the Creative Commons Attribution 4.0 License.
the Creative Commons Attribution 4.0 License.
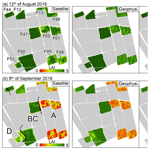
Added value of geophysics-based soil mapping in agro-ecosystem simulations
Johan A. Huisman
Lutz Weihermüller
Michael Herbst
Harry Vereecken
There is an increased demand for quantitative high-resolution soil maps that enable within-field management. Commonly available soil maps are generally not suited for this purpose, but digital soil mapping and geophysical methods in particular allow soil information to be obtained with an unprecedented level of detail. However, it is often difficult to quantify the added value of such high-resolution soil information for agricultural management and agro-ecosystem modelling. In this study, a detailed geophysics-based soil map was compared to two commonly available general-purpose soil maps. In particular, the three maps were used as input for crop growth models to simulate leaf area index (LAI) of five crops for an area of ∼ 1 km2. The simulated development of LAI for the five crops was evaluated using LAI obtained from multispectral satellite images. Overall, it was found that the geophysics-based soil map provided better LAI predictions than the two general-purpose soil maps in terms of correlation coefficient R2, model efficiency (ME), and root mean square error (RMSE). Improved performance was most apparent in the case of prolonged periods of drought and was strongly related to the combination of soil characteristics and crop type.
- Article
(12384 KB) - Full-text XML
- BibTeX
- EndNote
Detailed soil information on areas within a single field that require different treatment, so-called management zones, is key in agricultural management (King et al., 2005; Stafford et al., 1996; Sylvester-Bradley et al., 1999). An adequate characterization of such management zones can improve agricultural productivity and sustainability (King et al., 2005; Sylvester-Bradley et al., 1999) and help meet future food security challenges (Antle et al., 2017; Chartzoulakis and Bertaki, 2015). Technological advances in georeferencing, sensing, and computing have led to an increased possibility to apply within-field management strategies (Sylvester-Bradley et al., 1999). As a consequence, there is a growing demand for high-resolution soil maps that can substitute national- and regional-scale soil maps (Brevik et al., 2006), which are sometimes not available and often not suitable for agricultural applications and modelling (Della Chiesa et al., 2019; Nussbaum et al., 2018; Pätzold et al., 2008). However, high-resolution soil maps are generally costly to produce, and the added value of such detailed mapping products is typically difficult to assess.
Management zones are generally characterized by soils with relatively uniform characteristics (i.e. a soil unit). These soil units can potentially be obtained from a variety of commonly available thematic maps that provide spatially distributed soil information (e.g. geological, soil, and yield potential maps). However, these products are often insufficiently detailed (Franzen et al., 2002; Nawar et al., 2017; Robert, 1993) since they are discretized in relatively large polygons and provide qualitative information that might differ from the inputs that are useful for farmers (Krüger et al., 2013; Söderström et al., 2016). This is generally a consequence of the sparse point-scale soil sampling on which most of these maps are based (Gebbers and Adamchuk, 2010; Heuvelink and Webster, 2001), which is ∼ one point per hectare for highly detailed products (Rogge et al., 2018). Maps with higher sampling resolution such as the German soil taxation maps that were surveyed on a 50 m grid (four points per hectare) often do not provide improved results (Mertens et al., 2008). Denser sampling can be locally applied in order to obtain a more detailed and reliable soil characterization. However, this is time- and resource-consuming (King et al., 2005), and it is desirable to find more cost-effective mapping tools (Brevik et al., 2006).
Geophysical methods such as electromagnetic induction (EMI) have proven their potential in assisting agricultural applications (Binley et al., 2015) by providing a suitable alternative to dense soil sampling (Robinson et al., 2008). EMI measures the apparent electrical conductivity of the soil (ECa), which can be used to estimate soil characteristics and properties such as water content, textural properties, mineralization, porosity, and residual pore water content (Corwin and Lesch, 2005). Due to its high mobility, EMI can provide maps that range from the field to the catchment scale (Robinson et al., 2008). Despite these promising aspects, EMI has often been credited primarily for qualitative mapping (Binley et al., 2015). However, there is renewed interest in this technique because of the development of multi-coil instruments that allow multiple depths to be investigated simultaneously (Monteiro Santos et al., 2010; Saey et al., 2012; von Hebel et al., 2014), thus enabling the inversion of EMI data by combining such depths (Boaga, 2017; Mester et al., 2014; Tan et al., 2019; Von Hebel et al., 2019). Furthermore, EMI has already shown high potential for the determination of management zones in agricultural contexts (Brogi et al., 2019; Galambošová et al., 2014; King et al., 2005; Moral et al., 2010; Oldoni and Bassoi, 2016; Taylor et al., 2003; Terrón et al., 2015). Additional information about soil horizonation and texture can be added to EMI-derived maps using direct soil sampling and laboratory analysis (Brogi et al., 2019).
One drawback of EMI-derived soil maps is that they can only determine the geometry of potential management zones without directly providing information on the appropriate management of such areas (King et al., 2005). To increase their usefulness, geophysics-based soil maps can be used as input for process-oriented crop growth models (Brogi et al., 2020; Krüger et al., 2013) that account for factors limiting crop growth, such as water availability (Bonfante et al., 2015; Paz, 2000). Most process-oriented crop growth models rely on a 1D description of water flow in the soil column (Vereecken et al., 2016) and require detailed information on soil profile characteristics, including soil hydraulic properties (Boenecke et al., 2018). Some case studies in which geophysics-based soil information was successfully combined with crop growth models are available (Boenecke et al., 2018; Wong and Asseng, 2006). Recently, Brogi et al. (2020) successfully used inputs from an EMI-derived soil map to simulate soil water content dynamics and their effects on the growth of six crop types for a 90 ha study area. Furthermore, Krüger et al. (2013) showed that the consideration of soil depth derived from geophysical measurements improved the simulation of biomass production on a 4.4 ha experimental field compared to simulations based only on commonly available soil maps. Despite these promising results, there is need for further studies that link geophysics-based soil products and crop growth models. Moreover, the quantification of the added value of geophysics-based soil characterization for agro-ecosystem modelling applications has not been thoroughly investigated yet (Krüger et al., 2013), especially in areas larger than the field scale and for multiple crop and soil types.
The aim of this study is to assess the added value of a detailed soil map for agricultural applications in comparison to the use of commonly available soil maps. For this, a recently produced geophysics-based soil map (Brogi et al., 2019), a 1 : 5000 regional soil map (Röhrig, 1996), and a national soil taxation map (NRW, 1960) were used as input for the agro-ecosystem model AgroC. Simulations were made for five crops (i.e. silage maize, sugar beet, winter barley, winter rapeseed, and winter wheat) grown in 2016 on an area of approximately 1 km × 1 km, where water scarcity is known to have an effect on crop development (Rudolph et al., 2015). In a first step, the information provided by the three soil maps was compared. Next, AgroC simulations of leaf area index (LAI) based on the three soil maps were evaluated using LAI observations derived from multispectral satellite data. Finally, the added value of the geophysics-based soil map for the simulation of crop growth was investigated and discussed with a focus on the results for sugar beet.
2.1 Study area
The study site is located within a ∼ 1 km × 1 km area (Fig. 1a) in the Rur catchment near Selhausen (Germany; 50∘51′56′′ N 6∘27′03′′ E). It is composed of several agricultural fields cultivated in rotation by more than 20 different land owners, and thus the agricultural management is heterogeneous. The mean annual temperature and precipitation are 10.2 ∘C and 715 mm, respectively (Rudolph et al., 2015). The area is part of the Terrestrial Environmental Observatories (TERENO) network (Bogena et al., 2018; Schmidt et al., 2012; Simmer et al., 2015). Within this network, continuous measurements of meteorological parameters are performed in the centre of field F11 (Fig. 1a).
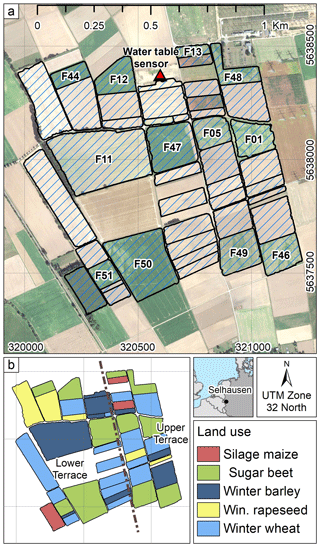
Figure 1(a) Satellite image of the study area with the fields used for comparison and codes of fields where sugar beet was grown in 2016 and (b) crops that were grown in each studied field in 2016 (ESRI, 2016).
In 2016, the 44 investigated fields were cropped with silage maize, sugar beet, winter barley, winter rapeseed, and winter wheat. The distribution of these crops in the study area is shown in Fig. 1b. The total area cultivated with each crop, as well as their approximate emergence and harvest date, is listed in Table 1. A more detailed investigation was performed in fields where sugar beet was grown (fields F01, F05, F13a, F46, F48, F49, F12, F47, F50, and F51 in Fig. 1a). For field F01, the sugar beet yield for 2016 was 61.4 t ha−1 of fresh beet, which is equal to 14.2 t ha−1 of dry beet assuming an average water content of 76.8 % (FAO, 1999).
Table 1Range of emergence and harvest dates and total area for the five crops cultivated in the study area in 2016.
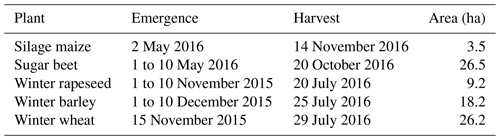
Previous studies at this site showed that soil heterogeneity has a strong influence on crop performance during long periods of water scarcity (Rudolph et al., 2015; von Hebel et al., 2018). This is most apparent on the upper terrace, which has an altitude of ∼ 110–113 m a.s.l. and is separated from the lower terrace (∼ 101–103 m a.s.l.) by a gentle slope with western exposition (Fig. 1). For both terraces, aeolian Pleistocene sediments and translocated Holocene loess (Röhrig, 1996) are generally dominant in the topsoil. Within the top 2 m of soil, sand and gravels from the Pleistocene and Holocene are consistently found below the aeolian sediments of the upper terrace and locally below the topsoils of the lower terrace (Brogi et al., 2019; Klostermann, 1992; Pätzold et al., 2008; Röhrig, 1996). The depth to these sand and gravels is known to be a strong control on crop water availability (Brogi et al., 2020; Rudolph et al., 2015; von Hebel et al., 2018).
Information on the spatial and temporal development of the five crops in 2016 was derived from multispectral satellite images. In particular, six LAI maps estimated from Level 3A images recorded by the RapidEye satellite constellation were available. These images covered the growing season of the five investigated crops and were acquired on 14 March, 20 April, 28 May, 9 June, 12 August, and 8 September 2016. In order to generate the LAI maps, the normalized difference vegetation index (NDVI) of the satellite images was first calculated using the red (RED; 630–685 nm) and the near-infrared (NIR; 760–850 nm) bands. In a second step, NDVI values were used to calculate the fractional vegetation cover (FVCNDVI) for each image using the NDVI of bare soil (NDVIS) and the fully vegetated state (NDVIV) (Beck et al., 2006; Xiao and Moody, 2005; Zeng et al., 2003). Finally, the LAINDVI was calculated from the FVCNDVI. In this final stage, the light extinction coefficient k(θ) of each crop type, which is a measure of attenuation of radiation in the canopy (Campbell, 1986; Norman and Campbell, 1989; Ross, 2012; Propastin and Erasmi, 2010), was calibrated using 45 in situ destructive LAI measurements that were acquired between 22 March and 7 September 2016. In the case of winter rapeseed and silage maize, no in situ LAI measurements were available, and k(θ) was obtained from literature values (Ali et al., 2015). A detailed description of the procedure used for this study area can be found in Brogi et al. (2020), whereas the general approach is described in more detail in Ali et al. (2015).
2.2 Available soil maps
Three different soil maps of the same area were used to inform agro-ecosystem simulations. Simulations were first performed using information from a geophysics-based soil map (Brogi et al., 2019) employing a methodology that was already successfully applied in this area by Brogi et al. (2020). In a following step, simulations were performed using information derived from two commonly available soil maps: (i) a regional soil map (Röhrig, 1996) with a scale of 1 : 5000 and (ii) a national soil and yield potential map (NRW, 1960) used in agricultural taxation.
The geophysics-based soil map (Fig. 2a) is a high-resolution product that was obtained by combining multi-configuration EMI measurements and direct soil sampling with subsequent laboratory analysis (Brogi et al., 2019). EMI measurements were performed using a CMD Mini Explorer – Special Edition (GF Instruments, Brno, Czech Republic) and resulted in six apparent electrical conductivity (ECa) maps, each with a different depth of investigation. These maps were analysed using a supervised classification methodology. This resulted in a map that showed the spatial distribution of 18 different soil types within the study area. Quantitative soil information was obtained using 100 augering locations (∼ one location per hectare) where horizon type and thickness were recorded. At these locations, soil samples were also collected to determine the grain size distribution of each soil horizon. Finally, information obtained through direct soil sampling was combined with the spatial distribution of the ECa-based soil units, which resulted in a soil map with 1 m resolution and quantitative soil information up to 2 m depth. The geophysics-based soil map consists of four sub-areas (A, B, C, and D) and a total of 18 soil units with an area between 0.6 and 16.0 ha. As shown in Fig. 2a for the soil units A1a and C1a, each soil unit of the geophysics-based soil map is provided with a soil profile that shows the depth of each horizon as well as the textural information (percentage of clay, silt, and sand plus gravels). A detailed description of the acquisition, management, and analysis of the geophysical data as well as the steps used to create this geophysics-based soil map can be found in Brogi et al. (2019). This geophysics-based product was recently used in several studies focused on the Selhausen study area (Brogi et al., 2020; Jakobi et al., 2020; Reichenau et al., 2020).
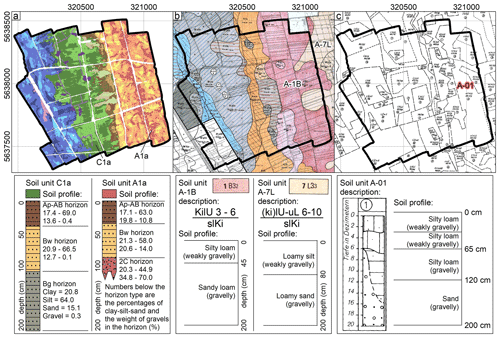
Figure 2(a) Geophysics-based soil map of the study area with examples of the quantitative description of the soil characteristics (soil units A1a and C1a are shown); (b) 1 : 5000 soil map of the study area with examples of the qualitative description of the soil characteristics (soil units A-1B and A-7L are shown) (Röhrig, 1996); and (c) soil taxation map of the study area and examples of the qualitative description of the soil characteristics (unit A-01 is shown) (NRW, 1960). The spatial distribution of all soil units within the study area and their codes are shown in Fig. 3.
The 1 : 5000 regional soil map is shown in Fig. 2b. This thematic map was first produced in 1984/85 and then revised in 1996 (Röhrig, 1996). It is commonly used in regional-scale projects (e.g. sustainable soil protection plans) and is part of the NRW official soil inventory (Geologischer Dienst NRW, 2018). The topographic base is the 1 : 5000 German base map (Deutsche Grundkarte), and soil information is obtained through direct soil sampling (augering). The distance between auger locations is typically <100 m (∼ one location per hectare). For each soil unit, information on soil type, soil texture (qualitative description), and thickness of the topsoil horizons is provided. Generally, the depth of the interface between two soil horizons is represented by a range (e.g. in soil unit A-1B of Fig. 2b, this interface is found between 0.3 and 0.6 m depth). This information was generalized by averaging the reported maximum and minimum values. According to this map, 13 soil units are found in the study area, with an area between 1.0 and 25.3 ha.
The soil taxation map is shown in Fig. 2c. It is used for the calculation of tax rates for farmers and land owners based on estimates of the yield potential (NRW, 1960). The scale of this map can vary across different regions in Germany and is 1 : 5000 for the study area. The map is based on the German cadastre, and soil information was obtained through direct soil sampling with a separation of 40 to 50 m between augering locations (∼ four locations per hectare). Each soil unit is described by a soil profile with 2.0 m depth, which is divided into up to four horizons. Each horizon carries qualitative information on soil texture. In some cases, the interface between two horizons is represented by a range, which again was generalized by averaging the reported maximum and minimum values (e.g. soil unit A-01 in Fig. 2c). In total, 10 different soil units were identified in the study area, and their area ranges between 0.7 and 17.5 ha.
The area covered by the five investigated crops in 2016 (Fig. 1b) and the geometry of the three soil maps (Fig. 3) were intersected. As a result, a set of unique soil–crop combinations was obtained for each soil map: (i) 72 unique soil–crop combinations for the geophysics-based soil map, (ii) 42 for the 1 : 5000 soil map, and (iii) 35 for the soil taxation map.
2.3 Similarities between sub-areas of the three soil maps
According to the geophysics-based soil map, the study area is divided into four sub-areas (A, B, C, and D) as described in Brogi et al. (2019). Sub-area A is located on the upper terrace, sub-area B is located on the slope between the two terraces, and sub-areas C and D are located on the lower terrace. Crop growth simulations for sub-areas B and C by Brogi et al. (2020) showed similar results. Thus, they were described together as a single sub-area BC in this study (Fig. 3). Generally, the geometry of the soil units described in the commonly available soil maps falls within one of the four sub-areas of the geophysics-based product. Thus, the soil units of the commonly available maps were matched with one of the sub-areas A, BC, and D. As a result, a clearer comparison between the simulations and the results obtained with different maps was possible. The distribution of the soil units described in the three soil maps is shown in Fig. 3, and the final unified code of each soil unit is provided in Table 2.
2.4 The AgroC model
The AgroC model (Herbst et al., 2008) was used to simulate crop growth within each of the soil–crop combinations. In particular, we were interested in investigating how differences in soil water content dynamics and water availability as a consequence of differences in soil layering and soil hydraulic parameters affected crop growth. The agro-ecosystem model AgroC couples three main modules: SOILCO2, SUCROS, and RothC. SOILCO2 simulates vertical fluxes of water, heat, and CO2 for a 1D soil column (Šimůnek et al., 1996; Šimůnek and Suarez, 1993); SUCROS simulates crop growth (Spitters et al., 1989); and RothC simulates organic carbon turnover (Coleman and Jenkinson, 2008).
In the SOILCO2 module, water flow in a given soil profile is described by the 1D Richards equation:
where θ (cm3 cm−3) is the volumetric water content, t is time, z is the vertical coordinate (cm), K(h) (cm h−1) is the hydraulic conductivity as a function of pressure head h (cm), and Q (cm3 cm−3 h−1) is the source–sink term accounting for root water uptake by crops. The hydraulic conductivity K and the volumetric water content θ as a function of pressure head are described by the Mualem–van Genuchten model:
and
where Ks is the saturated hydraulic conductivity (cm h−1), m is a parameter that is set equal to , θs and θr (cm3 cm−3) are the saturated and residual water content, respectively, α is a parameter corresponding approximately to the inverse of the air entry pressure (cm−1), n (dimensionless) is a parameter related to the pore size distribution (Van Genuchten, 1980), and Se is the dimensionless relative saturation defined as
The water demand is calculated in the SUCROS module. For a crop growing under optimal conditions, the potential evapotranspiration is divided between potential transpiration Tp (cm h−1) and potential soil evaporation Ep (cm h−1). Then, the potential root water uptake Sp (cm3 cm−3 h−1) is calculated using
where β is the depth-dependent root distribution function. Afterwards, the depth-specific actual root water uptake Q is obtained from the potential root water uptake Sp using
where the water uptake is scaled by a water uptake stress factor φ that is dependent on the pressure head. The φ(h) factor is calculated using the Feddes et al. (1978) approach:
where h0–3 (cm) are threshold pressure heads obtained from literature. These thresholds were set to h0=0 cm, cm, cm, and h3 = −16 000 cm (Vanclooster et al., 1995). The actual root water uptake is integrated over the rooting depth to obtain the actual transpiration. Afterwards, the ratio between actual and potential transpiration is used to simulate carbon assimilation and biomass production, which are also affected by additional variables such as temperature and solar radiation. A detailed description of this model and of the effects of simulated water stress on simulated crop growth can be found in Klosterhalfen et al. (2017) and in Brogi et al. (2020) for this study area.
2.5 Estimation of soil hydraulic parameters
Soil hydraulic parameters for each horizon of the soil units of all three soil maps were estimated from texture and bulk density using pedotransfer functions (PTFs) and a correction for gravel content as successfully done by Brogi et al. (2020) for the same area. Information on bulk density was not available. Therefore, the bulk density of the fine fraction <2 mm, BD<2, of each horizon was assumed to be 1.30 g cm−3 for the Ap horizon, 1.40 g cm−3 for the AB horizon, 1.50 g cm−3 for deeper horizons with fine sediments, and 1.60 g cm−3 for deeper horizons with coarse sediments. These estimates were based on literature values and on results from previous sampling campaigns conducted within the study area (Brogi et al., 2020; Ehlers et al., 1983; Unger and Jones, 1998). Unfortunately, the soil profiles from the 1 : 5000 soil map and from the soil taxation map are not provided with the depth of the interface between the Ap and AB. Therefore, in each soil profile, the first horizon was subdivided at a depth of 0.3 m as this was generally observed to be the depth of the Ap horizon in this study area.
The geophysics-based soil map provides quantitative information on soil texture for each soil unit. In this case, the percentages of clay, silt, and sand were directly used in the estimation of the soil hydraulic parameters. In contrast, the two commonly available soil maps provide a qualitative description of soil texture (i.e. a soil textural class). Generally, lookup tables are used in the case of such qualitative descriptions (Van Looy et al., 2017) in order to obtain class-average soil hydraulic parameters (Baker, 1978; Bouma, 1989). To obtain a consistent comparison between the three maps, these qualitative soil textural class descriptions were translated into quantitative percentages of sand, silt, and clay using the USDA soil textural classification (USDA, 2019). For this, the centroid of each soil textural class within the USDA triangle was calculated to determine the associated soil texture percentages (Table 3). In some cases, the presence of gravel was qualitatively described in the two commonly available soil maps. In these cases, a 25 % volume of gravel was assumed when the gravel content was defined as “gravelly” and 10 % was assumed when the gravel content was defined as “weakly gravelly” as these percentages matched those observed in the study area.
Table 3Percentage (%) of sand, silt, and clay of the centroid of relevant soil textural classes within the USDA soil texture triangle.
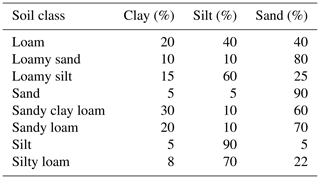
The dry bulk density was estimated for each soil horizon using the equation of Brakensiek and Rawls (1994):
where BDt is the dry bulk density of the soil, BD>2 is the dry bulk density of gravel material, and Gv is the volume of gravel calculated from the percentage of weight according to Flint and Childs (1984). Finally, the soil hydraulic parameters θs, θr, α, n, and Ks were estimated from the dry bulk density and from clay, silt, and sand percentages by using the PTFs of Rawls and Brakensiek (1985). In all the soil maps, the estimated Ks values of the coarse horizons in sub-areas A and D were corrected for gravel content using the correction from Brakensiek and Rawls (1994):
where Kb is the saturated hydraulic conductivity of the bulk soil, and Ks is obtained from the PTF of Rawls and Brakensiek (1985).
As shown in Brogi et al. (2020), the coarse horizon 2C (sand and gravel) that underlies the fine aeolian sediments in sub-area A and in parts of sub-area D is of primary importance for a sound simulation of crop performance within the investigated area. However, the properties of these deeper soil horizons were not adequately captured in the commonly available soil maps, and this resulted in simulated water contents that were unrealistically low. To avoid the introduction of such strong and unrealistic variations in the results of the agro-ecosystem simulations performed with the three soil maps, the soil hydraulic parameters obtained for the 2C horizon in sub-areas A and D of the geophysics-based soil map were integrated into the two other commonly available soil maps.
2.6 Setup and evaluation of AgroC simulations
The 1D soil column used in the AgroC simulations was discretized by using a maximum of 252 nodes with a spacing of 1 mm near the soil surface and a gradual increase with depth until a maximum of 10 mm. The simulation period extended from 1 July 2015 to 31 December 2016. The simulation domain of the soil units in sub-area BC extended to 2.0 m below the soil surface for all three soil maps. In these units, a variable pressure head with an annual sinusoidal variation based on water table depth observations in field F10 (Fig. 1) was used as the lower boundary condition. The groundwater table depth was set to a minimum of −2.0 m on 15 January and a maximum of −2.6 m on 15 July. The initial pressure head within the soil profile was defined through a spin-up simulation. To achieve this, the period from 1 January 2015 to 31 December 2016 was repeatedly run until no change in pressure head within the soil column was observed between consecutive model runs.
The soil units of sub-areas A and D have a coarse sand and gravel horizon at depth. In these soil units, the simulation domain extended 30 mm into the coarse horizon. As a result, the simulation domain varied in depth between 0.52 and 1.57 m for the geophysics-based soil map and from 0.33 to 1.43 m in the two commonly available soil maps. Free drainage was used as the lower boundary condition for these soil units. The initial pressure head of the lower coarse horizons was set to −10 mm, and hydrostatic equilibrium was assumed throughout the profile (Brogi et al., 2020).
The required crop-specific parameters were mostly obtained from various literature sources (Allen et al., 1998; Bolinder et al., 1997; Boons-Prins et al., 1993; Borg and Grimes, 1986; Penning de Vries et al., 1989; Spitters et al., 1989; Van Heemst, 1988; Vanclooster et al., 1995). Only the sub-area specific parameterization of the death rate of leaves for sugar beet, the start of the senescence stage for silage maize, and the partitioning of aboveground biomass for winter wheat were adapted as described in detail in Brogi et al. (2020). The crop-specific maximum rooting depth was set to 1.50 m for sugar beet and silage maize, 1.40 m for winter rapeseed, 1.20 m for winter barley, and 1.00 m for winter wheat. The method of Rum et al. (1974) was used to calculate the root distribution above these depths. In the case of the soil units of sub-areas A and D, rooting depth was reduced using the depth of the lower coarse horizon as it was assumed that roots cannot penetrate into such coarse soils (Daddow and Warrington, 1983).
Three criteria were used to quantify the agreement between the observed LAINDVI and LAI simulated with AgroC by using inputs from the three soil maps. The first was the root mean square error (RMSE):
where n represents the number of observations, Obsi is the observed value, and Simi is the simulated value. A lower RMSE indicates a better fit. The second criterion was the model efficiency (ME), which is calculated as
where is the observed mean. The ME can vary between −∞ and 1, and a value higher than 0 indicates that the model describes the data better than the mean. A ME value of 1 indicates perfect agreement between observations and predictions (Nash and Sutcliffe, 1970). The third and final criterion was the coefficient of determination R2:
where is the simulated mean. The value of R2 ranges between 0 and 1, and a value of 1 indicates perfect agreement.
3.1 Comparison of the spatial distribution of soil properties in the three maps
By visually comparing the three soil maps, it is apparent that the geomorphological border between the upper and lower terrace is similarly identified (division between sub-area A and BC in Fig. 3). In the geophysics-based soil map, this subdivision is based on the measured apparent electrical conductivity (ECa) data (Brogi et al., 2019), whereas the delineation in the commonly available soil maps was mainly based on coarse soil augering and topography since this limit approximately coincides with the top of the slope that divides these two sub-areas. The location of the subdivision between sub-areas BC and D showed stronger differences between the three maps. Again, this border is obtained from ECa data in the geophysics-based soil map. In this case, there is no topographic feature associated with this subdivision, and, in the other two maps, only the information from augering was available to determine its position. This likely explains why the subdivision between the two sub-areas locally coincides with field boundaries in the 1 : 5000 soil map and in the soil taxation map.
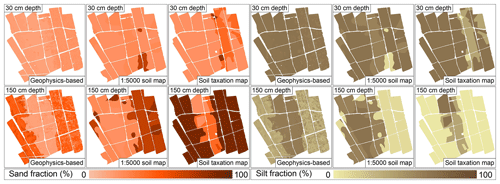
Figure 4Percentages of sand (orange colour scale) and silt (brown colour scale) at 0.30 m depth and at 1.50 m depth in the three soil maps.
The geophysics-based soil map generally identifies a larger number of soil units with more complex polygon shapes compared to the commonly available soil maps. This is a consequence of the high resolution of the measured ECa data. In this study area, a single agricultural field contained four to nine soil units according to the geophysics-based soil map. The two commonly available soil maps often integrate larger areas into one soil unit, and a single field is composed of a maximum of four soil units. However, many fields contain only one soil unit.
In the 1 : 5000 soil map and in the soil taxation map, the shallow soils found within the study area are generally silty loam and loamy silt above coarse sediments. As shown in Fig. 4, the grain size distribution obtained from the qualitative descriptions using Table 3 showed minor differences compared to the one provided by the soil units of the geophysics-based soil. For example, the estimated grain size distribution for loamy silt soil units of the commonly available maps was 25 % sand, 60 % silt, and 15 % clay, whereas the respective range of values found in the geophysics-based soil map was 13 %–24 % sand, ∼ 56 %–70 % silt, and ∼ 13 %–23 % clay. The estimated values for silty loam (22 % sand, 70 % silt, and 8 % clay) differed more from those of the geophysics-based soil map but still were in reasonable agreement. The underlying coarse materials found in sub-areas A and D were identified as loamy sand, sand, sandy clay loam, or sandy loam in the commonly available soil maps. In the case of these four soil textural classes, the sand fraction reported in Table 3 (60 %–90 %) was much higher than the values reported in the geophysics-based soil map (∼ 28 %–58 %). At the same time, the percentage of silt in the commonly available maps (5 %–10 %) was much lower compared to those of the geophysics-based map (∼ 30 %–54 %). Figure 4 illustrates these textural differences in sand and silt percentages between the three soil maps at a depth of 1.50 m.
The description of the topsoil (at 0.3 m depth) in the three soil maps is rather similar. In contrast, the geophysics-based soil map provides a different description of the underlying coarse horizons compared to the commonly available soil maps. It is important to note that the textural compositions found in the geophysics-based soil map were determined from laboratory analysis, whereas those provided by the other two maps were generated from field estimations (hand texturing). Due to these differences, soil hydraulic parameters of the coarse sediments vary strongly between the three maps, with the commonly available maps showing much higher values of Ks and θs. As already mentioned in the Materials and methods section, this resulted in unrealistic simulations with very low soil water content for the commonly available maps (results not shown). For this reason, the soil hydraulic parameters of the coarse horizons of sub-areas A and D of the geophysics-based soil map were integrated into the simulations based on the two commonly available soil maps.
Differences in the depth to the coarse sand and gravel that underlie the fine sediments in sub-areas A and D were observed between the three soil maps (Fig. 5). In all three maps, this depth was obtained from augering information and varied between 0.47 and 1.34 m in the geophysics-based soil map, between 0.30 and 1.50 m in the 1 : 5000 soil map, and between 0.40 and 1.40 m in the soil taxation map. Both the two commonly available soil maps showed a larger depth range than that of the geophysics-based soil map, with the 1 : 5000 soil map showing the largest depth range.
3.2 Performance of LAI simulations
Figure 6 shows a comparison between the mean observed LAINDVI for each soil–crop combination and the LAI simulated with AgroC by using inputs from the three soil maps. The LAI simulations based on the geophysics-based soil map (R2=0.925, ME = 0.919, and RMSE = 0.604) were more able to describe LAINDVI compared to the simulations based on the 1 : 5000 soil map (R2=0.887, ME = 0.869, and RMSE = 0.718) and the soil taxation map (R2=0.886, ME = 0.866, and RMSE = 0.719). However, the results for all three soil maps could be considered satisfactory as the improvement provided by the more detailed geophysics-based soil map is relatively small. This similarity in model quality can be explained by the simultaneous use of all simulated crops (silage maize, sugar beet, winter barley, winter rapeseed, and winter wheat) and all six RapidEye satellite images. Furthermore, this comparison was performed by using the mean value for each soil–crop combination, and this reduced the influence of small-scale variability of LAINDVI. These aspects will be investigated in more detail in the next section.
3.3 Pixel-by-pixel comparison of LAI simulations
Figure 7 shows a pixel-by-pixel comparison of observed LAINDVI and LAI simulated using inputs from the three soil maps. It is apparent how the highest pixel density (red) is close to the 1 : 1 line for the geophysics-based soil map, and more spread is observed for the two commonly available soil maps. Moreover, the error measures for the geophysics-based soil map (R2=0.884, ME = 0.878, and RMSE = 0.689) show slightly better results than those of the 1 : 5000 soil map (R2=0.858, ME = 0.847, and RMSE = 0.774) and considerably better results than those of the soil taxation map (R2=0.741, ME = 0.675, and RMSE = 1.126). The values of LAINDVI occasionally showed a rather strong variability within single soil–crop combinations. As a consequence, lower values of R2 and ME and higher values of RMSE are found in the pixel-by-pixel analysis compared to the previously discussed comparison that made use of the mean LAINDVI for each soil–crop combination.
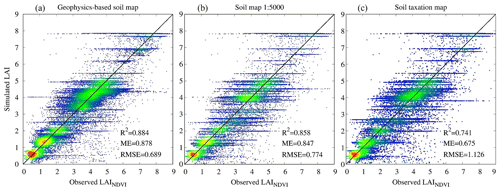
Figure 7Pixel-by-pixel comparison between simulated LAI and observed LAINDVI for simulations based on (a) the geophysics-based soil map, (b) the 1 : 5000 soil map, and (c) the soil taxation map. The colour indicates the density of events, with red representing the highest density and blue the lowest density.
Table 4R2, ME, and RMSE of the pixel-by-pixel comparison of the three soil maps performed between simulated LAI and observed LAINDVI of each soil–crop unit. The highest R2 and ME and the lowest RMSE at each date are marked in bold.
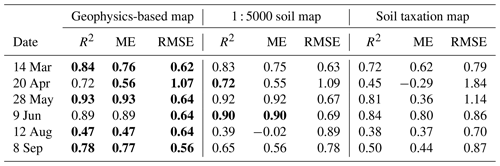
Table 4 shows the pixel-by-pixel performance of the three soil maps for each RapidEye satellite image. The geophysics-based soil map generally showed higher ME and higher R2 and lower RMSE. However, it is apparent that the three soil maps performed similarly in March, May, and June, when winter crops were grown (i.e. winter barley, winter rapeseed, and winter wheat), with the 1 : 5000 soil map showing marginally higher ME and R2 for individual dates. Given the sufficient amount of rain during the growth of these crops, simulations showed little crop water stress. This resulted in simulations with rather similar LAI despite using information from three different soil maps. In the case of the image from April, the geophysics-based soil map and the 1 : 5000 soil map outperformed the soil taxation map. However, a general decrease in performance can be observed for all three maps. This general decrease is likely caused by the high variability in LAINDVI between different agricultural fields, which is caused by the uncertainty in seeding and emergence dates of winter crops. Moreover, the flowering of winter rapeseed (yellow dots in Fig. 6) affected the estimated LAINDVI (Brogi et al., 2020). This may have additionally reduced the performance for all three soil maps in April. A strong drop in performance for all three soil maps was observed in August and September. At this stage, the comparison is based solely on silage maize and sugar beet as the other crop types were already harvested. Thus, the reduced correlation is likely related to the appearance of stress in these two crops that increased the spatial variability in LAINDVI. In this situation of high water stress, the geophysics-based soil map clearly outperformed the 1 : 5000 soil map and the soil taxation map, especially towards the end of the growing season.
3.4 Simulation of sugar beet
In a next step, simulations of sugar beet are analysed in more detail because of the importance of this crop (sugar beet represents 31.7 % of the investigated area) and because the previously described analysis showed that the difference in performance between the three soil maps was the greatest when this crop was still growing (August and September). Figure 8a–c show the simulated LAI (lines) and the observed LAINDVI (dots) for sugar beet grown on the soil units of sub-area A for the three soil maps. Figure 8d–f show the associated water stress simulated with AgroC. All these simulations made use of the same crop parameterization. Thus, the differences in simulated LAI are due to different degrees of water stress associated with the soil characteristics. As shown in Fig. 8a, the simulated LAI based on the geophysics-based soil map matched well with the observations. In fact, soil units A1a-d showed very similar LAI values for 28 May and 9 June. Later in the growing season (12 August and 8 September), simulated LAI of soil units A1a-d differed due to a different magnitude of water stress. The magnitude of water stress and the consequent reduction of LAI are well correlated with the depth of the coarse sediments, which was 0.86 m in soil unit A1a, 0.67 m in A1b, 0.58 m in A1c, and 0.49 m in A1d.
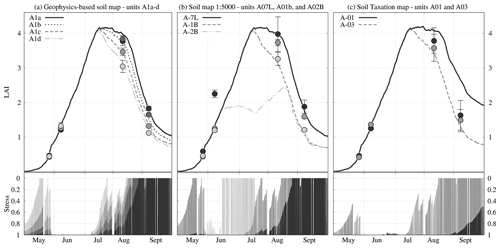
Figure 8Observed LAINDVI (dots) of sugar beet compared to the simulated LAI (lines) in sub-area A (upper plots) as well as corresponding stress occurrence (lower plots) simulated using input from (a) the geophysics-based soil map, (b) the 1 : 5000 soil map, and (c) the soil taxation map.
In the case of the three soil units of the 1 : 5000 soil map, the simulated LAI matched well the observations in May and June, with the only exception of soil unit A-07L, where observed LAINDVI is underestimated. Later in the growing season (August and September), soil unit A-7L again matched the observations. This was not the case for soil units A-1B and A-2B, where LAINDVI was underestimated. The mismatch between LAI simulations and observations for these two soil units is due to the assumed shallow depth to the coarse sediments (0.30 m in soil unit A-2B, 0.45 m in unit A-1B, and 0.80 m in A-7L). As previously discussed, this depth has a strong influence on the intensity and timing of water stress. Generally, the topsoil and the associated physical properties described in the 1 : 5000 soil map were rather similar to those of the geophysics-based soil map. As a consequence, the reduced match between simulated LAI and observed LAINDVI was caused by an underestimation of the depth to the coarse sediments in soil units A-1B and A-2B, which resulted in a higher water stress and lower LAI.
In the case of the soil taxation map, the observed LAINDVI for the two soil units A-01 and A-03 were rather similar and showed a strong variability within each unit (see dots and error bars in Fig. 8c). This suggests that the geometry of the two soil units did not capture the spatial differences in crop performance present in the LAINDVI. The soil physical properties of the uppermost horizon in the soil taxation map were rather different compared to those of the geophysics-based soil map. In contrast, the soil physical properties of the coarse sediments were equal to those of the geophysics-based soil map by design. However, the depth to these coarse sediments in the two soil units was generally deeper (1.20 m in A-01 and 0.70 m in A-03) than in the geophysics-based soil map. Due to these different soil physical properties and the overestimation of soil depth overestimation, lower water stress in soil unit A-01 caused a higher simulated LAI compared to the observations. This depth was better captured in soil unit A-03 that showed better LAI simulations.
Figure 9 shows the spatial distribution of LAINDVI for 12 August and 8 September. On both days, the large-scale pattern in LAINDVI associated with the differences between sub-areas A, BC, and D was clearly visible. In August (Fig. 9a), LAINDVI reached the maximum observed value in sub-area BC, whereas lower values were observed in sub-area A due to water stress. In September (Fig. 9b), the development of sugar beet was affected by water stress in both sub-area A and D, as indicated by the lower LAINDVI compared to sub-area BC.
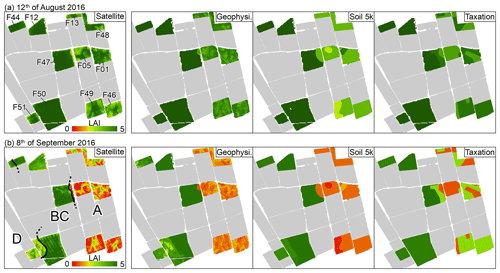
Figure 9Observed LAINDVI and simulated LAI obtained using input from the geophysics-based soil map (Geophysi.), the 1 : 5000 soil map (Soil 5k), and the soil taxation map (Taxation); row (a) shows the codes of the investigated fields and the comparison on 12 August 2016, and row (b) shows the geometry of sub-areas A, BC, and D and the comparison on 8 September 2016.
The simulated LAI obtained using the three soil maps captured the large-scale pattern in LAINDVI associated with the sub-areas A, BC, and D to some extent. The differences in crop performance between sub-area A and BC were sufficiently well represented in all three simulations. However, the border between low LAI in sub-area A and high LAI in sub-area BC is better represented in the geophysics-based approach (see field F47 in Fig. 9). The differences between the high LAI of sub-area BC and the intermediate LAI of sub-area D were not well represented in the two commonly available soil maps. In fact, the simulated LAI is very similar in the two sub-areas when using the 1 : 5000 soil map and the soil taxation map. This is related to the generally low water stress simulated in sub-area D that did not result in a meaningful reduction of LAI. In contrast, a substantial reduction of LAI was obtained within the simulations based on the geophysics-based soil map. The small-scale patterns in LAINDVI observed within each sub-area were also partly captured by the geophysics-based soil map for both dates. In contrast, simulations based on the other two soil maps did not provide results that represented these patterns. At the same time, it is clear that the small-scale variability in LAINDVI is not fully captured in our simulation approach because variations within individual soil–crop combinations were not considered.
Table 5RMSE between simulated LAI and LAINDVI in fields with sugar beet. The best result for each date is marked in bold.
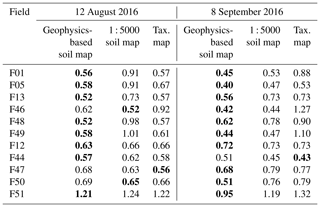
Table 5 provides the pixel-wise RMSE between simulated LAI and LAINDVI for all 11 fields where sugar beet was grown. Generally, these fields can be divided into three groups based on the RMSE of each soil map. The first group consists of the fields F01, F05, F13, F46, F48, and F49. In these fields located in sub-area A, the geophysics-based soil map provided improved simulation results for both dates compared to the simulations based on the two other soil maps as indicated by the lower RMSE. Field F46 was the only exception as the lowest values in August (RMSE = 0.52) were obtained with inputs from the 1 : 5000 soil map. In some cases, the difference in RMSE between the simulations based on the three soil maps was rather small, such as in field F48 and field F49. Nevertheless, the RMSE obtained in this group of fields using inputs from the geophysics-based soil map was lower for both days and was larger in September than in August. The second group consists of fields F12, F44, and F47 in sub-area BC. In these fields, no water stress was simulated for any of the soil maps. Therefore, all three soil maps provided accurate LAI simulations, as indicated by the low RMSE. The third group consist of fields F50 and F51 that are located in sub-area D. In August, the RMSE of the simulations performed using the three soil maps was rather similar. In contrast, the simulations based on the geophysics-based soil map clearly outperformed those based on the commonly available soil maps in September. This again corresponds with the simulated water stress in sub-area D, which was high in September but rather low in August.
Overall, the results clearly highlight that the improvements provided by the use of the geophysics-based soil map for the simulation of sugar beet are clearly dependent on the magnitude and timing of water stress. Simulations based on the three soil maps showed rather similar results in periods with sufficient water availability and in areas where water stress did not affect crop development. In contrast, in the presence of significant water stress, the use of the geophysics-based soil map reduced the RMSE between LAI simulations and observations compared with the use of the 1 : 5000 soil map or the soil taxation map. This reduction in RMSE varied substantially from field to field, with a minimum of 2 % and a maximum of 67 %. Likely, this variability is due to local differences in the quality of the soil characterization provided by the three soil maps. The area-averaged RMSE reduction in periods of significant water stress was 25 % compared to the 1 : 5000 soil map and 31 % compared to the soil taxation map.
In field F01 of sub-area A, the reduction of the RMSE between LAI simulations and observations provided by the use of the geophysics-based soil map varied between 15 % and 49 % on 8 September. In this field, the yield recorded by the farmer was 14.2 t ha−1 of dry beet biomass. The use of inputs from the geophysics-based soil map resulted in a simulated yield of 14.3 t ha−1, which was only 1 % higher than the actual yield. The yield simulated with inputs from the commonly available map showed an overestimated yield, with 15.4 ha−1 for the 1 : 5000 soil map (+8 %) and 17.3 ha−1 for the soil taxation map (+22 %). Similarly as in the case of LAI, the geophysics-based soil map outperformed the other two maps. However, these results should be interpreted carefully as the required information was only available for a rather small field within the study area. Nonetheless, it is anticipated that the geophysics-based soil map provides similar improvements in yield estimates as for the LAI simulations for other fields. This expectation is based on the tight relationship between LAI and other aspects of crop development, such as biomass production and ultimately yield. For this same reason, the assimilation of LAI data into crop growth model ensembles has been shown to improve yield estimates (Jonckheere et al., 2004; Tewes et al., 2020; Wilhelm et al., 2000).
When comparing the results provided by the use of the three maps, it has to be noted that the geophysics-based soil map was produced with a set of 100 sampling locations (one per hectare), which is similar to the sampling density of the 1 : 5000 soil map (∼ one per hectare) and lower than the one of the soil taxation map (∼ four per hectare). It can be argued that the geophysics-based soil map had an advantage resulting from the quantitative textural information based on laboratory analysis. To a certain extent, this holds true. However, it should be seen against the background that textural information on the coarse soils from the geophysics-based product was integrated into the simulations with the commonly available soil maps. Furthermore, the characterization of the upper soils only showed local differences between the three maps.
The reduction in performance of the 1 : 5000 soil map and of the soil taxation map compared to the geophysics-based soil map was strongly dependent on local soil characteristics. In general, it was found that this reduction in performance was caused by a poor representation of (i) the spatial geometry of individual soil units, (ii) the depth to the coarse sediments, (iii) the textural characteristics of the overlying sediments, and (iv) the subdivision between large sub-areas. The different quality of this soil representation affected the quality of the LAI simulations throughout the year and especially towards the end of the growing season of the summer crops.
In this study, agro-ecosystem simulations were performed on a 1 km × 1 km agricultural area by using information from three different soil maps: (i) a high-resolution geophysics-based soil map, (ii) a 1 : 5000 regional soil map, and (iii) a soil taxation map. The growth in 2016 of silage maize, sugar beet, winter barley, winter rapeseed, and winter wheat was simulated using the agro-ecosystem model AgroC. The LAI simulated with this model was compared with LAI observations determined from RapidEye satellite imagery. The simulations based on the geophysics-based soil map outperformed simulations based on the commonly available maps by consistently showing higher explained variance (R2) and model efficiency (ME) as well as lower root mean square error (RMSE). However, simulations for winter crops and in periods with limited water stress showed subtle improvements only. In contrast, the geophysics-based soil map clearly outperformed the commonly available soil maps in periods with moderate to high water stress that caused a reduction in crop performance, particularly for silage maize and sugar beet.
AgroC simulations for sugar beet were analysed in more detail. It was found that the commonly available soil representations were strongly outperformed by the geophysics-based product in areas with coarser soils and in periods with high water stress. This was related to a more accurate description in the geophysics-based soil map of (i) the depth to the coarse sediments; (ii) the soil texture of the overlying horizons; (iii) the subdivision of the four geomorphological sub-areas A, BC, and D; and (iv) the distribution of the soil units that are found in the study area. This study showed that a geophysics-based soil characterization combined with direct soil sampling provides an added value to agro-ecosystem modelling and allows for the improved simulation of crop LAI and crop performance. Thus, such geophysics-based soil maps may have a positive and quantifiable utility in agriculture and, depending on local soil characteristics, can support the use of advanced precision farming techniques and strategies that are not practicable when solely based on general-purpose soil maps.
Nevertheless, the strong dependence of the added value of the geophysics-based soil map on the crop type, soil characteristics, and precipitations calls for further research on such topics. For example, it would be important to estimate the added value of a geophysics-based soil map before this soil map is realized, so that farmers could make an informed decision on the profitability of such a mapping product. This could be achieved by investigating the specific pedoclimatic conditions of a given farm or by mapping small portions of the target area to provide an estimate of the costs and benefits of the final soil map. Similarly, by performing long-term simulations, farmers could be informed on the time span required to achieve returns on their investment. Such simulations should include agricultural practices that are key to certain areas such as irrigation, crop rotation, and different fertilization practices. In parallel, the use of different geophysical techniques (taken singularly or in combination) and their added value should be further investigated. In the case of electromagnetic induction (EMI), focus should be put on the different added value for agro-ecosystem models originating from rather simple apparent electrical conductivity (ECa) maps or from more refined products based on the calibration and inversion of ECa measurements.
The software codes are continuously updated and available by following the references provided throughout the paper or by contacting the authors. Part of the analysis and map production was conducted using ArcGIS version 10.7.1. Additional plots were produced using the portable command-line-driven graphing utility Gnuplot.
Data can be accessed by visiting the Terrestrial Environmental Observatories Data Discovery Portal at https://ddp.tereno.net/ddp/ (TERENO, 2021) and the Collaborative Research Centre (Transregio 32) database at https://www.tr32db.uni-koeln.de/site/index.php (Collaborative Research Centre/Transregio 32, 2021) or by contacting the authors.
The paper was conceptualized by CB and JAH. Methodology was developed by CB, JAH, LW, and MH. Data management and analysis was performed by CB. The original draft was prepared by CB. All authors have participated in writing, reviewing, and editing the final draft and subsequent submissions. All authors have read and agreed to the published version of the paper.
The authors declare that they have no conflict of interest.
Support for this study was received through the Terrestrial Environmental Observatories (TERENO), the Advanced Remote Sensing–Ground Truth Demo and Test Facilities (ACROSS) initiative, and the Deutsche Forschungsgemeinschaft (DFG, German Research Foundation) under Germany's Excellence Strategy – EXC 2070-390732324 project PhenoRob.
Financial support for this study was received through the Deutsche Forschungsgemeinschaft under the Transregional Collaborative Research Centre 32 – Patterns in Soil–Vegetation–Atmosphere Systems: Monitoring, Modelling and Data Assimilation.
The article processing charges for this open-access publication were covered by the Forschungszentrum Jülich.
This paper was edited by Nikolaus J. Kuhn and reviewed by Jacopo Boaga and one anonymous referee.
Ali, M., Montzka, C., Stadler, A., Menz, G., Thonfeld, F., and Vereecken, H.: Estimation and validation of RapidEye-based time-series of leaf area index for winter wheat in the Rur catchment (Germany), Remote Sens.-Basel, 7, 2808–2831, https://doi.org/10.3390/rs70302808, 2015.
Allen, R. G., Pereira, L. S., Raes, D., and Smith, M.: FAO Irrigation and drainage paper 56 – Crop evapotranspiration, Guidelines for computing crop water requirements, FAO, Rome, Italy, 300, D05109, available at: http://www.fao.org/3/X0490E/X0490E00.htm (last access: 13 May 2021), 1998.
Antle, J. M., Basso, B., Conant, R. T., Godfray, H. C. J., Jones, J. W., Herrero, M., Howitt, R. E., Keating, B. A., Munoz-Carpena, R., and Rosenzweig, C.: Towards a new generation of agricultural system data, models and knowledge products: Design and improvement, Agr. Syst., 155, 255–268, https://doi.org/10.1016/j.agsy.2016.10.002, 2017.
Baker, F. G.: Variability of hydraulic conductivity within and between nine Wisconsin soil series, Water Resour. Res., 14, 103–108, 1978.
Beck, P. S., Atzberger, C., Høgda, K. A., Johansen, B., and Skidmore, A. K.: Improved monitoring of vegetation dynamics at very high latitudes: A new method using MODIS NDVI, Remote Sens. Environ., 100, 321–334, 2006.
Binley, A., Hubbard, S. S., Huisman, J. A., Revil, A., Robinson, D. A., Singha, K., and Slater, L. D.: The emergence of hydrogeophysics for improved understanding of subsurface processes over multiple scales, Water Resour. Res., 51, 3837–3866, https://doi.org/10.1002/2015WR017016, 2015.
Boaga, J.: The use of FDEM in hydrogeophysics: A review, J. Appl. Geophys., 139, 36–46, https://doi.org/10.1016/j.jappgeo.2017.02.011, 2017.
Boenecke, E., Lueck, E., Ruehlmann, J., Gruendling, R., and Franko, U.: Determining the within-field yield variability from seasonally changing soil conditions, Precis. Agric., 19, 750–769, https://doi.org/10.1016/S0022-1694(01)00464-4, 2018.
Bogena, H. R., Montzka, C., Huisman, J. A., Graf, A., Schmidt, M., Stockinger, M., von Hebel, C., Hendricks-Franssen, H. J., van der Kruk, J., and Tappe, W.: The TERENO-Rur Hydrological Observatory: A Multiscale Multi-Compartment Research Platform for the Advancement of Hydrological Science, Vadose Zone J., 17, 1–22, https://doi.org/10.2136/vzj2018.03.0055, 2018.
Bolinder, M. A., Angers, D. A., and Dubuc, J. P.: Estimating shoot to root ratios and annual carbon inputs in soils for cereal crops, Agr. Ecosyst. Environ., 63, 61–66, 1997.
Bonfante, A., Agrillo, A., Albrizio, R., Basile, A., Buonomo, R., De Mascellis, R., Gambuti, A., Giorio, P., Guida, G., Langella, G., Manna, P., Minieri, L., Moio, L., Siani, T., and Terribile, F.: Functional homogeneous zones (fHZs) in viticultural zoning procedure: an Italian case study on Aglianico vine, SOIL, 1, 427–441, https://doi.org/10.5194/soil-1-427-2015, 2015.
Boons-Prins, E. R., De Koning, G. H. J., and Van Diepen, C. A.: Crop-specific simulation parameters for yield forecasting across the European Community, CABO-DLO [etc.], Simulation Report CABO-TT nr. 32, Centre for Agrobiological Research (CABO) and Department of Theoretical Production Ecology, Agricultural University Wageningen, Wageningen, the Netherlands, available at: https://library.wur.nl/WebQuery/wurpubs/fulltext/308997 (last access: 13 May 2021), 1993.
Borg, H. and Grimes, D. W.: Depth development of roots with time: An empirical description, T. ASAE, 29, 194–197, 1986.
Bouma, J.: Using soil survey data for quantitative land evaluation, in: Advances in Soil Science, edited by: Stewart, B. A., Springer, New York, USA, https://doi.org/10.1007/978-1-4612-3532-3_4, 177–213, 1989.
Brakensiek, D. L. and Rawls, W. J.: Soil containing rock fragments: effects on infiltration, Catena, 23, 99–110, 1994.
Brevik, E. C., Fenton, T. E., and Lazari, A.: Soil electrical conductivity as a function of soil water content and implications for soil mapping, Precis. Agric., 7, 393–404, https://doi.org/10.1007/s11119-006-9021-x, 2006.
Brogi, C., Huisman, J. A., Pätzold, S., von Hebel, C., Weihermüller, L., Kaufmann, M. S., van der Kruk, J., and Vereecken, H.: Large-scale soil mapping using multi-configuration EMI and supervised image classification, Geoderma, 335, 133–148, https://doi.org/10.1016/j.geoderma.2018.08.001, 2019.
Brogi, C., Huisman, J. A., Herbst, M., Weihermüller, L., Klosterhalfen, A., Montzka, C., Reichenau, T. G., and Vereecken, H.: Simulation of spatial variability in crop LAI and yield using agro-ecosystem modelling and geophysics-based quantitative soil information, Vadose Zone J., 19, e20009, https://doi.org/10.1002/vzj2.20009, 2020.
Campbell, G. S.: Extinction coefficients for radiation in plant canopies calculated using an ellipsoidal inclination angle distribution, Agr. Forest Meteorol., 36, 317–321, 1986.
Chartzoulakis, K. and Bertaki, M.: Sustainable water management in agriculture under climate change, Agric. Agric. Sci. Proc., 4, 88–98, https://doi.org/10.1016/j.aaspro.2015.03.011, 2015.
Coleman, K. and Jenkinson, D. S.: A model for the turnover of carbon in soil: Model desription and windows users guide, Tech. rep., Rothamsted Research, Harpenden, Herts (UK), ISBN 0-951-4456-8-5, 29 pp., 2008.
Collaborative Research Centre/Transregio 32: Database, available at: https://www.tr32db.uni-koeln.de/site/index.php, last access: 13 May 2021.
Corwin, D. L. and Lesch, S. M.: Apparent soil electrical conductivity measurements in agriculture, Comput. Electron. Agr., 46, 11–43, https://doi.org/10.1016/j.compag.2004.10.005, 2005.
Daddow, R. L. and Warrington, G.: Growth-limiting soil bulk densities as influenced by soil texture, Watershed Systems Development Group, USDA Forest Service Fort Collins, Colorado, USA, 17 pp., 1983.
Della Chiesa, S., la Cecilia, D., Genova, G., Balotti, A., Thalheimer, M., Tappeiner, U., and Niedrist, G.: Farmers as data sources: Cooperative framework for mapping soil properties for permanent crops in South Tyrol (Northern Italy), Geoderma, 342, 93–105, https://doi.org/10.1016/j.geoderma.2019.02.010, 2019.
Ehlers, W., Köpke, U., Hesse, F., and Böhm, W.: Penetration resistance and root growth of oats in tilled and untilled loess soil, Soil Till. Res., 3, 261–275, 1983.
ESRI: DIgitalGlobe, GeoEye, Earthstar Geographics, CNES/Airbus DS, USDA, AEX, Getmapping, Aerogrid, IGN, IGP, swisstopo, and the GIS User Comunity, 2016.
FAO: Agribusiness Handbooks, vol. 4, sugar beet and white sugar, FAO, 1999.
Feddes, R. A., Kowalik, J., and Zaradny, H.: Simulation of field water use and crop yield, Simulat. Mg., Wiley, ISBN: 9780470264638, 188 pp., 1978.
Flint, A. L. and Childs, S.: Physical Properties of Rock Fragments and Their Effect on Available Water in Skeletal Soils 1, in: Erosion and productivity of soils containing rock fragments, 91–103, Vol. 13, Wiley, https://doi.org/10.2136/sssaspecpub13.c10, 1984.
Franzen, D. W., Hopkins, D. H., Sweeney, M. D., Ulmer, M. K., and Halvorson, A. D.: Evaluation of soil survey scale for zone development of site-specific nitrogen management, Agron. J., 94, 381–389, 2002.
Galambošová, J., Rataj, V., Prokeinová, R., and Prešinská, J.: Determining the management zones with hierarchic and non-hierarchic clustering methods, Res. Agr. Eng., 60, S44–S51, https://doi.org/10.17221/34/2013-RAE, 2014.
Gebbers, R. and Adamchuk, V. I.: Precision agriculture and food security, Science, 327, 828–831, https://doi.org/10.1126/science.1182768, 2010.
Geologischer Dienst NRW: available at: https://www.gd.nrw.de, last access: 15 October 2018.
Herbst, M., Hellebrand, H., Bauer, J., Huisman, J., Šimůnek, J., Weihermüller, L., Graf, A., Vanderborght, J., and Vereecken, H.: Multiyear heterotrophic soil respiration: Evaluation of a coupled CO2 transport and carbon turnover model, Ecol. Model., 214, 271–283, https://doi.org/10.1016/j.ecolmodel.2008.02.007, 2008.
Heuvelink, G. B. M. and Webster, R.: Modelling soil variation: past, present, and future, Geoderma, 100, 269–301, 2001.
Jakobi, J., Huisman, J. H., Schrön, M., Fiedler, J., Brogi, C., Vereecken, H., and Bogena, H. R.: Error estimation for soil moisture measurements with cosmic-ray neutron sensing and implications for rover surveys, Frontiers in Water, 2, 10, https://doi.org/10.3389/frwa.2020.00010, 2020.
Jonckheere, I., Fleck, S., Nackaerts, K., Muys, B., Coppin, P., Weiss, M., and Baret, F.: Review of methods for in situ leaf area index determination, Part I: Theories, sensors and hemispherical photography, Agr. Forest Meteorol., 121, 19–35, https://doi.org/10.1016/j.agrformet.2003.08.027, 2004.
King, J. A., Dampney, P. M. R., Lark, R. M., Wheeler, H. C., Bradley, R. I., and Mayr, T. R.: Mapping potential crop management zones within fields: use of yield-map series and patterns of soil physical properties identified by electromagnetic induction sensing, Precis. Agric., 6, 167–181, 2005.
Klosterhalfen, A., Herbst, M., Weihermüller, L., Graf, A., Schmidt, M., Stadler, A., Schneider, K., Subke, J. A., Huisman, J. A., and Vereecken, H.: Multi-site calibration and validation of a net ecosystem carbon exchange model for croplands, Ecol. Model., 363, 137–156, https://doi.org/10.1016/j.ecolmodel.2017.07.028, 2017.
Klostermann, J.: Das Quartär der Niederrheinischen Bucht: Ablagerungen der letzten Eiszeit am Niederrhein, Geologisches Landesamt Nordrhein-Westfalen, Wolfratshausen, Germany, 200 pp., 1992.
Krüger, J., Franko, U., Fank, J., Stelzl, E., Dietrich, P., Pohle, M., and Werban, U.: Linking geophysics and soil function modeling – An application study for biomass production, Vadose Zone J., 12, 1–13, https://doi.org/10.2136/vzj2013.01.0015, 2013.
Mertens, F. M., Pätzold, S., and Welp, G.: Spatial heterogeneity of soil properties and its mapping with apparent electrical conductivity, J. Plant Nutr. Soil Sc., 171, 146–154, https://doi.org/10.1002/jpln.200625130, 2008.
Mester, A., Zimmermann, E., Van der Kruk, J., Vereecken, H., and Van Waasen, S.: Development and drift-analysis of a modular electromagnetic induction system for shallow ground conductivity measurements, Meas. Sci. Technol., 25, 055801, https://doi.org/10.1088/0957-0233/25/5/055801, 2014.
Monteiro Santos, F. A., Triantafilis, J., Bruzgulis, K. E., and Roe, J. A. E.: Inversion of multiconfiguration electromagnetic (DUALEM-421) profiling data using a one-dimensional laterally constrained algorithm, Vadose Zone J., 9, 117–125, https://doi.org/10.2136/vzj2009.0088, 2010.
Moral, F., Terrón, J., and Da Silva, J. M.: Delineation of management zones using mobile measurements of soil apparent electrical conductivity and multivariate geostatistical techniques, Soil Till. Res., 106, 335–343, https://doi.org/10.1016/j.still.2009.12.002, 2010.
Nash, J. E. and Sutcliffe, J. V.: River flow forecasting through conceptual models, Part I – A discussion of principles, J. Hydrol., 10, 282–290, https://doi.org/10.1016/0022-1694(70)90255-6, 1970.
Nawar, S., Corstanje, R., Halcro, G., Mulla, D., and Mouazen, A. M.: Delineation of Soil Management Zones for Variable-Rate Fertilization: A Review, Adv. Agron., 143, 175–245, https://doi.org/10.1016/bs.agron.2017.01.003, 2017.
Norman, J. M. and Campbell, G. S.: Canopy structure, in: Plant physiological ecology, edited by: Pearcy, R. W., Ehleringer, J. R., Mooney, H. A., and Rundel, P. W., Springer, Dordrecht, 301–325, https://doi.org/10.1007/978-94-009-2221-1_14, 1989.
NRW: Soil Taxation Map, Bödenschätrzungskarte: Bearbeitet nach den amtlichen Unterlagen der Bodenschätzung und des Geologischen Landesamts Nordrhein-Westfalen vom Regierungspräsidenten in Aachen und dem Geologischen Landesamt Nordrhein-Westfalen 1960, Landesvermessungsamt Nordrhein-Westfalen, 1960.
Nussbaum, M., Spiess, K., Baltensweiler, A., Grob, U., Keller, A., Greiner, L., Schaepman, M. E., and Papritz, A.: Evaluation of digital soil mapping approaches with large sets of environmental covariates, SOIL, 4, 1–22, https://doi.org/10.5194/soil-4-1-2018, 2018.
Oldoni, H. and Bassoi, L. H.: Delineation of irrigation management zones in a Quartzipsamment of the Brazilian semiarid region, Pesqui. Agropecu. Bras., 51, 1283–1294, https://doi.org/10.1590/S0100-204X2016000900028, 2016.
Pätzold, S., Mertens, F. M., Bornemann, L., Koleczek, B., Franke, J., Feilhauer, H., and Welp, G.: Soil heterogeneity at the field scale: a challenge for precision crop protection, Precis. Agric., 9, 367–390, https://doi.org/10.1007/s11119-008-9077-x, 2008.
Paz, J. O.: Analysis of spatial yield variability and economics of prescriptions for precision agriculture: a crop modeling approach, Retrospective Theses and Dissertations, 13920, https://doi.org/10.31274/rtd-180813-15274, 2000.
Penning de Vries, F., Jansen, D., ten Berge, H., and Bakema, A.: Simulation of ecophysiological processes of growth in several annual crops, Pudoc Wageningen, Wageningen, The Netherlands, 1989.
Propastin, P. and Erasmi, S.: A physically based approach to model LAI from MODIS 250 m data in a tropical region, Int. J. Appl. Earth Obs., 12, 47–59, https://doi.org/10.1016/j.jag.2009.09.013, 2010.
Rawls, W. J. and Brakensiek, D. L.: Prediction of soil water properties for hydrologic modeling, in: Watershed management in the eighties, edited by: Jones, E. B., 293–299, American Society of Civil Engineers, New York, NY, 1985.
Reichenau, T. G., Korres, W., Schmidt, M., Graf, A., Welp, G., Meyer, N., Stadler, A., Brogi, C., and Schneider, K.: A comprehensive dataset of vegetation states, fluxes of matter and energy, weather, agricultural management, and soil properties from intensively monitored crop sites in western Germany, Earth Syst. Sci. Data, 12, 2333–2364, https://doi.org/10.5194/essd-12-2333-2020, 2020.
Robert, P.: Characterization of soil conditions at the field level for soil specific management, Geoderma, 60, 57–72, 1993.
Robinson, D. A., Binley, A., Crook, N., Day-Lewis, F., Ferré, T. P. A., Grauch, V. J. S., Knight, R., Knoll, M., Lakshmi, V., and Miller, R.: Advancing process-based watershed hydrological research using near-surface geophysics: A vision for, and review of, electrical and magnetic geophysical methods, Hydrol. Process., 22, 3604–3635, https://doi.org/10.1002/hyp.6963, 2008.
Rogge, D., Bauer, A., Zeidler, J., Mueller, A., Esch, T., and Heiden, U.: Building an exposed soil composite processor (SCMaP) for mapping spatial and temporal characteristics of soils with Landsat imagery (1984–2014), Remote Sens. Environ., 205, 1–17, https://doi.org/10.1016/j.rse.2017.11.004, 2018.
Röhrig, W.: Bodenkarte 1:5000 zur landwirtschaftlichen Standorterkundung (BK5ÖL), 510410 and 510411, Geologisches Landesamt Nordrhein-Westfalen, Krefeld, Germany, 1996.
Ross, J.: The radiation regime and architecture of plant stands, Dr. W. Junk Publisher, The Hague, the Netherlands, 2012.
Rudolph, S., van der Kruk, J., Von Hebel, C., Ali, M., Herbst, M., Montzka, C., Pätzold, S., Robinson, D. A., Vereecken, H., and Weihermüller, L.: Linking satellite derived LAI patterns with subsoil heterogeneity using large-scale ground-based electromagnetic induction measurements, Geoderma, 241, 262–271, https://doi.org/10.1016/j.geoderma.2014.11.015, 2015.
Rum, G., Iamschula, D. E. B., and Paul, H. K.: Prevalence and interrelationships of root, Community Dent. Oral, 2, 295–304, 1974.
Saey, T., De Smedt, P., Islam, M. M., Meerschman, E., Van De Vijver, E., Lehouck, A., and Van Meirvenne, M.: Depth slicing of multi-receiver EMI measurements to enhance the delineation of contrasting subsoil features, Geoderma, 189, 514–521, https://doi.org/10.1016/j.geoderma.2012.06.010, 2012.
Schmidt, M., Reichenau, T. G., Fiener, P., and Schneider, K.: The carbon budget of a winter wheat field: An eddy covariance analysis of seasonal and inter-annual variability, Agr. Forest Meteorol., 165, 114–126, https://doi.org/10.1016/j.agrformet.2012.05.012, 2012.
Simmer, C., Thiele-Eich, I., Masbou, M., Amelung, W., Bogena, H., Crewell, S., Diekkrüger, B., Ewert, F., Hendricks Franssen, H.-J., and Huisman, J. A.: Monitoring and modeling the terrestrial system from pores to catchments: the transregional collaborative research center on patterns in the soil-vegetation-atmosphere system, B. Am. Meteorol. Soc., 96, 1765–1787, https://doi.org/10.1175/BAMS-D-13-00134.1, 2015.
Šimůnek, J. and Suarez, D. L.: Modeling of carbon dioxide transport and production in soil: 1. Model development, Water Resour. Res., 29, 487–497, 1993.
Šimůnek, J., Suarez, D. L., and Šejna, M.: The UNSATCHEM software package for simulating one-dimensional variably saturated water flow, heat transport, carbon dioxide production and transport, and multicomponent solute transport with major ion equilibrium and kinetic chemistry, Res. Rep., research report no. 141, U.S. Salinity Laboratory, Agricultural Research Service, U. S. Department of Agriculture, Riverside, California, 1996.
Söderström, M., Sohlenius, G., Rodhe, L., and Piikki, K.: Adaptation of regional digital soil mapping for precision agriculture, Precis. Agric., 17, 588–607, https://doi.org/10.1007/s11119-016-9439-8, 2016.
Spitters, C., van Keulen, H., and van Kraalingen, D.: A simple and univesral cro growth simulator: SUCROS87, in: Simulation and systems management in crop protection, Pudoc, 147–181, available at: https://library.wur.nl/WebQuery/wurpubs/fulltext/171923 (last access: 13 May 2021), 1989.
Stafford, J. V., Ambler, B., Lark, R., and Catt, J.: Mapping and interpreting the yield variation in cereal crops, Comput. Electron. Agr., 14, 101–119, 1996.
Sylvester-Bradley, R., Lord, E., Sparkes, D., Scott, R. K., Wiltshire, J. J. J., and Orson, J.: An analysis of the potential of precision farming in Northern Europe, Soil Use Manage., 15, 1–8, 1999.
Tan, X., Mester, A., von Hebel, C., Zimmermann, E., Vereecken, H., van Waasen, S., and van der Kruk, J.: Simultaneous calibration and inversion algorithm for multiconfiguration electromagnetic induction data acquired at multiple elevationsCalibration inversion for rigid-boom EMI, Geophysics, 84, EN1–EN14, https://doi.org/10.1190/geo2018-0264.1, 2019.
Taylor, J. C., Wood, G. A., Earl, R., and Godwin, R. J.: Soil factors and their influence on within-field crop variability, part II: spatial analysis and determination of management zones, Biosyst. Eng., 84, 441–453, https://doi.org/10.1016/S1537-5110(03)00005-9, 2003.
TERENO: Data Discovery Portal, available at: https://ddp.tereno.net/ddp/, last access: 13 May 2021.
Terrón, J. M., Blanco, J., Moral, F. J., Mancha, L. A., Uriarte, D., and Marques da Silva, J. R.: Evaluation of vineyard growth under four irrigation regimes using vegetation and soil on-the-go sensors, SOIL, 1, 459–473, https://doi.org/10.5194/soil-1-459-2015, 2015.
Tewes, A., Hoffmann, H., Krauss, G., Schäfer, F., Kerkhoff, C., and Gaiser, T.: New approaches for the assimilation of LAI measurements into a crop model ensemble to improve wheat biomass estimations, Agronomy, 10, 446, https://doi.org/10.3390/agronomy10030446, 2020.
Unger, P. W. and Jones, O. R.: Long-term tillage and cropping systems affect bulk density and penetration resistance of soil cropped to dryland wheat and grain sorghum, Soil Res., 45, 39–57, 1998.
USDA: Soil Texture Calculator, available at: https://www.nrcs.usda.gov/wps/portal/nrcs/detail/soils/survey/?cid=nrcs142p2_054167 (last access: 13 May 2021), 2019.
Van Genuchten, M. T.: A closed-form equation for predicting the hydraulic conductivity of unsaturated soils, Soil Sci. Soc. Am. J., 44, 892–898, 1980.
Van Heemst, H. D. J.: Plant data values required for simple crop growth simulation models: review and bibliography, CABO, Simulation Report CABO-TT nr. 17, Centre for Agrobiological Research (CABO) and Department of Theoretical Production Ecology, Agricultural University Wageningen, 1988. Simulation Reports CABO-TT, Wageningen, the Netherlands, available at: https://library.wur.nl/WebQuery/wurpubs/fulltext/218353 (last access: 13 May 2021), 1988.
Van Looy, K., Bouma, J., Herbst, M., Koestel, J., Minasny, B., Mishra, U., Montzka, C., Nemes, A., Pachepsky, Y. A., and Padarian, J.: Pedotransfer functions in Earth system science: challenges and perspectives, Rev. Geophys., 55, 1199–1256, https://doi.org/10.1002/2017RG000581, 2017.
Vanclooster, M., Viaene, P., and Diels, J. C. K.: WAVE, a mathematical model for simulating water and agrochemicals in the soil and the vadose environment, Reference and user's manual, release 2.0, Institute for Land and Water Management, Katholieke Universiteit, Leuven, Belgium, 154 pp., 1995.
Vereecken, H., Schnepf, A., Hopmans, J. W., Javaux, M., Or, D., Roose, T., Vanderborght, J., Young, M., Amelung, W., and Aitkenhead, M.: Modeling soil processes: Review, key challenges, and new perspectives, Vadose Zone J., 15, 1–57, https://doi.org/10.2136/vzj2015.09.0131, 2016.
von Hebel, C., Rudolph, S., Mester, A., Huisman, J. A., Kumbhar, P., Vereecken, H., and van der Kruk, J.: Three-dimensional imaging of subsurface structural patterns using quantitative large-scale multiconfiguration electromagnetic induction data, Water Resour. Res., 50, 2732–2748, https://doi.org/10.1002/2013WR014864, 2014.
von Hebel, C., Matveeva, M., Verweij, E., Rademske, P., Kaufmann, M. S., Brogi, C., Vereecken, H., Rascher, U., and van der Kruk, J.: Understanding soil and plant interaction by combining ground-based quantitative electromagnetic induction and airborne hyperspectral data, Geophys. Res. Lett., 45, 7571–7579, https://doi.org/10.1029/2018GL078658, 2018.
Von Hebel, C., Van der Kruk, J., Huisman, J. A., Mester, A., Altdorff, D., Endres, A. L., Zimmermann, E., Garré, S., and Vereecken, H.: Calibration, conversion, and quantitative multi-layer inversion of multi-coil rigid-boom electromagnetic induction data, Sensors, 19, 4753, https://doi.org/10.3390/s19214753, 2019.
Wilhelm, W., Ruwe, K., and Schlemmer, M. R.: Comparison of three leaf area index meters in a corn canopy, Crop Sci., 40, 1179–1183, 2000.
Wong, M. T. F. and Asseng, S.: Determining the causes of spatial and temporal variability of wheat yields at sub-field scale using a new method of upscaling a crop model, Plant Soil, 283, 203–215, https://doi.org/10.1007/s11104-006-0012-5, 2006.
Xiao, J. and Moody, A.: A comparison of methods for estimating fractional green vegetation cover within a desert-to-upland transition zone in central New Mexico, USA, Remote Sens. Environ., 98, 237–250, https://doi.org/10.1016/j.rse.2005.07.011, 2005.
Zeng, X., Rao, P., DeFries, R. S., and Hansen, M. C.: Interannual variability and decadal trend of global fractional vegetation cover from 1982 to 2000, J. Appl. Meteorol., 42, 1525–1530, 2003.