the Creative Commons Attribution 4.0 License.
the Creative Commons Attribution 4.0 License.
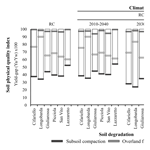
Refining physical aspects of soil quality and soil health when exploring the effects of soil degradation and climate change on biomass production: an Italian case study
Antonello Bonfante
Fabio Terribile
Johan Bouma
This study focuses on soil physical aspects of soil quality and health with the objective to define procedures with worldwide rather than only regional applicability, reflecting modern developments in soil physical and agronomic research and addressing important questions regarding possible effects of soil degradation and climate change. In contrast to water and air, soils cannot, even after much research, be characterized by a universally accepted quality definition and this hampers the internal and external communication process. Soil quality expresses the capacity of the soil to function. Biomass production is a primary function, next to filtering and organic matter accumulation, and can be modeled with soil–water–atmosphere–plant (SWAP) simulation models, as used in the agronomic yield-gap program that defines potential yields (Yp) for any location on earth determined by radiation, temperature and standardized crop characteristics, assuming adequate water and nutrient supply and lack of pests and diseases. The water-limited yield (Yw) reflects, in addition, the often limited water availability at a particular location. Actual yields (Ya) can be considered in relation to Yw to indicate yield gaps, to be expressed in terms of the indicator . Soil data to calculate Yw for a given soil type (the genoform) should consist of a range of soil properties as a function of past management (various phenoforms) rather than as a single representative dataset. This way a Yw-based characteristic soil quality range for every soil type is defined, based on semipermanent soil properties. In this study effects of subsoil compaction, overland flow following surface compaction and erosion were simulated for six soil series in the Destra Sele area in Italy, including effects of climate change. Recent proposals consider soil health, which appeals more to people than soil quality and is now defined by separate soil physical, chemical and biological indicators. Focusing on the soil function biomass production, physical soil health at a given time of a given type of soil can be expressed as a point (defined by a measured Ya) on the defined soil quality range for that particular type of soil, thereby defining the seriousness of the problem and the scope for improvement. The six soils showed different behavior following the three types of land degradation and projected climate change up to the year 2100. Effects are expected to be major as reductions of biomass production of up to 50 % appear likely under the scenarios. Rather than consider soil physical, chemical and biological indicators separately, as proposed now elsewhere for soil health, a sequential procedure is discussed, logically linking the separate procedures.
- Article
(2331 KB) - Full-text XML
- BibTeX
- EndNote
The concept of soil health has been proposed to communicate the importance of soils to stakeholders and policymakers (Moebius-Clune et al., 2016). This follows a large body of research on soil quality, recently reviewed by Bünemann et al. (2018). The latter conclude that research so far has hardly involved farmers and other stakeholders, consultants and agricultural advisors. This may explain why there are as of yet no widely accepted, operational soil quality indicators, in contrast to quality indicators for water and air which are even formalized into specific laws (e.g., EU Water Framework Directive). This severely hampers effective communication of the importance of soils, which is increasingly important to create broad awareness about the devastating effects of widespread soil degradation. New soil health initiatives expanding the existing soil quality discourse deserve to be supported. A National Soil Health Institute has been established in the USA (https://soilhealthinstitute.org/, last access: 29 December 2018) and Cornell University has published a guide for its comprehensive assessment after several years of experimentation (Moebius-Clune et al., 2016). Soil health is defined as “the continued capacity of the soil to function as a vital living ecosystem that sustains plants, animals and humans” (NRCS, 2012). Focusing attention in this paper to soil physical conditions, the Cornell assessment scheme (Moebius-Clune et al., 2016) distinguishes three soil physical parameters – wet aggregate stability, surface and subsurface hardness – to be characterized by penetrometers and the available water capacity (AWC; water held between 1∕3 and 15 bar). The National Soil Health Institute reports 19 soil health parameters, including 5 soil physical ones: water-stable aggregation, penetration resistance, bulk density, AWC and infiltration rate.
Techniques to determine aggregate stability and penetrometer resistance were introduced many years ago (e.g., Kemper and Chepil, 1965; Lowery, 1986; Shaw et al., 1943). Aggregate stability is a relatively static feature as compared with dynamic soil temperature and moisture content, with drawbacks in terms of (1) lack of uniform applied methodology (e.g., Almajmaie et al., 2017), (2) the inability of dry and wet sieving protocols to discriminate between management practices and soil properties (Le Bissonnais, 1996; Pulido Moncada et al., 2013), and above all (3) the fact that mechanical work applied during dry sieving is basically not experienced in real field conditions (Díaz-Zorita et al., 2002). Measured penetrometer resistances are known to be quite variable because of different modes of handling in practice and seasonal variation. Finally, the AWC is a static characteristic based on fixed values as expressed by laboratory measurements of the pressure head for field capacity and wilting point that do not correspond with field conditions (e.g., Bouma, 2018).
These drawbacks must be considered when suggesting the introduction for general use as physical soil health indicators. More recent developments in soil physics may offer alternative approaches, to be explored in this paper, that are more in line with the dynamic behavior of soils.
The definition of soil health is close to the soil quality concept introduced in the 1990s: “the capacity of the soil to function within ecosystem and land-use boundaries to sustain productivity, maintain environmental quality and promote plant and animal health” (Bouma, 2002; Bünemann et al., 2018; Doran and Parkin, 1994; Karlen et al., 1997). Discussions in the early 2000s have resulted in a distinction between inherent and dynamic soil quality. The former would be based on relatively stable soil properties as expressed in soil types that reflect the long-term effect of the soil-forming factors corresponding with the basic and justified assumption of soil classification that soil management should not change a given classification. Nevertheless, soil functioning of a given soil type can vary significantly as a result of the effects of past and current soil management, even though the name of the soil type does not change (this can be the soil series as defined in USDA Soil Taxonomy, Soil Survey Staff, 2014, as expressed in Table 1) but the lowest level in other soil classification systems would also apply. In any case, the classification should be unambiguous. Dynamic soil quality would reflect possible changes as a result of soil use and management over a human timescale, which can have a semipermanent character when considering, for example, subsoil plow pans (e.g., Moebius-Clune et al., 2016). This was also recognized by Droogers and Bouma (1997) and Rossiter and Bouma (2018) when defining different soil phenoforms reflecting effects of land use for a given genoform as distinguished in soil classification. Distinction of different soil phenoforms was next translated into a range of characteristically different soil qualities by using simulation techniques (Bouma and Droogers, 1998). The term soil health appears to have a higher appeal for land users and citizens at large than the more academic term soil quality, possibly because the term “health” has a direct connotation with human wellbeing in contrast to the more distant and abstract term “quality”. Humans differ and so do soils; some soils are genetically more healthy than others and a given soil can have different degrees of health at any given time, which depends not only on soil properties but also on past and current management and weather conditions. Moebius-Clune et al. (2016) have recognized the importance of climate variation by stating that their proposed system only applies to the northeast of the USA and its particular climate and soil conditions. This represents a clear limitation and could in time lead to a wide variety of local systems with different parameters that would inhibit effective communication to the outside world. This paper will therefore explore possibilities for a science-based systems approach with general applicability. To apply the soil health concept to a wider range of soils in other parts of the world, the attractive analogy with human health not only implies that health has to be associated with particular soil individuals, but also with climate zones. In addition, current questions about soil behavior often deal with possible effects of climate change. In this paper, the proposed systems analysis can – in contrast to the procedures presented so far – also deal with this issue. Using soils as a basis for the analysis is only realistic when soil types can be unambiguously defined, as was demonstrated by Bonfante and Bouma (2015) for five soil series in the Italian Destra Sele area that will also be the focus of this study. In most developed countries where soil surveys have been completed, soil databases provide extensive information on the various soil series, including parameters needed to define soil quality and soil health in a systems analysis as shown, for example, for clay soils in the Netherlands (Bouma and Wösten, 2016). The recent report of the National Academy of Sciences, Engineering and Medicine (2018), emphasizes the need for the type of systems approaches as followed in this study.
The basic premise of the soil health concept, as advocated by Moebius-Clune et al. (2016) and others, is convincing. Soil characterization programs since the early part of the last century have been exclusively focused on soil chemistry and soil chemical fertility and this has resulted in not only effective recommendations for the application of chemical fertilizers but also in successful pedological soil characterization research. But soils are living bodies in a landscape context and not only chemical but also physical and biological processes govern soil functions. The soil health concept considers therefore not only soil chemical characteristics, which largely correspond with the ones already present in existing soil fertility protocols, but also with physical and biological characteristics that are determined with well-defined methods, with particular emphasis on soil biological parameters (Moebius-Clune et al., 2016). However, the proposed soil physical methods by Moebius-Clune et al. (2016) do not reflect modern soil physical expertise and procedures need to have a universal rather than a regional character, while pressing questions about the effects of soil degradation and future climate change need to be addressed as well. The proposed procedures do not allow this. Explorative simulation studies can be used to express possible effects of climate change as, obviously, measurements in the future are not feasible. Also, only simulation models can provide a quantitative, interdisciplinary integration of soil–water–atmosphere–plant (SWAP) processes that are key to both the soil quality and soil health definitions, as mentioned above.
In summary, the objectives of this paper are to (i) explore alternative procedures to characterize soil physical quality and health by applying a systems analysis by modeling the soil–water–atmosphere–plant system, an analysis that is valid anywhere on earth; (ii) apply the procedure to develop quantitative expressions for the effects of different forms of soil degradation; and (iii) explore effects of climate change for different soils also considering different forms of soil degradation.
2.1 Soil functions as a starting point
The soil quality and health definitions both mention “the continued capacity of a soil to function” (FAO, 2008; Bünemann et al., 2018). Soil functions therefore have a central role in the quality and health debate. EC (2006) defined the following soil functions: (1) biomass production, including agriculture and forestry; (2) storing, filtering and transforming nutrients, substances and water; (3) biodiversity pool, such as habitats, species and genes; (4) physical and cultural environment for humans and human activities; (5) source of raw material; (6) acting as carbon pool; and (7) archive of geological and archaeological heritage. Functions 4, 5 and 7 are not covered in this contribution since, if considered relevant, specific measures have to be taken to set soils apart by legislative measures. The other functions are directly and indirectly related to Function 1, biomass production. Of course, soil processes not only offer contributions to biomass production, but also to filtering, biodiversity preservation and carbon storage. Inter- and transdisciplinary approaches are needed to obtain a complete characterization, requiring interaction with other disciplines, such as agronomy, hydrology, ecology and climatology and, last but not least, with stakeholders and policymakers. Soil functions thus contribute to ecosystem services and, ultimately, to all 17 UN Sustainable Development Goals (e.g., Bouma, 2016, 2014; Keesstra et al., 2016). However, in the context of this paper, attention will be focused on Function 1, biomass production.
Soil physical aspects play a crucial role when considering the role of soil in biomass production, as expressed by Function 1, which is governed by the dynamics of the soil–water–atmosphere–plant system in three ways:
-
Roots provide the link between the soil and plant. Rooting patterns as a function of time are key factors for crop uptake of water and nutrients. Deep rooting patterns imply less susceptibility to moisture stress. Soil structure, the associated bulk densities and the soil water content determine whether or not roots can penetrate the soil. When water contents are too high, either because of the presence of a water table or of a dense, slowly permeable soil horizon impeding vertical flow, roots will not grow because of lack of oxygen. For example, compact plow pans, resulting from the application of pressure on wet soil by agricultural machinery, can strongly reduce rooting depth. In fact, soil compaction is a major form of soil degradation that may affect up to 30 % of soils in some areas (e.g., FAO and ITPS, 2015).
-
Availability of water during the growing season is another important factor that requires, for a start, infiltration of all rainwater into the soil and its containment in the unsaturated zone, constituting “green water” (e.g., Falkenmark and Rockström, 2006). When precipitation rates are higher than the infiltrative capacity of soils, water will flow laterally away over the soil surface, possibly leading to erosion and reducing the amount of water available for plant growth.
-
The climate and varying weather conditions among the years govern biomass production. Rainfall varies in terms of quantities, intensities and patterns. Radiation and temperature regimes vary as well. In this context, definitions of location-specific potential yield (Yp), water-limited yield (Yw) and actual yield (Ya) are important, as will be discussed later.
Soil Function 2 first requires soil infiltration of water followed by good contact between percolating water and the soil matrix, where clay minerals and organic matter can adsorb cations and organic compounds, involving chemical processes that will be considered when defining soil chemical quality. However, not only the adsorptive character of the soil is important but also the flow rate of applied water that can be affected by climatic conditions or by management when irrigating. Rapid flow rates generally result in poor filtration as was demonstrated for viruses and fecal bacteria in sands and silt loam soils (Bouma, 1979).
Soil functions 3 and 6 are a function of the organic matter content of the soil (or % organic carbon – %OC), the quantity of which is routinely measured in chemical soil characterization programs (also in the soil health protocols mentioned earlier that also define methods to measure soil respiration). The organic matter content of soils is highly affected by soil temperature and moisture regimes and soil chemical conditions. Optimal conditions for root growth in terms of water, air and temperature regimes will also be favorable for soil biological organisms, linking soil functions 1, 3 and 6.
When defining soil physical aspects of soil quality and soil health, focused on soil Function 1, parameters that integrate various aspects will have to be defined, such as (1) weather data; (2) the infiltrative capacity of the soil surface, considering rainfall intensities and quantities; (3) rootability as a function of soil structure, defining thresholds beyond which rooting is not possible; and (4) hydraulic and root extraction parameters that allow a dynamic characterization of the soil–water–atmosphere–plant system. This system can only be realized by process modeling, which requires the five parameters listed above, and is therefore an ideal vehicle to realize interdisciplinary cooperation. Simulation models of the soil–water–atmosphere–plant system are ideal to integrate these various aspects.
2.2 The role of dynamic modeling of the soil–water–atmosphere–plant system
When analyzing soil quality and soil health, emphasis must be on the dynamics of vital, living ecosystems requiring a dynamic approach that is difficult to characterize with static soil characteristics (such as bulk density, organic matter content and texture) except when these characteristics are used as input data into dynamic simulation models of the soil–water–plant–climate system. Restricting attention to soil physical characteristics, hydraulic conductivity (K) and moisture retention properties (O(h)) of soils are applied in such dynamic models. Measurement procedures are complex and can only be made by specialists, making them unsuitable for general application in the context of soil quality and health. They can, however, be easily derived from pedotransfer functions (PTFs) that relate static soil characteristics such as bulk density, texture and %OC to these two properties, as recently summarized by Van Looy et al. (2017). The latter soil characteristics are available in existing soil databases and are required information for the dynamic models predicting biomass production.
Simulation models of the soil–water–atmosphere–plant system, such as the Soil Water Atmosphere Plant model (SWAP) (Kroes et al., 2008) to be discussed later in more detail, integrate weather conditions, infiltration rates, rooting patterns and soil hydrological conditions in a dynamic systems approach that also allows exploration of future conditions following climate change. The worldwide agronomic yield-gap program (http://www.yieldgap.org, last access: 29 December 2018) can be quite helpful when formulating a soil quality and health program with a global significance. So-called water-limited yields (Yw) can be calculated, assuming optimal soil fertility and lack of pests and diseases (e.g Gobbett et al., 2017; van Ittersum et al., 2013; Van Oort et al., 2017). Yw reflects climate conditions at any given location in the world as it is derived from potential production (Yp) that reflects radiation, temperature and basic plant properties, assuming that water and nutrients are available and pests and diseases do not occur. Yw reflects local availability of water. Yw is usually, but not always, lower than Yp. Yw can therefore act as a proxy value for physical soil quality, focusing on Function 1. Note that Yp and Yw, while providing absolute science-based points of reference, include assumptions on soil fertility and crop health.
Actual yields (Ya) are often lower than Yw (e.g., Van Ittersum et al., 2013). The ratio Ya ∕ Yw is an indicator of the so-called yield gap showing how much potential there is at a given site to improve production (http://www.yieldgap.org) (Bouma, 2002). When multiplied with 100, a number between 1 and 100 is obtained as a quantitative measure of the yield gap for a given type of soil. Yw can be calculated for a non-degraded soil. Ya should ideally be measured but can also be calculated as was done in this exploratory study (in terms of Yw values) on the basis of the assumed effects of different forms of soil degradation, such as subsoil soil compaction, poor water infiltration at the soil surface due to surface compaction, or crusting and erosion. This requires the introduction of a compact layer (plow pan) in the soil, a reduction of rainfall amounts with the volume of estimated overland flow and the removal of topsoil. Each variant of the analyzed soil series represents a phenoform. In this exploratory study Ya values were simulated but, ideally, field observations should be made in a given soil type to define effects of management as explored, for example, by Pulleman et al. (2000) for clay soils and Sonneveld et al. (2002) for sandy soils. They developed phenoforms based on different %OC of surface soil and such phenoforms could also have been included here to provide a link with soil biology, but field data were not available to do so. Field work identifying phenoforms includes important interaction with farmers as also mentioned by Moebius-Clune et al. (2016). Sometimes, soil degradation processes, such as erosion, may be so severe that the soil classification (the soil genoform) changes. Then, the soil quality and soil health discussion shifts to a different soil type.
This approach will now be explored with a particular focus on the Mediterranean environment. Physical soil quality is defined by Yw for each soil, considering a soil without assumed degradation phenomena (the reference) and for three variants (hypothetical Ya, expressed in terms of Yw) with (1) a compacted plow layer; (2) a compacted soil surface resulting in overland flow; and (3) removal of topsoil following erosion, without a resulting change in the soil classification. This way a characteristic range of Yw values is obtained for each of the six soil series, reflecting positive and negative effects of soil management and representing a range of soil physical quality values of the particular soil series considered. Within this range an actual value of Ya will indicate the soil physical health of the particular soil at a given time and its position within the range of values will indicate the severity of the problem and potential for possible improvement.
The ratio is calculated to obtain a numerical value that represents soil health as a point value, representing actual conditions. Health is relatively low when real conditions occur in the lower part of the soil quality range for that particular soil and relatively high when it occurs in the upper range. Again, in this exploratory study measured values (at current climate conditions) for Ya have not been made, so Ya only applies to the three degraded soil forms being distinguished here where hypothetical effects of soil degradation have been simulated as related to the corresponding calculated Yw values. Of course, actual measured Ya values cannot be determined at all when considering future climate scenarios and simulation is the only method allowing exploratory studies. We assume that climate change will not significantly affect soil formation processes until the year 2100. Soil properties will therefore stay the same.
To allow estimates of the possible effects of climate change, the Representative Concentration Pathway (RCP) 8.5 scenario based on the Intergovernmental Panel on Climate Change (IPCC) modeling approach will be applied. Obviously, only computer simulations can be used when exploring future conditions – another important reason to use dynamic simulation modeling in the context of characterizing soil quality and soil health. The approach in this paper extends earlier studies on soil quality for some major soil types in the world that did not consider aspects of soil health nor effects of climate change (Bouma, 2002; Bouma et al., 1998).
2.3 Simulation modeling
The soil–water–atmosphere–plant model (Kroes et al., 2008) was applied to solve the soil water balance. SWAP is an integrated physically based simulation model of water, solute and heat transport in the saturated–unsaturated zone in relation to crop growth. In this study only the water flow module was used; it assumes unidimensional vertical flow processes and calculates the soil water flow through the Richards equation. Soil water retention θ(h) and hydraulic conductivity K(θ) relationships as proposed by van Genuchten (1980) were applied. The unit gradient was set as the condition at the bottom boundary. The upper boundary conditions of SWAP in agricultural crops are generally described by the potential evapotranspiration ETp, irrigation and daily precipitation. Potential evapotranspiration was then partitioned into potential evaporation and potential transpiration according to the LAI (leaf area index) evolution, following the approach of Ritchie (1972). The water uptake and actual transpiration were modeled according to Feddes et al. (1978), where the actual transpiration declines from its potential value through the parameter α, varying between 0 and 1 according to the soil water potential.
The model was calibrated and validated by measured soil water content data at different depths for Italian conditions (Bonfante et al., 2010; Crescimanno and Garofalo, 2005) and in the same study area by Bonfante et al. (2011, 2017). In particular, the model was evaluated in two farms inside of Destra Sele area, on three different soils (Udic Calciustert, Fluventic Haplustept and Typic Calciustoll), under maize crop (two cropping seasons) during the regional project Campania Nitrati (Regione Campania, 2008) (Table 2).
Soil hydraulic properties of soil horizons in the area were estimated by the pedotransfer function HYPRES (Wösten et al., 1999). A reliability test of this PTF was performed on θ(h) and K(θ) measured in the laboratory by the evaporation method (Basile et al., 2006) on 10 undisturbed soil samples collected in the Destra Sele area. The data obtained were compared with estimates by HYPRES and were considered to be acceptable (RMSE = 0.02 m3 m−3) (Bonfante et al., 2015).
Simulations were run considering a soil without assumed degradation phenomena (the reference) and for three variants with a compacted plow layer, surface runoff and erosion, as discussed above.
- i.
The compacted plow layer was applied at −30 cm (10 cm of thickness) with the following physical characteristics: 0.30 WC at saturation, 1.12 n, 0.004 “a” and Ks of 2 cm day−1. Roots were restricted to the upper 30 cm of the soil.
- ii.
Runoff from the soil surface was simulated removing ponded water resulting form intensive rainfall events. Rooting depth was assumed to be −80 cm.
- iii.
Erosion was simulated for the Ap horizon, reducing the upper soil layer to 20 cm. The maximum rooting depth was assumed to be 60 cm (A + B horizon) with a higher root density in the Ap horizon.
Variants were theoretical but based on local knowledge of the Sele Plain. Compaction is relevant considering the highly specialized and intensive horticulture land use of the Sele Plain which typically involves repetitive soil tillage at similar depth. Runoff and erosion easily occur at higher altitude plain areas especially where the LON0, CIF0/RAG0, and GIU0 soil types occur (Fig. 1).
2.4 Soils in the Destra Sele area in Italy
The Destra Sele study area, the plain of the Sele river (22 000 ha, of which 18 500 ha is farmed), is situated in the south of Campania, southern Italy (Fig. 1). The main agricultural production consists of irrigated crops (maize, vegetables and fruit orchards), greenhouse-grown vegetables and mozzarella cheese from water buffalo herds. The area can be divided into four different landform classes (foothills, alluvial fans, fluvial terraces and dunes) with heterogeneous parent materials in which 20 different soil series were distinguished (within Inceptisol, Alfisol, Mollisol, Entisol and Vertisol soil orders) (Regione Campania, 1996), according to Soil Taxonomy (Soil Survey Staff, 1999). Six soil series were selected in the area to test application of the soil quality and soil health concepts. Representative data for the soils are presented in Table 1.
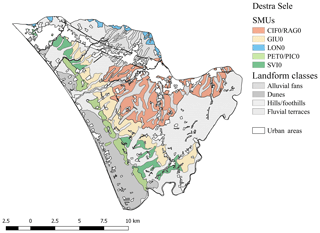
Figure 1The four landform classes of the Destra Sele area and the soil map units (SMUs) of selected six soil typological units (STUs, which are similar to the USDA soil series) (CIF0/RAG0, Cifariello; GIU0, Giuliarossa; LAZ0, Lazzaretto; LON0, Longobarda; PET0/PIC0, Picciola; SVI0, San Vito).
Decision trees were developed to test whether the selection process of the soil series was based on stable criteria, allowing extrapolation of results from measured to unmeasured locations when considering effects of climate change. While extrapolation in space of soil series data has been a common procedure in soil survey (e.g., Soil Survey Staff, 2014; Bouma et al., 2012), extrapolation in time has not received as much attention. A basic principle of many taxonomic soil classification systems is a focus on stable soil characteristics when selecting diagnostic criteria for soil types. Also, emphasis on morphological features allows, in principle, a soil classification without requiring elaborate laboratory analyses (e.g., Soil Survey Staff, 2014). A given soil classification should, in order to obtain permanent names, not change following traditional management measures, such as plowing. This does, however, not apply to all soils and therefore a different name will have to be assigned.
This way, soil classification results in an assessment of the (semi)permanent physical constitution of a given soil in terms of its horizons and textures. That is why soil quality is defined for each soil type as a characteristic range of Yw values, representing different effects of soil management that have not changed the soil classification.
2.5 Climate information
Future climate scenarios were obtained by using the high-resolution regional climate model (RCM) COSMO-CLM (Rockel et al., 2008), with a configuration employing a spatial resolution of 0.0715∘ (about 8 km), which was optimized over the Italian area. The validations performed showed that these model data agree closely with different regional high-resolution observational datasets, in terms of both average temperature and precipitation in Bucchignani et al. (2015) and in terms of extreme events in Zollo et al. (2015).
In particular, the Representative Concentration Pathway 8.5 scenario was applied, based on the IPCC modeling approach to generate greenhouse gas (GHG) concentrations (Meinshausen et al., 2011). Initial and boundary conditions for running RCM simulations with COSMO-CLM were provided by the general circulation model CMCC-CM (Scoccimarro et al., 2011), whose atmospheric component (ECHAM5) has a horizontal resolution of about 85 km. The simulations covered the period from 1971 to 2100; more specifically, the CMIP5 historical experiment (based on historical greenhouse gas concentrations) was used for the period 1976–2005 (reference climate scenario – RC), while for the period 2006–2100, a simulation was performed using the IPCC scenario mentioned. The analysis of results was made on RC (1971–2005) and RCP 8.5 divided into three different time periods (2010–2040, 2040–2070 and 2070–2100). Daily reference evapotranspiration (ET0) was evaluated according to the Hargreaves and Samani (1985) equation (HS). The reliability of this equation in the study area was performed by Fagnano et al. (2001), comparing the HS equation with the Penman–Monteith (PM) equation (Allen et al., 1998).
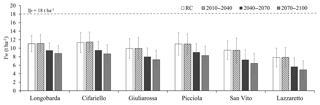
Figure 2Simulated Yw values for all soil series, considering the reference climate (RC; 1971–2005) and future climate scenarios (RCP 8.5) expressed for three time periods (2010–2040, 2040–2070 and 2070–2100). The Yp (potential yield) is the maize production for the Destra Sele area assuming optimal irrigation and fertilization and no pests and diseases. Yp is only calculated for the reference climate.
Under the RCP 8.5 scenario the temperature in Destra Sele is expected to increase by approximately 2 ∘C respectively every 30 years to 2100 starting from the RC. The differences in temperature between RC and the period 2070–2100 showed an average increase in minimum and maximum temperatures of about 6.2 ∘C (for both min and max). The projected increase in temperatures produces an increase in the expected ET0. In particular, during the maize growing season, an average increase in ET0 of about 18 % is expected until 2100.
3.1 Soil physical quality of the soil series, as expressed by Yw, under current and future climates
Soil physical quality of the six soil series, expressed as calculated Yw values for the reference climate and for future climate scenario RCP 8.5 as well as for three time periods, is shown in Fig. 2. Considering current climate conditions, the Longobarda and Cifariello soils with loamy textures have the highest values, while the sandy soil Lazzaretto is lower. This can be explained by greater water retention of loamy soils (180 and 152 mm of AWC in the first 80 cm for Longobarda and Cifariello respectively) compared to the sandy soil (53 mm of AWC in the first 80 cm for Lazzaretto). The effects of climate change are most pronounced and quite clear for the two periods after 2040. Reductions compared with the period up to 2040 range from 20 %–40 %, the highest values associated with sandier soil textures. This follows from the important reduction of projected rainfall during the cropping season (Fig. 3) ranging from an average value of 235 (±30) mm in the 2010–2040 period to 185 (±26) mm (−21 %) and to 142 (±24) mm (−40 %) in the 2040–2070 and 2070–2100 periods respectively (significant at p<0.01). The figure also includes a value for Yp, potential production (under RC with optimal irrigation), which is 18 t ha−1, well above the Yw values. Only a Yp value is presented for current conditions because estimates for future climates involve too many unknown factors.
3.2 Projected effects of soil degradation processes
3.2.1 Projected effects of subsoil compaction
The projected effects of soil compaction are shown in Fig. 4. The effects of compaction are very strong in all soils, demonstrating that restricting the rooting depth has major effects on biomass production. Compared with the reference, reductions in Yw do not occur in the first time window (2010–2040), while the projected lower precipitation rates are expected to have a significant effect on all soils, strongly reducing Yw values by 44 %–55 % with, again, highest values in the sandy soils. Clearly, any effort to increase the effective rooting patterns of crops should be a key element when considering attempts to combat effects of climate change. Data indicate that reactions are soil specific.
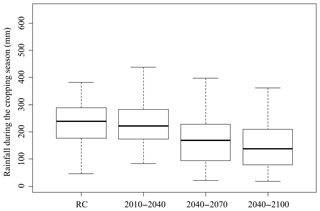
Figure 3Cumulated rainfall during the maize growing season (April–August) in the four climate periods.
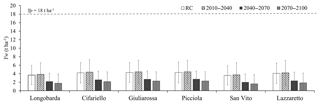
Figure 4The projected effects of simulated soil compaction on Yw for all the soil series assuming the presence of a compacted plow layer at 30 cm depth. Other terms are explained in Fig. 2.
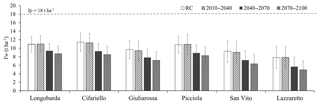
Figure 5The projected effects of simulated surface runoff of water on Yw for all the soil series. Runoff occurs when rainfall intensity is higher than the assumed infiltrative capacity of the soil. Other terms are explained in Fig. 2.
3.2.2 Projected effects of overland flow
Results presented in Fig. 5 show relatively small differences (5 % or less) with results presented in Fig. 2 that were based on complete infiltration of rainwater. This implies that surface crusting or compaction of surface soil, leading to lower infiltration rates and more surface runoff, does not seem to have played a major role here in the assumed scenarios. Real field measurements may well produce different results. Even though projected future climate scenarios predict rains with higher intensities that were reflected in the climate scenarios being run, the effects of lower precipitation, as shown in Fig. 3, appear to dominate.
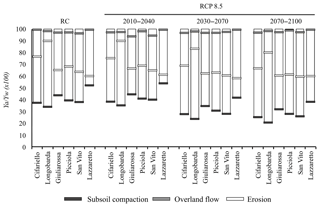
Figure 7Range of soil physical quality indexes for all the soil series, expressing the effects of different forms of soil degradation and climate change. The vertical bars for each type of soil (the genoform) represent a “thermometer” indicating a characteristic range of values obtained by establishing a series of phenoforms, represented by their Yw values. Soil quality for a given soil is thus represented by a characteristic range of values. Soil health is indicated by the particular location of an actual Ya within this range.
3.2.3 Projected effects of erosion
Results presented in Fig. 6, show significant differences with results presented in Fig. 2. Yw values are lower in all soils as compared with reference climate conditions, but loamy and clayey subsoils still can still provide moisture to plant roots, leading to relatively low reductions of Yw (e.g 10 %–20 % for the Longobarda and Cifariello soils, with an AWC of the remaining 60 cm depth of 150 and 120 mm respectively) even though topsoils with a relatively high organic matter content have been removed. Next are the Picciola, Giuliarossa and San Vito soils with reductions between 35 % and 45 %, all with an AWC of approximately 107 mm. Effects of erosion are strongest in the sandy Lazzaretto soil, where loss of the A horizon has a relatively strong effect on the moisture supply capacity of the remaining soil with an AWC of 33 mm up to the new 60 cm depth. The reduction with the reference level is 30 %, which is relatively low because the reference level was already low as well. Projected effects of climate change are again strong for all soils, leading to additional reductions of Yw of approximately 30 %.
3.2.4 Indicators for the soil quality range
Figure 7 presents the physical soil quality ranges for all the soil series, expressed separately as bars for each of the climate periods. The index illustrates that ranges are significantly different. The upper limit is theoretically 100 %. But Van Ittersum et al. (2013) have suggested that an 80 % limit would perhaps be more realistic. Figure 7, ranging to 100 %, shows the lower limits for the ranges to vary from for example 35 (Longobarda) to 55 (Lazaretto) for the reference climate with values for the three phenoforms in between. decreases as a projected reaction to climate change (e.g., 20 for Longobarda and 40 for Lazaretto). This provides important signals for the future.
As discussed, the presented ranges are soil specific and are based on hypothetical conditions associated with different forms of land degradation. Field research may well result in different ranges also possibly considering different soil degradation factors beyond compaction, surface runoff and erosion. Nevertheless, principles involved are identical. Ranges presented in Fig. 7 represent a physical soil quality range that is characteristic for that particular type of soil. Actual values (Ya) will fit somewhere in this range and will thus indicate how far they are removed from the maximum and minimum value, thereby presenting a quantitative measure for soil physical health. This cannot only be important for communication purposes but it also allows a judgment of the effects of different forms of degradation in different soils as well as potential for improvement.
Linking the soil quality and soil health discussion with the international research program on the yield gap allows direct and well-researched expressions for crop yields, defining soil Function 1, as discussed above. The potential yield (Yp) and water-limited yield (Yw) concepts apply worldwide and provide, therefore, a sound theoretical basis for a general soil quality and health classification, avoiding many local and highly diverse activities as reviewed by Büneman et al. (2018). Of course, different indicator crops will have to be defined for different areas in the world.
Linking soil quality and health to specific and well-defined soil types is essential because soil types, such as the soil series presented in this paper, uniquely reflect soil-forming processes in a landscape context. They provide much more information than just a collection of soil characteristics, such as texture, organic matter content and bulk density. They are well known to stakeholders and policymakers in many countries. A good example is the USA where state soils have been defined.
Defining (semipermanent) soil quality for specific soil types, in terms of a characteristic range of Yw values reflecting effects of different forms of land management, represents a quantification of the more traditional soil survey interpretations or land evaluations where soil performance was judged by qualitative, empirical criteria. (e.g., FAO, 2007; Bouma et al 2012).
In this exploratory study, hypothetical effects of three forms of soil degradation were tested. In reality, soil researchers should go to the field and assemble data for a given soil series as shown on soil maps, establishing a characteristic range of properties, following the example of Pulleman et al. (2000) for a clay soil and Sonneveld et al. (2002) for a sand soil, but not restricting attention to %OC, as in these two studies, but including at least bulk density measurements. This way, a characteristic series of phenoforms can be established. Physical soil quality (for a given soil type, which is the genoform) has a characteristic range of Yw values, as shown in Fig. 7. Soil physical health at any given time is reflected by the position of real Ya values within that range and can be expressed by a number .
One could argue that this range acts as a thermometer for a particular type of soil allowing the determination of the physical health of a given soil by the placement of Ya.
But calculating Yw has implications beyond defining physical soil quality and health. As discussed, Yw not only reflects the effects of soil moisture regimes but also assumes that chemical conditions for crop growth are optimal and that pests and diseases do not occur. Defining Yw can thus function as a starting point of a general soil quality and soil health discussion. If Ya is lower than Yw the reasons must be found. Is it lack of water, nutrients, or occurrence of pests and diseases? Irrigation may be difficult to realize but fertility can be restored rather easily and many methods, biological or chemical, are available to combat pests and diseases. If phenoforms that consider different %OC of surface soil (as discussed above) were to be included, low %OC contents could also be a reason for relatively low Yw values. This would cover soil biological quality with %OC acting as proxy value. This way, the Yw analysis can be a logical starting point for follow-up discussions defining appropriate forms of future soil management.
This paper has focused on physical aspects but the proposed procedure has the potential to extend the discussion to chemical and biological aspects, to be further explored in future. Rather than consider the physical, chemical and biological aspects separately, each with their own indicators as proposed by Moebius-Clune et al. (2016), following a logical and interconnected sequence considering first pedological (soil types) and soil physical (Yw) characterizations, to be followed by analyzing chemical and biological aspects, that can possibly explain relatively low Ya values could be more effective. This is more relevant because the definition of reproducible biological soil health parameters is still an object of study (Wade et al., 2018) and %OC might be an acceptable proxy for soil biology for the time being. Recent tests of current soil health protocols have not resulted in the adequate expression of soil conditions in North Carolina (Roper et al., 2017), indicating the need for further research as suggested in this paper.
-
Lack of widely accepted, operational criteria to express soil quality and soil health is a barrier for effective external communication of the importance of soil science.
-
Using well-defined soil types as carriers of information on soil quality and soil health can improve communication to stakeholders and the policy arena.
-
A universal system defining soil quality and soil health is needed based on reproducible scientific principles that can be applied all over the world, avoiding a multitude of different local systems. Models of the soil–water–atmosphere–plant system can fulfil this role.
-
Connecting with the international yield-gap program, applying soil–water–atmosphere–plant simulation models, will facilitate cooperation with agronomists which is essential to quantify the important soil Function 1: biomass production.
-
The proposed system allows an extension of classical soil classification schemes, defining genoforms, by allowing estimates of effects of various forms of past and present soil management (phenoforms) within a given genoform that often strongly affects soil performance. Quantitative information thus obtained can improve current empirical and qualitative soil survey interpretations and land evaluation.
-
Rather than consider physical, chemical and biological aspects of soil quality and health separately, a combined approach starting with pedological and soil physical aspects followed by chemical and biological aspects, all to be manipulated by management, is to be preferred.
-
Only the proposed modeling approach allows exploration of possible effects of climate change on future soil behavior, which is a necessity considering societal concerns and questions.
-
Field work, based on existing soil maps to select sampling locations for a given genoform, is needed to identify a characteristic range of phenoforms for a given genoform, which, in turn, can define a characteristic soil quality range by calculating Yw values.
The underlying research data used can be accessed as follows: soil map and related soil information (the data used are already reported in the Table 1 of this paper) can be downloaded at http://www.agricoltura.regione.campania.it/pubblicazioni/pdf/destra-sele.pdf (last access: 8 January 2018). The data are freely distributed by the Italian regional public body of Regione Campania Assessorato all'Agricoltura SeSIRCA (http://www.agricoltura.regione.campania.it/, last access: 8 January 2018). The report has been printed by Società Editrice Imago Media (e-mail: magomedia@inwind.it). The climate information (RCP 8.5 scenario) applied in the research study has been published by Bucchignani et al. (2015) and the raw version can be requested from the CMCC, Euro-Mediterranean Center on Climate Change (https://www.cmcc.it/, last access: 8 January 2018).
AB contributed the soil data and simulation results, FT contributed the background soil data and JB suggested the study.
The authors declare that they have no conflict of interest.
We acknowledge Eugenia Monaco and Langella Giuliano for
supporting the analysis of the climate scenario; the Regional Models and
Geo-Hydrogeological Impacts Division, Centro Euro-Mediterraneo sui
Cambiamenti Climatici (CMCC, Euro-Mediterranean Center on Climate Change),
Capua (CE), Italy; and Paola Mercogliano and Edoardo Bucchignani for the climate information applied in
this work.
Edited by: Paul Hallett
Reviewed by: David Rossiter and Edward A. Nater
Allen, R. G., Pereira, L. S., Raes, D., Smith, M., and W, A.B.: Crop evapotranspiration – Guidelines for computing crop water requirements – FAO Irrigation and drainage paper 56, Irrig. Drain., 1–15, https://doi.org/10.1016/j.eja.2010.12.001, 1998.
Almajmaie, A., Hardie, M., Acuna, T., and Birch, C.: Evaluation of methods for determining soil aggregate stability, Soil Till. Res. 167, 39–45, https://doi.org/10.1016/j.still.2016.11.003, 2017.
Basile, A., Coppola, A., De Mascellis, R., and Randazzo, L.: Scaling approach to deduce field unsaturated hydraulic properties and behavior from laboratory measurements on small cores, Vadose Zone J., 5, 1005–1016, https://doi.org/10.2136/vzj2005.0128, 2006.
Bonfante, A. and Bouma, J.: The role of soil series in quantitative land evaluation when expressing effects of climate change and crop breeding on future land use, Geoderma, 259–260, 187–195, 2015.
Bonfante, A., Basile, A., Acutis, M., De Mascellis, R., Manna, P., Perego, A., and Terribile, F.: SWAP, CropSyst and MACRO comparison in two contrasting soils cropped with maize in Northern Italy, Agr. Water Manage., 97, 1051–1062, https://doi.org/10.1016/j.agwat.2010.02.010, 2010.
Bonfante, A., Basile, A., Manna, P., and Terribile, F.: Use of Physically Based Models to Evaluate USDA Soil Moisture Classes, Soil Sci. Soc. Am. J., 75, 181–191, https://doi.org/10.2136/sssaj2009.0403, 2011.
Bonfante, A., Monaco, E., Alfieri, S. M., De Lorenzi, F., Manna, P., Basile, A., and Bouma, J.: Climate change effects on the suitability of an agricultural area to maize cultivation: Application of a new hybrid land evaluation system, Adv. Agron., 133, 33–69, https://doi.org/10.1016/bs.agron.2015.05.001, 2015.
Bonfante, A., Impagliazzo, A., Fiorentino, N., Langella, G., Mori, M., and Fagnano, M.: Supporting local farming communities and crop production resilience to climate change through giant reed (Arundo donax L.) cultivation: An Italian case study, Sci. Total Environ., 601–602, 603–613, https://doi.org/10.1016/j.scitotenv.2017.05.214, 2017.
Bouma, J.: Subsurface applications of sewage effluent, in: Planning the uses and management of land. Agronomy 21, edited by: Beatty, M. T., Petersen, G. W., and Swindale, L. D., ASA-CSSA-SSSA, Madison, USA, 665–703, 1979.
Bouma, J.: Land quality indicators of sustainable land management across scales, Agr. Ecosyst. Environ., 88, 129–136, https://doi.org/10.1016/S0167-8809(01)00248-1, 2002.
Bouma, J.: Soil science contributions towards sustainable development goals and their implementation: linking soil functions with ecosystem services, J. Plant Nutr. Soil Sci., 177, 111–120, 2014.
Bouma, J.: Hydropedology and the societal challenge of realizing the 2015 United Nations Sustainable Development Goals, Vadose Zone J., 15, 36–48, https://doi.org/10.2136/vzj2016.09.0080, 2016.
Bouma, J.: Comment on Minashy and Mc Bratney, 2017. Limited effect of organic matter on soil available water capacity, Eur. J. Soil Sci., 69, p. 154, https://doi.org/10.1111/ejss.12509, 2018.
Bouma, J. and Droogers, P.: A procedure to derive land quality indicators for sustainable agricultural production, World Bank Discuss. Pap., 103–110 available at: http://www.archive.org/details/plantrelationsfi00coul (last access: 29 December 2018), 1998.
Bouma, J. and Wösten, J. H. M.: How to characterize good and greening in the EU Common Agricultural Policy (CAP): The case of clay soils in the Netherlands, Soil Use Manage., 32, 546–552, 2016.
Bouma, J., Batjes, N. H., and Groot, J. J. R.: Exploring land quality effects on world food supply, Geoderma, 86, 43–59, 1998.
Bouma, J., Stoorvogel, J. J., and Sonneveld, W. M. P.: Land Evaluation for Landscape Units. Handbook of Soil Science, Second Edition, edited by: Huang, P. M., Li, Y., and Summer, M., chap. 34. P.34-1 to 34-22, CRC Press, London, UK, Boca Raton, New York, USA, 2012.
Bucchignani, E., Montesarchio, M., Zollo, A. L., and Mercogliano, P.: High-resolution climate simulations with COSMO-CLM over Italy: performance evaluation and climate projections for the 21st century, Int. J. Climatol., 36, 735–756, 2015.
Bünemann, E. K., Bongiorno, G., Bai, Z., Creamer, R. E., De Deyn, G., de Goede, R., Fleskens, L., Geissen, V., Kuyper, T. W., Mäder, P., Pulleman, M. M., Sukkel, W., van Groenigen, J. W., and Brussaard, L.: Soil quality – A critical review, Soil Biol. Biochem., 120, 105–125, 2018.
Crescimanno, G. and Garofalo, P.: Application and evaluation of the SWAP model for simulating water and solute transport in a cracking clay soil, Soil Sci. Soc. Am. J., 69, 1943–1954, 2005.
Díaz-Zorita, M., Duarte, G. A., and Grove, J. H.: A review of no-till systems and soil management for sustainable crop production in the subhumid and semiarid Pampas of Argentina, Soil Till. Res., 65, 1–18, 2002.
Doran, J. W. and Parkin, T. B.: Defining and assessing soil quality, in: Defining Soil Quality for a Sustainable Environment, edited by: Doran, J. W., Coleman, D. C., Bezdicek, D. F., and Stewart, B. A., Soil Science Society of America Journal, Madison, USA, 3–21, https://doi.org/10.2136/sssaspecpub35.c1, 1994.
Droogers, P. and Bouma, J.: Soil survey input in exploratory modeling of sustainable soil management practices, Soil Sci. Soc. Am. J., 61, 1704–1710, 1997.
European Commission (EC): Communication from the Commission to the Council, the European Parliament, the European Economic and Social Committee and the Committee of the Regions, Thematic Strategy for Soil Protection, COM 231 Final, Brussels, Belgium, 2006.
Fagnano, M., Acutis, M., and Postiglione, L.: Valutazione di un metodo semplificato per il calcolo dell'ET0 in Campania, Model. di Agric. sostenibile per la pianura meridionale Gest. delle risorse idriche nelle pianure irrigue, Gutenberg, Salerno, Italy, 2001.
Falkenmark, M. and Rockström, J.: The new blue and green water paradigm: Breaking new ground for water resources planning and management, J. Water Res. Plan. Man., 132-3, https://doi.org/10.1061/(ASCE)0733-9496(2006)132:3(129), 2006.
FAO: Land Evaluation: Towards a revised framework. Land & Water Discussion paper 6, FAO, Rome, Italy, 2007.
FAO: An international technical workshop Investing in sustainable crop intensification The case for improving soil health, Integrated Crop Management Vol.6-2008, FAO, Rome, Italy, 2008.
FAO, ITPS: Status of the World's Soil Resources (SWSR) – Main Report, Food and Agriculture Organization of the United Nations and Intergovernmental Technical Panel on Soils, Rome, Italy, 650 pp., 2015.
Feddes, R. A., Kowalik, P. J., and Zaradny, H.: Simulation of field water use and crop yield, PUDOC (Centre for Agricultural Publishing and Documentation), Wageningen, the Netherlands, 1978.
Gobbett, D. L., Hochman, Z., Horan, H., Garcia, J. N., Grassini, P., and Cassman, K. G.: Yield gap analysis of rainfed wheat demonstrates local to global relevance, J. Agr. Sci., 155, 282–299, 2017.
Hargreaves, G. H. and Samani, Z. A.: Reference crop evapotranspiration from temperature, Appl. Eng. Agric., 1, 96–99, 1985.
Karlen, D. L., Mausbach, M. J., Doran, J. W., Cline, R. G., Harris, R. F., and Schuman, G. E.: Soil quality: a concept, definition, and framework for evaluation (a guest editorial), Soil Sci. Soc. Am. J., 61, 4–10, 1997.
Keesstra, S. D., Bouma, J., Wallinga, J., Tittonell, P., Smith, P., Cerdà, A., Montanarella, L., Quinton, J. N., Pachepsky, Y., van der Putten, W. H., Bardgett, R. D., Moolenaar, S., Mol, G., Jansen, B., and Fresco, L. O.: The significance of soils and soil science towards realization of the United Nations Sustainable Development Goals, SOIL, 2, 111–128, https://doi.org/10.5194/soil-2-111-2016, 2016.
Kemper, W. D. and Chepil, W. S.: Size distribution of aggregates, CA Black Methods of soil analysis. Part I. Agron. Monogr. 9, ASA and SSSA, Madison, WI, USA, Size Distrib. aggregates, 499–509, 1965.
Kroes, J. G., Van Dam, J. C., Groenendijk, P., Hendriks, R. F. A., and Jacobs, C. M. J.: SWAP version 3.2. Theory description and user manual, Alterra Rep., Wageningen, the Netherlands, 1649, 2008.
Le Bissonnais, Y. L.: Aggregate stability and assessment of soil crustability and erodibility: I. Theory and methodology, Eur. J. Soil Sci., 47, 425–437, 1996.
Lowery, B.: A Portable Constant-rate Cone Penetrometer 1, Soil Sci. Soc. Am. J., 50, 412–414, 1986.
Meinshausen, M., Smith, S. J., Calvin, K., Daniel, J. S., Kainuma, M. L. T., Lamarque, J. F., Matsumoto, K., Montzka, S. A., Raper, S. C. B., Riahi, K., Thomson, A., Velders, G. J. M., and van Vuuren, D. P. P.: The RCP greenhouse gas concentrations and their extensions from 1765 to 2300, Clim. Change, 109, 213–241, https://doi.org/10.1007/s10584-011-0156-z, 2011.
Moebius-Clune, B. N., Moebius-Clune, D. J., Gugino, B. K., Idowu, O. J., Schindelbeck, R. R., Ristow, A. J., van Es, H. M., Thies, J. E., Shayler, H. A., McBride, M. B., Kurtz, K. S. M., Wolfe, D. W., and Abawi, G. S.: Comprehensive assessment of soil health: The Cornell Framework Manual, edn. 3.1, Cornell Univ., Ithaca, NY, USA, 2016.
National Academy of Sciences, Engineering and Medecine: Consensus Study Report: Science breakthroughs to advance Food and Agricultural Research by 2030. National Academic Press, Washington, D.C., USA, 2018.
Natural Resources Conservation Services (NRCS): Soil Health, available at: http://www.nrcs.usda.gov/wps/portal/nrcs/main/soils/health/ (last access: 23 June 2016), 2012.
Pulido Moncada, M., Gabriëls, D., Lobo, D., Rey, J. C., and Cornelis, W.: Field assessment of soil structural quality in tropical conditions, in: Desertification and Land Degradation: Processes and Mitigation. UNESCO Chair of Eremology, Ghent University, Ghent, Belgium, 135–143, 2013.
Pulleman, M. M., Bouma, J., Van Essen, E. A., and Meijles, E. W.: Soil organic matter content as a function of different land use history, Soil Sci. Soc. Am. J., 64, 689–693, 2000.
Regione_Campania: I Suoli della Piana in Destra Sele. Progetto carta dei Suoli della Regione Campania in scala 1 : 50.000 e lotto CP1 e Piana destra Sele (Salerno), Regione Campania, Assessorato all'Agricoltura, SeSIRCA, available at: http://www.agricoltura.regione.campania.it/pubblicazioni/pdf/destra-sele.pdf (last access: 8 January 2019), 1996.
Regione Campania: La ricerca sull'inquinamento da nitrati nei suoli campani: un approccio modellistico nella gestione agro-ambientale, Regione Campania, Assessorato all'Agricoltura ed alle Attività Produttive, SeSIRCA, Napoli, Italy, 2008.
Ritchie, J. T.: Model for predicting evaporation from a row crop with incomplete cover, Water Resour. Res., 8, 1204–1213, 1972.
Rockel, B., Will, A., and Hense, A.: The regional climate model COSMO-CLM (CCLM), Meteorol. Z., 17, 347–348, 2008.
Roper, W. R., Osmond, D. L., Heitman, J. L., Wagger, M. G., and Reberg-Horton, S. C.: Soil health indicators do not differentiate among agronomic management systems in North Carolina soils, Soil Sci. Soc. Am. J., 81, 828–843, https://doi.org/10.2136/sssaj2016.12.0400, 2017.
Rossiter, D. G. and Bouma, J.: A new look at soil phenoforms – Definition, identification, mapping, Geoderma, 314, 113–121, 2018.
Scoccimarro, E., Gualdi, S., Bellucci, A., Sanna, A., Fogli, P. G., Manzini, E., Vichi, M., Oddo, P., and Navarra, A.: Effects of Tropical Cyclones on Ocean Heat Transport in a High-Resolution Coupled General Circulation Model, J. Climate, 24, 4368–4384, https://doi.org/10.1175/2011jcli4104.1, 2011.
Shaw, B. T., Haise, H. R., and Farnsworth, R. B.: Four Years' Experience with a Soil Penetrometer 1, Soil Sci. Soc. Am. J., 7, 48–55, 1943.
Soil Survey Staff: Keys to soil taxonomy, A basic system of soil classification for making and interpreting soil surveys, 2nd edn., Agricultural Handbook 436, Natural Resources Conservation Service, USDA, Washington, D.C., USA, 869 pp., 1999.
Soil Survey Staff: Keys to Soil Taxonomy, 12th edn., USDA-Natural Resources Conservation Service, Washington, D.C., USA, 2014.
Sonneveld, M. P. W., Bouma, J., and Veldkamp, A.: Refining soil survey information for a Dutch soil series using land use history, Soil Use Manage., 18, 157–163, 2002.
Van Genuchten, M. T.: A closed-form equation for predicting the hydraulic conductivity of unsaturated soils, Soil Sci. Soc. Am. J., 44, 892–898, 1980.
van Ittersum, M. K., Cassman, K. G., Grassini, P., Wolf, J., Tittonell, P., and Hochman, Z.: Yield gap analysis with local to global relevance a review, F. Crop. Res., 143, 4–17, 2013.
Van Looy, K., Bouma, J., Herbst, M., Koestel, J., Minasny, B., Mishra, U., Montzka, C., Nemes, A., Pachepsky, Y., Padarian, J., Schaap, M. G., Tóth, B., Verhoef, A., Vanderborght, J., van der Ploeg, M. J., Weihermüller, L., Zacharias, S., Zhang, Y., and Vereecken, H.: Pedotransfer functions in Earth system science: challenges and perspectives, Rev. Geophys., 55, 1199–1256, https://doi.org/10.1002/2017RG000581, 2017.
Van Oort, P. A. J., Saito, K., Dieng, I., Grassini, P., Cassman, K. G., and Van Ittersum, M. K.: Can yield gap analysis be used to inform R&D prioritisation?, Glob. Food Secur., 12, 109–118, 2017.
Wade, J., Culman, S. W., Hurisso, T. T., Miller, R. O., Baker, L., and Horwath, W. R.: Sources of variability that compromise mineralizable carbon as a soil health indicator, Soil Sci. Soc. Am. J., 82, 243–252, 2018.
Wösten, J. H., Lilly, A., Nemes, A., and Le Bas, C.: Development and use of a database of hydraulic properties of European soils, Geoderma, 90, 169–185, https://doi.org/10.1016/S0016-7061(98)00132-3, 1999.
Zollo, A. L., Turco, M., and Mercogliano, P.: Assessment of hybrid downscaling techniques for precipitation over the Po river basin, in: Engineering Geology for Society and Territory, vol. 1, edited by: Lollino, G., Manconi, A., Clague, J., Shan, W., and Chiarle, M., Springer, Cham, Switzerland, 2015.