and wheat.
Finally, we used data on global N use (EarthStat) to explore potential reductions in fertilizer use for both maize and wheat, separately. We used a value of 200 kg N ha−1 yr−1 as our N input threshold, as this is the value from our regression model at which gains in yields level off. We created a new data layer for those areas that have N input rates greater than 200 kg N ha−1 yr−1. We then calculated the potential N reductions, in tonnes, by multiplying this new data layer by EarthStat cultivated maize and wheat lands, separately. Finally, we divided the potential reduction in N input (in tonnes) by total N input (in tonnes) as provided through the EarthStat data product.
The dataset generated and analyzed during the current study is available through the KNB repository: https://doi.org/10.5063/F19W0CQ5 (Oldfield et al., 2018).
The supplement related to this article is available online at: https://doi.org/10.5194/soil-5-15-2019-supplement.
EEO, MAB, and SAW conceived the study. EEO and SAW performed data analysis. EEO wrote the first draft of the manuscript. All authors contributed to data interpretation and paper writing.
The authors declare that they have no conflict of interest.
Thanks to Samuel Adiku, Klaus Birhofer, and Tim Kautz for their contributions
of data. Thanks also to the SNAPP working group on “Managing Soil Carbon”
for their support, as well as Deborah Bossio, Indy Burke, Jon Fisher,
Cheryl Palm, Pete Raymond, and the Bradford Lab Group for comments on earlier
drafts.
Edited by:
Cornelia Rumpel
Reviewed by: two anonymous referees
Adhikari, K. and Hartemink, A. E.: Linking soils to ecosystem services – A global review, Geoderma, 262, 101–111, https://doi.org/10.1016/j.geoderma.2015.08.009, 2016.
Adiku, S. G. K., Jones, J. W., Kumaga, F. K., and Tonyigah, A.: Effects of crop rotation and fallow residue management on maize growth, yield and soil carbon in a savannah-forest transition zone of Ghana, J. Agr. Sci., 147, 313–322, https://doi.org/10.1017/s002185960900851x, 2009.
Agegnehu, G., Bass, A. M., Nelson, P. N., and Bird, M. I.: Benefits of biochar, compost and biochar-compost for soil quality, maize yield and greenhouse gas emissions in a tropical agricultural soil, Sci. Total Environ., 543, 295–306, https://doi.org/10.1016/j.scitotenv.2015.11.054, 2016.
Albizua, A., Williams, A., Hedlund, K., and Pascual, U.: Crop rotations including ley and manure can promote ecosystem services in conventional farming systems, Appl. Soil Ecol., 95, 54–61, https://doi.org/10.1016/j.apsoil.2015.06.003, 2015.
Alijani, K., Bahrani, M. J., and Kazemeini, S. A.: Short-term responses of soil and wheat yield to tillage, corn residue management and nitrogen fertilization, Soil Till. Res., 124, 78–82, https://doi.org/10.1016/j.still.2012.05.005, 2012.
Araya, T., Cornelis, W. M., Nyssen, J., Govaerts, B., Getnet, F., Bauer, H., Amare, K., Raes, D., Haile, M., and Deckers, J.: Medium-term effects of conservation agriculture based cropping systems for sustainable soil and water management and crop productivity in the Ethiopian highlands, Field Crop Res., 132, 53–62, https://doi.org/10.1016/j.fcr.2011.12.009, 2012.
Atreya, K., Sharma, S., Bajracharya, R. M., and Rajbhandari, N. P.: Applications of reduced tillage in hills of central Nepal, Soil Till. Res., 88, 16–29, https://doi.org/10.1016/j.still.2005.04.003, 2006.
Bai, Y. H., He, J., Li, H. W., Wang, Q. J., Chen, H., Kuhn, N. J., Hikel, H., Chen, F., and Gong, Y. S.: Soil Structure and Crop Performance After 10 Years of Controlled Traffic and Traditional Tillage Cropping in the Dryland Loess Plateau in China, Soil Sci., 174, 113–119, https://doi.org/10.1097/SS.0b013e3181981ddc, 2009.
Banwart, S. S., Black, H. B., Cai, Z. Z., Gicheru, P. G., Joosten, H. J., Victoria, R. V., Milne, E. E., Noellemeyer, E. N., Pascual, U. P., Nziguheba, G. G., Vargas, R. R., Bationo, A. B., Buschiazzo, D. B., de-Brogniez, D. D., Melillo, J. M., Richter, D. R., Termansen, M. T., van Noordwijk, M. N., Goverse, T. G., Ballabio, C. C., Bhattacharyya, T. B., Goldhaber, M. M., Nikolaidis, N. N., Zhao, Y. Z., Funk, R. F., Duffy, C. C., Pan, G. P., la Scala, N. L., Gottschalk, P. G., Batjes, N. B., Six, J., van Wesemael, B. W., Stocking, M. S., Bampa, F. B., Bernoux, M. B., Feller, C. C., Lemanceau, P. P., and Montanarella, L. L.: Benefits of soil carbon: report on the outcomes of an international scientific committee on problems of the environment rapid assessment workshop, Carbon Manag., 5, 185–192, 2014.
Bauer, A. and Black, A. L.: Organic carbon effects on available water capacity of three soil textural groups, Soil Sci. Soc. Am. J., 56, 248–254, 1992.
Bauer, A. and Black, A. L.: Quantification of the Effect of Soil Organic Matter Content on Soil Productivity, Soil Sci. Soc. Am. J., 58, 185, https://doi.org/10.2136/sssaj1994.03615995005800010027x, 1994.
Bedada, W., Karltun, E., Lemenih, M., and Tolera, M.: Long-term addition of compost and NP fertilizer increases crop yield and improves soil quality in experiments on smallholder farms, Agr. Ecosyst. Environ., 195, 193–201, https://doi.org/10.1016/j.agee.2014.06.017, 2014.
Bhardwaj, A. K., Jasrotia, P., Hamilton, S. K., and Robertson, G. P.: Ecological management of intensively cropped agro-ecosystems improves soil quality with sustained productivity, Agr. Ecosyst. Environ., 140, 419–429, https://doi.org/10.1016/j.agee.2011.01.005, 2011.
Bhattacharyya, R., Das, T. K., Sudhishri, S., Dudwal, B., Sharma, A. R., Bhatia, A., and Singh, G.: Conservation agriculture effects on soil organic carbon accumulation and crop productivity under a rice-wheat cropping system in the western Indo-Gangetic Plains, Eur. J. Agron., 70, 11–21, https://doi.org/10.1016/j.eja.2015.06.006, 2015.
Birkhofer, K., Bezemer, T. M., Bloem, J., Bonkowski, M., Christensen, S., Dubois, D., Ekelund, F., Fließbach, A., Gunst, L., Hedlund, K., Mäder, P., Mikola, J., Robin, C., Setala, H., Tatin-Froux, F., van der Putten, W. H., and Scheu, S.: Long-term organic farming fosters below and aboveground biota: Implications for soil quality, biological control and productivity, Soil Biol. Biochem., 40, 2297–2308, https://doi.org/10.1016/j.soilbio.2008.05.007, 2008.
Boddey, R. M., Jantalia, C. P., Conceia à O, P. C., Zanatta, J. A., Bayer, C. L., Mielniczuk, J. O., Dieckow, J., Santos, dos, H. P., Denardin, J. E., Aita, C., Giacomini, S. J., Alves, B. J. R., and Urquiaga, S.: Carbon accumulation at depth in Ferralsols under zero-till subtropical agriculture, Glob. Change Biol., 16, 784–795, https://doi.org/10.1111/j.1365-2486.2009.02020.x, 2010.
Bolker, B. M., Brooks, M. E., Clark, C. J., Geange, S. W., Poulsen, J. R., Stevens, M. H. H., and White, J.-S. S.: Generalized linear mixed models: a practical guide for ecology and evolution, Trends Ecol. Evol., 24, 127–135, https://doi.org/10.1016/j.tree.2008.10.008, 2009.
Boulal, H., Gomez-Macpherson, H., and Villalobos, F. J.: Permanent bed planting in irrigated Mediterranean conditions: Short-term effects on soil quality, crop yield and water use efficiency, Field Crop Res., 130, 120–127, https://doi.org/10.1016/j.fcr.2012.02.026, 2012.
Bremer, E., Janzen, H. H., and Johnston, A. M.: Sensitivity of total, light fraction and mineralizable organic matter to management practices in a Lethbridge soil, Can. J. Soil Sci., 74, 131–138, 1994.
Calegari, A., Hargrove, W. L., Rheinheimer, D. D. S., Ralisch, R., Tessier, D., de Tourdonnet, S., and de Fatima Guimarães, M.: Impact of Long-Term No-Tillage and Cropping System Management on Soil Organic Carbon in an Oxisol: A Model for Sustainability, Agron. J., 100, 1013–1017, https://doi.org/10.2134/agronj2007.0121, 2008.
Cambardella, C. A., Gajda, A. M., Doran, J. W., Wienhold, B. J., and Kettler, T. A.: Assessment Methods for Soil Carbon, edited by: Lal, R., Kimble, J. M., Follett, R. F., and Stewart, B. A., CRC Press, Boca Raton, 2001.
Campbell, C. A., VandenBygaart, A. J., Zentner, R. P., McConkey, B. G., Smith, W., Lemke, R., Grant, B., and Jefferson, P. G.: Quantifying carbon sequestration in a minimum tillage crop rotation study in semiarid southwestern Saskatchewan, Can. J. Soil Sci., 87, 235–250, 2007.
Cassman, K. G.: Ecological intensification of cereal production systems: Yield potential, soil quality, and precision agriculture, P. Natl. Acad. Sci. USA, 96, 5952–5959, https://doi.org/10.1073/pnas.96.11.5952, 1999.
Castellano, M. J., Mueller, K. E., Olk, D. C., Sawyer, J. E., and Six, J.: Integrating plant litter quality, soil organic matter stabilization, and the carbon saturation concept, Glob. Change Biol., 21, 1–10, https://doi.org/10.1111/gcb.12982, 2015.
Castellanos-Navarrete, A., Rodríguez-Aragonés, C., De Goede, R. G. M., Kooistra, M. J., Sayre, K. D., Brussaard, L., and Pulleman, M. M.: Earthworm activity and soil structural changes under conservation agriculture in central Mexico, Soil Till. Res., 123, 61–70, https://doi.org/10.1016/j.still.2012.03.011, 2012.
Celik, I., Gunal, H., Budak, M., and Akpinar, C.: Effects of long-term organic and mineral fertilizers on bulk density and penetration resistance in semi-arid Mediterranean soil conditions, Geoderma, 160, 236–243, https://doi.org/10.1016/j.geoderma.2010.09.028, 2010.
Chabbi, A., Lehmann, J., Ciais, P., Loescher, H. W., Cotrufo, M. F., Don, A., SanClements, M., Schipper, L., Six, J., Smith, P., and Rumpel, C.: Aligning agriculture and climate policy, Nature Clim. Change, 7, 307–309, 2017.
Chen, H. X., Zhao, Y., Feng, H., Li, H. J., and Sun, B. H.: Assessment of climate change impacts on soil organic carbon and crop yield based on long-term fertilization applications in Loess Plateau, China, Plant Soil, 390, 401–417, https://doi.org/10.1007/s11104-014-2332-1, 2015.
Chirinda, N., Olesen, J. E., Porter, J. R., and Schjønning, P.: Soil properties, crop production and greenhouse gas emissions from organic and inorganic fertilizer-based arable cropping systems, Agr. Ecosyst. Environ., 139, 584–594, https://doi.org/10.1016/j.agee.2010.10.001, 2010.
Cid, P., Carmona, I., Murillo, J. M., and Gomez-Macpherson, H.: No-tillage permanent bed planting and controlled traffic in a maize-cotton irrigated system under Mediterranean conditions: Effects on soil compaction, crop performance and carbon sequestration, Eur. J. Agron., 61, 24–34, https://doi.org/10.1016/j.eja.2014.08.002, 2014.
Costa, S. E. V. G. A., Souza, E. D., Anghinoni, I., Flores, J. P. C., Vieira, F. C. B., Martins, A. P., and Ferreira, E. V. O.: Patterns in phosphorus and corn root distribution and yield in long-term tillage systems with fertilizer application, Soil Till. Res., 109, 41–49, https://doi.org/10.1016/j.still.2010.04.003, 2010.
Culman, S. W., Snapp, S. S., Green, J. M., and Gentry, L. E.: Short- and long-term labile soil carbon and nitrogen dynamics reflect management and predict corn agronomic performance, Agron. J., 105, 493–502, https://doi.org/10.2134/agronj2012.0382, 2013.
Datta, S. P., Rattan, R. K., and Chandra, S.: Labile soil organic carbon, soil fertility, and crop productivity as influenced by manure and mineral fertilizers in the tropics, J. Plant Nutr. Soil Sc., 173, 715–726, https://doi.org/10.1002/jpln.200900010, 2010.
DeMaria, I. C., Nnabude, P. C., and de Castro, O. M.: Long-term tillage and crop rotation effects on soil chemical properties of a Rhodic Ferralsol in southern Brazil, Soil Till. Res., 51, 71–79, https://doi.org/10.1016/S0167-1987(99)00025-2, 1999.
de Moraes Sa, J. C., Tivet, F., Lal, R., Briedis, C., Hartman, D. C., Santos, dos, J. Z., and dos Santos, J. B.: Long-term tillage systems impacts on soil C dynamics, soil resilience and agronomic productivity of a Brazilian Oxisol, Soil Till. Res., 136, 38–50, https://doi.org/10.1016/j.still.2013.09.010, 2014.
D'Hose, T., Cougnon, M., De Vliegher, A., Vandecasteele, B., Viaene, N., Cornelis, W., Van Bockstaele, E., and Reheul, D.: The positive relationship between soil quality and crop production: A case study on the effect of farm compost application, Appl. Soil Ecol., 75, 189–198, https://doi.org/10.1016/j.apsoil.2013.11.013, 2014.
Diacono, M., Ferri, D., Ciaccia, C., Tittarelli, F., Ceglie, F., Verrastro, V., Ventrella, D., Vitti, C., and Montemurro, F.: Bioassays and application of olive pomace compost on emmer: effects on yield and soil properties in organic farming, Acta. Agr. Scand. B-S. P., 62, 510–518, https://doi.org/10.1080/09064710.2012.663785, 2012.
Drinkwater, L. E., Wagoner, P., and Sarrantonio, M.: Legume-based cropping systems have reduced carbon and nitrogen losses, Nature, 396, 262–265, 1998.
Edmeades, D. C.: The long-term effects of manures and fertilisers on soil productivity and quality: a review, Nutr. Cycl. Agroecosys., 66, 165–180, 2003.
FAO: Underpinning conservation agriculture's benefits: the roots of soil health and function, Food and Agriculture Organization of the United Nations, Rome, 2008.
Foley, J. A., Ramankutty, N., Brauman, K. A., Cassidy, E. S., Gerber, J. S., Johnston, M., Mueller, N. D., O'Connell, C., Ray, D. K., West, P. C., Balzer, C., Bennett, E. M., Carpenter, S. R., Hill, J., Monfreda, C., Polasky, S., Rockström, J., Sheehan, J., Siebert, S., Tilman, D., and Zaks, D. P. M.: Solutions for a cultivated planet, Nature, 478, 337–342, https://doi.org/10.1038/nature10452, 2011.
Friedl, M. A., Sulla-Menashe, D., Tan, B., Schneider, A., Ramankutty, N., Sibley, A., and Huang, X.: MODIS Collection 5 global land cover: Algorithm refinements and characterization of new datasets, Remote Sens. Environ., 114, 168–182, https://doi.org/10.1016/j.rse.2009.08.016, 2010.
Gelman, A.: Scaling regression inputs by dividing by two standard deviations, Stat. Med., 27, 2865–2873, https://doi.org/10.1002/sim.3107, 2008.
Giller, K. E., Witter, E., Corbeels, M., and Tittonell, P.: Conservation agriculture and smallholder farming in Africa: The heretics' view, Field Crop Res., 114, 23–34, 2009.
Godfray, H. C. J., Beddington, J. R., Crute, I. R., Haddad, L., Lawrence, D., Muir, J. F., Pretty, J., Robinson, S., Thomas, S. M., and Toulmin, C.: Food Security: The Challenge of Feeding 9 Billion People, Science, 327, 812–818, https://doi.org/10.1126/science.1185383, 2010.
Grandy, A. S., Loecke, T. D., Parr, S., and Robertson, G. P.: Long-term trends in nitrous oxide emissions, soil nitrogen, and crop yields of till and no-till cropping systems, J. Environ. Qual., 35, 1487–1495, https://doi.org/10.2134/jeq2005.0166, 2006.
Guo, S. L., Wu, J. S., Coleman, K., Zhu, H. H., Li, Y., and Liu, W. Z.: Soil organic carbon dynamics in a dryland cereal cropping system of the Loess Plateau under long-term nitrogen fertilizer applications, Plant Soil, 353, 321–332, https://doi.org/10.1007/s11104-011-1034-1, 2012.
Guo, Z. L., Cai, C. F., Li, Z. X., Wang, T. W., and Zheng, M. J.: Crop residue effect on crop performance, soil N2O and CO2 emissions in alley cropping systems in subtropical China, Agroforest. Sys., 76, 67–80, https://doi.org/10.1007/s10457-008-9170-1, 2009.
Haddaway, N. R., Hedlund, K., Jackson, L. E., Kätterer, T., Lugato, E., Thomsen, I. K., Jørgensen, H. B., and Söderström, B.: What are the effects of agricultural management on soil organic carbon in boreo-temperate systems?, Environmental Evidence, 4, 23, https://doi.org/10.1186/s13750-015-0049-0, 2015.
Hatfield, J. L., Sauer, T. J., and Cruse, R. M.: Soil: The Forgotten Piece of the Water, Food, Energy Nexus, Adv. Agron., 143, 1–46, https://doi.org/10.1016/bs.agron.2017.02.001, 2017.
He, J., Li, H. W., Rasaily, R. G., Wang, Q. J., Cai, G. H., Su, Y. B., Qiao, X. D., and Liu, L. J.: Soil properties and crop yields after 11 years of no tillage farming in wheat-maize cropping system in North China Plain, Soil Till. Res., 113, 48–54, https://doi.org/10.1016/j.still.2011.01.005, 2011.
Hengl, T., de Jesus, J. M., MacMillan, R. A., Batjes, N. H., Heuvelink, G. B. M., Ribeiro, E., Samuel-Rosa, A., Kempen, B., Leenaars, J. G. B., Walsh, M. G., and Gonzalez, M. R.: SoilGrids1km – Global Soil Information Based on Automated Mapping, edited by B. Bond-Lamberty, Plos One, 9, e105992, https://doi.org/10.1371/journal.pone.0105992, 2014.
Herrick, J. E.: Soil quality: an indicator of sustainable land management?, Appl. Soil Ecol., 15, 75–83, 2000.
Hijbeek, R., van Ittersum, M. K., ten Berge, H. F. M., Gort, G., Spiegel, H., and Whitmore, A. P.: Do organic inputs matter – a meta-analysis of additional yield effects for arable crops in Europe, Plant Soil, 411, 293–303, https://doi.org/10.1007/s11104-016-3031-x, 2017.
Hobbs, N. T. and Hilborn, R.: Alternatives to statistical hypothesis testing in ecology: A guide to self teaching, Ecol. Appl., 16, 5–19, 2006.
Hossain, M. S., Hossain, A., Sarkar, M. A. R., Jahiruddin, M., da Silva, J. A. T., and Hossain, M. I.: Productivity and soil fertility of the rice-wheat system in the High Ganges River Floodplain of Bangladesh is influenced by the inclusion of legumes and manure, Agr. Ecosyst. Environ., 218, 40–52, https://doi.org/10.1016/j.agee.2015.11.017, 2016.
Hu, C., Li, S. L., Qiao, Y., Liu, D. H., and Chen, Y. F.: Effects of 30 years repeated fertilizer applications on soil properties, microbes and crop yields in rice-wheat copping systems, Exp. Agr., 51, 355–369, https://doi.org/10.1017/s0014479714000350, 2015.
Hu, W. G., Jiao, Z. F., Wu, F. S., Liu, Y. J., Dong, M. X., Ma, X. J., Fan, T. L., An, L. Z., and Feng, H. Y.: Long-term effects of fertilizer on soil enzymatic activity of wheat field soil in Loess Plateau, China, Ecotoxicology, 23, 2069–2080, https://doi.org/10.1007/s10646-014-1329-0, 2014.
Janzen, H. H.: The soil carbon dilemma: Shall we hoard it or use it?, Soil Biol. Biochem., 38, 419–424, https://doi.org/10.1016/j.soilbio.2005.10.008, 2006.
Johnston, A. E., Poulton, P. R., and Coleman, K.: Soil Organic Matter: Its Importance in Sustainable Agriculture and Carbon Dioxide Fluxes, Adv. Agron., 101, 1–57, https://doi.org/10.1016/S0065-2113(08)00801-8, 2009.
Kaihura, F. B. S., Kullaya, I. K., Kilasara, M., Aune, J. B., Singh, B. R., and Lal, R.: Soil quality effects of accelerated erosion and management systems in three eco-regions of Tanzania, Soil Till. Res., 53, 59–70, https://doi.org/10.1016/s0167-1987(99)00077-x, 1999.
Karbozova Saljnikov, E., Funakawa, S., Akhmetov, K., and Kosaki, T.: Soil organic matter status of Chernozem soil in North Kazakhstan: effects of summer fallow, Soil Biol. Biochem., 36, 1373–1381, https://doi.org/10.1016/j.soilbio.2004.02.027, 2004.
Kautz, T., Stumm, C., Kösters, R., and Köpke, U.: Effects of perennial fodder crops on soil structure in agricultural headlands, J. Plant Nutr. Soil Sc., 173, 490–501, https://doi.org/10.1002/jpln.200900216, 2010.
Kazemeini, S. A., Bahrani, M. J., Pirasteh-Anosheh, H., and Momeni, S. M. M.: Maize growth and yield as affected by wheat residues and irrigation management in a no-tillage system, Arch. Agron. Soil Sci., 60, 1543–1552, https://doi.org/10.1080/03650340.2014.896457, 2014.
Kemper, W. D. and Koch, E. J.: Aggregate stability of soils from Western United States and Canada, United States Department of Agriculture, Washington, DC, 1966.
Kravchenko, A. N. and Bullock, D. G.: Correlation of corn and soybean grain yield with topography and soil properties, Agron. J., 92, 75–83, https://doi.org/10.2134/agronj2000.92175x, 2000.
Kucharik, C. J., Brye, K. R., Norman, J. M., Foley, J. A., Gower, S. T., and Bundy, L. G.: Measurements and modeling of carbon and nitrogen cycling in agroecosystems of southern Wisconsin: Potential for SOC sequestration during the next 50 years, Ecosystems, 4, 237–258, https://doi.org/10.1007/s10021-001-0007-2, 2001.
Lal, R.: Soil Carbon Sequestration Impacts on Global Climate Change and Food Security, Science, 304, 1623–1627, https://doi.org/10.1126/science.1097396, 2004.
Larsen, E., Grossman, J., Edgell, J., Hoyt, G., Osmond, D., and Hu, S. J.: Soil biological properties, soil losses and corn yield in long-term organic and conventional farming systems, Soil Till. Res., 139, 37–45, https://doi.org/10.1016/j.still.2014.02.002, 2014.
Lebbink, G., Vanfaassen, H. G., Vanouwerkerk, C., and Brussaard, L.: The Dutch Programme on Soil Ecology of Arable Farming Systems: Farm management monitoring program and general results, Agr. Ecosyst. Environ., 51, 7–20, https://doi.org/10.1016/0167-8809(94)90032-9, 1994.
Lehmann, J. and Kleber, M.: The contentious nature of soil organic matter, Nature, 528, 60–68, https://doi.org/10.1038/nature16069, 2015.
Leogrande, R., Vitti, C., Stellacci, A. M., Cocozza, C., and Ventrella, D.: Response of wheat crop during transition to organic system under Mediterranean conditions, Int. J. Plant Prod., 10, 565–577, 2016.
Li, Z. T., Yang, J. Y., Drury, C. F., and Hoogenboom, G.: Evaluation of the DSSAT-CSM for simulating yield and soil organic C and N of a long-term maize and wheat rotation experiment in the Loess Plateau of Northwestern China, Agr. Syst., 135, 90–104, https://doi.org/10.1016/j.agsy.2014.12.006, 2015.
Liu, E. K., Teclemariam, S. G., Yan, C. R., Yu, J. M., Gu, R. S., Liu, S., He, W. Q., and Liu, Q.: Long-term effects of no-tillage management practice on soil organic carbon and its fractions in the northern China, Geoderma, 213, 379–384, https://doi.org/10.1016/j.geoderma.2013.08.021, 2014.
Liu, H. T., Meng, J., Bo, W. J., Cheng, D., Li, Y., Guo, L. Y., Li, C. H., Zheng, Y. H., Liu, M. Z., Ning, T. Y., Wu, G. L., Yu, X. F., Feng, S. F., Tana, W. Y., Li, J., Li, L. J., Zeng, Y., Liu, S. V., and Jiang, G. M.: Biodiversity management of organic farming enhances agricultural sustainability, Sci. Rep., 6, 23816, https://doi.org/10.1038/srep23816, 2016.
Liu, X. E., Li, X. G., Hai, L., Wang, Y. P., Fu, T. T., Turner, N. C., and Li, F. M.: Film-Mulched Ridge-Furrow Management Increases Maize Productivity and Sustains Soil Organic Carbon in a Dryland Cropping System, Soil Sci. Soc. Am. J., 78, 1434–1441, https://doi.org/10.2136/sssaj2014.04.0121, 2014.
Liu, X. Y., Ye, Y. X., Liu, Y. M., Zhang, A., Zhang, X. H., Li, L. Q., Pan, G. X., Kibue, G. W., Zheng, J. F., and Zheng, J. W.: Sustainable biochar effects for low carbon crop production: A 5-crop season field experiment on a low fertility soil from Central China, Agr. Syst., 129, 22–29, https://doi.org/10.1016/j.agsy.2014.05.008, 2014.
López-Garrido, R., Madejón, E., León-Camacho, M., Girón, I., Moreno, F., and Murillo, J. M.: Reduced tillage as an alternative to no-tillage under Mediterranean conditions: A case study, Soil Till. Res., 140, 40–47, https://doi.org/10.1016/j.still.2014.02.008, 2014.
Loveland, P. and Webb, J.: Is there a critical level of organic matter in the agricultural soils of temperate regions: A review, Soil Till. Res., 70, 1–18, 2003.
Lu, X. L., Lu, X. N., Tanveer, S. K., Wen, X. X., and Liao, Y. C.: Effects of tillage management on soil CO2 emission and wheat yield under rain-fed conditions, Soil Res., 54, 38–48, https://doi.org/10.1071/sr14300, 2016.
Lucas, S. T. and Weil, R. R.: Can a Labile Carbon Test be Used to Predict Crop Responses to Improve Soil Organic Matter Management?, Agron. J., 104, 1160–1170, https://doi.org/10.2134/agronj2011.0415, 2012.
Ma, Q., Yu, W. T., Jiang, C. M., Zhou, H., and Xu, Y. G.: The influences of mineral fertilization and crop sequence on sustainability of corn production in northeastern China, Agr. Ecosyst. Environ., 158, 110–117, https://doi.org/10.1016/j.agee.2012.05.023, 2012.
Ma, Z. M., Chen, J., Lyu, X. D., Liu, L. L., and Siddique, K. H. M.: Distribution of soil carbon and grain yield of spring wheat under a permanent raised bed planting system in an arid area of northwest China, Soil Till. Res., 163, 274–281, https://doi.org/10.1016/j.still.2016.05.010, 2016.
Madejón, E., Lopes, R., Murillo, J. M., and Cabrera, F.: Agricultural use of three (sugar-beet) vinasse composts: effect on crops and chemical properties of a Cambisol soil in the Guadalquivir river valley (SW Spain), Agr. Ecosyst. Environ., 84, 55–65, https://doi.org/10.1016/s0167-8809(00)00191-2, 2001.
Majumder, B., Mandal, B., and Bandyopadhyay, P. K.: Soil organic carbon pools and productivity in relation to nutrient management in a 20-year-old rice-berseem agroecosystem, Biol. Fert. Soils, 44, 451–461, 2008.
Mandal, N., Dwivedi, B. S., Meena, M. C., Singh, D., Datta, S. P., Tomar, R. K., and Sharma, B. M.: Effect of induced defoliation in pigeonpea, farmyard manure and sulphitation pressmud on soil organic carbon fractions, mineral nitrogen and crop yields in a pigeonpea-wheat cropping system, Field Crop Res., 154, 178–187, https://doi.org/10.1016/j.fcr.2013.08.007, 2013.
Masto, R. E., Chhonkar, P. K., Singh, D., and Patra, A. K.: Soil quality response to long-term nutrient and crop management on a semi-arid Inceptisol, Agr. Ecosyst. Environ., 118, 130–142, https://doi.org/10.1016/j.agee.2006.05.008, 2007.
Mikanová, O., Šimon, T., and Javůrek, M.: Relationships between winter wheat yields and soil carbon under various tillage systems, https://doi.org/10.17221/512/2012-PSE, 2012.
Minasny, B., Malone, B. P., McBratney, A. B., Angers, D. A., Arrouays, D., Chambers, A., Chaplot, V., Chen, Z.-S., Cheng, K., Das, B. S., Field, D. J., Gimona, A., Hedley, C. B., Hong, S. Y., Mandal, B., Ben P Marchant, Martin, M., McConkey, B. G., Mulder, V. L., O'Rourke, S., Richer-de-Forges, A. C., Odeh, I., Padarian, J., Paustian, K., Pan, G., Poggio, L., Savin, I., Stolbovoy, V., Stockmann, U., Sulaeman, Y., Tsui, C.-C., Vågen, T.-G., van Wesemael, B., and Winowiecki, L.: Soil carbon 4 per mille, Geoderma, 292, 59–86, https://doi.org/10.1016/j.geoderma.2017.01.002, 2017.
Mishra, V. K., Srivastava, S., Bhardwaj, A. K., Sharma, D. K., Singh, Y. P., and Nayak, A. K.: Resource conservation strategies for rice-wheat cropping systems on partially reclaimed sodic soils of the Indo-Gangetic region, and their effects on soil carbon, Nat. Resour. Forum, 39, 110–122, https://doi.org/10.1111/1477-8947.12071, 2015.
Monfreda, C., Ramankutty, N., and Foley, J. A.: Farming the planet: 2. Geographic distribution of crop areas, yields, physiological types, and net primary production in the year 2000, Global Biogeochem. Cy., 22, GB1022, https://doi.org/10.1029/2007GB002947, 2008.
Mueller, N. D., Gerber, J. S., Johnston, M., Ray, D. K., Ramankutty, N., and Foley, J. A.: Closing yield gaps through nutrient and water management, Nature, 490, 254–257, https://doi.org/10.1038/nature11420, 2012.
Mupangwa, W., Twomlow, S., and Walker, S.: Cumulative effects of reduced tillage and mulching on soil properties under semi-arid conditions, J. Arid Environ., 91, 45–52, https://doi.org/10.1016/j.jaridenv.2012.11.007, 2013.
Nakagawa, S. and Schielzeth, H.: A general and simple method for obtaining R2 from generalized linear mixed-effects models, in: Methods in Ecology and Evolution, edited by: O'Hara, R. B., 4, 133–142, https://doi.org/10.1111/j.2041-210x.2012.00261.x, 2013.
N'Dayegamiye, A.: Mixed paper mill sludge effects on corn yield, nitrogen efficiency, and soil properties, Agron. J., 98, 1471–1478, https://doi.org/10.2134/agronj2005.0339, 2006.
Niu, L. A., Hao, J. M., Zhang, B. Z., and Niu, X. S.: Influences of long-term fertilizer and tillage management on soil fertility of the North China plain, Pedosphere, 21, 813–820, 2011.
Njoku, C. and Mbah, C. N.: Effect of burnt and unburnt rice husk dust on maize yield and soil physico-chemical properties of an ultisol in Nigeria, Biological Agriculture and Horticulture, 28, 49–60, https://doi.org/10.1080/01448765.2012.664374, 2012.
NRC: Understanding agricultural sustainability, in Toward Sustainable Agricultural Systems in the 21st Century, 1–29, National Academies Press, Washington, DC, 2010.
NRCS: Farming in the 21st century: a practical approach to improve soil health, USDA, Natural Resources Conservation Service, Washington, DC, 2012.
NSTC (National Science and Technology Council): The State and Future of U.S. Soils, Washington DC, available at: https://obamawhitehouse.archives.gov/sites/default/files/microsites/ostp/ssiwg_framework_december_2016.pdf (last access 20 December 2018), 2016.
Oelofse, M., Markussen, B., Knudsen, L., Schelde, K., Olesen, J. E., Jensen, L. S., and Bruun, S.: Do soil organic carbon levels affect potential yields and nitrogen use efficiency? An analysis of winter wheat and spring barley field trials, Eur. J. Agron., 66, 62–73, https://doi.org/10.1016/j.eja.2015.02.009, 2015.
Oldfield, E. E., Wood, S. A., Palm, C. A., and Bradford, M. A.: How much SOM is needed for sustainable agriculture?, Front. Ecol. Environ., 13, 527–527, 2015.
Oldfield, E. E., Wood, S. A., and Bradford, M. A.: Direct effects of soil organic matter on productivity mirror those observed with organic amendments, Plant Soil, 348, 1–11, https://doi.org/10.1007/s11104-017-3513-5, 2017.
Oldfield, E. E., Bradford, M. A., and Wood, S. A.: Yield and SOC data from published studies, https://doi.org/10.5063/F19W0CQ5, 2018.
Palm, C. A., Myers, R. J. K., and Nandwa, S. M.: Combined Use of Organic and Inorganic Nutrient Sources for Soil Fertility Maintenance and Replenishment, Replenishing Soil Fertility in Africa, SSSA Special Publication, 51, 193–217, https://doi.org/10.2136/sssaspecpub51.c8, 1997.
Palm, C. A., Giller, K. E., Mafongoya, P. L., and Swift, M. J.: Management of organic matter in the tropics: translating theory into practice, Nutr. Cycl. Agroecosys., 61, 63–75, https://doi.org/10.1023/A:1013318210809, 2001.
Pan, G., Smith, P., and Pan, W.: The role of soil organic matter in maintaining the productivity and yield stability of cereals in China, Agr. Ecosyst. Environ., 129, 344–348, https://doi.org/10.1016/j.agee.2008.10.008, 2009.
Paul, B. K., Vanlauwe, B., Ayuke, F., Gassner, A., Hoogmoed, M., Hurisso, T. T., Koala, S., Lelei, D., Ndabamenye, T., Six, J., and Pulleman, M. M.: Medium-term impact of tillage and residue management on soil aggregate stability, soil carbon and crop productivity, Agr. Ecosyst. Environ., 164, 14–22, https://doi.org/10.1016/j.agee.2012.10.003, 2013.
Poulton, P., Johnston, J., Macdonald, A., White, R., and Powlson, D.: Major limitations to achieving “4 per 1000” increases in soil organic carbon stock in temperate regions: Evidence from long-term experiments at Rothamsted Research, United Kingdom, Glob. Change Biol., 24, 2563–2584, https://doi.org/10.1111/gcb.14066, 2018.
Powlson, D. S., Whitmore, A. P., and Goulding, K. W. T.: Soil carbon sequestration to mitigate climate change: a critical re-examination to identify the true and the false, Eur. J. Soil Sci., 62, 42–55, https://doi.org/10.1111/j.1365-2389.2010.01342.x, 2011.
Pribyl, D. W.: A critical review of the conventional SOC to SOM conversion factor, Geoderma, 156, 75–83, https://doi.org/10.1016/j.geoderma.2010.02.003, 2010.
Qin, W., Wang, D. Z., Guo, X. S., Yang, T. M., and Oenema, O.: Productivity and sustainability of rainfed wheat-soybean system in the North China Plain: results from a long-term experiment and crop modelling, Sci. Rep., 5, 17514, https://doi.org/10.1038/srep17514, 2015.
Quiroga, A., Fernández, R., and Noellemeyer, E.: Grazing effect on soil properties in conventional and no-till systems, Soil Till. Res., 105, 164–170, https://doi.org/10.1016/j.still.2009.07.003, 2009.
Ramankutty, N., Hertel, T., Lee, H. L., and Rose, S. K.: Global Agricultural Land Use Data Global Agricultural Land Use Data for Integrated Assessment Modeling, in: Human-induced climate change: An interdisciplinary assessment, Cambridge University Press, New York, 2007.
Rasmussen, C., Heckman, K., Wieder, W. R., Keiluweit, M., Lawrence, C. R., Berhe, A. A., Blankinship, J. C., Crow, S. E., Druhan, J. L., Pries, C. E. H., Marin-Spiotta, E., Plante, A. F., Schädel, C., Schimel, J. P., Sierra, C. A., Thompson, A., and Wagai, R.: Beyond clay: towards an improved set of variables for predicting soil organic matter content, Biogeochemistry, 137, 297–306, https://doi.org/10.1007/s10533-018-0424-3, 2018.
Rasmussen, P. E., Allmaras, R. R., Rohde, C. R., and Roager, N. C.: Crop Residue Influences on Soil Carbon and Nitrogen in a Wheat-Fallow System1, Soil Sci. Soc. Am. J., 44, 596, https://doi.org/10.2136/sssaj1980.03615995004400030033x, 1980.
Raymond, P. A., David, M. B., and Saiers, J. E.: The impact of fertilization and hydrology on nitrate fluxes from Mississippi watersheds, Curr. Opin. Env. Sust., 4, 212–218, https://doi.org/10.1016/j.cosust.2012.04.001, 2012.
Reeves, D. W.: The role of soil organic matter in maintaining soil quality in continuous cropping systems, Soil Till. Res., 43, 131–167, 1997.
Reeves, M., Lal, R., Logan, T., and Sigarán, J.: Soil nitrogen and carbon response to maize cropping system, nitrogen source, and tillage, Soil Sci. Soc. Am. J., 61, 1387–1392, 1997.
Robertson, G. P., Gross, K. L., Hamilton, S. K., Landis, D. A., Schmidt, T. M., Snapp, S. S., and Swinton, S. M.: Farming for Ecosystem Services: An Ecological Approach to Production Agriculture, Bioscience, 64, 404–415, https://doi.org/10.1093/biosci/biu037, 2014.
Sadeghi, H. and Bahrani, M. J.: Effects of crop residue and nitrogen rates on yield and yield components of two dryland wheat (Triticum aestivum L.) cultivars, Plant Prod. Sci., 12, 497–502, 2009.
Saikia, P., Bhattacharya, S. S., and Baruah, K. K.: Organic substitution in fertilizer schedule: Impacts on soil health, photosynthetic efficiency, yield and assimilation in wheat grown in alluvial soil, Agr. Ecosyst. Environ., 203, 102–109, https://doi.org/10.1016/j.agee.2015.02.003, 2015.
Sanderman, J., Hengl, T., and Fiske, G. J.: Soil carbon debt of 12,000 years of human land use, P. Natl. Acad. Sci. USA, 114, 9575–9580, https://doi.org/10.1073/pnas.1706103114, 2017.
Scalise, A., Tortorella, D., Pristeri, A., Petrovičová, B., Gelsomino, A., Lindström, K., and Monti, M.: Legume-barley intercropping stimulates soil N supply and crop yield in the succeeding durum wheat in a rotation under rainfed conditions, Soil Biol. Biochem., 89, 150–161, https://doi.org/10.1016/j.soilbio.2015.07.003, 2015.
Seremesic, S., Milosev, D., Djalovic, I., Zeremski, T., and Ninkov, J.: Management of soil organic carbon in maintaining soil productivity and yield stability of winter wheat, Plant Soil Environ., 57, 216–221, 2011.
Šimon, T., Kunzová, E., and Friedlová, M.: The effect of digestate, cattle slurry and mineral fertilization on the winter wheat yield and soil quality parameters, Plant Soil Environ., 62, 522–527, 2015.
Singh, V. K. and Dwivedi, B. S.: Yield and nitrogen use efficiency in wheat, and soil fertility status as influenced by substitution of rice with pigeon pea in a rice-wheat cropping system, Aust. J. Exp. Agr., 46, 1185–1194, https://doi.org/10.1071/ea04046, 2006.
Singh, V. K., Yadvinder-Singh, Dwivedi, B. S., Singh, S. K., Majumdar, K., Jat, M. L., Mishra, R. P., and Rani, M.: Soil physical properties, yield trends and economics after five years of conservation agriculture based rice-maize system in north-western India, Soil Till. Res., 155, 133–148, https://doi.org/10.1016/j.still.2015.08.001, 2016.
Sisti, C. P. J., Santos, dos, H. P., Kohhann, R., Alves, B. J. R., Urquiaga, S., and Boddey, R. M.: Change in carbon and nitrogen stocks in soil under 13 years of conventional or zero tillage in southern Brazil, Soil Till. Res., 76, 39–58, https://doi.org/10.1016/j.still.2003.08.007, 2004.
Soldevilla-Martinez, M., Martin-Lammerding, D., Tenorio, J. L., Walter, I., Quemada, M., and Lizaso, J. I.: Simulating improved combinations tillage-rotation under dryland conditions, Span. J. Agric. Res., 11, 820–832, https://doi.org/10.5424/sjar/2013113-3747, 2013.
Spargo, J. T., Cavigelli, M. A., Mirsky, S. B., Maul, J. E., and Meisinger, J. J.: Mineralizable soil nitrogen and labile soil organic matter in diverse long-term cropping systems, Nutr. Cycl. Agroecosys., 90, 253–266, https://doi.org/10.1007/s10705-011-9426-4, 2011.
Stine, M. A. and Weil, R. R.: The relationship between soil quality and crop productivity across three tillage systems in South Central Honduras, Am. J. Alternative Agr., 17, 2–8, 2002.
Stockmann, U., Padarian, J., McBratney, A., Minasny, B., de Brogniez, D., Montanarella, L., Hong, S. Y., Rawlins, B. G., and Field, D. J.: Global soil organic carbon assessment, Glob. Food Secur.-Agr., 6, 9–16, https://doi.org/10.1016/j.gfs.2015.07.001, 2015.
Tejada, M., Rodriguez-Morgado, B., Gómez, I., Franco-Andreu, L., Benitez, C., and Parrado, J.: Use of biofertilizers obtained from sewage sludges on maize yield, Eur. J. Agr., 78, 13–19, https://doi.org/10.1016/j.eja.2016.04.014, 2016.
Tiecher, T., Santos, dos, D. R., and Calegari, A.: Soil organic phosphorus forms under different soil management systems and winter crops, in a long term experiment, Soil Till. Res., 124, 57–67, https://doi.org/10.1016/j.still.2012.05.001, 2012.
Trabucco, A. and Zomer, R. J.: Global Aridity Index (Global-Aridity) and Global Potential Evapo-Transpiration (Global-PET) Geospatial Database, CGIAR Consortium for Spatial Information, Published online, available from the CGIAR-CSI GeoPortal at: https://cgiarcsi.community/data/global-aridity-and-pet-database/ (last accessed: January 2019), 2009.
van Groenigen, K. J., Hastings, A., Forristal, D., Roth, B., Jones, M., and Smith, P.: Soil C storage as affected by tillage and straw management: An assessment using field measurements and model predictions, Agr. Ecosyst. Environ., 140, 218–225, https://doi.org/10.1016/j.agee.2010.12.008, 2011.
Vieira, F. C. B., Bayer, C., Zanatta, J. A., Dieckow, J., Mielniczuk, J., and He, Z. L.: Carbon management index based on physical fractionation of soil organic matter in an Acrisol under long-term no-till cropping systems, Soil Till. Res., 96, 195–204, https://doi.org/10.1016/j.still.2007.06.007, 2007.
Vieira, F. C. B., Bayer, C., Zanatta, J., and Ernani, P. R.: Organic matter kept Al toxicity low in a subtropical no-tillage soil under long-term (21-year) legume-based crop systems and N fertilisation, Soil Res., 47, 707–714, https://doi.org/10.1071/SR08273, 2009.
Vitousek, P. M., Naylor, R., Crews, T., David, M. B., Drinkwater, L. E., Holland, E., Johnes, P. J., Katzenberger, J., Martinelli, L. A., Matson, P. A., Nziguheba, G., Ojima, D., Palm, C. A., Robertson, G. P., Sanchez, P. A., Townsend, A. R., and Zhang, F. S.: Nutrient Imbalances in Agricultural Development, Science, 324, 1519–1520, https://doi.org/10.1126/science.1170261, 2009.
Wang, J. Y., Yan, X. Y., and Gong, W.: Effect of long-term fertilization on soil productivity on the North China Plain, Pedosphere, 25, 450–458, 2015.
Wang, Q. J., Lu, C. Y., Li, H. W., He, J., Sarker, K. K., Rasaily, R. G., Liang, Z. H., Qiao, X. D., Hui, L., and Mchugh, A. D. J.: The effects of no-tillage with subsoiling on soil properties and maize yield: 12-Year experiment on alkaline soils of Northeast China, Soil Till. Res., 137, 43–49, https://doi.org/10.1016/j.still.2013.11.006, 2014a.
Wang, Z. G., Jin, X., Bao, X. G., Li, X. F., Zhao, J. H., Sun, J. H., Christie, P., and Li, L.: Intercropping Enhances Productivity and Maintains the Most Soil Fertility Properties Relative to Sole Cropping, Plos One, 9, e113984, https://doi.org/10.1371/journal.pone.0113984, 2014b.
Williams, A., Hunter, M. C., Kammerer, M., Kane, D. A., Jordan, N. R., Mortensen, D. A., Smith, R. G., Snapp, S., and Davis, A. S.: Soil Water Holding Capacity Mitigates Downside Risk and Volatility in US Rainfed Maize: Time to Invest in Soil Organic Matter?, edited by: Gonzalez-Andujar, J. L., Plos One, 11, e0160974, https://doi.org/10.1371/journal.pone.0160974, 2016.
Wortman, S. E., Galusha, T. D., Mason, S. C., and Francis, C. A.: Soil fertility and crop yields in long-term organic and conventional cropping systems in Eastern Nebraska, Renew. Agr. Food Syst., 27, 200–216, https://doi.org/10.1017/s1742170511000317, 2012.
Wu, J., Wang, W., Wang, X., Zhu, L., Yang, H., Han, X., Gao, J., Guo, W., and Bian, X.: Residue management affects greenhouse gas emissions and soil organic carbon in wheat-rice rotation system, Fresen. Environ. Bull., 24, 2751–2762, 2015.
Yang, J. M., Yang, J. Y., Dou, S., Yang, X. M., and Hoogenboom, G.: Simulating the effect of long-term fertilization on maize yield and soil C/N dynamics in northeastern China using DSSAT and CENTURY-based soil model, Nutr. Cycl. Agroecosys., 95, 287–303, https://doi.org/10.1007/s10705-013-9563-z, 2013.
Yang, J., Gao, W., and Ren, S. R.: Long-term effects of combined application of chemical nitrogen with organic materials on crop yields, soil organic carbon and total nitrogen in fluvo-aquic soil, Soil Till. Res., 151, 67–74, https://doi.org/10.1016/j.still.2015.03.008, 2015.
Yang, Z. C., Zhao, N., Huang, F., and Lv, Y.: Long-term effects of different organic and inorganic fertilizer treatments on soil organic carbon sequestration and crop yields on the North China Plain, Soil Till. Res., 146, 47–52, https://doi.org/10.1016/j.still.2014.06.011, 2015.
Yeboah, S., Zhang, R., Cai, L., Li, L., Xie, J., Luo, Z., Liu, J., and Wu, J.: Tillage effect on soil organic carbon, microbial biomass carbon and crop yield in spring wheat-field pea rotation, Plant Soil Environ., 62, 279–285, https://doi.org/10.17221/66/2016-pse, 2016.
Zhang, S. X., Chen, X. W., Jia, S. X., Liang, A. Z., Zhang, X. P., Yang, X. M., Wei, S. C., Sun, B. J., Huang, D. D., and Zhou, G. Y.: The potential mechanism of long-term conservation tillage effects on maize yield in the black soil of Northeast China, Soil Till. Res., 154, 84–90, https://doi.org/10.1016/j.still.2015.06.002, 2015.
Zhang, W. J., Xu, M. G., Wang, B. R., and Wang, X. J.: Soil organic carbon, total nitrogen and grain yields under long-term fertilizations in the upland red soil of southern China, Nutr. Cycl. Agroecosys., 84, 59–69, https://doi.org/10.1007/s10705-008-9226-7, 2009.
Zhang, Y. L., Li, C. H., Wang, Y. W., Hu, Y. M., Christie, P., Zhang, J. L., and Li, X. L.: Maize yield and soil fertility with combined use of compost and inorganic fertilizers on a calcareous soil on the North China Plain, Soil Till. Res., 155, 85–94, https://doi.org/10.1016/j.still.2015.08.006, 2016.
Zhao, Y. C., Yan, Z. B., Qin, J. H., Ma, Z. J., Zhang, Y. F., and Zhang, L.: The potential of residues of furfural and biogas as calcareous soil amendments for corn seed production, Environ. Sci. Pollut. R., 23, 6217–6226, https://doi.org/10.1007/s11356-015-5828-1, 2016.
Zomer, R. J., Trabucco, A., Bossio, D. A., and Verchot, L. V.: Climate change mitigation: A spatial analysis of global land suitability for clean development mechanism afforestation and reforestation, Agr. Ecosyst. Environ., 126, 67–80, https://doi.org/10.1016/j.agee.2008.01.014, 2008.
Zomer, R. J., Bossio, D. A., Sommer, R., and Verchot, L. V.: Global Sequestration Potential of Increased Organic Carbon in Cropland Soils, Sci. Rep., 6, 1–8, https://doi.org/10.1038/s41598-017-15794-8, 2017.
Zvomuya, F., Janzen, H. H., Larney, F. J., and Olson, B. M.: A Long-Term Field Bioassay of Soil Quality Indicators in a Semiarid Environment, Soil Sci. Soc. Am. J., 72, 683, https://doi.org/10.2136/sssaj2007.0180, 2008.