the Creative Commons Attribution 4.0 License.
the Creative Commons Attribution 4.0 License.
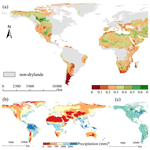
Advancing studies on global biocrust distribution
Siqing Wang
Li Ma
Liping Yang
Yali Ma
Yafeng Zhang
Changming Zhao
Biological soil crusts (biocrusts hereafter) cover a substantial proportion of the dryland ecosystem and play crucial roles in ecological processes such as biogeochemical cycles, water distribution, and soil erosion. Consequently, studying the spatial distribution of biocrusts holds great significance for drylands, especially on a global scale, but it remains limited. This study aimed to simulate global-scale investigations of biocrust distribution by introducing three major approaches, namely spectral characterization indices, dynamic vegetation models, and geospatial models, while discussing their applicability. We then summarized the present understanding of the factors influencing biocrust distribution. Finally, to further advance this field, we proposed several potential research topics and directions, including the development of a standardized biocrust database, enhancement of non-vascular vegetation dynamic models, integration of multi-sensor monitoring, extensive use of machine learning, and a focus on regional research co-development. This work will significantly contribute to mapping the biocrust distribution and thereby advance our understanding of dryland ecosystem management and restoration.
- Article
(4470 KB) - Full-text XML
-
Supplement
(405 KB) - BibTeX
- EndNote
Biological soil crusts (biocrusts hereafter) are continuous biotic complexes that live in the topsoil, formed by different proportions of photosynthetic autotrophic (e.g., cyanobacteria, algae, lichens, mosses) and heterotrophic (e.g., bacteria, fungi, archaea) organisms colloidal with soil particles, usually with a thickness of a few millimeters to a few centimeters (Weber et al., 2022). Biocrusts occupy a wide range of ecological niches in mid-latitudes and in polar and alpine regions, covering approximately 11 % of the global land area (Porada et al., 2019). In particular, biocrusts are well-adapted to water-limited, nutrient-poor, and hostile environments, such as arid and semi-arid areas characterized by low ratios of precipitation to potential evaporation (0.05–0.5) (Pravalie, 2016; Read et al., 2014; Weber et al., 2016).
As vital components of dryland ecosystems, biocrusts fulfill many essential ecological functions. They contribute to stabilizing the soil surface, improving soil permeability, and enhancing water-holding capacity within the upper few centimeters of soil (Sun et al., 2023; Shi et al., 2023; Gao et al., 2017). By participating in various biogeochemical cycles, biocrusts were estimated to contribute to 15 % of terrestrial net primary productivity and 40 %–85 % of biological nitrogen fixation (Elbert et al., 2012; Rodriguez-Caballero et al., 2018). They also impact ecohydrological processes by altering soil microclimate and redistributing soil water (Kidron et al., 2022; Tucker et al., 2017). Moreover, biocrusts influence seed capture and soil seed banks (Kropfl et al., 2022), thereby mediating plant growth and community assembly (Havrilla and Barger, 2018; Song et al., 2022). The extent and magnitude of these ecological functions and services depend on the spatial distribution of biocrusts. Therefore, it is crucial to understand their distribution.
Despite the significance of biocrusts, previous studies have primarily focused on their contributions to carbon and nitrogen cycling across various habitats and climates (Hu et al., 2019; Morillas and Gallardo, 2015), as well as to interspecific interactions and biocrust biodiversity (Machado de Lima et al., 2021; Munoz-Martin et al., 2019), rather than their spatial distribution. Countries like China, the United States, Spain, Australia, and Israel, most of which have extensive dryland areas, have attempted to make breakthroughs on this issue (Fig. 1a). However, other dryland countries and regions, such as central and southern Africa, where the biocrust distribution has been reported, still suffer from a paucity of studies and data on biocrusts (Fig. 1b; Rodriguez-Caballero et al., 2022b). This geographical imbalance in biocrust distribution studies has resulted in most knowledge remaining at local to regional scales, with very limited discoveries on a global scale (Maestre et al., 2021).
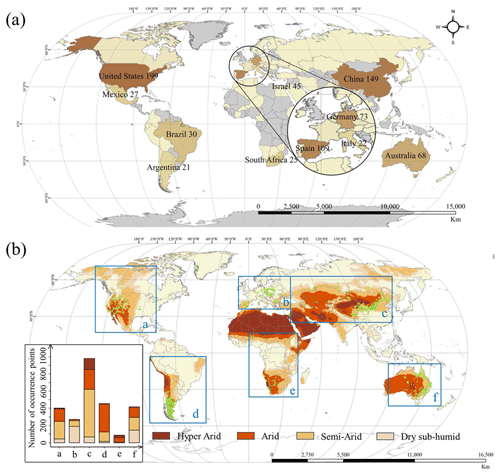
Figure 1Literature review of biocrust distribution studies. (a) Map of hotspot countries for biocrust distribution research. Numbers represent the publication count by authors from different countries from 1990 to 2022, and the top 12 countries are shown. The database is Web of Science, TS = (”biogenic crust*” OR ”biological crust*” OR ”biological soil crust*” OR ”biocrust*” OR ”microphytic crust*” OR ”microbiotic crust*” OR ”cyanobacterial*” OR ”algal*” OR ”lichen*” OR ”moss*” OR ”biotic crust*”) AND (”mapping*” OR ”distribution*” OR “ spatial pattern*”) AND (”dryland” OR ”hyper*arid*” OR ”arid*” OR ”semi*arid*” OR ”dry subhumid*”), with research interests in environmental sciences and/or ecology and a total of 700 papers. (b) Global biocrust data distribution, based on field surveys and literature compilation. The bar chart counts the number of entries for biocrust records (presence/absence or cover) for six continents (regions). Datasets have been collected and expanded from the published database (Chen et al., 2020; Rodriguez-Caballero et al., 2018) to 3848 items, Ning Chen et al. (unpublished data).
In this study, we aimed to sort out and advance the understanding of biocrust distribution from three perspectives: the applicability and comparison of research methods (Sect. 2), clarification of factors influencing biocrust distribution (Sect. 3), and challenges and strategies for future studies on biocrust distribution (Sect. 4). This work is expected to deepen our understanding of dryland ecosystem processes and to provide a scientific basis for conserving dryland ecosystems and their responses to global change.
Three methods are commonly used to study biocrust distribution: spectral characterization, vegetation dynamic modeling, and geospatial modeling. This section provides an overview of these methods, including their basic principles, case studies, adaptability, and limitations.
2.1 Spectral characterization index
With advances in remote sensing and geo-information technology, spectroscopy offers a feasible method of characterizing distribution features from a physical point of view. Differences in absorption or reflection of specific wavelengths by different ground covers can effectively identify soil surface objects (Rodriguez-Caballero et al., 2015). By identifying biocrust-specific bands from reflectance spectral images (Karnieli et al., 1999), it is possible to construct a presence-absence map of biocrust distribution (Fig. 2a).
Currently, spectral characterization indices have been widely applied in many areas of drylands. For example, cyanobacterial biocrusts are widely distributed in the Sahara region of Africa (Beaugendre et al., 2017) and the Negev Desert of Israel (Panigada et al., 2019); for the latter, studies invented the biocrust index (CI) based on remotely sensed imagery to access the characteristics of localized changes in biocrust distribution over 31 years (Karnieli, 1997; Noy et al., 2021). Sun et al. (2024) developed the fraction biocrust cover index (FBCI) based on radiative transfer and mapped biocrust distribution over a desert area at 10 m resolution, showing well-matched results between the model and field observations (RMSE of 0.0774, systematic deviation of −4.05 %). In the Gurbantünggüt Desert, a study constructed the biological soil crust index (BSCI), with lichen biocrust as the dominant group, and mapped the distribution of biocrusts with high accuracy (accuracy of 94.7 %, kappa coefficient of 0.82) (Chen et al., 2005); spatially, biocrusts cover 28.7 % of the area, with a high and uniform cover in the southern part of the desert and a scattered distribution in other regions (Zhang et al., 2007). In the Loess Plateau, red–green–blue (RGB) image-based biocrust monitoring showed that variability in biocrust cover decreased logarithmically with increasing plot size until a critical size of 1 m2, after which biocrust cover remained approximately constant (S. Wang et al., 2022).
For the spectral characterization method, it is critical to determine the threshold of spectral bands that represent biocrusts. For instance, at an aerosol optical depth of 0.2, the BSCI ranges from 4.13 to 6.23 and narrows to 4.58–5.69 with increasingly poor atmospheric conditions. Overly strict or loose threshold ranges can easily lead to biocrust omission or misidentification. To improve the accuracy of biocrust identification, some researchers utilized the hyperspectral sensor's continuous waveband capabilities and created the Continuum Removal Crust Identification Algorithm (CRCIA) (Chamizo et al., 2012b; Weber et al., 2008). Baxter et al. (2021), innovatively applying the random forest algorithm to spectral feature classification and achieving an accuracy of 78.5 % in biocrust recognition. Additionally, two other indices, the sandy land ratio crust index (SRCI) and the desert ratio crust index (DRCI), were introduced to account for differences between sandy land (fractional vegetation cover, FVC, < 20 %) and desert environments, improving mapping accuracy by approximately 6 % (Z. Wang et al., 2022).
The spectral characterization method is easy to use and, thus, facilitates access to continuous long-term dynamics of biocrust distribution. However, mosses and vascular plants are generally mixed up in this method because their reflectance characteristics are similar across all wavelengths, especially when mosses are wet, which makes them indistinguishable (Fang et al., 2015). Therefore, the spectral characterization method mainly applies to situations where biocrust cover is greater than 30 % and where plant cover is less than 10 % (Beaugendre et al., 2017). It should be noted that the existing indexes mostly correspond to biocrust cover consisting of specific dominant groups in specific environments, which cannot be directly extrapolated to areas with highly heterogeneous environments (Table 1). Wetting or disturbance may also lead to large fluctuations in the reflectance of different land types, interfering with biocrust distribution monitoring (Rodriguez-Caballero et al., 2015; Weber and Hill, 2016).
2.2 Dynamic global vegetation models (DGVMs)
Dynamic global vegetation models (DGVMs) are another major method for estimating vegetation cover (Deng et al., 2022). These models mainly focus on simulating the biogeochemical processes (e.g., carbon and water cycles) and the metabolic and hydrological processes of organisms (Fig. 2b) (Lenton et al., 2016; Porada et al., 2017). DGVMs have significant advantages in mapping biocrust distribution because their assumptions have clear biological implications (Cuddington et al., 2013). Porada et al. (2013) focused on CO2 diffusion rates and photosynthetic processes under dynamic water content saturation in dryland biocrusts. By parameterizing long-term climate data and disturbance intervals and averaging simulation results for the past 20 years for each grid point, they estimated that biocrusts cover 11 % of the global terrestrial land surface (Fig. 3a) (Porada et al., 2019). Specifically, the light and dark cyanobacteria were widely distributed in deserts, savannas, grasslands, and Mediterranean woodlands at low latitudes, with their presence increasing to some extent with increasing dryness. In contrast, mosses were mainly distributed in middle and high latitudes and polar regions.
Dynamic vegetation models can be combined with cross-scale remotely sensed data to quantify the geographic distribution and biogeochemical effects of plants, replacing traditional measurements. However, the uneven distribution density of biocrust data points along the aridity gradient or a small amount of data may lead to poor prediction of global-scale distributions (Quillet et al., 2010). So far, non-vascular vegetation has not received enough attention, and only the lichen and bryophyte model (LiBry) used in the above case is uniquely suited to emulating biocrust distribution (Porada et al., 2019, 2013). The LiBry model includes variations in biocrust cover strategy under disturbance and its growth, but it relies heavily on subjective experience and model parameterization, which is still immature compared to dynamic models of vascular vegetation.
2.3 Geospatial models
Directly relating vegetation presence or cover to environmental data, instead of indirectly via biological processes, is another important way to obtain biocrust distribution (Beaugendre et al., 2017; Fischer and Subbotina, 2014; Skidmore et al., 2011). Classic statistical models can serve this purpose. However, they still require comprehensive expert knowledge on how environmental factors affect biocrusts (Pearce et al., 2001), which is hard to obtain and prone to bias. Geospatial models, which integrate machine learning tools with field survey data and remote sensing data, hold the most promise (Fig. 2c) (Crego et al., 2022). They are also known as species distribution models or ecological niche models (Brown and Anderson, 2014; Jiménez-Valverde et al., 2008; Soberon and Nakamura, 2009). At the global scale, there has been only one study that predicted biocrust distribution patterns using geospatial modeling (Rodriguez-Caballero et al., 2018); this study found that biocrust covers 12.2 % of the global land surface area, which is about 1.79 × 107 km2 (Fig. 3b).
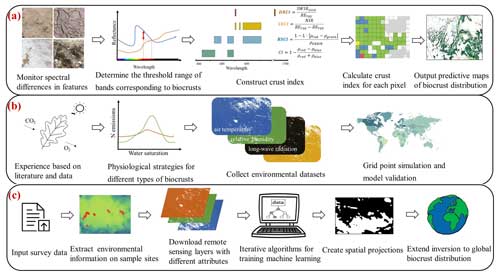
Figure 2Summary of three major approaches to studying biocrust distribution. Workflow of applying spectral characterization method (a), dynamic vegetation model (b), and geospatial model (c) in biocrust distribution studies. See the main text for a more detailed introduction to these methods.
Compared with the results of the dynamic vegetation model, the simulation accuracy (R2∼0.8) and mapping resolution (0.5° × 0.5°) of the geospatial model were improved. Biocrust distribution is generally consistent in the large deserts of Asia, western America, Europe, and Oceania, while some semi-arid regions, such as the northern and southern margins of the African Sahara Desert, South Asia, and central North America, have significantly higher biocrust cover in the projection by Rodriguez-Caballero et al. (2018). We estimate that this may be because geospatial modeling focuses more on the influence of climate as the Mediterranean climate and tropical desert climate in the Sahara Desert, as well as the tropical desert climate of northwestern South Asia, are suitable for biocrust survival. Additionally, the large number and high cover of biocrust training sets in central North America could have contributed to the generally high predicted cover in machine learning.
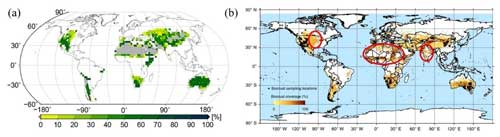
Figure 3Maps of global biocrust distribution. (a) Prediction based on vegetation dynamic model (Porada et al., 2019). (b) Prediction based on geospatial model (Rodriguez-Caballero et al., 2018). Permissions have been obtained from the relevant sources: Porada et al. (2019) and Rodriguez-Caballero et al. (2018).
As black boxes, geospatial models are largely non-interpretable and, thus, less capable of capturing the key mechanisms behind phenomena, which may limit their applications. Under this methodological framework, only the direct effects of various environmental indicators are considered. For example, it focuses on the direct effect of precipitation on biocrust distribution while ignoring the indirect effects, such as interactions among shrubs, grasses, and biocrusts (Wang et al., 2024). In addition, to avoid confounding model predictions, the inclusion of environmental factors should be based on their relevance to biocrusts, and expert knowledge should still be needed to a certain degree (Mäkinen et al., 2022). One should consider not only natural conditions such as climate, topography, and soil but also data on human activities such as afforestation, trampling, and population density to be environmental indicators in the model. It should be noted that the superimposition of environmental layers of different resolutions may cause deviations in results to some extent, which is unavoidable (Zhao et al., 2024). Despite the above limitations of geospatial modeling, with sufficient computing power, observation data regarding biocrust distribution, and suitable environmental information, geospatial models are supposed to be relatively optimal solutions for predicting biocrust distributions (Table 1).
It is of great importance to clarify the environmental variables associated with biocrust distribution. On the one hand, it helps to frame the range of data selection before modeling, and on the other hand, it aids in identifying patterns of biocrust distribution in the context of dynamic changes and various types of environmental information, thereby facilitating the prediction of distribution evolution on longer timescales. Numerous modeling studies (Kidron and Xiao, 2023; Li et al., 2023; Rodriguez-Caballero et al., 2018) have demonstrated that, on the global scale, biocrust distribution is mainly influenced by water conditions, temperature, soil properties, fire, and disturbance (Bowker et al., 2016).
With regard to water conditions, in general, total precipitation (Fig. 4b) is considered to be critical in determining the distribution of biocrusts (Eldridge and Tozer, 1997). Increased precipitation can lead to higher levels of lichen and moss cover, while algal cover may initially increase and then decrease (Budel et al., 2009; Marsh et al., 2006; Zhao et al., 2014). It should be noted that precipitation can also promote the growth of vascular plants, and continuous high cover of vascular plants and litterfall will limit the space available to biocrusts (Bowker et al., 2005). In addition to the total amount of precipitation, the seasonality and frequency of precipitation cannot be ignored (Budel et al., 2009). Winter precipitation and/or smaller rain events benefit biocrusts, especially when mean annual precipitation is less than 500 mm. Meanwhile, a high frequency of precipitation can lead to the dominance of biocrusts over vascular plants (Chamizo et al., 2016; Jia et al., 2019). Experimental evidence shows that precipitation events of 5 mm are able to maintain the normal physiological and ecological functions of the biocrust on the Colorado Plateau, USA, while ever lower precipitation events of 1.2 mm can rapidly kill moss biocrust (Reed et al., 2012). Non-precipitation water input is another important water resource type. The Namib Desert receives little rainfall, but lichens and moss biocrusts can reach a relatively high cover (∼ 70 %) (Budel et al., 2009). This is because local water vapor tends to condense into fog or dew, which facilitates the survival of three-dimensional species (such as leafy lichens) by trapping air moisture (Eldridge et al., 2020; Kidron, 2019; Li et al., 2021). Similarly, lichen biocrusts are widely distributed in the western US along the Mexican coast due to the high air humidity (dew formation for almost one-third of the year) (McCune et al., 2022; Miranda-González and McCune, 2020).
When it comes to temperature, relatively high soil temperature can create an environment of high evaporation that impedes biocrust colonization (Garcia-Pichel et al., 2013). Regarding air temperature, warming by 4 °C could alter biocrust community structure, resulting in a sharp decrease in moss biocrust cover and an increase in cyanobacterial biocrust cover. This effect becomes even more significant when warming interacts with time and precipitation treatments (Ferrenberg et al., 2015). Recent studies have shown that historical and future temperature changes also affect biocrust distribution. For example, the climate legacy over the last 20 000 years could indirectly affect the distribution and relative species richness of biocrusts by altering vegetation cover and soil pH (Eldridge and Delgado-Baquerizo, 2019). Additionally, under future scenarios of increased temperature and aridity, biocrust cover is predicted to decrease by approximately 25 % by the end of the century, with communities shifting towards early cyanobacterial biocrusts (Rodríguez-Caballero et al., 2022).
With regard to soil properties, it was commonly believed that finer soils benefit biocrust growth (Belnap et al., 2014; Williams et al., 2013). However, some scientists have challenged this notion (Fig. 4c). For example, Kidron (2018) argued that soils with high dust or fine grains are not a necessary condition for biocrust distribution. Qiu et al. (2023) suggested that soils with small amounts of gravel (0.04 %–22.34 % content, 0.58 % being optimal) are more favorable for biocrusts. Another study has shown that the soil parent material determines the degree of surface weathering and the water-holding capacity of the soil, thus indirectly influencing the distribution of biocrusts (Bowker and Belnap, 2008). Gypsum or calcareous soils tend to develop mosses and lichens (Elbert et al., 2012), while sandy soils tend to develop cyanobacteria (Root and McCune, 2012).
In terms of fire, the grassland is a major life form in dryland ecosystems, making it crucial to explore the effects of fire events on biocrust distribution (Palmer et al., 2022). Fire-induced soil warming can alter the resource allocation and dynamic growth mechanisms between biocrusts and vascular plants (McCann et al., 2021), potentially leading to a reduction in species richness and cover of biocrusts, especially cyanobacteria and algae (Abella et al., 2020; Palmer et al., 2020). Condon and Pyke (2018) showed that moss cover increases with time after fire, with no significant change in lichen cover.
With regard to disturbance, activities such as grazing, agricultural practices, and land development can significantly impact biocrust distribution. Studies have demonstrated that grazing intensity can lead to substantial changes in biocrust cover. For instance, in Patagonian rangelands, biocrust cover decreased by 85 %, 89 %, and 98 % under light, medium, and heavy grazing, respectively (Velasco Ayuso et al., 2019). In the Loess Plateau, total biocrust cover remained almost unchanged under light grazing (<30.00 goat dung m−2), but there were variations in community structure, with an increase in cyanobacteria biocrusts (23.1 %) and a decrease in moss biocrusts (42.2 %) due to a reduction in vascular plant cover (Ma et al., 2023). Tillage practices can disrupt the soil surface, leading to a reduction in biocrust cover (6 % on average) and diversity, with lichens struggling to survive in tilled fields compared to mosses (Durham et al., 2018). Additionally, late-successional biocrusts exhibit higher tolerance compared to pre-successional biocrusts. Moss biocrusts, for instance, can maintain soil microbial biomass and nematode abundance better under trampling disturbance compared to cyanobacteria and lichen biocrusts (Yang et al., 2018). However, contrarily to this view, it has been observed that cyanobacterial biocrusts increased in terms of cover from 81 % to 99 % after trampling, while lichen and moss biocrusts decreased from 1.5 % and 18 % to less than 0.5 %. Furthermore, mining activities can significantly reduce the photosynthetic potential of biocrusts, particularly affecting the recovery of cyanobacterial biocrusts (Gabay et al., 2022).
There are other factors to consider. On a global scale, biocrust distribution is also closely linked to biogeographic isolation. Strong spatial heterogeneity, accompanied by spatial distance, can create barriers to the dispersal of propagules (spores, fungal bodies), which indirectly impedes colonization of the biocrusts (Garcia-Pichel et al., 2013). In addition, factors such as vascular plant cover, topography, and solar radiation also influence biocrust distribution, though to a lesser extent than the factors mentioned above. For further insights, readers are encouraged to consult Chap. 10 of Biological Soil Crusts: An Organizing Principle in Drylands, which provides an overview of the control and distribution patterns of biocrusts from micro to global scales (Bowker et al., 2016).
To sum up, climate is the most important factor influencing global biocrust distribution, especially in drylands where water is precious to the organisms. However, exploration of the roles of climatic factors such as rainfall seasonality and atmospheric drought still needs much further effort (Wright and Collins, 2024), especially in the context of global climate change. Although more attention has been paid to the physical properties of soils, the roles of their chemical properties, such as the nitrogen (N) and phosphorus (P) content, need to be taken more seriously. Fire and disturbance are usually ignored. However, due to the trend towards warmer and drier environments, as well as an increasing population and the need to sustain livelihoods, their influences on biocrust distribution may become more important. As one of the basic processes on a global scale, biogeographic isolation or changes in land use should be paid more attention. With the increasing number of biocrust data points, we can expect that this aspect will see a surge in research.
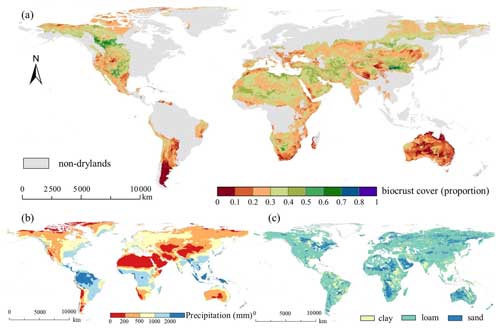
Figure 4Biocrust distribution and its critical influencing factors. (a) Biocrust cover map and its influencing factors. (a) Global biocrust distribution by random forest modeling. Based on a global biocrust database constructed by Ning Chen et al. (unpublished data), we expanded the biocrust data to 3848 entries through literature compilation and field surveys and fitted them with four types of remotely sensed environmental data, including climate, land use, soil properties, and elevation, to finally predict the suitable areas for the biocrust distribution and to quantify the biocrust cover. (b) Global average annual precipitation (1970–2020) – data from the WorldClim database (version 2.1). (c) Global soil texture distribution – data from HWSD (Harmonized World Soil Database, version 1.2). Precipitation and soil texture were taken as examples of environmental factors.
Biocrusts play a crucial role in dryland ecosystems, making it essential to understand their current status and distribution dynamics. For influencing factors (Sect. 3), traditional observational studies and controlled experiments offer multiple perspectives of foundational knowledge. For assessing biocrust distribution patterns (Sect. 2), the methods shift from traditional approaches to spectral index, vegetation dynamics, and geospatial models that span multiple subjects like ecology, biology, geology, and computer science. However, high-precision biocrust distribution data across geographic units remain scarce, and current research methods are still limited. To further advance studies of biocrust distribution, we propose the following aspects for consideration.
4.1 Building standardized biocrusts database
Currently, biocrust data are fragmented, low in volume, and derived from narrow sources, largely limiting spatial prediction from points to broader areas. Thus, we suggest that there be a global effort to build a standardized and specialized biocrust database. This database should include consistent data items (such as the main types and cover of biocrusts, latitude, longitude, and cover) and adhere to uniform inclusion criteria. Such a database is an important infrastructure for mapping global biocrust distribution, serving as the benchmark for training and validating spectral characteristics, DGVMs, and geospatial models (Engel et al., 2023). Given the difficulty of conducting field surveys worldwide, compiling biocrust data from the published literature or other sources would be a primary approach (Fig. 4a). To date, several published studies have assembled 900–1000 data points on biocrust presence or absence from the literature (including 584 data points on biocrust cover) (Chen et al., 2020; Eldridge et al., 2020; Havrilla et al., 2019; Rodriguez-Caballero et al., 2018). However, compiling from the literature has large narrowness and is still far from building a standardized and specialized biocrust database. While open databases are not specialized in relation to biocrusts, some of them may provide valuable additions (Fig. 5). For instance, the biodiversity and specimen datasets such as GBIF and the Atlas of Living Australia (Belbin and Williams, 2015; García-Roselló et al., 2015) contain a large amount of information on species, including mosses and lichens (Table 2), potentially offering hundreds or even thousands of entries of biocrust occurrence or cover. Similarly, global, national, and regional plant flora can significantly contribute to building the standardized and specialized biocrust database. For example, sPlot includes ∼ 2 million vegetation plot data points (Sabatini et al., 2021), and the European Vegetation Archive (EVA) also holds 1.6 million entries over the globe (Chytrý et al., 2016). Regional datasets like the Environmental Monitoring of Arid and Semiarid Regions (MARAS) have surveyed 426 sites (up to September 2020) and provide regular access to 624.50 km2 of rangeland vegetation spatial patterns, species diversity, soil functional indices, climatic data, and landscape photographs in the Patagonia region of Argentina and Chile (Oliva et al., 2020). Concerns about land use products are also necessary. Global land use maps, based on the PROBA-V sensor, which contain spatial information for the moss and lichen layer, have an annual update frequency and a resolution of 100 m. Additionally, an increasing number of amateurs contribute significantly to global species information entries through species identification apps, which are user-friendly and widely accessible. The citizen science project iNaturalist is a very good example (Wolf et al., 2022). Furthermore, when collecting and collating data from non-academic sources, the combination of web crawlers and text analysis can help in obtaining biocrust data and addressing key ecological issues.
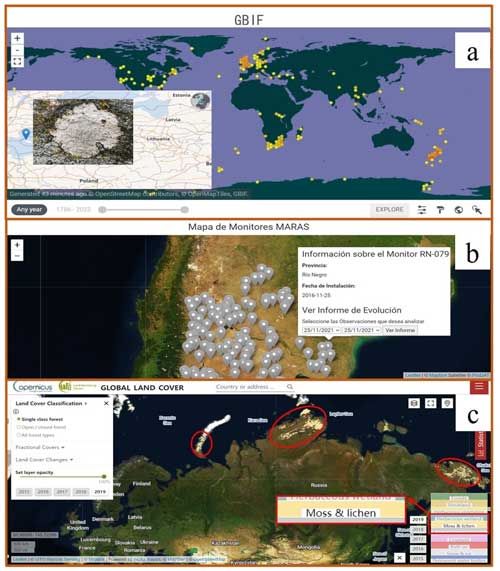
Figure 5Potential approaches to building a standardized biocrust database. (a) Distribution of lichens in the GBIF database with an example photo; (b) environmental-monitor distribution map of MARAS database; (c) distribution of mosses and lichens in the PROBAV_LC100 database (light-yellow area) in northern Asia, for instance.
4.2 Improving non-vascular vegetation dynamic models
There are only two DGVMs applicable to non-vascular organisms – LiBry and ECHAM6-HAM2-BIOCRUST (Rodriguez-Caballero et al., 2022a). Despite their utility, these models still require performance improvements. Future directions for enhancing these models could include incorporating spatial self-organization of non-vascular organisms (Gassmann et al., 2000), the effects of fire (Thonicke et al., 2001), vegetation–environment feedback processes (Quillet et al., 2010), functional traits (Boulangeat et al., 2012), intraspecific–interspecific interactions (Boulangeat et al., 2014), and seasonal dynamics. Moreover, the physical properties, photosynthetic capacity, and carbon and nitrogen allocation of biocrusts change along environmental gradients in complex and context-dependent ways. These factors should be incorporated into DGVMs (Fatichi et al., 2019). Spatially explicit DGVMs may be one key to effectively improving the accuracy of simulations in future studies, although they are data-intensive. Also, biocrusts are significantly influenced by hydrological processes and, in turn, affect these processes (Chen et al., 2018; Whitney et al., 2017). However, ecohydrological models, which focus on hydrological processes, are rarely connected to global biocrust distribution predictions. Jia et al. (2019) attempted to incorporate biocrust cover as a system state variable in an ecohydrological model, investigating biocrust cover under varying rainfall gradients. By feeding ecohydrological models with global environmental data, particularly hydrological variables, these models could offer a new approach to predicting biocrust distribution on a global scale.
4.3 Integrated application of high-quality sensors
The spectral characterization method lies in the differences in the spectral reflectance of biocrusts and other land types at various wavelengths. Consequently, the accuracy of the results is contingent on the quality of the sensors used. Previous studies often employed a single sensor with fixed band intervals for distinguishing biocrusts, potentially missing critical spectral features of different land types (Chamizo et al., 2012a). If the biocrust index can be constructed by combining and comparing the full-band spectral data from multiple terrestrial sensors, infrared cameras, and other devices, the errors will be reduced to a certain extent, thus improving the classification accuracy (Z. Wang et al., 2022). In addition, the unique advantages of hyperspectral data, which include large data volumes and narrow bands, allow for the development of new biocrust discrimination standards when combined with observational data. If further estimation of biocrust cover can be achieved on this basis, it will be a significant contribution to the study of large-scale biocrust distribution (Rodríguez-Caballero et al., 2017). To date, high-resolution sensors have proven to be successful in monitoring lichens and mosses (Blanco-Sacristan et al., 2021), and the release of such products is something important to look out for in the future.
4.4 Making full use of machine learning
Machine learning can be combined with remote sensing products to uncover complex features from big data, enabling the prediction of global biocrust distribution (Collier et al., 2022). This data-driven approach has powerful predictive capabilities, especially for mapping species distribution, and can largely avoid the errors of missing or misidentifying biocrusts caused by traditional methods (relying on field measurements to determine threshold ranges) (Z. Wang et al., 2022). In remote sensing image classification, mature machine learning algorithms include support vector machines, single decision trees, random forests, artificial neural networks, etc. (Yu et al., 2020). Ensemble models combining multiple algorithms have been widely used in the field of species distribution but have seen relatively few applications in biocrust prediction. In the future, using machine learning to identify parameters for dynamic models of biocrusts may be one of the most promising methods to predict biocrust distribution (Perry et al., 2022).
4.5 Regional research synergy development
Research on biocrust distribution has shown significant spatial and climatic imbalances. The areas that have been studied are relatively concentrated in countries such as China, the United States, Spain, Australia, and Israel. Although there are large areas of dryland distributed in Africa (other than South Africa), central Asia, central South America, and northern North America, research on biocrusts in these regions is scarce. These unbalanced regional research efforts constrain the advancement of studies on global biocrust distribution. Therefore, how to coordinate and promote the common progress of regional research is an urgent issue at present. Climatically, in addition to the drylands, the cold zones may be another important area to explore biocrust distribution (Pushkareva et al., 2016). On the Tibetan Plateau, studies have investigated the spatial variations of different types of biocrust communities across climatic gradients and their effects on soil temperature features and freezing duration (Ming et al., 2022; Wei et al., 2022). These findings highlight the need for more studies on biocrust distribution in the alpine areas.
Biocrusts are of great significance to the ecohydrological processes, soil material cycling, landscape shaping, and biodiversity conservation in drylands. To date, numerous studies have tried to fill the knowledge gap with regard to biocrust distribution at the regional scale. However, global-scale research remains scarce, and mapping accuracy is still insufficient, directly leading to ambiguities in ecological function assessment and prediction. Therefore, advancing global-scale biocrust distribution research requires a more comprehensive consideration of the applicability of previous methods and a broader knowledge base to help select environmental indicators. For future work in this field, we advocate for closer cooperation among scientists to build a global standardized database incorporating multiple sources of biocrust data. This effort should primarily focus on expanding biocrust data items in understudied regions where biocrusts have been reported, thereby creating a larger, multi-habitat training set. Meanwhile, modern learning tools, such as deep learning, should be broadly applied to high-quality sensor image segmentation, data classification, and model parameter tuning. Finally, long-term monitoring and simulation are necessary to better understand the dynamics of ecological restoration in drylands and the response of biocrusts to environmental changes.
The biocrust database constructed by Ning Chen et al. (unpublished data) is available in the Supplement.
The supplement related to this article is available online at: https://doi.org/10.5194/soil-10-763-2024-supplement.
SW co-conceived the idea, collected data on the biological soil crust, wrote the first draft, prepared the figures, and revised the paper. LM, LY, YM, and YZ collected data on biological soil crusts and revised the paper. CZ co-conceived the idea. NC co-conceived the idea, collected data on the biological soil crust, revised the paper, and provided support for funding.
The contact author has declared that none of the authors has any competing interests.
Publisher's note: Copernicus Publications remains neutral with regard to jurisdictional claims made in the text, published maps, institutional affiliations, or any other geographical representation in this paper. While Copernicus Publications makes every effort to include appropriate place names, the final responsibility lies with the authors.
We are grateful to Zhiguang Zhao, Lixun Zhang, and Chao Guan for their helpful discussions while preparing the first draft.
The study was supported by the National Natural Science Foundation of China (grant nos. 32271620 and 31971452), the National Key Research and Development Program of China (grant no. 2023YFF0805603), and the Central University Basic Research Funds (grant nos. lzujbky-2021-ey16 and lzujbky-2023-eyt01).
This paper was edited by Elizabeth Bach and reviewed by Eva Dettweiler-Robinson, Brianne Palmer, and one anonymous referee.
Abella, S. R., Gentilcore, D. M., and Chiquoine, L. P.: Resilience and alternative stable states after desert wildfires, Ecol. Monogr., 91, 1–19, https://doi.org/10.1002/ecm.1432, 2020.
Baxter, C., Mallen-Cooper, M., Lyons, M. B., and Cornwell, W. K.: Measuring reflectance of tiny organisms: The promise of species level biocrust remote sensing, Meth. Ecol. Evol., 12, 2174–2183, https://doi.org/10.1111/2041-210x.13690, 2021.
Beaugendre, N., Malam Issa, O., Choné, A., Cerdan, O., Desprats, J.-F., Rajot, J. L., Sannier, C., and Valentin, C.: Developing a predictive environment-based model for mapping biological soil crust patterns at the local scale in the Sahel, Catena, 158, 250–265, https://doi.org/10.1016/j.catena.2017.06.010, 2017.
Belbin, L. and Williams, K. J.: Towards a national bio-environmental data facility: experiences from the Atlas of Living Australia, Int. J. Geogr. Inf. Sci., 30, 108–125, https://doi.org/10.1080/13658816.2015.1077962, 2015.
Belnap, J., Miller, D. M., Bedford, D. R., and Phillips, S. L.: Pedological and geological relationships with soil lichen and moss distribution in the eastern Mojave Desert, CA, USA, J. Arid Environ., 106, 45–57, https://doi.org/10.1016/j.jaridenv.2014.02.007, 2014.
Blanco-Sacristan, J., Panigada, C., Gentili, R., Tagliabue, G., Garzonio, R., Martin, M. P., Ladron de Guevara, M., Colombo, R., Dowling, T. P. F., and Rossini, M.: UAV RGB, thermal infrared and multispectral imagery used to investigate the control of terrain on the spatial distribution of dryland biocrust, Earth Surf. Proc. Land., 46, 2466–2484, https://doi.org/10.1002/esp.5189, 2021.
Boulangeat, I., Philippe, P., Abdulhak, S., Douzet, R., Garraud, L., Lavergne, S., Lavorel, S., van Es, J., Vittoz, P., and Thuiller, W.: Improving plant functional groups for dynamic models of biodiversity: at the crossroads between functional and community ecology, Glob. Change Biol., 18, 3464–3475, https://doi.org/10.1111/j.1365-2486.2012.02783.x, 2012.
Boulangeat, I., Georges, D., and Thuiller, W.: FATE-HD: a spatially and temporally explicit integrated model for predicting vegetation structure and diversity at regional scale, Glob. Change Biol., 20, 2368–2378, https://doi.org/10.1111/gcb.12466, 2014.
Bowker, M. A. and Belnap, J.: A simple classification of soil types as habitats of biological soil crusts on the Colorado Plateau, USA, J. Veg. Sci., 19, 831–840, https://doi.org/10.3170/2008-8-18454, 2008.
Bowker, M. A., Belnap, J., Davidson, D. W., and Phillips, S. L.: Evidence for Micronutrient Limitation of Biological Soil Crusts: Importance to Arid-Lands Restoration, Ecol. Appl., 15, 1941–1951, https://doi.org/10.1890/04-1959, 2005.
Bowker, M. A., Belnap, J., Büdel, B., Sannier, C., Pietrasiak, N., Eldridge, D. J., and Rivera-Aguilar, V.: Controls on Distribution Patterns of Biological Soil Crusts at Micro- to Global Scales, in: Biological Soil Crusts: An Organizing Principle in Drylands, edited by: Weber, B., Büdel, B., and Belnap, J., Springer International Publishing, Cham, 173–197, https://doi.org/10.1007/978-3-319-30214-0_10, 2016.
Brown, J. L. and Anderson, B.: SDMtoolbox: a python-based GIS toolkit for landscape genetic, biogeographic and species distribution model analyses, Meth. Ecol. Evol., 5, 694–700, https://doi.org/10.1111/2041-210x.12200, 2014.
Budel, B., Darienko, T., Deutschewitz, K., Dojani, S., Friedl, T., Mohr, K. I., Salisch, M., Reisser, W., and Weber, B.: Southern African biological soil crusts are ubiquitous and highly diverse in drylands, being restricted by rainfall frequency, Microb. Ecol., 57, 229–247, https://doi.org/10.1007/s00248-008-9449-9, 2009.
Chamizo, S., Cantón, Y., Miralles, I., and Domingo, F.: Biological soil crust development affects physicochemical characteristics of soil surface in semiarid ecosystems, Soil Biol. Biochem., 49, 96–105, https://doi.org/10.1016/j.soilbio.2012.02.017, 2012a.
Chamizo, S., Stevens, A., Canton, Y., Miralles, I., Domingo, F., and Van Wesemael, B.: Discriminating soil crust type, development stage and degree of disturbance in semiarid environments from their spectral characteristics, Eur. J. Soil Sci., 63, 42–53, https://doi.org/10.1111/j.1365-2389.2011.01406.x, 2012b.
Chamizo, S., Belnap, J., Eldridge, D. J., Cantón, Y., and Malam Issa, O.: The Role of Biocrusts in Arid Land Hydrology, in: Biological Soil Crusts: An Organizing Principle in Drylands, edited by: Weber, B., Büdel, B., and Belnap, J., Springer International Publishing, Cham, 321–346, https://doi.org/10.1007/978-3-319-30214-0_17, 2016.
Chen, J., Zhang, M. Y., Wang, L., Shimazaki, H., and Tamura, M.: A new index for mapping lichen-dominated biological soil crusts in desert areas, Remote Sens. Environ., 96, 165–175, https://doi.org/10.1016/j.rse.2005.02.011, 2005.
Chen, N., Jayaprakash, C., Yu, K., and Guttal, V.: Rising Variability, Not Slowing Down, as a Leading Indicator of a Stochastically Driven Abrupt Transition in a Dryland Ecosystem, Am. Nat., 191, E1–E14, https://doi.org/10.1086/694821, 2018.
Chen, N., Yu, K. L., Jia, R. L., Teng, J. L., and Zhao, C. M.: Biocrust as one of multiple stable states in global drylands, Sci. Adv., 6, eaay3763, https://doi.org/10.1126/sciadv.aay3763, 2020.
Chytrý, M., Hennekens, S. M., Jiménez-Alfaro, B., Knollová, I., Dengler, J., Jansen, F., Landucci, F., Schaminée, J. H. J., Aćić, S., Agrillo, E., Ambarl, D., Angelini, P., Apostolova, I., Attorre, F., Berg, C., Bergmeier, E., Biurrun, I., Botta-Dukát, Z., Brisse, H., Campos, J. A., Carlón, L., Čarni, A., Casella, L., Csiky, J., Ćušterevska, R., Dajić Stevanović, Z., Danihelka, J., De Bie, E., de Ruffray, P., De Sanctis, M., Dickoré, W. B., Dimopoulos, P., Dubyna, D., Dziuba, T., Ejrnaes, R., Ermakov, N., Ewald, J., Fanelli, G., Fernández-González, F., FitzPatrick, Ú., Font, X., García-Mijangos, I., Gavilán, R. G., Golub, V., Guarino, R., Haveman, R., Indreica, A., Işik Gürsoy, D., Jandt, U., Janssen, J. A. M., Jiroušek, M., Kącki, Z., Kavgac, A., Kleikamp, M., Kolomiychuk, V., Krstivojević Ćuk, M., Krstonoić, D., Kuzemko, A., Lenoir, J., Lysenko, T., Marcenò, C., Martynenko, V., Michalcová, D., Moeslund, J. E., Onyshchenko, V., Pedashenko, H., Pérez-Haase, A., Peterka, T., Prokhorov, V., Raomavičius, V., Rodríguez-Rojo, M. P., Rodwell, J. S., Rogova, T., Ruprecht, E., Rūsiṅa, S., Seidler, G., Šibík, J., Šilc, U., Škvorc, Ž., Sopotlieva, D., Stančić, Z., Svenning, J.-C., Swacha, G., Tsiripidis, I., Turtureanu, P. D., Uğurlu, E., Uogintas, D., Valachovič, M., Vashenyak, Y., Vassilev, K., Venanzoni, R., Virtanen, R., Weekes, L., Willner, W., Wohlgemuth, T., and Yamalov, S.: European Vegetation Archive (EVA): an integrated database of European vegetation plots, Appl. Veg. Sci., 19, 173–180, https://doi.org/10.1111/avsc.12191, 2016.
Collier, E. A., Perroy, R. L., Reed, S. C., and Price, J. P.: Mapping biological soil crusts in a Hawaiian dryland, Int. J. Remote Sens., 43, 484–509, https://doi.org/10.1080/01431161.2021.2003904, 2022.
Condon, L. A. and Pyke, D. A.: Fire and Grazing Influence Site Resistance to Bromus tectorum Through Their Effects on Shrub, Bunchgrass and Biocrust Communities in the Great Basin (USA), Ecosystems, 21, 1416–1431, https://doi.org/10.1007/s10021-018-0230-8, 2018.
Crego, R. D., Stabach, J. A., Connette, G., and Leroy, B.: Implementation of species distribution models in Google Earth Engine, Divers. Distrib., 28, 904–916, https://doi.org/10.1111/ddi.13491, 2022.
Cuddington, K., Fortin, M., Gerber, L., Hastings, A., Liebhold, A., O'Connor, M., and Ray, C.: Process-based models are required to manage ecological systems in a changing world, Ecosphere, 4, 1–12, 2013.
Deng, M., Meng, X., Lu, Y., Li, Z., Zhao, L., Niu, H., Chen, H., Shang, L., Wang, S., and Sheng, D.: The Response of Vegetation to Regional Climate Change on the Tibetan Plateau Based on Remote Sensing Products and the Dynamic Global Vegetation Model, Remote Sensing, 14, 3337, https://doi.org/10.3390/rs14143337, 2022.
Durham, R. A., Doherty, K. D., Antoninka, A. J., Ramsey, P. W., and Bowker, M. A.: Insolation and disturbance history drive biocrust biodiversity in Western Montana rangelands, Plant Soil, 430, 151–169, https://doi.org/10.1007/s11104-018-3725-3, 2018.
Elbert, W., Weber, B., Burrows, S., Steinkamp, J., Buedel, B., Andreae, M. O., and Pöschl, U.: Contribution of cryptogamic covers to the global cycles of carbon and nitrogen, Nat. Geosci., 5, 459–462, https://doi.org/10.1038/ngeo1486, 2012.
Eldridge, D. J. and Delgado-Baquerizo, M.: The influence of climatic legacies on the distribution of dryland biocrust communities, Glob. Change Biol., 25, 327–336, https://doi.org/10.1111/gcb.14506, 2019.
Eldridge, D. J. and Tozer, M. E.: Environmental factors relating to the distribution of terricolous bryophytes and lichens in semi-arid eastern Australia, Bryologist, 100, 28–39, 1997.
Eldridge, D. J., Reed, S., Travers, S. K., Bowker, M. A., Maestre, F. T., Ding, J., Havrilla, C., Rodriguez-Caballero, E., Barger, N., Weber, B., Antoninka, A., Belnap, J., Chaudhary, B., Faist, A., Ferrenberg, S., Huber-Sannwald, E., Malam Issa, O., and Zhao, Y.: The pervasive and multifaceted influence of biocrusts on water in the world's drylands, Glob. Change Biol., 26, 6003–6014, https://doi.org/10.1111/gcb.15232, 2020.
Engel, T., Bruelheide, H., Hoss, D., Sabatini, F. M., Altman, J., Arfin-Khan, M. A. S., Bergmeier, E., Černý, T., Chytrý, M., Dainese, M., Dengler, J., Dolezal, J., Field, R., Fischer, F. M., Huygens, D., Jandt, U., Jansen, F., Jentsch, A., Karger, D. N., Kattge, J., Lenoir, J., Lens, F., Loos, J., Niinemets, Ü., Overbeck, G. E., Ozinga, W. A., Penuelas, J., Peyre, G., Phillips, O., Reich, P. B., Römermann, C., Sandel, B., Schmidt, M., Schrodt, F., Velez-Martin, E., Violle, C., and Pillar, V.: Traits of dominant plant species drive normalized difference vegetation index in grasslands globally, Global Ecol. Biogeogr., 32, 695–706, https://doi.org/10.1111/geb.13644, 2023.
Fang, S., Yu, W., and Qi, Y.: Spectra and vegetation index variations in moss soil crust in different seasons, and in wet and dry conditions, International Journal of Applied Earth Observation and Geoinformation, 38, 261–266, https://doi.org/10.1016/j.jag.2015.01.018, 2015.
Fatichi, S., Pappas, C., Zscheischler, J., and Leuzinger, S.: Modelling carbon sources and sinks in terrestrial vegetation, New Phytol., 221, 652–668, https://doi.org/10.1111/nph.15451, 2019.
Ferrenberg, S., Reed, S. C., and Belnap, J.: Climate change and physical disturbance cause similar community shifts in biological soil crusts, P. Natl. Acad. Sci. USA, 112, 12116–12121, https://doi.org/10.1073/pnas.1509150112, 2015.
Fischer, T. and Subbotina, M.: Climatic and soil texture threshold values for cryptogamic cover development: a meta analysis, Biologia, 69, 1520–1530, https://doi.org/10.2478/s11756-014-0464-7, 2014.
Gabay, T., Rotem, G., Gillor, O., and Ziv, Y.: Understanding changes in biocrust communities following phosphate mining in the Negev Desert, Environ. Res., 207, 112200, https://doi.org/10.1016/j.envres.2021.112200, 2022.
Gao, L., Bowker, M. A., Xu, M., Sun, H., Tuo, D., and Zhao, Y.: Biological soil crusts decrease erodibility by modifying inherent soil properties on the Loess Plateau, China, Soil Biol. Biochem., 105, 49–58, https://doi.org/10.1016/j.soilbio.2016.11.009, 2017.
Garcia-Pichel, F., Loza, V., Marusenko, Y., Mateo, P., and Potrafka, R. M.: Temperature Drives the Continental-Scale Distribution of Key Microbes in Topsoil Communities, Science, 340, 1574–1577, https://doi.org/10.1126/science.1236404, 2013.
García-Roselló, E., Guisande, C., Manjarrés-Hernández, A., González-Dacosta, J., Heine, J., Pelayo-Villamil, P., González-Vilas, L., Vari, R. P., Vaamonde, A., Granado-Lorencio, C., and Lobo, J. M.: Can we derive macroecological patterns from primary Global Biodiversity Information Facility data?, Global Ecol. Biogeogr., 24, 335–347, https://doi.org/10.1111/geb.12260, 2015.
Gassmann, F., Klotzli, F., and Walther, G. R.: Simulation of observed types of dynamics of plants and plant communities, J. Veg. Sci., 11, 397–408, https://doi.org/10.2307/3236632, 2000.
Havrilla, C. A. and Barger, N. N.: Biocrusts and their disturbance mediate the recruitment of native and exotic grasses from a hot desert ecosystem, Ecosphere, 9, e02361, https://doi.org/10.1002/ecs2.2361, 2018.
Havrilla, C. A., Chaudhary, V. B., Ferrenberg, S., Antoninka, A. J., Belnap, J., Bowker, M. A., Eldridge, D. J., Faist, A. M., Huber-Sannwald, E., Leslie, A. D., Rodriguez-Caballero, E., Zhang, Y., Barger, N. N., and Vries, F.: Towards a predictive framework for biocrust mediation of plant performance: A meta-analysis, J. Ecol., 107, 2789–2807, https://doi.org/10.1111/1365-2745.13269, 2019.
Hu, P., Zhang, W., Xiao, L., Yang, R., Xiao, D., Zhao, J., Wang, W., Chen, H., and Wang, K.: Moss-dominated biological soil crusts modulate soil nitrogen following vegetation restoration in a subtropical karst region, Geoderma, 352, 70–79, https://doi.org/10.1016/j.geoderma.2019.05.047, 2019.
Jia, R., Chen, N., Yu, K., and Zhao, C.: High rainfall frequency promotes the dominance of biocrust under low annual rainfall, Plant Soil, 435, 257–275, https://doi.org/10.1007/s11104-018-3880-6, 2019.
Jiménez-Valverde, A., Lobo, J. M., and Hortal, J.: Not as good as they seem: the importance of concepts in species distribution modelling, Divers. Distrib., 14, 885–890, https://doi.org/10.1111/j.1472-4642.2008.00496.x, 2008.
Karnieli, A.: Development and implementation of spectral crust index over dune sands, Int. J. Remote Sens., 18, 1207–1220, https://doi.org/10.1080/014311697218368, 1997.
Karnieli, A., Kidron, G. J., Glaesser, C., and Ben-Dor, E.: Spectral characteristics of cyanobacteria soil crust in semiarid environments, Remote Sens. Environ., 69, 67–75, https://doi.org/10.1016/s0034-4257(98)00110-2, 1999.
Kidron, G. J.: Biocrust research: A critical view on eight common hydrological-related paradigms and dubious theses, Ecohydrology, 12, e2061, https://doi.org/10.1002/eco.2061, 2018.
Kidron, G. J.: The enigmatic absence of cyanobacterial biocrusts from the Namib fog belt: Do dew and fog hold the key?, Flora, 257, 151416, https://doi.org/10.1016/j.flora.2019.06.002, 2019.
Kidron, G. J. and Xiao, B.: A false paradigm? Do biocrust types necessarily reflect “successional stages”?, Ecohydrology, 17, e2610, https://doi.org/10.1002/eco.2610, 2023.
Kidron, G. J., Lichner, L., Fischer, T., Starinsky, A., and Or, D.: Mechanisms for biocrust-modulated runoff generation – A review, Earth-Sci. Rev., 231, 104100, https://doi.org/10.1016/j.earscirev.2022.104100, 2022.
Kropfl, A. I., Distel, R. A., Cecchi, G. A., and Villasuso, N. M.: Functional Role of Moss Biocrust in Disturbed Semiarid Shrublands of North-Eastern Patagonia, Argentina, Appl. Ecol. Env. Res., 20, 905–917, https://doi.org/10.15666/aeer/2001_905917, 2022.
Lenton, T. M., Dahl, T. W., Daines, S. J., Mills, B. J., Ozaki, K., Saltzman, M. R., and Porada, P.: Earliest land plants created modern levels of atmospheric oxygen, P. Natl. Acad. Sci. USA, 113, 9704–9709, https://doi.org/10.1073/pnas.1604787113, 2016.
Li, S., Bowker, M. A., and Xiao, B.: Biocrusts enhance non-rainfall water deposition and alter its distribution in dryland soils, J. Hydrol., 595, 126050, https://doi.org/10.1016/j.jhydrol.2021.126050, 2021.
Li, X., Sun, J., Zhang, H., Tan, H., Hui, R., Qi, J., Zhang, P., and Ward, N. D.: Warming decreases desert ecosystem functioning by altering biocrusts in drylands, J. Appl. Ecol., 60, 2676–2687, https://doi.org/10.1111/1365-2664.14528, 2023.
Ma, X., Zhao, Y., Yang, K., Ming, J., Qiao, Y., Xu, M., and Pan, X.: Long-term light grazing does not change soil organic carbon stability and stock in biocrust layer in the hilly regions of drylands, J. Arid Land, 15, 940–959, https://doi.org/10.1007/s40333-023-0064-x, 2023.
Machado de Lima, N. M., Muñoz-Rojas, M., Vázquez-Campos, X., and Branco, L. H. Z.: Biocrust cyanobacterial composition, diversity, and environmental drivers in two contrasting climatic regions in Brazil, Geoderma, 386, 114914, https://doi.org/10.1016/j.geoderma.2020.114914, 2021.
Maestre, F. T., Benito, B. M., Berdugo, M., Concostrina-Zubiri, L., Delgado-Baquerizo, M., Eldridge, D. J., Guirado, E., Gross, N., Kefi, S., Le Bagousse-Pinguet, Y., Ochoa-Hueso, R., and Soliveres, S.: Biogeography of global drylands, New Phytol., 231, 540–558, https://doi.org/10.1111/nph.17395, 2021.
Mäkinen, J., Numminen, E., Niittynen, P., Luoto, M., and Vanhatalo, J.: Spatial confounding in Bayesian species distribution modeling, Ecography, 2022, e06183, https://doi.org/10.1111/ecog.06183, 2022.
Marsh, J., Nouvet, S., Sanborn, P., and Coxson, D.: Composition and function of biological soil crust communities along topographic gradients in grasslands of central interior British Columbia (Chilcotin) and southwestern Yukon (Kluane), Can. J. Botany, 84, 717–736, https://doi.org/10.1139/b06-026, 2006.
McCann, E., Reed, S. C., Saud, P., Reibold, R. H., Howell, A., and Faist, A. M.: Plant growth and biocrust-fire interactions across five North American deserts, Geoderma, 401, 115325, https://doi.org/10.1016/j.geoderma.2021.115325, 2021.
McCune, B., Yang, S., Jovan, S., and Root, H. T.: Climate and epiphytic macrolichen communities in the Four Corners region of the USA, Bryologist, 125, 70–90, https://doi.org/10.1639/0007-2745-125.1.070, 2022.
Ming, J., Zhao, Y., Wu, Q., He, H., and Gao, L.: Soil temperature dynamics and freezing processes for biocrustal soils in frozen soil regions on the Qinghai–Tibet Plateau, Geoderma, 409, 115655, https://doi.org/10.1016/j.geoderma.2021.115655, 2022.
Miranda-González, R. and McCune, B.: The weight of the crust: Biomass of crustose lichens in tropical dry forest represents more than half of foliar biomass, Biotropica, 52, 1298–1308, https://doi.org/10.1111/btp.12837, 2020.
Morillas, L. and Gallardo, A.: Biological soil crusts and wetting events: Effects on soil N and C cycles, Appl. Soil Ecol., 94, 1–6, https://doi.org/10.1016/j.apsoil.2015.04.015, 2015.
Munoz-Martin, M. A., Becerra-Absalon, I., Perona, E., Fernandez-Valbuena, L., Garcia-Pichel, F., and Mateo, P.: Cyanobacterial biocrust diversity in Mediterranean ecosystems along a latitudinal and climatic gradient, New Phytol., 221, 123–141, https://doi.org/10.1111/nph.15355, 2019.
Noy, K., Ohana-Levi, N., Panov, N., Silver, M., and Karnieli, A.: A long-term spatiotemporal analysis of biocrusts across a diverse arid environment: The case of the Israeli-Egyptian sandfield, Sci. Total Environ., 774, 145154, https://doi.org/10.1016/j.scitotenv.2021.145154, 2021.
Oliva, G., Dos Santos, E., Sofia, O., Umana, F., Massara, V., Garcia Martinez, G., Caruso, C., Cariac, G., Echevarria, D., Fantozzi, A., Butti, L., Bran, D., Gaitan, J., Ferrante, D., Paredes, P., Dominguez, E., and Maestre, F. T.: The MARAS dataset, vegetation and soil characteristics of dryland rangelands across Patagonia, Sci. Data, 7, 327, https://doi.org/10.1038/s41597-020-00658-0, 2020.
Palmer, B., Hernandez, R., and Lipson, D. A.: The fate of biological soil crusts after fire: A meta-analysis, Global Ecol. Conserv., 24, e01380, https://doi.org/10.1016/j.gecco.2020.e01380, 2020.
Palmer, B., Lawson, D., and Lipson, D. A.: Years After a Fire, Biocrust Microbial Communities are Similar to Unburned Communities in a Coastal Grassland, Microb. Ecol., 85, 1028–1044, https://doi.org/10.1007/s00248-022-02137-y, 2022.
Panigada, C., Tagliabue, G., Zaady, E., Rozenstein, O., Garzonio, R., Di Mauro, B., De Amicis, M., Colombo, R., Cogliati, S., Miglietta, F., and Rossini, M.: A new approach for biocrust and vegetation monitoring in drylands using multi-temporal Sentinel-2 images, Prog. Phys. Geogr., 43, 496–520, https://doi.org/10.1177/0309133319841903, 2019.
Pearce, J. L., Cherry, K., Drielsma, M., Ferrier, S., and Whish, G.: Incorporating expert opinion and fine-scale vegetation mapping into statistical models of faunal distribution, J. Appl. Ecol., 38, 412–424, https://doi.org/10.1046/j.1365-2664.2001.00608.x, 2001.
Perry, G. L. W., Seidl, R., Bellvé, A. M., and Rammer, W.: An Outlook for Deep Learning in Ecosystem Science, Ecosystems, 25, 1700–1718, https://doi.org/10.1007/s10021-022-00789-y, 2022.
Porada, P., Weber, B., Elbert, W., Pöschl, U., and Kleidon, A.: Estimating global carbon uptake by lichens and bryophytes with a process-based model, Biogeosciences, 10, 6989–7033, https://doi.org/10.5194/bg-10-6989-2013, 2013.
Porada, P., Pöschl, U., Kleidon, A., Beer, C., and Weber, B.: Estimating global nitrous oxide emissions by lichens and bryophytes with a process-based productivity model, Biogeosciences, 14, 1593–1602, https://doi.org/10.5194/bg-14-1593-2017, 2017.
Porada, P., Tamm, A., Raggio, J., Cheng, Y., Kleidon, A., Pöschl, U., and Weber, B.: Global NO and HONO emissions of biological soil crusts estimated by a process-based non-vascular vegetation model, Biogeosciences, 16, 2003–2031, https://doi.org/10.5194/bg-16-2003-2019, 2019.
Pravalie, R.: Drylands extent and environmental issues. A global approach, Earth-Sci. Rev., 161, 259–278, https://doi.org/10.1016/j.earscirev.2016.08.003, 2016.
Pushkareva, E., Johansen, J. R., and Elster, J.: A review of the ecology, ecophysiology and biodiversity of microalgae in Arctic soil crusts, Polar Biol., 39, 2227–2240, https://doi.org/10.1007/s00300-016-1902-5, 2016.
Qiu, D., Bowker, M. A., Xiao, B., Zhao, Y., Zhou, X., and Li, X.: Mapping biocrust distribution in China's drylands under changing climate, Sci. Total Environ., 905, 167211, https://doi.org/10.1016/j.scitotenv.2023.167211, 2023.
Quillet, A., Peng, C., and Garneau, M.: Toward dynamic global vegetation models for simulating vegetation-climate interactions and feedbacks: recent developments, limitations, and future challenges, Environ. Rev., 18, 333–353, https://doi.org/10.1139/a10-016, 2010.
Read, C. F., Duncan, D. H., Vesk, P. A., Elith, J., and Wan, S.: Biocrust morphogroups provide an effective and rapid assessment tool for drylands, J. Appl. Ecol., 51, 1740–1749, https://doi.org/10.1111/1365-2664.12336, 2014.
Reed, S. C., Coe, K. K., Sparks, J. P., Housman, D. C., Zelikova, T. J., and Belnap, J.: Changes to dryland rainfall result in rapid moss mortality and altered soil fertility, Nat. Clim. Change, 2, 752–755, https://doi.org/10.1038/nclimate1596, 2012.
Rodriguez-Caballero, E., Knerr, T., and Weber, B.: Importance of biocrusts in dryland monitoring using spectral indices, Remote Sens. Environ., 170, 32–39, https://doi.org/10.1016/j.rse.2015.08.034, 2015.
Rodríguez-Caballero, E., Escribano, P., Olehowski, C., Chamizo, S., Hill, J., Cantón, Y., and Weber, B.: Transferability of multi- and hyperspectral optical biocrust indices, ISPRS J. Photogramm., 126, 94–107, https://doi.org/10.1016/j.isprsjprs.2017.02.007, 2017.
Rodriguez-Caballero, E., Belnap, J., Budel, B., Crutzen, P. J., Andreae, M. O., Pöschl, U., and Weber, B.: Dryland photoautotrophic soil surface communities endangered by global change, Nat. Geosci., 11, 185–189, https://doi.org/10.1038/s41561-018-0072-1, 2018.
Rodriguez-Caballero, E., Stanelle, T., Egerer, S., Cheng, Y., Su, H., Canton, Y., Belnap, J., Andreae, M. O., Tegen, I., and Reick, C. H.: Global cycling and climate effects of aeolian dust controlled by biological soil crusts, Nat. Geosci., 15, 458–463, https://doi.org/10.1038/s41561-022-00942-1, 2022a.
Rodríguez-Caballero, E., Reyes, A., Kratz, A., Caesar, J., Guirado, E., Schmiedel, U., Escribano, P., Fiedler, S., and Weber, B.: Effects of climate change and land use intensification on regional biological soil crust cover and composition in southern Africa, Geoderma, 406, 115508, https://doi.org/10.1016/j.geoderma.2021.115508, 2022b.
Root, H. T. and McCune, B.: Regional patterns of biological soil crust lichen species composition related to vegetation, soils, and climate in Oregon, USA, J. Arid Environ., 79, 93–100, https://doi.org/10.1016/j.jaridenv.2011.11.017, 2012.
Sabatini, F. M., Lenoir, J., Hattab, T., Arnst, E. A., Chytrý, M., Dengler, J., De Ruffray, P., Hennekens, S. M., Jandt, U., Jansen, F., Jiménez-Alfaro, B., Kattge, J., Levesley, A., Pillar, V. D., Purschke, O., Sandel, B., Sultana, F., Aavik, T., Aćić, S., Acosta, A. T. R., Agrillo, E., Alvarez, M., Apostolova, I., Arfin Khan, M. A. S., Arroyo, L., Attorre, F., Aubin, I., Banerjee, A., Bauters, M., Bergeron, Y., Bergmeier, E., Biurrun, I., Bjorkman, A. D., Bonari, G., Bondareva, V., Brunet, J., Čarni, A., Casella, L., Cayuela, L., Černý, T., Chepinoga, V., Csiky, J., Ćuterevska, R., De Bie, E., Gasper, A. L., De Sanctis, M., Dimopoulos, P., Dolezal, J., Dziuba, T., El-Sheikh, M. A. E. R. M., Enquist, B., Ewald, J., Fazayeli, F., Field, R., Finckh, M., Gachet, S., Galán-de-Mera, A., Garbolino, E., Gholizadeh, H., Giorgis, M., Golub, V., Alsos, I. G., Grytnes, J. A., Guerin, G. R., Gutiérrez, A. G., Haider, S., Hatim, M. Z., Hérault, B., Hinojos Mendoza, G., Hölzel, N., Homeier, J., Hubau, W., Indreica, A., Janssen, J. A. M., Jedrzejek, B., Jentsch, A., Jürgens, N., Kącki, Z., Kapfer, J., Karger, D. N., Kavgac, A., Kearsley, E., Kessler, M., Khanina, L., Killeen, T., Korolyuk, A., Kreft, H., Kühl, H. S., Kuzemko, A., Landucci, F., Lengyel, A., Lens, F., Lingner, D. V., Liu, H., Lysenko, T., Mahecha, M. D., Marcenò, C., Martynenko, V., Moeslund, J. E., Monteagudo Mendoza, A., Mucina, L., Müller, J. V., Munzinger, J., Naqinezhad, A., Noroozi, J., Nowak, A., Onyshchenko, V., Overbeck, G. E., Pärtel, M., Pauchard, A., Peet, R. K., Peñuelas, J., Pérez-Haase, A., Peterka, T., Petřík, P., Peyre, G., Phillips, O. L., Prokhorov, V., Rašomavičius, V., Revermann, R., Rivas-Torres, G., Rodwell, J. S., Ruprecht, E., Rūsiṅa, S., Samimi, C., Schmidt, M., Schrodt, F., Shan, H., Shirokikh, P., Šibík, J., Šilc, U., Sklenář, P., Škvorc, Ž., Sparrow, B., Sperandii, M. G., Stančić, Z., Svenning, J. C., Tang, Z., Tang, C. Q., Tsiripidis, I., Vanselow, K. A., Vásquez Martínez, R., Vassilev, K., Vélez-Martin, E., Venanzoni, R., Vibrans, A. C., Violle, C., Virtanen, R., Wehrden, H., Wagner, V., Walker, D. A., Waller, D. M., Wang, H. F., Wesche, K., Whitfeld, T. J. S., Willner, W., Wiser, S. K., Wohlgemuth, T., Yamalov, S., Zobel, M., and Bruelheide, H.: sPlotOpen – An environmentally balanced, open-access, global dataset of vegetation plots, Global Ecol. Biogeogr., 30, 1740–1764, https://doi.org/10.1111/geb.13346, 2021.
Shi, W., Pan, Y.-X., Zhang, Y.-F., Hu, R., and Wang, X.-P.: The effect of different biocrusts on soil hydraulic properties in the Tengger Desert, China, Geoderma, 430, 116304, https://doi.org/10.1016/j.geoderma.2022.116304, 2023.
Skidmore, A. K., Franklin, J., Dawson, T. P., and Pilesjö, P.: Geospatial tools address emerging issues in spatial ecology: a review and commentary on the Special Issue, Int. J. Geogr. Inf. Sci., 25, 337–365, https://doi.org/10.1080/13658816.2011.554296, 2011.
Soberon, J. and Nakamura, M.: Niches and distributional areas: Concepts, methods, and assumptions, P. Natl. Acad. Sci. USA, 106, 19644–19650, 2009.
Song, G., Hui, R., Yang, H., Wang, B., and Li, X.: Biocrusts mediate the plant community composition of dryland restoration ecosystems, Sci. Total Environ., 844, 157135, https://doi.org/10.1016/j.scitotenv.2022.157135, 2022.
Sun, F., Xiao, B., Kidron, G. J., and Tuller, M.: Towards the effects of moss-dominated biocrusts on surface soil aeration in drylands: Air permeability analysis and modeling, Catena, 223, 106942, https://doi.org/10.1016/j.catena.2023.106942, 2023.
Sun, H., Ma, X., Liu, Y., Zhou, G. Y., Ding, J. L., Lu, L., Wang, T. J., Yang, Q. L., Shu, Q. T., and Zhang, F.: A New Multiangle Method for Estimating Fractional Biocrust Coverage From Sentinel-2 Data in Arid Areas, IEEE T. Geosci. Remote Sens., 62, 4404015, https://doi.org/10.1109/tgrs.2024.3361249, 2024.
Thonicke, K., Venevsky, S., Sitch, S., and Cramer, W.: The role of fire disturbance for global vegetation dynamics: coupling fire into a Dynamic Global Vegetation Model, Global Ecol. Biogeogr., 10, 661–677, https://doi.org/10.1046/j.1466-822x.2001.00175.x, 2001.
Tucker, C. L., McHugh, T. A., Howell, A., Gill, R., Weber, B., Belnap, J., Grote, E., and Reed, S. C.: The concurrent use of novel soil surface microclimate measurements to evaluate CO2 pulses in biocrusted interspaces in a cool desert ecosystem, Biogeochemistry, 135, 239–249, https://doi.org/10.1007/s10533-017-0372-3, 2017.
Velasco Ayuso, S., Oñatibia, G. R., Maestre, F. T., and Yahdjian, L.: Grazing pressure interacts with aridity to determine the development and diversity of biological soil crusts in Patagonian rangelands, Land Degrad. Dev., 31, 488–499, https://doi.org/10.1002/ldr.3465, 2019.
Wang, S., Liu, B., Zhao, Y., Gao, L., Yin, B., Yang, K., and Ji, J.: Determination of the representative elementary area (REA) of biocrusts: A case study from the Hilly Loess Plateau region, China, Geoderma, 406, 115502, https://doi.org/10.1016/j.geoderma.2021.115502, 2022.
Wang, Y., Xiao, B., Wang, W., and Kidron, G. J.: Interactions between biocrusts and herbaceous communities are divergent in dry and wet semiarid ecosystems, Sci. Total Environ., 941, 173759, https://doi.org/10.1016/j.scitotenv.2024.173759, 2024.
Wang, Z., Wu, B., Zhang, M., Zeng, H., Yang, L., Tian, F., Ma, Z., and Wu, H.: Indices enhance biological soil crust mapping in sandy and desert lands, Remote Sens. Environ., 278, 113078, https://doi.org/10.1016/j.rse.2022.113078, 2022.
Weber, B. and Hill, J.: Remote Sensing of Biological Soil Crusts at Different Scales, in: Biological Soil Crusts: An Organizing Principle in Drylands, edited by: Weber, B., Büdel, B., and Belnap, J., Springer International Publishing, Cham, 215–234, https://doi.org/10.1007/978-3-319-30214-0_12, 2016.
Weber, B., Olehowski, C., Knerr, T., Hill, J., Deutschewitz, K., Wessels, D. C. J., Eitel, B., and Buedel, B.: A new approach for mapping of Biological Soil Crusts in semidesert areas with hyperspectral imagery, Remote Sens. Environ., 112, 2187–2201, https://doi.org/10.1016/j.rse.2007.09.014, 2008.
Weber, B., Budel, B., and Belnap, J. (Eds.): Biological Soil Crusts: An Organizing Principle in Drylands, Springer Nature, https://doi.org/10.1007/978-3-319-30214-0, 2016.
Weber, B., Belnap, J., Budel, B., Antoninka, A. J., Barger, N. N., Chaudhary, V. B., Darrouzet-Nardi, A., Eldridge, D. J., Faist, A. M., Ferrenberg, S., Havrilla, C. A., Huber-Sannwald, E., Malam Issa, O., Maestre, F. T., Reed, S. C., Rodriguez-Caballero, E., Tucker, C., Young, K. E., Zhang, Y., Zhao, Y., Zhou, X., and Bowker, M. A.: What is a biocrust? A refined, contemporary definition for a broadening research community, Biol. Rev., 97, 1768–1785, https://doi.org/10.1111/brv.12862, 2022.
Wei, X., Qin, F., Han, B., Zhou, H., Liu, M., and Shao, X.: Spatial variations of bacterial communities associated with biological soil crusts along a climatic gradient in alpine grassland ecosystems, Plant Soil, 480, 493–506, https://doi.org/10.1007/s11104-022-05595-y, 2022.
Whitney, K. M., Vivoni, E. R., Duniway, M. C., Bradford, J. B., Reed, S. C., and Belnap, J.: Ecohydrological role of biological soil crusts across a gradient in levels of development, Ecohydrology, 10, e1875, https://doi.org/10.1002/eco.1875, 2017.
Williams, A. J., Buck, B. J., Soukup, D. A., and Merkler, D. J.: Geomorphic controls on biological soil crust distribution: A conceptual model from the Mojave Desert (USA), Geomorphology, 195, 99–109, https://doi.org/10.1016/j.geomorph.2013.04.031, 2013.
Wolf, S., Mahecha, M. D., Sabatini, F. M., Wirth, C., Bruelheide, H., Kattge, J., Moreno Martinez, A., Mora, K., and Kattenborn, T.: Citizen science plant observations encode global trait patterns, Nat. Ecol. Evol., 6, 1850–1859, https://doi.org/10.1038/s41559-022-01904-x, 2022.
Wright, A. J. and Collins, S. L.: Drought experiments need to incorporate atmospheric drying to better simulate climate change, BioScience, 74, 65–71, https://doi.org/10.1093/biosci/biad105, 2024.
Yang, H., Liu, C., Liu, Y., and Xing, Z.: Impact of human trampling on biological soil crusts determined by soil microbial biomass, enzyme activities and nematode communities in a desert ecosystem, Eur. J. Soil Biol., 87, 61–71, https://doi.org/10.1016/j.ejsobi.2018.05.005, 2018.
Yu, H., Cooper, A. R., and Infante, D. M.: Improving species distribution model predictive accuracy using species abundance: Application with boosted regression trees, Ecol. Model., 432, 109202, https://doi.org/10.1016/j.ecolmodel.2020.109202, 2020.
Zhang, Y. M., Chen, J., Wang, L., Wang, X. Q., and Gu, Z. H.: The spatial distribution patterns of biological soil crusts in the Gurbantunggut Desert, Northern Xinjiang, China, J. Arid Environ., 68, 599–610, https://doi.org/10.1016/j.jaridenv.2006.06.012, 2007.
Zhao, Y., Qin, N., Weber, B., and Xu, M.: Response of biological soil crusts to raindrop erosivity and underlying influences in the hilly Loess Plateau region, China, Biodivers. Conserv., 23, 1669–1686, https://doi.org/10.1007/s10531-014-0680-z, 2014.
Zhao, Y., Ji, J., Zhang, W., Ming, J., Huang, W., and Gao, L.: Characteristics of spatial and temporal variability in the distribution of biological soil crusts on the Loess Plateau, China, Chinese J. Appl. Ecol., 35, 739–748, 2024.