the Creative Commons Attribution 4.0 License.
the Creative Commons Attribution 4.0 License.
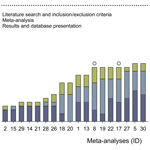
Quality assessment of meta-analyses on soil organic carbon
Julia Fohrafellner
Sophie Zechmeister-Boltenstern
Rajasekaran Murugan
Elena Valkama
Soil organic carbon (SOC) plays a vital role in the global carbon cycle and is a potential sink for carbon dioxide. Agricultural management practices can support carbon sequestration and, therefore, offer potential removal strategies whilst also improving overall soil quality. Meta-analysis allows one to summarize results from primary articles by calculating an overall effect size and to reveal the source of variation across studies. The number of meta-analyses published in the field of agriculture is continuously rising. At the same time, more and more articles refer to their synthesis work as a meta-analysis, despite applying less than rigorous methodologies. As a result, poor-quality meta-analyses are published and may lead to questionable conclusions and recommendations to scientists, policymakers, and farmers.
This study aims at quantitatively analyzing 31 meta-analyses, published between the years of 2005 and 2020, studying the effects of different management practices on SOC. We compiled a set of quality criteria suitable for soil and agricultural sciences by adapting existing meta-analytical guidelines from other disciplines. The set is supported by a scoring scheme that allows for a quantitative analysis. The retrieved meta-analyses were structured according to 11 management categories, such as tillage, cover crops, crop residue management, and biochar application, which allowed us to assess the state of knowledge on these categories. Major deficiencies were found in the use of standard metrics for effect size calculation, independence of effect sizes, standard deviation extraction for each study, and study weighting by the inverse of variance. Only 1 out of 31 SOC meta-analyses, which studied the effects of no tillage/reduced tillage compared with conventional tillage, was found to be of high quality. Therefore, improved meta-analyses on the effects of organic agriculture, biochar, fertilization, or crop diversification on SOC are urgently needed.
We conclude that, despite efforts over the last 15 years, the quality of meta-analyses on SOC research is still low. Thus, in order for the scientific community to provide high-quality synthesis work and to make advancements in the sustainable management of agricultural soils, we need to adapt rigorous methodologies of meta-analysis as quickly as possible.
- Article
(3639 KB) - Full-text XML
-
Supplement
(892 KB) - BibTeX
- EndNote
1.1 Meta-analysis as a method and its application in different disciplines
Meta-analysis was first defined by Glass (1976, p. 3) as “the statistical analysis of a large collection of results for the purpose of integrating these findings”. A newer, more precise definition by Koricheva and Gurevitch (2013a) describes it as “a set of statistical methods for combining the magnitude of the outcomes (effect sizes) across different data sets addressing the same research question”. It supports the structuring of the increasing amount of information (Koricheva and Gurevitch, 2014), which researchers of all fields face, and offers tools to process information with increased precision and reliability (Cooper et al., 2019b; Nakagawa and Cuthill, 2007).
Meta-analysis was developed to facilitate quantitative evidence synthesis in medical, social, and behavioral sciences (Gurevitch et al., 2018; Hedges et al., 1999). The method was first applied in ecology and evolutionary biology about 30 years ago, at a time when the need for quantitative assessment of urgent issues such as climate change or biodiversity losses arose. Since then, meta-analysis has developed within the field of ecology, establishing centers and collaborations for research synthesis (Gurevitch et al., 2018). The results of these contributions frequently provide relevant stakeholders and decision-makers with evidence-based information (Stewart, 2010).
In agricultural research, meta-analysis has only attracted a broader interest in the last decade (Fig. 1). Particularly, the use of meta-analysis as a tool to investigate the effects of agricultural management practices on relevant response variables, such as yield or soil physical or chemical parameters, is becoming increasingly prominent (Haddaway et al., 2017; Bai et al., 2019; Li et al., 2020). As these developments are rather recent, knowledge on the appropriate meta-analytical methodology is still finding its place in the research community.
Because of their close relationship, many methodological approaches of meta-analyses in ecology are also transferable to the field of agriculture and soil sciences. For instance, when comparing several agricultural studies, looking at a specific treatment effect compared to a control, contradictory outcomes are sometimes reported. By including the results of all studies and calculating a summary treatment effect, meta-analysis allows us to combine the available knowledge, regardless of the outcome, and calculate one number, which tells us about the overall estimated effect, thereby overcoming conflicting evidence. Therefore, combining results across several sites or assessing the impacts of environmental drivers, such as climate change, are tasks that are processable by meta-analysis (Koricheva and Gurevitch, 2014). Nevertheless, research on agriculture and soil has encountered issues, which are often specific to these fields. First, changes in soil, like soil organic carbon (SOC), are often slower and more difficult to detect (due to the small sample size and spatial variability within a site and between sites) (Mäkipää et al., 2008) compared with other physiological and biogeochemical changes, such as changes in plant tissue. Moreover, changes in SOC due to management practices have different responses depending on soil depths that need to be considered when summarizing results across studies. Agricultural systems are very complex, as not only pedoclimatic conditions influence soil but agricultural management practices also impact variables of interest. In particular, the mix or combination of practices (e.g., tillage and crop residue retention) makes it difficult to distinguish between sources of effects (Xiao et al., 2021). Therefore, it is crucial to define not only the treatment but also the control of the experiments precisely to allow for the computation of heterogeneity. Lastly, when it comes to soil parameters and indicators, several methods are available for computation, which may cause difficulties when comparing outcomes. A good example is bulk density, which can be measured in a field experiment or estimated using pedotransfer functions in order to compute SOC stocks from concentrations. The potential uncertainty that arises when applying a pedotransfer function developed in a particular area to different sites (Schillaci et al., 2021) can diminish the precision of final results.
1.2 Available guidelines and their applicability
Currently, no collaborations or guidelines for publishing systematic reviews or meta-analyses on agricultural or soil issues exist. In contrast, the healthcare (the Cochrane Collaboration) and social science (the Campbell Collaboration) fields had already established such collaborative networks to develop high-quality reviews in the 1990s (Gurevitch et al., 2018; Collaboration for Environmental Evidence, 2018). These collaborations are focused on specific disciplines, but some of their tools, such as training or the “Cochrane Handbook for Systematic Reviews of Interventions”, are partly applicable to agricultural and soil research (Table S1 in the Supplement). Moreover, there are other voluntary guidelines available that aim to support researchers in, e.g., reporting or producing meta-analyses. Checklists for evaluating social science research synthesis (Cooper et al., 2019a) or evidence-based minimum item sets for reporting in systematic reviews and meta-analysis, such as the Preferred Reporting Items for Systematic Reviews and Meta-Analyses (PRISMA) website (Page et al., 2021), support synthesis consumers and authors. PRISMA-EcoEvo is a PRISMA extension for syntheses in ecology and evolutionary biology, which can be used for reporting, planning, registration, and reviewing (O'Dea et al., 2021). Moreover, for meta-analyses in ecology, a checklist of quality criteria is available (Koricheva and Gurevitch, 2014). The Collaboration for Environmental Evidence (CEE) provides guidelines and standards for evidence synthesis in environmental management that can be used for conducting, commissioning, or using the findings of systematic reviews and systematic maps in environmental management. Further, reporting standards (the RepOrting standards for Systematic Evidence Syntheses – ROSES), a checklist for appraisal of confidence of evidence reviews (the Collaboration for Environmental Evidence Synthesis Assessment Tool – CEESAT), and open-access online training courses are offered by CEE. The collaboration even brought forth Environmental Evidence, a journal facilitating the publication of evidence synthesis in environmental management (https://environmentalevidencejournal.biomedcentral.com/, last access: 17 January 2023). Lastly, reviews by Philibert et al. (2012), Beillouin et al. (2019), and Krupnik et al. (2019) have assessed the quality of agronomic meta-analyses or compared different meta-analytical methods with the help of quality criteria; however, they are formulated rather generally.
Although all of these guidelines are available, they each use different criteria which are sometimes not reported exhaustively (Koricheva and Gurevitch, 2014), making it difficult to apply them to different disciplines (Nakagawa and Cuthill, 2007; Lortie et al., 2015), such as for the quality assessment of meta-analyses in agricultural and soil sciences. Additionally, as mentioned above, soil and agricultural scientists encounter specific issues, different from those faced in ecology or medicine, when aiming to synthesize research outcomes meta-analytically. The guidelines and standards for evidence synthesis in environmental management and the CEESAT checklist by CEE clearly benefit scientists and other consumers of soil and agricultural meta-analyses; however, they mainly focus on systematic reviews and maps and also contain elements not mandatory in meta-analysis (e.g., registration), such as gathering the maximum available relevant literature or performing critical appraisal. Moreover, the guideline is exhaustive and requires inexperienced readers' time and effort to understand. Those who are not aiming to become experts in the method themselves might not be able to find the time for such elaborate reading.
1.3 Why we need meta-analytical guidelines in agricultural and soil research
The contribution of agriculture to the global anthropogenic greenhouse gas (GHG) emissions (Tubiello et al., 2015) and the possibility of sequestering carbon through improved soil management in the form of SOC (Smith, 2012; Paustian et al., 2016; Smith et al., 2005) are topics that have occupied soil and agricultural researchers over the last decades. Since 2000, the number of articles published on SOC has increased yearly (Fig. 1), due to climate change pushing the scientific community to search for mitigation and adaption opportunities in numerous ways, such as through agronomic practices. Carbon sequestration in soils has gained increased resonance with respect to the European Union political agenda (EU Green Deal, Farm to Fork Strategy, EU Soil Strategy for 2030) – especially since the launch of the “4/1000 Initiative: Soils for Food Security and Climate” at COP 21 (21st session of the Conference of the Parties) as well as the publication of the global potentials of this initiative (Minasny et al., 2017).
Simultaneously, the number of meta-analyses published in the field of agriculture is continuously rising. We searched the Web of Science Core Collection for all available entries on “meta-analysis AND agriculture” since the year 2000 (Fig. 1, search conducted 13 January 2022). Between 2000 and 2010, there was little change in the number of meta-analyses published; a steady rise can only be seen since 2010. The increasing amount of available information, not only in agriculture and SOC research but also across all scientific fields, is creating the need to synthesize data into a form that is easier to comprehend and that allows for the detection of overarching patterns (Culina et al., 2018). Unfortunately, as a consequence of the rising popularity of this method, more and more publications refer to their synthesis work as meta-analyses, despite applying less than rigorous methodologies. Many times, the term is misapplied to publications synthesizing information of primary studies, regardless of the methodologies used (Gurevitch et al., 2018). In fact, only studies using well-established statistical procedures – most importantly suitable effect size calculation, correct study weighting by the inverse of variance (1/variance), analysis of possible heterogeneity, and appropriate statistical models that account for the structure of the meta-analytical data – should use the term “meta-analysis” to describe their synthesis method (Vetter et al., 2013; Gurevitch et al., 2018). When applying “nonstandard metrics”, which refer to using methods other than the effect size as defined by Borenstein et al. (2009) to quantitatively synthesize primary studies, articles should not be called a meta-analysis nor claim that “effect sizes” were calculated, as these terms are specific to the meta-analytical methodology (Borenstein et al., 2009; Koricheva and Gurevitch, 2014; Cooper et al., 2019c). It is important to promote this clear definition in order to allow the distinction between a “true” meta-analysis and other forms of synthesis work, such as correlation analyses or analyses through machine learning.
The previously mentioned reviews by Philibert et al. (2012) and Krupnik et al. (2019), who analyzed the quality of meta-analyses in agronomy, found that the overall quality of meta-analyses in this field is low. Philibert et al. (2012) concluded that more than half of the publications in the searched databases mentioned meta-analyses as a method but did not carry the method out. Further issues regarding effect size metrics, weighting, and heterogeneity analysis were found. The more recent review by Krupnik et al. (2019), who analyzed meta-analyses studying the effects of conservation and organic agriculture on yield, also reported lacks in heterogeneity testing and weighting. Similarly, Beillouin et al. (2019), who studied meta-analyses on crop diversification, found issues with weighting, sensitivity analysis, and database presentation. These results imply that the methodology applied in agronomic meta-analyses is variable and often not done according to standard metrics. The authors of the reviews concluded that there is a need for the improvement of meta-analyses in agronomy.
Finally, it is a misconception that a high number of citations always equals quality (Aksnes et al., 2019; Leydesdorff et al., 2016). Koricheva and Gurevitch (2014) found that cases of incorrect usage of the term meta-analysis can even be encountered in high-impact journals. This suggests that not only authors but also peer reviewers and journal editors do occasionally misunderstand the rules under which a meta-analysis must be conducted. O'Leary et al. (2016) analyzed the effects of the journal impact factor on review quality and concluded that a high impact factor does not guarantee high quality of reviews; therefore, the aforementioned authors did not recommend the use of the impact factor as a proxy for review quality.
All of this provides reason to assume that core criteria, necessary for conducting meta-analyses, are not clear to many researchers in the field of agricultural and soil sciences. As a result, poor-quality meta-analyses are published that might report questionable conclusions and recommendations to other scientists, policymakers, and farmers. Moreover, the interest in SOC sequestration and the subsequent increase in related publications raises questions as to whether meta-analyses synthesizing this knowledge currently exist, and, if so, whether their quality shows similar trends to agricultural meta-analyses reviewed in the past by Philibert et al. (2012), Beillouin et al. (2019), and Krupnik et al. (2019).
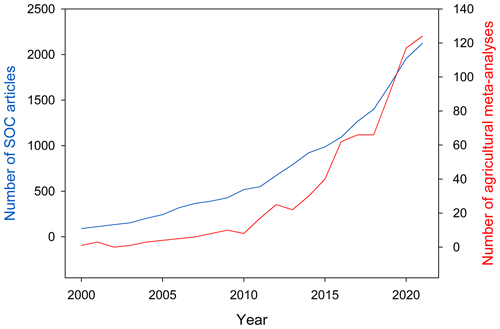
Figure 1The number of meta-analyses in agriculture and primary research articles on soil organic carbon published between 1 January 2000 and 31 December 2021. The search was conducted on 13 January 2022 on the Web of Science Core Collection (searched in “Topic”), and results were taken from the Web of Science “Analyze Results” tool. The Boolean search string for MA in agriculture was as follows: “meta-analysis AND agriculture” and “carbon”. The Boolean search string for articles on SOC was as follows: “soil organic carbon”.
1.4 Objectives
This study aims to quantitatively analyze 31 meta-analyses, published between the years 2005 and 2020, studying the effects of different management practices on SOC that are relevant for European cropland. We compiled a set of quality criteria suitable for soil and agricultural sciences by adapting existing meta-analytical guidelines from other disciplines. The set is supported by a scoring scheme that allows for a quantitative analysis. A subsequent evaluation of the management practices studied in these SOC meta-analyses gives information on which agricultural operations require more or improved research. Finally, the aim was to demonstrate how to conduct a quick assessment of the meta-analyses relevant for decision-making. We chose a chapter of the IPCC “Special Report – Climate Change and Land” (Jia et al., 2019) and analyzed the quality of cited meta-analyses by using the most critical criteria of the compiled criteria set.
2.1 Quality criteria set
The set of quality criteria is based on the previous work of many experienced researchers with expert knowledge on meta-analysis (Table S1). The “Checklist of quality criteria for meta-analysis for research synthesis, peer reviewers and editors” by Koricheva and Gurevitch (2014) was used as a basis for the composition of the 17 quality criteria (Table 1). Their checklist is also built upon the previous efforts of other scientists who established sets of quality criteria in the fields of ecology, environmental management, conservation biology, and agronomy. Other literature, such as the “Introduction to Meta-Analysis” by Borenstein et al. (2009), “Handbook to Meta-analysis in Ecology and Evolution” by Koricheva et al. (2013b), and “Handbook of Research Synthesis and Meta-Analysis” by Cooper et al. (2019c), further supported the criteria construction and act as sources for an in-depth explanation of those criteria, thereby providing the reader with additional information (Table S2).
The 17 quality criteria were structured according to three groups: “Literature search and inclusion/exclusion criteria”, “Meta-analysis”, and “Results and database presentation” (Table 1). Additionally, a further division of the “quality criteria” into “sub-criteria” was conducted to provide more detailed guidance. Each quality criterion or – if available – sub-criterion, was specified with the help of the “Is the criterion applied in meta-analysis (to what extent)” column, which offers the reader possible options, based on the availability of data or items within the analyzed meta-analysis. Each option ends with a numerical “Score” that indicates its quality. All individual scores can be summarized into a total score with a maximum of 30; the higher the total score, the better the overall quality of the meta-analysis. Furthermore, the quality criteria and sub-criteria are specified in the “Description” column to provide the reader with more detailed information. The final column offers references to relevant literature, supporting the authors' decisions with respect to criteria formulation and scoring. In the Supplement (Table S2), an extended version of this column can be found, where direct quotes of cited experts are provided.
Of these 17 quality criteria, we defined three as so-called “cutoff” criteria (criteria 6–8 in Table 1), namely “Effect size”, “Standard deviation extracted (or computed from statistics)”, and “Studies weighted by 1/variance”. When these criteria are not fulfilled by a meta-analysis, the most essential and relevant steps in this specific synthesis method are not met. These cutoff criteria aim to help consumers of soil and agricultural meta-analyses to identify the defining elements of the article and judge whether it is a true meta-analysis or not. As we wanted to highlight criterion 8, Studies weighted by 1/variance, and credit meta-analyses that did weight all studies correctly, we attributed a maximum obtainable score of 4 to this criterion.
2.2 Quality assessment of meta-analyses on SOC
2.2.1 Inclusion criteria, exclusion criteria, and search strategy
First, inclusion criteria (IC) and exclusion criteria (EC) were defined to create a framework for the literature screening (Table 2). Studies were included when they used the term meta-analysis in their title, abstract, or author keywords (IC1); the land uses included were arable land or crop land, also in combination with others land uses, such as agroforestry or grassland (IC2); the assessment of the effects of one or several management practices on SOC was the aim of the study (IC3); and European experiments were a part of the (global) meta-analyses, as we wanted to collect and evaluate syntheses relevant for Europe (IC7). Articles were only included when they fulfilled all seven inclusion criteria. Articles were excluded when, for example, modeling was used to obtain SOC results (EC1).
The second step was the collection of existing meta-analyses on SOC changes due to different agricultural management practices. Therefore, the Web of Science Core Collection (time frame 1900–2020) and Scopus (time frame 1960–2020) databases were searched on 5 January 2021. Due to limited human resources, only these two scientific databases were searched. The following Boolean search string was used to retrieve relevant articles: (meta-analy∗) AND soil AND (agriculture OR management) AND (SOC OR OC OR “soil organic carbon” OR “organic carbon”). A total of 552 articles were found (344 in Web of Science and 208 in Scopus) and automatic (conducted by Mendeley and JabRef software) and manual duplicate removal reduced the results by 167 articles (Fig. 2). The results were compared with the meta-analyses identified by Bolinder et al. (2020), who synthesized meta-analyses studying the effects of several management practices on SOC changes in agroecosystems. This led to the identification of one further study that complied with our inclusion and exclusion criteria (Table 2) and, therefore, was included in our evaluation. A total of 386 articles were exported into Microsoft Excel and screened by title, abstract, and full text according to the predefined inclusion and exclusion criteria. In total, 31 meta-analyses relevant for the scope of our study were found. Many articles were excluded, as they did not contain the word meta-analysis in their title, abstract, or keywords; SOC was not the response variable of interest; or the studies investigated did not include European sites. Figure 2 shows a flow diagram of the complete screening process. Full information on the literature gathering (Table S3), all 386 retrieved articles, and the screening decisions (Table S4) can be found in the Supplement. The complete reference list of the 31 meta-analyses can be found in the Appendix (Table A1).
2.2.2 Quality analysis
The 31 retrieved meta-analyses were evaluated by two authors with respect to quality according to the set of quality criteria in Table 1. Each article was read thoroughly to ascertain whether certain criteria were fulfilled or not. Total scores for each meta-analysis were calculated, with a maximum reachable score of 30. The complete assessment containing the scores for each meta-analysis and all calculations can be found in the Supplement (Tables S2, S5). SigmaPlot version 14.5 and Microsoft Excel version 1808 were used for the plotting of figures and tables and for calculations.
2.2.3 Management categories
The retrieved data also offered the possibility to analyze the state of knowledge on meta-analyses studying management effects on SOC. The aim was to assess how many meta-analyses were conducted on a certain management practice and whether their quality was sufficient to stop the production of new meta-analyses on the respective practices. This information will aid future research by guiding it towards knowledge needs and avoiding redundant work. Therefore, we grouped the meta-analyses according to the management practices they studied. A total of 11 management categories were formed and are described in Table 3. These categories aim to structure the collected SOC meta-analyses and allow a simplified investigation. As some meta-analyses studied the effects of more than one practice, they were added to all respective categories.
Finally, the total number of articles per category was calculated, and the meta-analyses with the highest scores were identified. Simultaneously, information on the treatment and control, the geographical scale, and soil depth was extracted. As the overall score does not give information on whether the cutoff criteria were fulfilled, we extracted this information as well. We presented the overall effect sizes of the meta-analyses only when both of these elements were fulfilled.
Overall treatment effects on SOC are shown as a percentage change from the control; when results were displayed as a log response ratio (LnR), we calculated percentages using Eq. (1):
2.3 Quick assessment of meta-analyses relevant for policy-making – an example
To provide readers with an example of the impacts of meta-analytical quality on policy- and decision-making, we screened “Chapter 2: Land–climate interactions”, of the Intergovernmental Panel on Climate Change (IPCC) “Special Report – Climate Change and Land” (Jia et al., 2019) for cited articles that used the term meta-analysis in the title. We chose this report by the IPCC, as their outputs are highly relevant for combating the global climate crisis and are often the basis of policy-making (IPCC, 2019) as well as the fact that this exact chapter is deeply connected to the contents of this review. In total, 16 articles were retrieved and checked against the cutoff criteria of the abovementioned quality criteria set (Table S6).
The investigation of the 31 meta-analyses studying management effects on SOC, published between 1990 and 2020, found that Ogle et al. (2005) published the first article on this topic. Nevertheless, the synthesis did not qualify as a formal meta-analysis, as no effect size was calculated. The first formal meta-analysis on SOC was published by Luo et al. (2010), who looked at the effect of no tillage versus conventional tillage. Overall, the number of SOC meta-analyses, published between 2005 and 2020, increased over time (Fig. 3a). Scores, which were calculated based on the fulfillment of the quality criteria, also experienced a rise (15-year period) and were significantly related to the publication year () (Fig. 3b) (normal distribution of scores tested with a Shapiro–Wilk test; P=0.115). If the observed rise in quality is projected into the future, without any intervention, a score of 30 will only be reached by the year 2033. As the meta-analysis by Haddaway et al. (2017) (ID = 10; score = 29) is an outlier that influences the regression result, we also calculated how the prognosis would change if we removed this meta-analysis. The new regression line () estimates that scores of 30 will be reached in 2034.
Table 3Defined management categories, their included management practices and meta-analyses that studied their effects on SOC.
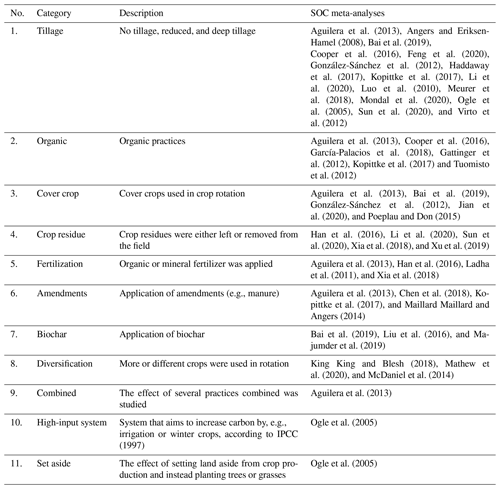
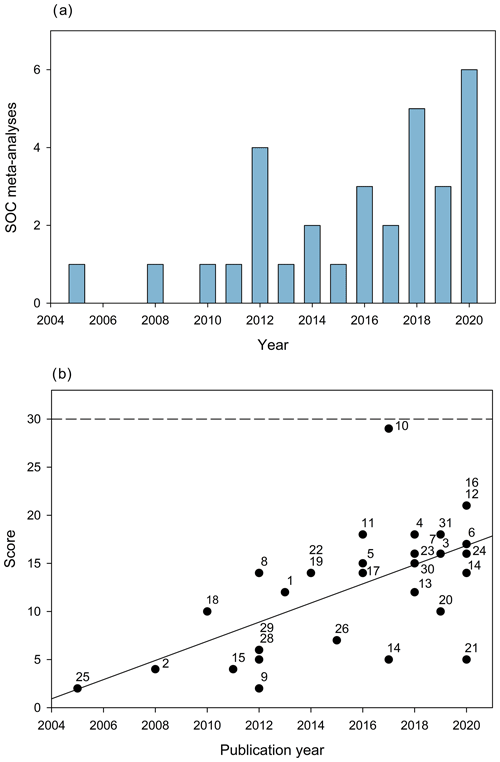
Figure 3(a) The number of SOC meta-analyses published per year. (b) Scores of SOC meta-analyses over time (between 2005 and 2020) and the corresponding regression line. The numbers beside the dots indicate the meta-analysis ID (ID and linked author information given in Tables A1 and S2). The dashed line indicates the maximum score of 30.
3.1 Literature search and inclusion/exclusion criteria
The 17 quality criteria are clustered into three groups (Table 1). The first one, Literature search and inclusion/exclusion criteria consists of five quality criteria; the first criterion, “Literature search”, was satisfied by more than half of the meta-analyses (Fig. 4). In nearly a quarter of the analyses, authors checked the reference lists of other existing meta-analyses and reviews for available literature. Therefore, the usefulness of this method seems to be widely underestimated. By comparing retrieved literature to other existing publications, we can not only gain confidence in our search strategy but also encounter information which might be difficult to find otherwise (e.g., gray literature).
Inclusion and exclusion criteria as well as a description of the treatment and control were presented by almost all meta-analyses (although we only analyzed whether the treatment and control were described, not if they were comparable across included studies). Moderators were described by over half of SOC meta-analyses. Description of moderators, including their range (for continuous explanatory variables) or groups (for categorical explanatory variables) are necessary to present the way in which moderator analysis will be conducted. Results for the sub-criteria can be found in the Supplement (Table S5).
3.2 Meta-analysis
The Meta-analysis group consisted of nine quality criteria (Table 1) that were satisfied by the SOC meta-analyses to various extents. Effect sizes were calculated according to standard metrics by 74 % of meta-analyses (Fig. 5a). Almost half of the meta-analyses used the log response ratio for effect size calculation and about a third applied the raw mean difference or standardized mean difference. Standard deviations (SDs) were extracted (or computed from available statistics) from all primary studies by 16 % of meta-analyses and were partly extracted (correctly extracted for some studies but ignored or roughly estimated by, e.g., calculating the mean SD from available SDs or reassigning as of the mean, for the rest) by 42 % of meta-analyses (Fig. 5b). Weighting each study by 1/variance was done by 13 % of meta-analyses (Fig. 5c). In total, 19 % of SOC meta-analyses weighted only some studies by 1/variance, as they only extracted or computed SDs from some studies (and, therefore, received a score of 1 for criterion 7; for a detailed description of the criterion for weighting, see quality criterion 8 in Table 1). Accordingly, weighting was not done in over two-thirds of analyses. We classified these three criteria (Effect size, Standard deviation extracted (or computed from statistics), and Studies weighted by 1/variance) as cutoff criteria (criteria 6–8 in Table 1). When these criteria were not fulfilled, a purported meta-analysis was deemed not to be an actual meta-analysis. In our quality assessment, we acknowledged when authors partially weighted by 1/variance (as they only partially extracted SDs) with one point for each. Nevertheless, we urge authors to extract SDs for each study (or compute them from available statistics) and further weight them by 1/variance in order to conduct a high-quality meta-analysis.
In Figs. 6 and 7, satisfaction of criteria 9–14 and 15–17, respectively, are displayed in the form of stacked bars which show the percentage of meta-analyses that did fulfill the cutoff criteria (n=4) and those that did not (n=27; a total of 31). In the following, we will describe only the results for all 31 SOC meta-analyses. For the individual results, the reader is referred to the figures. Corresponding data used for the calculation of these results can be found in the Supplement (Table S7).
Subgroup analysis and meta-regression, which identify the source of variation between studies, were assessed by almost half of meta-analyses (Fig. 6). The models applied and the software used were reported more frequently. Only about 25 % of meta-analyses accounted for the nonindependence of effect size, whereas the rest failed to do so. Bulk density was measured in 35 % of meta-analyses, but the other 65 % used pedotransfer function to estimate this parameter, thereby introducing a source of uncertainty into the SOC stock estimation. Lastly, sensitivity analysis of the meta-analytical results was rarely done.
3.3 Results and database presentation
Figure 7 shows the results for the Results and database presentation group. Almost half of the meta-analyses displayed their results in the form of figures or tables. Summarized effect sizes and confidence intervals or moderator analysis were presented graphically or in tabular form by 65 % and 68 % of meta-analyses, respectively. Forest plots were presented by 6 % of meta-analyses. Metadata were presented in over two-thirds of analyses, whereas a full database was made available to the readers in 13 % of cases and was made partly available in 3 % of cases (for a further explanation, see criterion 17 in Table 1). Information on the calculation of these results can be found in the Supplement (Table S7).
3.4 Overarching findings
When looking at the overall results across the three quality criteria groups, quality varied greatly among the 31 analyses, with a maximum score of 29, a minimum score of 2, and a median of 14. Haddaway et al. (2017) produced a high-quality meta-analysis that received the highest score according to our assessment; however, they used the raw mean difference to calculate effect sizes, which may not be the most suitable for meta-analyses in the soil and agricultural field. In Sect. 4.2., we will go into more detail on this issue. There were seven meta-analyses with scores up to 5, but the majority achieved scores between 5 and 15. A total of 10 meta-analyses reached scores between 15 and 20, whereas only 3 reached a score above 20. Only 4 out of 31 meta-analyses are true meta-analyses, as they used standard metrics for effect size calculation and weighted all studies by 1/variance (Fig. 8).
3.5 Analyzing management categories
Management practices studied in the meta-analyses were counted in order to assess their incidence. We found that almost half of the 31 meta-analyses studied the effects of tillage on SOC (in some cases besides other management practices) (Table 4). Other practices studied frequently were “organic agriculture” and “cover crop cultivation” (six times each). Data on “crop residue”, “fertilization”, “amendments”, “biochar”, and “diversification” were synthesized less often. The effects of “combined practices”, “high input”, and “setting aside” on SOC were each assessed once. We found that meta-analyses that passed the cutoff criteria were available for 4 out of the 11 management categories (tillage, cover crop, crop residue, and amendment). For tillage, we decided to show the three meta-analyses with the best scores (Bai et al., 2019; Haddaway et al., 2017; Li et al., 2020), as several analyses above average quality were available. Nevertheless, only Haddaway et al. (2017) fulfilled the criteria for effect size calculation, SDs, and weighting whilst also achieving a high overall score; therefore Haddaway et al. (2017) is the one publication providing a high-quality meta-analysis on the effects of management practices on SOC. In the Organic, Fertilization, Biochar, Diversification, Combined, High-input system, and Set aside categories, no meta-analyses conducted according to the standards are currently available. In the last column of Table 4, overall effect sizes for SOC can be found. As Haddaway et al. (2017) calculated effect sizes using the raw mean difference, it was not possible to transform their results from stock into percentages. For the five management categories where no meta-analyses were weighed by 1/variance (Fertilization, Diversification, Combined, High-input system, and Set aside), the overall effect sizes for SOC change are not displayed. When looking at the retrieved data on SOC changes per management category (Table 4), it is apparent that the largest increases in SOC compared with the controls were achieved in the Organic, Cover crop, Amendments, and Biochar categories.
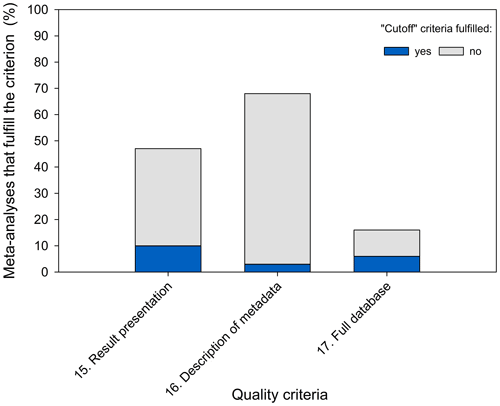
Figure 7Compliance of meta-analyses with the criteria in the Results and database presentation group.
Table 4Meta-analyses with the highest score per management category, effect size and weighting used, and overall SOC responses.
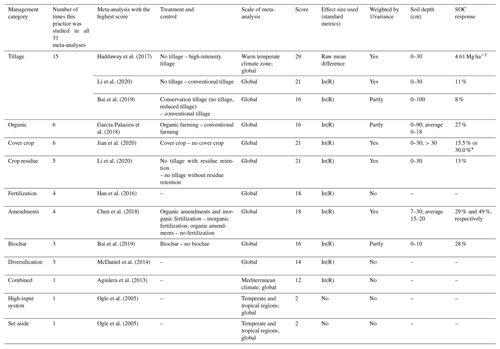
Meta-analyses that did not calculate effect sizes according to standard metrics or weight by 1/variance do not qualify as meta-analyses; therefore, their results are not presented. The maximum achievable score is 30. ∗ Results for when all comparisons were included or only comparisons with SD values were included, respectively.
3.6 Example of quick quality assessment of meta-analyses, relevant for policy-making
Our quick analysis of the IPCC Special Report – Climate Change and Land (Jia et al., 2019) found that, out of 16 articles, more than 50 % of articles did not qualify as true meta-analyses, as 5 did not calculate effect sizes according to standard metrics and 4 failed to extract SDs and to weight by 1/variance. Seven articles did, in fact, conduct meta-analysis correctly. Six meta-analyses used the log response ratio to calculate effect sizes, and one used the standardized mean difference. These seven meta-analyses extracted SDs for each study and weighted by 1/variance. Calculations and references for all 16 analyzed articles can be found in Table S6.
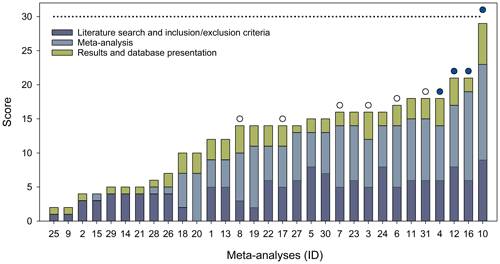
Figure 8Scores of individual SOC meta-analyses displayed as scores per group and sorted from lowest to highest achieved score. The meta-analysis ID and full reference information appear in Table A1. The dashed line indicates the maximum reachable score of 30. Filled circles indicate true meta-analyses, which used standard metrics for effect size calculation and weighted each study by inverse variance. Open circles indicate meta-analyses that weighted some studies by 1/variance.
Previous guidelines and expert knowledge on meta-analysis from other disciplines were adapted to construct an easy-to-use set of criteria for the quantitative quality assessment of meta-analyses in soil and agricultural research. With the help of these criteria, we analyzed 31 meta-analyses studying the effects of different management practices on SOC. Moreover, the retrieved meta-analyses were structured according to 11 categories of agricultural management practices, which allowed us to assess and analyze the state of knowledge on these categories. Hence, recommendations for future meta-analytical research and the general improvement of the applied methodology can be given. We found major deficiencies in the reporting of literature searches, application of standard metrics for effect size calculation, correct weighting by 1/variance, extraction of independent effect sizes, and database presentation. The quality of meta-analyses rose over time (15-year period) and was significantly correlated with publication year (R2=0.382). Similar trends have been observed in quality assessments of meta-analyses in the medical (Jamshidi et al., 2018) and environmental (Beillouin et al., 2019) fields.
In the following, we will discuss the results of the quality assessment of meta-analyses on SOC using the findings of four quality assessments of meta-analyses and quantitative reviews in agronomy and ecology. We included the study by Philibert et al. (2012), focusing on agri-environment and agri-biodiversity; the review of Krupnik et al. (2019), looking at conservation and organic agriculture; the study by Beillouin et al. (2019), examining crop diversification; and the excellent evaluation of meta-analyses in plant ecology by Koricheva and Gurevitch (2014). To simplify the discussion, not all information for the 17 quality criteria was extracted from the reviews. Instead, we selected quality criteria to be discussed according to (1) the information available in most of the reviews, which allowed a comparison of results, and (2) relevance (as, e.g., effect size metrics), as certain quality criteria are more important than others.
4.1 Literature search and inclusion and exclusion criteria
The comparison of reviews for the “Literature search” criterion showed that our study found higher compliance (53 %) with this criterion than those of Philibert et al. (2012) or Koricheva and Gurevitch (2014) (Table 5). Beillouin et al. (2019) reported that 46 % of meta-analyses presented the search string and 86 % presented the eligibility criteria. Krupnik et al. (2019) found that all analyzed meta-analyses presented the literature search sufficiently. This high agreement may be caused by the small study number (n=17) or the definition of less demanding criteria by the authors.
A quality criterion that is of special significance to the soil and agricultural field is the inclusion of gray literature. Here, exceptionally large amounts of data are available, as governmental research activities are not focused on publishing results in scientific journals. Therefore, although the inclusion of gray literature is not compulsory, it is highly encouraged (Culina et al., 2018). When conducting a meta-analysis on an international or global scale, analysts will find that gray literature is often available in national languages only, which complicates and restricts its inclusion. Nevertheless, the most essential part of searching for literature, whether scientific or gray, is complete reporting. Our results show that this reporting of search strategies is often limited. Therefore, essential information to allow the reproduction of the study is lacking, and possible differences in outcomes between meta-analyses, studying the same effects, cannot be fully explained. If a synthesis is not replicable, it cannot be fully trusted, as mistakes in methodological proceedings are possible (Haddaway et al., 2020; Parker et al., 2016). In another review, Hungate et al. (2009) showed how important complete reporting of search and screening strategy is. Lack of transparency prompted criticism of the results of meta-analyses. Nonidentical time frames over which literature was gathered; differences regarding inclusion criteria; and, in our eyes most importantly, limited search methods can influence the number of articles found and included in a meta-analysis. This indicates the need to draw quality criteria and disseminate good practices across research fields and to improve the power of meta-analytical results.
4.2 Meta-analysis
Effect size calculation is an essential and mandatory part of meta-analysis (Koricheva and Gurevitch, 2014). Therefore, the term meta-analysis should only be used when data are quantitatively synthesized as described in the textbooks of Borenstein et al. (2009), Cooper et al. (2019c), and Koricheva et al. (2013b). The investigation regarding the compliance of our SOC meta-analyses with the “Effect size calculated according to standard metrics” criterion showed that about three-quarters of meta-analyses did calculate effect sizes according to such metrics. Koricheva and Gurevitch (2014) came to similar conclusions in their review of meta-analyses in plant ecology (Table 5). Further, only about half of SOC meta-analyses used the log response ratio for effect size calculation.
These findings indicate that correct calculations of effect sizes are not applied consistently in the fields of SOC and plant ecology, although they represent the most fundamental and critical part in meta-analysis. Among the several possible choices of effect size metrics, we recommend using log response ratios when conducting soil and agricultural meta-analyses. These ratios are easy to interpret, and the effect sizes are not affected by the different variances of the control and experimental groups. Overall, they are more suitable for meta-analyses studying agricultural management effects on soil parameters, such as SOC, than the standardized mean difference (Hedges' d). When using the standardized mean difference, the results are more difficult to interpret (especially for policymakers or farmers) compared with log response ratios, which can be back-transformed to percent changes from the control.
In Sect. 3.3, we mentioned that, in our opinion, the raw mean difference (also called the unstandardized mean difference) is not suitable for calculating effect sizes in the field of soil and agricultural research. Unlike the response ratio, the raw mean difference does not consider variations in control levels, which are often highly variable across field experiments, particularly at a global scale. In the case of SOC studies, control levels may vary between 10 and 100 t C ha−1, which makes using the raw mean difference between the treatment and control as an index of effect size meaningless. It may result in similar effect sizes for the relatively large and relatively small responses, as illustrated in Fig. 9. Therefore, the raw mean difference can only be applied when all experiments studied in the meta-analysis use the same scale (Borenstein et al., 2009). The raw mean difference usually does not result in a normal distribution of effect sizes, which is a prerequisite. Although this metric is easy to use, it may be suitable for meta-analyses when controls do not present a large variation across studies. That, however, is hardly ever achieved for the diversity of pedoclimatic conditions.
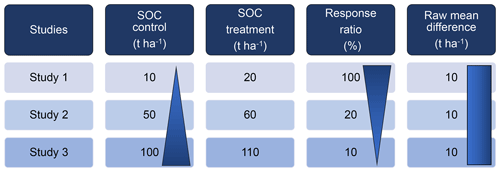
Figure 9Example of the relationship between the SOC levels in the control and effect sizes measured as a response ratio or raw mean difference for three studies. The response ratio indicates increasing effect size with decreasing control level. The raw mean difference indicates equal effect sizes for all experiments and does not consider variation in control levels. Triangles indicate an increase or decrease in values; the rectangle indicates constant values.
Weighting is essential, as different studies have different precision, and more precise studies with larger sample sizes need to be more heavily weighted in an analysis. The weighting should be done by 1/variance. Applying the weighting in other ways (e.g., by sample size) can lead to several problems, such as the introduction of unknown biases (e.g., Maillard and Angers, 2014; Han et al., 2016). When studies are not weighted at all (e.g., King and Blesh, 2018), the variation within and between studies is not separated. Therefore, common-effects and random-effects models are not useable, leading to difficulties in assessing heterogeneity (Gurevitch et al., 2018). All of these possible biases can adulterate the results of meta-analyses and, therefore, lead to false conclusions. According to findings by Hungate et al. (2009), depending on the functions used for weighting, differences in mean estimates of the effect sizes can be found. Weighting by sample size or not weighting resulted in comparable effect size estimates which were often larger than when weighted by 1/variance. Our assessment showed that only 13 % of SOC meta-analyses were weighted by 1/variance, whereas Philibert et al. (2012) found 37 % compliance. Koricheva and Gurevitch (2014) reported that three-quarters of meta-analyses were weighted by 1/variance. Meta-analyses studied by Krupnik et al. (2019) were weighted by sample size and, therefore, are not correctly conducted according to our set of criteria. Beillouin et al. (2019) found that 40 % of meta-analyses, studying diversification effects, were weighted by 1/variance (and, in some cases, by sample size).
When using a random-effects or mixed-effects model, effect sizes might show a certain amount of variability that cannot be explained by sampling errors alone, raising the question of whether moderator effects may have influenced the results. A moderator is a third variable that conditions the relations between two others. Therefore, moderator analysis must be conducted to identify their effects (Lipsey, 2019). In agricultural and soil sciences, abiotic factors (e.g., climatic zone, temperature, soil pH, and clay content) as well as other applied management practices can moderate the results and should subsequently be accounted for (Valkama et al., 2015). Moderators can be analyzed by subgroup analysis or meta-regression. Subgroup analysis is suitable for categorical moderators which can be described in the form of groups, such as climate zone (e.g., tropical, continental, and Mediterranean). In contrast, meta-regression is suitable for continuous moderators (e.g., duration of experiment, and soil pH). We found that moderator analysis in the form of a Q test was performed by about half of the analyzed SOC meta-analyses. Results from the reviews of Philibert et al. (2012), Koricheva and Gurevitch (2014), and Beillouin et al. (2019) showed that meta-analyses in agri-environment, plant ecology, and conservation agriculture complied almost twice as much with this criterion.
Another issue frequently found in meta-analyses is the nonindependence of effect size estimates, which occurs when effect sizes are not extracted independently and are instead somehow related to each other – for example, observations from different soil layers, from different treatment levels, or from sites located nearby that share the same pedoclimatic conditions. This nonindependence can lead to the underestimation of the standard error of the mean effect and, subsequently, can impact the free evaluations of the effects' statistical significance. Therefore, meta-analysts should be aware of the sources of nonindependence and should select only one effect size among several related effect sizes (Gurevitch and Hedges, 1999; Nakagawa et al., 2017). An example would be the inclusion of only the treatment effect of cover crop mix A on SOC, compared with a control with no cover crops, although the results of several other mixes (B, C, and D) are available too. As they have been conducted in different plots but on the same site, they share the same control and pedoclimatic characteristics and, therefore, are not independent. The same applies to several observations (e.g., SOC) taken from multiple sublayers/horizons or varying treatment levels (e.g., fertilization experiments). It should also be acknowledged that, in order to conduct a high-quality meta-analysis, the number of included independent studies/experiments from primary articles should be sufficient to allow the calculation of a rigorous overall effect estimate and to study the source of variation across studies. Hedges et al. (1999) structured the sample size requirements as follows: n≥50, a large body of primary data; , intermediate; n≤20, small. It is recommended to include at least 50 independent studies in a meta-analysis in order to obtain reasonably accurate 95 % confidence intervals for effect sizes.
Lastly, the degree of sensitivity of meta-analytical results should be assessed. When results are sensitive to factors such as publication bias, it is indicated that these factors need specific attention (Koricheva and Gurevitch, 2014). Funnel plots can support the interpretation of statistics by visualizing bias and highlighting outliers (Borenstein et al., 2009); these outliers should then be excluded, and the analyses should be conducted without them to see if the overall results are affected (Rothstein et al., 2013). Another possibility is the testing via the fail-safe number. The computation of this number allows us to detect how many additional studies it would take to reduce the overall effect to a nonsignificant one (Rosenthal's method) or an arbitrary minimal level (Orwin's method) (Borenstein et al., 2009). Philibert et al. (2012) reported that less than 10 % of meta-analyses conducted sensitivity analysis. About 30 % of SOC meta-analyses fulfilled this criterion. Beillouin et al. (2019) and Krupnik et al. (2019) found that about 40 % of meta-analyses conducted sensitivity analysis, whereas Koricheva and Gurevitch (2014) found a higher agreement of their meta-analyses or reviews with this criterion.
4.3 Results and database presentation
In the “Result and database presentation” group, the presentation and availability of results and full databases, which give all necessary information to reproduce an analysis, were compared. Extracted data should be provided to an extent sufficient to inform readers about all subsequent synthesis work (Woodcock et al., 2014).
The results of the moderator analysis should be displayed in the form of figures or tables. For subgroup analysis, a summary forest plot (see Fig. 1c in Gurevitch et al., 2018) is suitable. This plot should not be confused with the classic forest plot, which shows all calculated effect sizes, corresponding confidence intervals, and summary effect size. Meta-regression can be displayed in the form of, e.g., a bubble plot (see Fig. 1d in Gurevitch et al., 2018).
Finally, if sufficient data are not provided to update studies, information must be gathered once again, causing redundant work. Full data sets promote data use by others and enable updates and the detection of errors (Koricheva and Gurevitch, 2014). Of all five reviews, our findings complied least with this criterion (Table 5). Only 16 % of SOC meta-analyses reported databases, including all relevant information to allow the recalculation of effect sizes. Overall, results were poor. Philibert et al. (2012) received similar results, Koricheva and Gurevitch (2014) and Beillouin et al. (2019) found higher correspondences, and Krupnik et al. (2019) identified the highest agreement (over 70 %) with the criterion. This might be explained by the small sample size or less demanding criteria, as in our analysis of the Literature search reported criterion.
4.4 Management categories
The results (Table 4) show that the management category “Tillage” was studied by 15 meta-analyses, with the highest score of 29 by the meta-analysis of Haddaway et al. (2017), who provided an in-depth and high-quality synthesis of no-tillage/reduced-tillage versus conventional-tillage effects on SOC at a global level using the raw mean difference as the effect size. A review of agricultural meta-analyses recently published by Young et al. (2021) found 14 meta-studies looking at the effects of no tillage on SOC. Beillouin et al. (2021), who provide findings of the available meta-analyses studying various land management practices at a global scale, identified over 20 studies on tillage effects. Therefore, we suggest that the topic is well covered for the moment, and no further global meta-analyses are needed until there are a substantial number of new publications or new potential moderator effects of interest. Nevertheless, according to our findings, high-quality meta-analyses and systematic reviews studying tillage effects on SOC in specific pedoclimatic zones or continents, such as Europe, are still missing.
The maximum score (16) in the organic management category was reached by the publication of García-Palacios et al. (2018), which lacked in-depth reporting of the search strategy and independency of effect sizes, used studies where pedotransfer functions were applied, did not check for outliers, only partly extracted SDs, and thus weighted partly by 1/variance. Regarding the effect of cover crops on SOC, Jian et al. (2020) produced the meta-analysis that reached the highest score (21) out of six meta-analyses in this category. Reporting of literature searches and effect size calculations was conducted well, but the study failed to calculate moderator effects and to conduct sensitivity analysis, had nonindependent effect sizes, and included studies with pedotransfer function application. Lessmann et al. (2022), who evaluated the global variation in SOC sequestration through improved cropland management, found six meta-studies analyzing cover crop effects on SOC. In the “Crop residue” category, the maximum score of 21 was reached by the meta-analysis of Li et al. (2020). Literature search reporting, effect size calculation, and moderator analysis was done well, but effect sizes were not extracted independently, outliers were not assessed, and a full database was not provided. Maximum scores in all of the other management categories were not above 18. Therefore, we conclude that there is a need for further and improved meta-analyses on all management categories, except no tillage/reduced tillage versus conventional tillage.
4.5 Impact of meta-analysis quality on policy-making
In our quick quality assessment of meta-analyses cited in Chapter 2: Land–climate interactions of the IPCC Special Report – Climate Change and Land (Jia et al., 2019), we found that over 50% of studies (9 out of 16) that used the term meta-analysis in their title were in fact not true meta-analyses, as they did not fulfill the cutoff criteria. As not even the key criteria for conducting a meta-analysis were followed by these articles, the quality of the overall work and, therefore, the reliability of their results is unsure. In a study by O'Leary et al. (2016), 92 reviews were assessed with respect to their value for decision-making with the help of CEESAT (Woodcock et al., 2014; Collaboration for Environmental Evidence, 2020), which contains elements for analyzing transparency, objectivity, and comprehensiveness. They found that the evidence reviews performed poorly, with a median score of 2.5 (of a possible 39). Furthermore, many of these reviews showed low reliability with respect to their methodology, which enhances the risk that the current knowledge is not adequately reflected. They concluded that “such reviews thus have the potential to misinform decision-making, especially if selectively used by stakeholders with particular priorities“ (O'Leary et al., 2016, p. 80).
Scientific literature is increasingly used for environmental management decision-making (Dicks et al., 2014). Especially documents that synthesize the results of multiple studies and peer-reviewed publications are primary sources of information for respondents (Seavy and Howell, 2010). Although science is by far not the only factor influencing policy decisions, there have been cases in which scientific findings have had crucial impacts on policy changes (Pullin and Knight, 2012). Therefore, researchers are obligated to ensure that their evidence reviews (such as meta-analyses) accurately reflect the primary evidence base and are reliable and transparent (O'Leary et al., 2016).
4.6 How to fix the problem
The described limitations call for advances in meta-analyses conducted in soil and agricultural research. Firstly, to improve the overall quality, it is crucial to support education at the university level and implement training for interested scientists and stakeholders. Gurevitch et al. (2018) stressed that such training should be part of the curriculum for higher-degree students. Furthermore, they point out that not only scientists but also editors, reviewers, and science–policy practitioners would greatly benefit from knowledge on meta-analytical methodology, as it would enable them to assess the quality of meta-analyses and interpret results.
Secondly, readers of meta-analyses should check for the presence of key elements assuring the transparency and replicability of the article (Lortie et al., 2015). Krupnik et al. (2019) argue that scientists and policymakers need to evaluate meta-analyses critically regarding treatment definition, data collection, and analysis. The results of meta-analyses on highly politicized agronomic topics should be interpreted especially carefully. We fully agree with these claims and support the appeal to be critical when it comes to meta-analytical outcomes. The proposed set of quality criteria should aid this demanding process.
An issue that meta-analysts frequently face, is that many primary publications do not report SDs, which are needed to calculate variance and subsequently weight studies by 1/variance. As a result, many studies cannot be included in the meta-analysis, thereby reducing the amount of valuable information needed to gain rigorous results. To solve this issue, a new tool named “EX-TRACT” was recently developed (Acutis et al., 2022). The easy-to-use Excel worksheet application allows one to obtain pooled error standard deviations (sw) from ANOVA and multiple comparison test (MCT) outcomes. By using this tool, we can double the number of studies that can be included in a meta-analysis (Acutis et al., 2022) and avoid discarding primary literature which fits our scope.
Another available and highly useful tool allows the computation of SOC stock and its SD for a single soil layer based on SOC concentration and bulk density (also from multiple sublayers) (Tadiello et al., 2022). The Excel workbook automatically computes the means of stocks and SDs, saving the results in a ready-to-use database. This is especially helpful when conducting a meta-analysis. As, in original articles, SOC observations are often presented for multiple sublayers but not for the complete soil profile, meta-analysts tend to extract all available observations per a study, leading to a nonindependence of effect sizes. With the help of this tool, it is possible to “fuse” the results from all layers into one, independent effect size.
The publication of protocols prior to a meta-analysis would benefit the method by allowing constructive criticism and suggestions for improvement by the scientific community (Moher et al., 2015; Brandt et al., 2013). Gurevitch et al. (2018) described that the preregistration of planned meta-analyses, which are then peer-reviewed and published before the actual analysis is conducted, can aid the reduction of selective reporting and publication bias. Systematic review protocols for environmental sciences from the Environmental Evidence journal or the ROSES initiative are available and can be used for the construction of meta-analytical protocols. Protocols then can be published in suitable journals (e.g., MethodsX)
Lastly, another viable asset in improving the quality of future meta-analyses in soil science would be the creation of a European meta-analysis hub that focuses on (1) the development of high-quality products, (2) the assessment of quality, and (3) the creation of a European database. The database should comprise all available information of former meta-analyses on soil and agricultural research, providing researchers with valuable data. With the help of this database, new meta-analyses, studying management practices relevant for the pedoclimatic zones present in Europe, could be conducted. This is important, as the inclusion of global experiments into an analysis can lead to overdiversification and, therefore, to the combination of “apples and oranges”, which is not expedient.
Quality assessment of meta-analyses, especially in the complex agricultural setup, allows for the detection of rigorous synthesis efforts and their distinction from work with lower quality. Meta-analyses in soil and agricultural research may encounter specific issues, which differ from those encountered in other fields like medicine, environment, or ecology. Therefore, we adapted meta-analytical guidelines from other disciplines to construct an easy-to-use set of criteria suited to quantitatively assess the quality of meta-analyses in agriculture and soil sciences. With the help of these criteria, we further investigated the quality of 31 meta-analyses studying the effects of agricultural management practices on SOC. By doing so, we aimed to present the application of the criteria set and analyze the quality of quantitative reviews within this prominent topic. Our analysis showed that the overall quality of analyses improved over time, but only one achieved a high score. Deficits were found in literature search, statistical analyses, and data presentation. The correct weighting of effect sizes by 1/variance was found to be a challenge for many authors. In some cases, the term meta-analysis is still falsely used to describe quantitative syntheses of any style, independent of the methodology applied. The analysis also revealed that out of 11 identified management categories studied by the meta-analyses, only the effects of no tillage/reduced tillage versus conventional tillage on SOC have been studied sufficiently in the form of a high-quality meta-analytical synthesis.
Our results indicate that the quality of meta-analyses in agricultural and soil sciences is, despite all efforts, still not satisfactory. As the information presented in summarizing research articles is frequently used by decision-makers, this can also have negative impacts on evidence-based policy-making. It is high time that the agricultural and soil scientific community adapts rigorous meta-analytical methodologies and improves the quality of its output. We believe that the method is a viable and indispensable tool in the quantitative synthesis of agricultural and soil research, and only with combined efforts and collaborations between stakeholders across disciplines will we be able to overcome the presented challenges.
No data sets were produced in this work. The data sets collated by meta-analyses are available from the sources listed in Table A1.
The supplement related to this article is available online at: https://doi.org/10.5194/soil-9-117-2023-supplement.
JF and EV compiled the set of quality criteria and performed the quality assessment. SZB initiated the study and raised the funding. All authors contributed to the conceptual planning of the study as well as to writing the paper and interpreting the results. All authors provided input on earlier versions of the manuscript and read and approved of the final submission.
The contact author has declared that none of the authors has any competing interests.
Publisher's note: Copernicus Publications remains neutral with regard to jurisdictional claims in published maps and institutional affiliations.
The authors wish to thank Marco Acutis, Damien Beillouin, and Tommaso Tadiello for their thoughtful comments. We are also grateful to Dylan Goff for proofreading the manuscript.
This project has received funding from the European Union's Horizon 2020 Research and Innovation program (grant agreement no. 862695 EJP SOIL).
This paper was edited by Raphael Viscarra Rossel and reviewed by John Koestel and Bas van Wesemael.
Acutis, M., Tadiello, T., Perego, A., di Guardo, A., Schillaci, C., and Valkama, E.: EX-TRACT: An excel tool for the estimation of standard deviations from published articles, Environ. Model. Softw., 147, 105236, https://doi.org/10.1016/j.envsoft.2021.105236, 2022.
Aguilera, E., Lassaletta, L., Gattinger, A., and Gimeno, B. S.: Managing soil carbon for climate change mitigation and adaptation in Mediterranean cropping systems: A meta-analysis, Agr. Ecosyst. Environ., 168, 25–36, https://doi.org/10.1016/j.agee.2013.02.003, 2013.
Angers, D. A. and Eriksen-Hamel, N. S.: Full-Inversion tillage and organic carbon distribution in soil profiles: A Meta-Analysis, Soil Sci. Soc. Am. J., 72, 1370–1374, https://doi.org/10.2136/sssaj2007.0342, 2008.
Aksnes, D. W., Langfeldt, L., and Wouters, P.: Citations, Citation Indicators, and Research Quality: An Overview of Basic Concepts and Theories, Sage Open, 9, https://doi.org/10.1177/2158244019829575, 2019.
Bai, X., Huang, Y., Ren, W., Coyne, M., Jacinthe, P.-A., Tao, B., Hui, D., Yang, J., and Matocha, C.: Responses of soil carbon sequestration to climate-smart agriculture practices: A meta-analysis, Glob. Chang. Biol., 25, 2591–2606, https://doi.org/10.1111/gcb.14658, 2019.
Beillouin, D., Ben-Ari, T., and Makowski, D.: Evidence map of crop diversification strategies at the global scale, Environ. Res. Lett., 14, 123001, https://doi.org/10.1088/1748-9326/ab4449, 2019.
Beillouin, D., Cardinael, R., Berre, D., Boyer, A., Corbeels, M., Fallot, A., Feder, F., and Demenois, J.: A global overview of studies about land management, land-use change, and climate change effects on soil organic carbon, Global Change Biol., 28, 1690–1702, https://doi.org/10.1111/gcb.15998, 2021.
Bolinder, M. A., Crotty, F., Elsen, A., Frac, M., Kismanyoky, T., Lipiec, J., Tits, M., Toth, Z., and Katterer, T.: The effect of crop residues, cover crops, manures and nitrogen fertilization on soil organic carbon changes in agroecosystems: a synthesis of reviews, Mitig. Adapt. Strat. Gl., 25, 929–952, https://doi.org/10.1007/s11027-020-09916-3, 2020.
Borenstein, M., Hedges, L. V., Higgins, J., and Rothstein, H.: Introduction to meta-analysis, Wiley, 421 pp., 2009.
Brandt, K., Sêrednicka-Tober, D., Baranìski, M., Sanderson, R., Leifert, C., and Seal, C.: Methods for Comparing Data across Differently Designed Agronomic Studies: Examples of Different Meta-analysis Methods Used to Compare Relative Composition of Plant Foods Grown Using Organic or Conventional Production Methods and a Protocol for a Systematic Review, J. Agr. Food Chem., 61, 7173–7180, https://doi.org/10.1021/jf4008967, 2013.
Chen, Y., Camps-Arbestain, M., Shen, Q., Singh, B., and Cayuela, M. L.: The long-term role of organic amendments in building soil nutrient fertility: a meta-analysis and review, Nutr. Cycl. Agroecosyst. 111, 103–125, https://doi.org/10.1007/s10705-017-9903-5, 2018.
Collaboration for Environmental Evidence: Guidelines and Standards for Evidence Synthesis in Environmental Management, Version 5, edited by: Pullin, A. S., Frampton, G. K., Livoreil, B., and Petrokofsky, G., https://environmentalevidence.org/information-for-authors/ (last access: 17 January 2023), 2018.
Collaboration for Environmental Evidence: The Collaboration for Environmental Evidence Synthesis Appraisal Tool (CEESAT), Version 2.1., https://environmentalevidence.org/ceeder/about-ceesat/ (last access: 17 January 2023), 2020.
Cooper, J., Baranski, M., Stewart, G., Nobel-de Lange, M., Bàrberi, P., Fließbach, A., Peigné, J., Berner, A., Brock, C., Casagrande, M., Crowley, O., David, C., De Vliegher, A., Döring, T. F., Dupont, A., Entz, M., Grosse, M., Haase, T., Halde, C., Hammerl, V., Huiting, H., Leithold, G., Messmer, M., Schloter, M., Sukkel, W., van der Heijden, M. G. A., Willekens, K., Wittwer, R., and Mäder, P.: Shallow non-inversion tillage in organic farming maintains crop yields and increases soil C stocks: a meta-analysis, Agron. Sustain. Dev., 36, https://doi.org/10.1007/s13593-016-0354-1, 2016.
Cooper, H., Hedges, L. V., and Valentine, J. C.: Potentials and Limitations of Research Synthesis, in: The Handbook of Research Synthesis and Meta-Analysis, edited by: Cooper, H., Hedges, L. V., and Valentine, J. C., Russell Sage Foundation, 518–525, 2019a.
Cooper, H., Hedges, L. V., and Valentine, J. C.: Research Synthesis as a Scientific Process, in: The Handbook of Research Synthesis and Meta-Analysis, edited by: Cooper, H., Hedges, L. V., and Valentine, J. C., Russell Sage Foundation, New York, 4–15, 2019b.
Cooper, H., Hedges, L. V., and Valentine, J. C.: The handbook of research synthesis and meta-analysis, 3rd ed., Russell Sage Foundation, New York, 556 pp., 2019c.
Côté, I. M., Curtis, P. S., Rothstein, H. R., and Stewart, G. B.: Gathering Data: searching Literature and selection Criteria, Princeton University Press, 37–51, 2013.
Culina, A., Crowther, T. W., Ramakers, J. J. C., Gienapp, P., and Visser, M. E.: How to do meta-analysis of open datasets, Nat. Ecol. Evol., 2, 1053–1056, https://doi.org/10.1038/s41559-018-0579-2, 2018.
Curtis, P. S., Mengersen, K., Lajeunesse, M. J., Rothstein, H. R., and Stewart, G. B.: Extraction and Critical appraisal of Data, in: Handbook of Meta-analysis in Ecology and Evolution, edited by: Koricheva, J., Gurevitch, J. and Mengersen, K., Princeton University Press, Princeton, 52–60, ISBN 9780691137285, 2013.
Dicks, L. V., Walsh, J. C., and Sutherland, W. J.: Organising evidence for environmental management decisions: A “4S” hierarchy, Trends Ecol. Evol., 29, 607–613, https://doi.org/10.1016/j.tree.2014.09.004, 2014.
Feng, Q., An, C., Chen, Z., and Wang, Z.: Can deep tillage enhance carbon sequestration in soils?, A meta-analysis towards GHG mitigation and sustainable agricultural management, Renew. Sustain. Energy Rev., 133, 110293, https://doi.org/10.1016/j.rser.2020.110293, 2020.
García-Palacios, P., Gattinger, A., Bracht-Jørgensen, H., Brussaard, L., Carvalho, F., Castro, H., Clément, J.-C., De Deyn, G., D'Hertefeldt, T., Foulquier, A., Hedlund, K., Lavorel, S., Legay, N., Lori, M., Mäder, P., Martínez-García, L. B., da Silva, P., Muller, A., Nascimento, E., Reis, F., Symanczik, S., Paulo Sousa, J., and Milla, R.: Crop traits drive soil carbon sequestration under organic farming, J. Appl. Ecol., 55, 2496–2505, https://doi.org/10.1111/1365-2664.13113, 2018.
Gattinger, A., Muller, A., Haeni, M., Skinner, C., Fliessbach, A., Buchmann, N., Mäder, P., Stolze, M., Smith, P., Scialabba, N. E. H., and Niggli, U.: Enhanced top soil carbon stocks under organic farming, P. Natl. Acad. Sci. USA, 109, 18226–18231, https://doi.org/10.1073/pnas.1209429109, 2012.
Glass, G. V: Primary, Secondary, and Meta-Analysis of Research, Educ. Res., 5, 3–8, https://doi.org/10.3102/0013189X005010003, 1976.
Gonçalves, R. S. and Musen, M. A.: Analysis: The variable quality of metadata about biological samples used in biomedical experiments, Sci. Data, 6, 1–15, https://doi.org/10.1038/sdata.2019.21, 2019.
González-Sánchez, E. J., Ordóñez-Fernández, R., Carbonell-Bojollo, R., Veroz-González, O., and Gil-Ribes, J. A.: Meta-analysis on atmospheric carbon capture in Spain through the use of conservation agriculture, Soil Tillage Res., 122, 52–60, https://doi.org/10.1016/j.still.2012.03.001, 2012.
Gurevitch, J. and Hedges, L. V.: Statistical Issues in Ecological Meta-Analyses, Ecology, 80, 1142, https://doi.org/10.2307/177061, 1999.
Gurevitch, J., Koricheva, J., Nakagawa, S., and Stewart, G.: Meta-analysis and the science of research synthesis, Nature, 555, 175–182, https://doi.org/10.1038/nature25753, 2018.
Haddaway, N. R., Hedlund, K., Jackson, L. E., Katterer, T., Lugato, E., Thomsen, I. K., Jorgensen, H. B., and Isberg, P.-E.: How does tillage intensity affect soil organic carbon?, A systematic review, Environ. Evid., 6, https://doi.org/10.1186/s13750-017-0108-9, 2017.
Haddaway, N. R., Bethel, A., Dicks, L. V., Koricheva, J., Macura, B., Petrokofsky, G., Pullin, A. S., Savilaakso, S., and Stewart, G. B.: Eight problems with literature reviews and how to fix them, Nat. Ecol. Evol., 4, 1582–1589, https://doi.org/10.1038/s41559-020-01295-x, 2020.
Han, P., Zhang, W., Wang, G., Sun, W., and Huang, Y.: Changes in soil organic carbon in croplands subjected to fertilizer management: a global meta-analysis, Sci. Rep., 6, 27199, https://doi.org/10.1038/srep27199, 2016.
Hedges, L. V., Gurevitch, J., and Curtis, P. S.: The Meta-Analysis of Response Ratios in Experimental Ecology, Ecology, 80, 1150, https://doi.org/10.2307/177062, 1999.
Hungate, B. A., van Groenigen, K. J., Six, J., Jastrow, J. D., Luo, Y., de Graaff, M. A., van Kessel, C., and Osenberg, C. W.: Assessing the effect of elevated carbon dioxide on soil carbon: A comparison of four meta-analyses, Global Change Biol., 15, 2020–2034, https://doi.org/10.1111/j.1365-2486.2009.01866.x, 2009.
IPCC: Revised 1996 IPCC guidelines for national greenhouse gas inventories: Reference Manual (Volume 3), edited by: Houghton, J. T., Meira, L. G., Filho, L. G., Lim, B., Treanton, K., Mamaty, I., Bonduki, Y., Griggs, D. J. and Callender, B. A. (Eds), Intergovernmental Panel on Climate Change, Bracknell, United Kingdom, 1997.
IPCC, 2019: Summary for Policymakers. In: Climate Change and Land: an IPCC special report on climate change, desertification, land degradation, sustainable land management, food security, and greenhouse gas fluxes in terrestrial ecosystems, edited by: Shukla, P. R., Skea, J., Calvo Buendia, E., Masson-Delmotte, V., Pörtner, H. O., Roberts, D. C., Zhai, P., Slade, R., Connors, S., van Diemen, R., Ferrat, M., Haughey, E., Luz, S., Neogi, S., Pathak, M., Petzold, J., Portugal Pereira, J., Vyas, P., Huntley, E., Kissick, K., Belkacemi, M., and Malley, J., https://doi.org/10.1017/9781009157988.001, 2019.
Jamshidi, L., Heyvaert, M., Declercq, L., Fernández-Castilla, B., Ferron, J. M., Moeyaert, M., Beretvas, S. N., Onghena, P., and van den Noortgate, W.: Methodological quality of meta-analyses of single-case experimental studies, Res. Dev. Disabil., 79, 97–115, https://doi.org/10.1016/j.ridd.2017.12.016, 2018.
Jia, G., Shevliakova, E., Artaxo, P., de Noblet-Ducoudré, N., Houghton, R., House, J., Kitajima, K., Lennard, C., Popp, A., Sirin, A., Sukumar, R., and Verch, L.: Land–climate interactions, in: Climate Change and Land: an IPCC special report on climate change, desertification, land degradation, sustainable land management, food security, and greenhouse gas fluxes in terrestrial ecosystems, edited by: Shukla, P. R., Skea, J., Calvo Buendia, E., Masson-Delmotte, V., Pörtner, H.-O., Roberts, D. C., Zhai, P., Slade, R., Connors, S., van Diemen, R., Ferrat, M., Haughey, E., Luz, S., Neogi, S., Pathak, M., Petzold, J., Portugal Pereira, J., Vyas, P., Huntley, K., Kissick, K., Belkacemi, M., and Malley, J., https://doi.org/10.1017/9781009157988.004, 2019.
Jian, J., Du, X., Reiter, M. S., and Stewart, R. D.: A meta-analysis of global cropland soil carbon changes due to cover cropping, Soil Biol. Biochem., 143, 107735, https://doi.org/10.1016/j.soilbio.2020.107735, 2020.
King, A. E. and Blesh, J.: Crop rotations for increased soil carbon: Perenniality as a guiding principle: Perenniality, Ecol. Appl., 28, 249–261, https://doi.org/10.1002/eap.1648, 2018.
Kopittke, P. M., Dalal, R. C., Finn, D., and Menzies, N. W.: Global changes in soil stocks of carbon, nitrogen, phosphorus, and sulphur as influenced by long-term agricultural production, Global Change Biol., 23, 2509–2519, https://doi.org/10.1111/gcb.13513, 2017.
Koricheva, J. and Gurevitch, J.: Place of Meta-analysis among other Methods of research synthesis, in: Handbook of Meta-analysis in Ecology and Evolution, edited by: Koricheva, J., Gurevitch, J., and Mengersen, K., Princton University Press, Princeton, 3–13, 2013a.
Koricheva, J. and Gurevitch, J.: Uses and misuses of meta-analysis in plant ecology, J. Ecol., 102, 828–844, https://doi.org/10.1111/1365-2745.12224, 2014.
Koricheva, J., Gurevitch, J., and Mengersen, K. (Eds.): Handbook of Meta-analysis in Ecology and Evolution, Princeton University Press, 520 pp., 2013b.
Krupnik, T. J., Andersson, J. A., Rusinamhodzi, L., Corbeels, M., Shennan, C., and Gérard, B.: Does size matter?, A critical review of meta-analysis in agronomy, Exp. Agric., 55, 200–229, https://doi.org/10.1017/S0014479719000012, 2019.
Ladha, J. K., Reddy, C. K., Padre, A. T., and van Kessel, C.: Role of Nitrogen Fertilization in Sustaining Organic Matter in Cultivated Soils, J. Environ. Qual., 40, 1756–1766, https://doi.org/10.2134/jeq2011.0064, 2011.
Lessmann, M., Ros, G. H., Young, M. D., and de Vries, W.: Global variation in soil carbon sequestration potential through improved cropland management, Global Change Biol., 28, 1162–1177, https://doi.org/10.1111/gcb.15954, 2022.
Leydesdorff, L., Bornmann, L., Comins, J. A., and Milojević, S.: Citations: Indicators of Quality? The Impact Fallacy, Front. Res. Metr. Anal., 1, https://doi.org/10.3389/frma.2016.00001, 2016.
Li, Y., Li, Z., Chang, S. X., Cui, S., Jagadamma, S., Zhang, Q., and Cai, Y.: Residue retention promotes soil carbon accumulation in minimum tillage systems: Implications for conservation agriculture, Sci. Total Environ., 740, 140147, https://doi.org/10.1016/j.scitotenv.2020.140147, 2020.
Lipsey, M. W.: Identifying Potentially Interesting Variables And Analysis Opportunities, in: The Handbook of Research Synthesis and Meta-Analysis, edited by: Cooper, H., Hedges, L. V., and Valentine, J. C., Russell Sage Foundation, New York, 142–151, 2019.
Liu, S., Zhang, Y., Zong, Y., Hu, Z., Wu, S., Zhou, J., Jin, Y., and Zou, J.: Response of soil carbon dioxide fluxes, soil organic carbon and microbial biomass carbon to biochar amendment: a meta-analysis, Global Change Biol., 8, 392–406, https://doi.org/10.1111/gcbb.12265, 2016.
Lortie, C. J., Lau, J., and Lajeunesse, M. J.: Graphical Presentation of results, in: Handbook of Meta-analysis in Ecology and Evolution, edited by: Koricheva, J., Gurevitch, J., and Mengersen, K., Princeton University Press, Princeton, 339–347, 2013.
Lortie, C. J., Stewart, G., Rothstein, H., and Lau, J.: How to critically read ecological meta-analyses, Res. Synth. Meth., 6, 124–133, https://doi.org/10.1002/jrsm.1109, 2015.
Luo, Z., Wang, E., and Sun, O. J.: Can no-tillage stimulate carbon sequestration in agricultural soils?, A meta-analysis of paired experiments, Agr. Ecosyst. Environ., 139, 224–231, https://doi.org/10.1016/j.agee.2010.08.006, 2010.
Maillard, É. and Angers, D. A.: Animal manure application and soil organic carbon stocks: A meta-analysis, Glob. Chang. Biol., 20, 666–679, https://doi.org/10.1111/gcb.12438, 2014.
Majumder, S., Neogi, S., Dutta, T., Powel, M. A., and Banik, P.: The impact of biochar on soil carbon sequestration: Meta-analytical approach to evaluating environmental and economic advantages, J. Environ. Manage., 250, 109466, https://doi.org/10.1016/j.jenvman.2019.109466, 2019.
Mäkipää, R., Muukkonen, P., and Peltoniemi, M.: the costs of monitoring changes in forest soil carbon stocks, Boreal Environ. Res., 13 (suppl. B), 120–130, ISSN 1797-2469, 2008.
Mathew, I., Shimelis, H., Mutema, M., Minasny, B., and Chaplot, V.: Crops for increasing soil organic carbon stocks – A global meta analysis, Geoderma, 367, 114230, https://doi.org/10.1016/j.geoderma.2020.114230, 2020.
Mayo-Wilson, E. and Grant, S.: Transparent Reporting: Registrations, Protocols, and Final Reports, in: Handbook of Meta-analysis in Ecology and Evolution, edited by: Cooper, H., Hedges, L. V., and Valentine, J. C., Russell Sage Foundation, New York, 471–488, 2019.
McDaniel, M. D., Tiemann, L. K., and Grandy, A. S.: Does agricultural crop diversity enhance soil microbial biomass and organic matter dynamics?, A meta-analysis, Ecol. Appl., 24, 560–570, https://doi.org/10.1890/13-0616.1, 2014.
Meurer, K. H. E., Haddaway, N. R., Bolinder, M. A., and Kätterer, T.: Tillage intensity affects total SOC stocks in boreo-temperate regions only in the topsoil – A systematic review using an ESM approach, Earth-Sci. Rev., 177, 613–622, https://doi.org/10.1016/j.earscirev.2017.12.015, 2018.
Minasny, B., Malone, B. P., McBratney, A. B., Angers, D. A., Arrouays, D., Chambers, A., Chaplot, V., Chen, Z. S., Cheng, K., Das, B. S., Field, D. J., Gimona, A., Hedley, C. B., Hong, S. Y., Mandal, B., Marchant, B. P., Martin, M., McConkey, B. G., Mulder, V. L., O'Rourke, S., Richer-de-Forges, A. C., Odeh, I., Padarian, J., Paustian, K., Pan, G., Poggio, L., Savin, I., Stolbovoy, V., Stockmann, U., Sulaeman, Y., Tsui, C. C., Vågen, T. G., van Wesemael, B., and Winowiecki, L.: Soil carbon 4 per mille, Geoderma, 292, 59–86, https://doi.org/10.1016/j.geoderma.2017.01.002, 2017.
Moher, D., Shamseer, L., Clarke, M., Ghersi, D., Liberati, A., Petticrew, M., Shekelle, P., Stewart, L. A., and Group, P.-P.: Preferred reporting items for systematic review and meta-analysis protocols (PRISMA-P) 2015 statement, Syst. Rev., 4, 1–9, https://doi.org/10.1186/2046-4053-4-1, 2015.
Mondal, S., Chakraborty, D., Bandyopadhyay, K., Aggarwal, P., and Rana, D. S.: A global analysis of the impact of zero-tillage on soil physical condition, organic carbon content, and plant root response, L. Degrad. Dev., 31, 557–567, https://doi.org/10.1002/ldr.3470, 2020.
Nakagawa, S. and Cuthill, I. C.: Effect size, confidence interval and statistical significance: A practical guide for biologists, Biol. Rev., 82, 591–605, https://doi.org/10.1111/j.1469-185X.2007.00027.x, 2007.
Nakagawa, S., Noble, D. W. A., Senior, A. M., and Lagisz, M.: Meta-evaluation of meta-analysis: Ten appraisal questions for biologists, BMC Biol., 15, 1–14, https://doi.org/10.1186/s12915-017-0357-7, 2017.
O'Dea, R. E., Lagisz, M., Jennions, M. D., Koricheva, J., Noble, D. W. A., Parker, T. H., Gurevitch, J., Page, M. J., Stewart, G., Moher, D., and Nakagawa, S.: Preferred reporting items for systematic reviews and meta-analyses in ecology and evolutionary biology: a PRISMA extension, Biol. Rev., 96, 1695–1722, https://doi.org/10.1111/brv.12721, 2021.
Ogle, S. M., Breidt, F. J., and Paustian, K.: Agricultural management impacts on soil organic carbon storage under moist and dry climatic conditions of temperate and tropical regions, Biogeochemistry, 72, 87–121, https://doi.org/10.1007/s10533-004-0360-2, 2005.
O'Leary, B. C., Kvist, K., Bayliss, H. R., Derroire, G., Healey, J. R., Hughes, K., Kleinschroth, F., Sciberras, M., Woodcock, P., and Pullin, A. S.: The reliability of evidence review methodology in environmental science and conservation, Environ. Sci. Pol., 64, 75–82, https://doi.org/10.1016/j.envsci.2016.06.012, 2016.
Page, M. J., McKenzie, J. E., Bossuyt, P. M., Boutron, I., Hoffmann, T. C., Mulrow, C. D., Shamseer, L., Tetzlaff, J. M., Akl, E. A., Brennan, S. E., Chou, R., Glanville, J., Grimshaw, J. M., Hróbjartsson, A., Lalu, M. M., Li, T., Loder, E. W., Mayo-Wilson, E., McDonald, S., McGuinness, L. A., Stewart, L. A., Thomas, J., Tricco, A. C., Welch, V. A., Whiting, P., and Moher, D.: The PRISMA 2020 statement: an updated guideline for reporting systematic reviews, BMJ, 372, https://doi.org/10.1136/bmj.n71, 2021.
Parker, T. H., Forstmeier, W., Koricheva, J., Fidler, F., Hadfield, J. D., Chee, Y. E., Kelly, C. D., Gurevitch, J., and Nakagawa, S.: Transparency in Ecology and Evolution: Real Problems, Real Solutions, Trend. Ecol. Evol., 31, 711–719, https://doi.org/10.1016/j.tree.2016.07.002, 2016.
Paustian, K., Lehmann, J., Ogle, S., Reay, D., Robertson, G. P., and Smith, P.: Climate-smart soils, 532, 49–57, https://doi.org/10.1038/nature17174, 2016.
Philibert, A., Loyce, C., and Makowski, D.: Assessment of the quality of meta-analysis in agronomy, Agr. Ecosyst. Environ., 148, 72–82, https://doi.org/10.1016/j.agee.2011.12.003, 2012.
Poeplau, C. and Don, A.: Carbon sequestration in agricultural soils via cultivation of cover crops – A meta-analysis, Agr. Ecosyst. Environ., 200, 33–41, https://doi.org/10.1016/j.agee.2014.10.024, 2015.
Pullin, A. S. and Knight, T. M.: Science informing Policy – A health warning for the environment, https://doi.org/10.1186/2047-2382-1-15, 2012.
Rothstein, H., Lortie, C. J., Stewart, G. B., Koricheva, J., and Gurevitch, J.: Quality standards for research syntheses, 323–339, ISBN 9780691137285, 2013.
Schillaci, C., Perego, A., Valkama, E., Märker, M., Saia, S., Veronesi, F., Lipani, A., Lombardo, L., Tadiello, T., Gamper, H. A., Tedone, L., Moss, C., Pareja-Serrano, E., Amato, G., Kühl, K., Dămătîrcă, C., Cogato, A., Mzid, N., Eeswaran, R., Rabelo, M., Sperandio, G., Bosino, A., Bufalini, M., Tunçay, T., Ding, J., Fiorentini, M., Tiscornia, G., Conradt, S., Botta, M., and Acutis, M.: New pedotransfer approaches to predict soil bulk density using WoSIS soil data and environmental covariates in Mediterranean agro-ecosystems, Sci. Total Environ., 780, 146609, https://doi.org/10.1016/j.scitotenv.2021.146609, 2021.
Seavy, N. E. and Howell, C. A.: How can we improve information delivery to support conservation and restoration decisions?, Biodivers. Conserv., 19, 1261–1267, https://doi.org/10.1007/s10531-009-9752-x, 2010.
Smith, P.: Soils and climate change, https://doi.org/10.1016/j.cosust.2012.06.005, 2012.
Smith, P., Andrén, O., Karlsson, T., Perälä, P., Regina, K., Rounsevell, M., and van Wesemael, B.: Carbon sequestration potential in European croplands has been overestimated, Global Change Biol., 11, 2153–2163, https://doi.org/10.1111/j.1365-2486.2005.01052.x, 2005.
Stewart, G.: Meta-analysis in applied ecology, Biol. Lett., 6, 78–81, https://doi.org/10.1098/rsbl.2009.0546, 2010.
Sun, W., Canadell, J. G., Yu, Lijun, Yu, Lingfei, Zhang, W., Smith, P., Fischer, T., and Huang, Y.: Climate drives global soil carbon sequestration and crop yield changes under conservation agriculture, Glob. Chang. Biol., 26, 3325–3335, https://doi.org/10.1111/gcb.15001, 2020.
Tadiello, T., Perego, A., Valkama, E., Schillaci, C., and Acutis, M.: Computation of total soil organic carbon stock and its standard deviation from layered soils, MethodsX, 9, 101662, https://doi.org/10.1016/j.mex.2022.101662, 2022.
Tubiello, F. N., Salvatore, M., Ferrara, A. F., House, J., Federici, S., Rossi, S., Biancalani, R., Condor Golec, R. D., Jacobs, H., Flammini, A., Prosperi, P., Cardenas-Galindo, P., Schmidhuber, J., Sanz Sanchez, M. J., Srivastava, N., and Smith, P.: The Contribution of Agriculture, Forestry and other Land Use activities to Global Warming, 1990–2012, Global Change Biol., 21, 2655–2660, https://doi.org/10.1111/gcb.12865, 2015.
Tuomisto, H. L., Hodge, I. D., Riordan, P., and Macdonald, D. W.: Does organic farming reduce environmental impacts? – A meta-analysis of European research, J. Environ. Manage., 112, 309–320, https://doi.org/10.1016/j.jenvman.2012.08.018, 2012.
Valkama, E., Virkajärvi, P., Uusitalo, R., Ylivainio, K., and Turtola, E.: Meta-analysis of grass ley response to phosphorus fertilization in Finland, Grass Forage Sci., 71, 36–53, https://doi.org/10.1111/gfs.12156, 2015.
Vetter, D., Rucker, G., and Storch, I.: Meta-analysis: A need for well-defined usage in ecology and conservation biology, Ecosphere, 4, 1–24, https://doi.org/10.1890/ES13-00062.1, 2013.
Virto, I., Barre, P., Burlot, A., and Chenu, C.: Carbon input differences as the main factor explaining the variability in soil organic C storage in no-tilled compared to inversion tilled agrosystems, Biogeochemistry, 108, 17–26, https://doi.org/10.1007/s10533-011-9600-4, 2012.
Woodcock, P., Pullin, A. S., and Kaiser, M. J.: Evaluating and improving the reliability of evidence syntheses in conservation and environmental science: A methodology, Biol. Conserv., 176, 54–62, https://doi.org/10.1016/j.biocon.2014.04.020, 2014.
Xia, L., Lam, S. K., Wolf, B., Kiese, R., Chen, D., and Butterbach-Bahl, K.: Trade-offs between soil carbon sequestration and reactive nitrogen losses under straw return in global agroecosystems, Glob. Chang. Biol., 24, 5919–5932, https://doi.org/10.1111/gcb.14466, 2018.
Xiao, L., Kuhn, N. J., Zhao, R., and Cao, L.: Net effects of conservation agriculture principles on sustainable land use: A synthesis, Global Change Biol., 27, 6321–6330, https://doi.org/10.1111/gcb.15906, 2021.
Xu, H., Sieverding, H., Kwon, H., Clay, D., Stewart, C., Johnson, J. M. F., Qin, Z., Karlen, D. L., and Wang, M.: A global meta-analysis of soil organic carbon response to corn stover removal, Global Change Biol., 11, 1215–1233, https://doi.org/10.1111/gcbb.12631, 2019.
Young, M. D., Ros, G. H., and de Vries, W.: Impacts of agronomic measures on crop, soil, and environmental indicators: A review and synthesis of meta-analysis, Agr. Ecosyst. Environ., 319, 107551, https://doi.org/10.1016/j.agee.2021.107551, 2021.