the Creative Commons Attribution 4.0 License.
the Creative Commons Attribution 4.0 License.
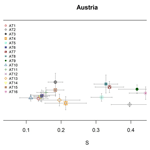
Evaluating the Tea Bag Index approach for different management practices in agroecosystems using long-term field experiments in Austria and Sweden
Maria Regina Gmach
Martin Anders Bolinder
Lorenzo Menichetti
Thomas Kätterer
Heide Spiegel
Olle Åkesson
Jürgen Kurt Friedel
Andreas Surböck
Agnes Schweinzer
Taru Sandén
Litter decomposition is an important factor affecting local and global C cycles. It is known that decomposition through soil microbial activity in ecosystems is mainly influenced by soil type and climatic conditions. However, for agroecosystems, there remains a need for a better understanding of how management practices influence litter decomposition. This study examined the effect of different management practices on decomposition at 29 sites with long-term (mean duration of 38 years) field experiments (LTEs) using the Tea Bag Index (TBI) protocol with standard litter (rooibos and green tea) developed by Keuskamp et al. (2013). The objective was to determine if the TBI decomposition rate (k) and stabilization factor (S) are sensitive enough to detect differences in litter decomposition between management practices as well as how they interact with edaphic factors, crop type and local climatic conditions. Tea bags were buried and collected after ∼90 d at 16 Austrian and 13 Swedish sites. The treatments in the Austrian LTEs focused on mineral and organic fertilizer application, tillage systems and crop residue management, whereas those in Sweden addressed cropping systems, mineral fertilizer application and tillage systems. The results for Austria showed that the incorporation of crop residue and high-N fertilizer application increased k, compared with crop residue removal and low or no N application, respectively. Minimum tillage had significantly higher k compared with reduced and conventional tillage. In Sweden, fertilized plots showed higher S than non-fertilized plots and high-N fertilizer had the highest k. Growing spring cereal led to higher k than forage crops. Random forest regressions for Austria and Sweden jointly showed that k and S were mainly governed by climatic conditions, which explained more than 70 % of their variation. However, under similar climatic conditions, management practices strongly influenced decomposition dynamics. It would be appropriate to apply the TBI approach to a more large-scale network using LTEs for agroecosystems, in order to improve the index's usefulness as an indicator of the effect of management practices on litter decomposition dynamics, particularly linking it with the potential for C storage.
- Article
(3516 KB) - Full-text XML
-
Supplement
(420 KB) - BibTeX
- EndNote
Soil organic carbon (SOC) is one of the most used indicators of soil quality, as its dynamics help regulate ecosystem functionality via its influence on physical, biological and chemical soil properties, which are critical for nutrient cycling and soil fertility (Davidson et al., 2006; Janzen, 2015). Management practices, such as fertilizer application, use of catch and cover crops, organic amendments, length of bare fallow periods, permanent surface protection with perennial crops, tillage practices, and aboveground crop residue management, impact the SOC balance of agroecosystems (Kätterer and Bolinder, 2022; Sandén et al., 2018; Paustian et al., 2016).
Agricultural soils play a crucial role in the global carbon (C) cycle due to their C sink capacity (Paustian et al., 2016; Lal, 2004). In this context, improved management practices that maintain or increase SOC stocks are considered essential in national greenhouse gas reporting systems (IPCC, 2006) as well as in other international incentives, such as the “4 per mille” initiative (Minasny et al., 2017). The SOC balance is dynamic and is determined by the difference between annual C inputs to soil and annual C outputs via the decay of existing soil organic matter and microbial activity, the latter of which is the main contributor to stable SOC (Tiefenbacher et al., 2021; Bolinder et al., 2007). Management practices have a great impact on these two factors by affecting either the amount of C input/output through decomposition or both factors simultaneously.
Litter decomposition is a complex biogeochemical process controlled by several biotic and abiotic factors, where the biological activity of decomposers varies with soil properties and is driven largely by climatic conditions (Daebeler et al., 2022; Bradford et al., 2016; Cleveland et al., 2014; Gholz et al., 2000). Decomposition and SOC stabilization are long-term processes; therefore, long-term field experiments (LTEs) are among the most useful resources for quantifying the impact of management practices on litter decomposition, SOC changes and soil functioning (Sandén et al., 2018; Kätterer et al., 2012; Bergkvist and Öborn, 2011). Within LTEs, in situ experiments determining litter mass loss over time are important for understanding SOC dynamics, nutrient cycling and colonization by soil biota under field conditions. The traditional method that has been used in ecology for more than 50 years consists of litterbag studies: known quantities of various organic materials are buried in the soil and are then successively retrieved at different intervals (Kampichler and Bruckner, 2009; Burgess et al., 2002; Bocock and Gilbert, 1957). These studies are not always comparable because they are subject to variations in factors such as litter type, mesh size, and sample preparation and analytical methods; moreover, the placement of litterbags may alter the microclimate for decomposers (Kampichler and Bruckner, 2009). Therefore, Keuskamp et al. (2013) developed a standardized, low-cost and time-efficient methodology called the Tea Bag Index (TBI). This technique characterizes the decomposition process using commercially available tea bags: green tea represents labile organic material, whereas rooibos tea is a surrogate for recalcitrant litter. A decomposition rate (k) and a stabilization factor (S) are also obtained, along with their chemical composition and the respective weight lost at a single point in time after an incubation period of ca. 90 d in the soil. The TBI approach is particularly useful for assessing geographical differences in decomposition dynamics, as results are directly comparable across sites, varying only with local edaphic and seasonal environmental conditions (Keuskamp et al., 2013).
In several studies, the TBI has been used as an indicator of biological (Sandén et al., 2021; Costantini et al., 2018) and microbial activity (Daebeler et al., 2022; Treharne et al., 2019; Tóth et al., 2017), ecosystem functioning, nutrient cycling (Zaller et al., 2016), and soil quality (Tresch et al., 2018; Buchholz et al., 2017). Generally, this follows the concepts of soil quality as reviewed by Bünemann et al. (2018), where TBI is primarily a biological soil quality indicator. According to the TBI community, data collected from networks of researchers and citizen scientists to construct a global TBI map (https://www.teatime4science.org, last access: 20 June 2022) show that most studies have used the TBI approach for different forest and grassland ecosystems or urban soils, whereas studies in agricultural fields represent less than 15 % of the TBI database. Indeed, there are only a few published studies (Daebeler et al., 2022; Dossou-Yovo et al., 2022; Struijk et al., 2022; Fu et al., 2021; Sandén et al., 2020; Barel et al., 2019; Poeplau et al., 2018; Sievers and Cook, 2018) that have employed the TBI approach to evaluate agroecosystems, and it is not clear if this method is sensitive enough to detect differences between management practices.
This study used the TBI approach to investigate the effect of management practices on the decomposition rate (k) and stabilization factor (S) at several LTEs in Austria and Sweden that have different soil characteristics and climatic conditions and are subjected to various management practices.
To the best of our knowledge, this is the first analysis using the TBI approach with such a large number of LTEs and management practices for agroecosystems. The management practices included organic amendments, crop rotations, aboveground crop residue handling, mineral fertilizer application and tillage. Our objectives were as follows: (i) to evaluate if the TBI k and S parameters are sensitive enough to distinguish litter decomposition between different management practices, (ii) to quantify the effect of management practices on k and S, and (iii) to identify the most important local climate and/or soil properties affecting litter decomposition in Austria and Sweden.
Table 1Experimental sites in Austria: site acronym; location name; year of TBI measurements; and category and type of LTE, including its duration, main crop cultivated during the TBI measurements and management treatments.
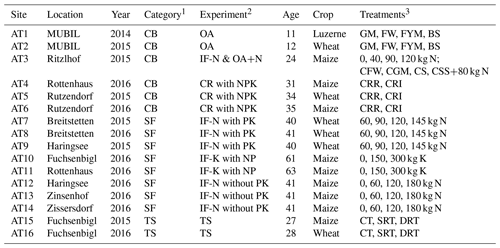
1 CB: carbon balance practices; SF: soil fertility; TS: tillage systems. 2 OA: organic amendments; IF-N & OA+N: N inorganic fertilization and organic amendments plus mineral N; CR & IF-P with NK: crop residue and P inorganic fertilization with 90 and 40 kg ha−1 of N and K, respectively; IF-N with PK: N inorganic fertilization with 55 and 180 kg ha−1 of P and K, respectively; IF-N without PK: N inorganic fertilization; IF-K with NP: K inorganic fertilization with 40 and 120 kg ha−1 of P and K, respectively; TS: tillage system. 3 GM: green manure; FW: municipal compost and green manure; FYM: farmyard manure; BS: biogas slurry; CFW: compost food waste with 175 kg N ha−1; CGM: compost green manure with 175 kg N ha−1; CS: compost slurry with 175 kg N ha−1; CSS: compost sewage sludge with 175 kg N ha−1; CRR: crop residue removed; CRI: crop residue incorporated; CT: conventional tillage; SRT: shallow reduced tillage; DRT: deep reduced tillage.
2.1 Study sites
2.1.1 Austria
We used 16 Austrian (AT) sites, selecting contrasting management practices (as treatments) from three different categories of LTEs in areas where management practices had been in place for 11 to 63 years (see details in Table 1). The TBI measurements were made in 2014, 2015 and 2016. Measurements sometimes took place in more than 1 year at the same LTE (e.g., MUBIL), and the sites were abbreviated as AT1 to AT16. Six experiment categories involved carbon balance practices (CB) focusing on organic matter inputs such as compost and crop residue, eight sites were studying soil fertility (SF) in terms of differences in mineral N and P fertilization, and two sites examined the tillage system (TS). The sites are located in several agricultural areas across the country (Fig. 1), with diverse soil textures (Table S1 in the Supplement) and variable crop types (Table 1) and climatic characteristics (Table 3) during the TBI measurement years. More details for some of the sites are available in specific publications: AT3 (Spiegel et al., 2018; Lehtinen et al., 2017; Tatzber et al., 2015; Aichberger and Söllinger, 2009), AT4 to AT6 (Spiegel et al., 2018; Lehtinen et al., 2014), and AT15 and AT16 (Tatzber et al., 2015; Spiegel et al., 2007). In addition, Sandén et al. (2018) puts the Austrian LTEs in the context of other European LTEs.
The purpose of the Austrian C balance LTEs was threefold: (i) to assess the sustainability of stockless vs. livestock-keeping organic farming management on soil and crop traits (AT1–AT2), (ii) to investigate the effects of compost amendments on soil and crops (AT3), and (iii) to compare crop residue incorporation and removal (AT4–AT6). The compost amendment LTE and crop residue incorporation LTEs also included mineral fertilizer application, whereas AT1 and AT2 only focused on different organic fertilizer treatments.
The eight soil fertility LTEs (AT7–AT14) all focused on the effect of mineral fertilizer on soil and crop properties. In most cases, treatments studied different amounts of mineral nitrogen fertilizer, although AT9 and AT12 also investigated the effect of applying different amounts of K fertilizer. Nitrogen fertilizer was applied in four stages and potassium was applied in three stages, according to the Austrian guidelines for fertilizer (BMLFUW, 2017).
For the tillage system experiments, conventional tillage (CT) was compared with deep reduced tillage (DRT) and shallow reduced tillage (SRT). Regular moldboard plowing to 25–30 cm soil depth was applied in the CT treatment, a cultivator was used to a depth of 15–20 cm in autumn in the RT treatment and a rotary driller that loosened the soil to a depth of 5–8 cm was used in MT treatment. The soil was turned over only in the CT treatment, where inversion tillage incorporated the crop residue. Fertilizer application was crop-specific according to the Austrian guidelines for fertilizing (BMLFUW, 2017).
Table 2Experimental sites in Sweden: site acronym; location name; and type of LTE, including its duration, main crop cultivated during the TBI measurements and management treatments.
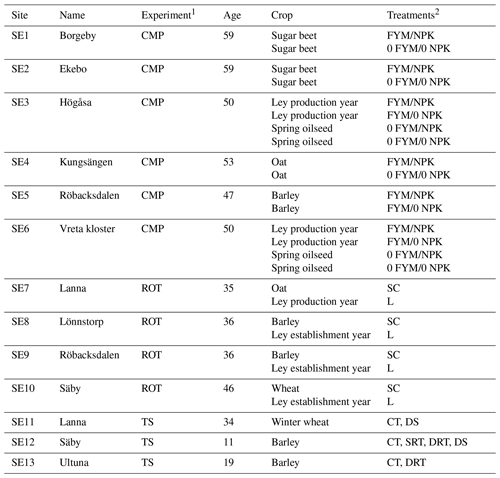
1 CMP: combined management practices; ROT: rotation systems; TS: tillage systems. 2 FYM/NPK: maximum amount of farmyard manure and maximum doses of NPK; 0 FYM/0 NPK: no manure and no NPK application; FYM/0 NPK: maximum amount of farmyard manure and no NPK application; 0 FYM/NPK: no manure and maximum doses of NPK; SC: spring cereal; L: ley; CT: conventional tillage; DS: direct seeding; SRT: shallow reduced tillage; DRT: deep reduced tillage.
Table 3Annual climatic characteristics for the Austrian and Swedish sites during the entire year of measurements and only during the TBI period (shown in days), corresponding to the period between the date of placement and the last retrieval date of the tea bags.
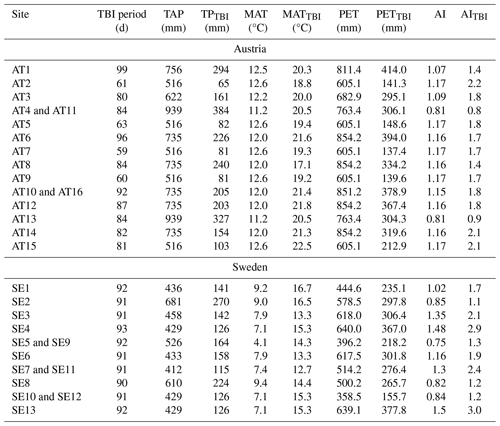
TAP: total annual precipitation; TPTBI: total precipitation during the TBI period; MAT: mean annual temperature; MATTBI: mean temperature during the TBI period; PET: total annual potential evapotranspiration; PETTBI: potential evapotranspiration during the TBI period; AI: annual aridity index (PET divided by TAP); AITBI: aridity index during the TBI period.
2.1.2 Sweden
We used 13 Swedish (SE) sites, selecting contrasting treatments from three different categories of LTEs in areas where the management practices had been in place for 11 to 59 years (see details in Table 2). The TBI measurements at these sites were made in 1 year (2016) and were abbreviated as SE1 to SE13. Six sites involved combined management practices (CMP), four studied the effect of rotation systems (ROT) and three sites had tillage systems (TS). The sites are located in several agricultural areas across the country (Fig. 1), and they had diverse soil textures and variable crop types (Table 2) and climatic characteristics (Table 3) during the year of TBI measurements. Bergkvist and Öborn (2011) give a general description of all of these LTEs. Only a brief description of treatments is provided below. More details on the sites with combined management practices are given by Carlgren and Mattson (2001), more details on sites with tillage systems are provided by Arvidsson and Håkansson (2014), and more insights into the rotation experiments are outlined in Poeplau et al. (2015).
The initial purpose of the six LTEs with combined management practices (SE1–SE6) was to compare the change from a traditional mixed-farming production system, including crops and livestock, into a pure cash crop system, by studying their effects on the sustainability of crop production and soil properties (referred to as soil fertility experiments). The dairy production treatments exclusively contain perennial grass–clover leys and receive one farmyard manure (FYM) application per rotation. The cash crop treatments consist of annual crops (i.e., oilseed replaces leys in the rotation) without manure application (0 FYM) and only receive mineral fertilizer application (NPK, nitrogen–phosphorus–potassium). The PK applications in all of the treatments that we selected were aimed at achieving a rapid buildup of soil PK: the amount applied was initially to replace the PK exported in harvested products (i.e., maintenance principle), and an extra amount was then added to the initial application (corresponding to the maximum treatment). The N rates in all NPK treatments also corresponded to the maximum application rate and were adapted depending on the crop type: spring cereals, oilseeds and leys received 125 kg, whereas sugar beet received 210 kg N ha−1 yr−1. We also used control plots that received no NPK (0 NPK). As a third factor, aboveground crop residue removal takes place in all FYM treatments, simulating the use of harvest residue for fodder or bedding material that is recycled as manure. The southern sites have 4-year rotations, whereas those in central Sweden have 6-year rotations. The northern site (SE5) is slightly different from the others, consisting of a 7-year rotation, and was used to study only the livestock-based production system. For rotation experiment purposes, we compared extreme treatments representing two rotations from four LTEs (SE7–SE10), with the main objective of studying changes in SOC (referred to as humus balance experiments), i.e., a continuous spring cereal (SC) system and ley-dominated rotations (L). The straw was removed from the plots every year in the SC treatments, and L consisted of a grass–clover mixture re-established every fourth year. Both rotations received P and K in accordance with the maintenance principle, and SC and L received 120 and 150 kg N ha−1 yr−1, respectively.
In the three tillage experiments (SE11–SE13), the conventional tillage (CT) and direct seeding (DS) treatments were the same for all sites, consisting of inversion plowing to a depth of 20–23 cm and the use of a disk seed drill, respectively. The shallow (5–7 cm) and deep (∼12 cm) reduced tillage treatments (SRT and DRT, respectively) consisted of primary tillage operations made in the autumn, most commonly with a chisel plow. The main crops in all of the tillage system experiments were winter and spring cereals (occasionally oilseed), fertilized in accordance with local recommendations and with the aboveground residue chopped and left in the field.
2.2 TBI method and sampling design
The TBI method was used according to the protocol established by Keuskamp et al. (2013) to determine litter decomposition using two types of commercial tetrahedron-shaped tea bags by Lipton Unilever (green tea and rooibos tea). The green tea (Camellia sinensis; EAN: 8722700055525) has a high cellulose content, a higher soluble fraction and a lower C:N ratio, whereas the rooibos tea (Aspalathus linearis; EAN: 8722700188438) has a high lignin content, a lower soluble fraction and a higher C:N ratio, which is expected to slow decomposition (Keuskamp et al., 2013). The synthetic tea bag material has a mesh size of 0.25 mm, allowing access to microorganisms, very fine roots and root hairs.
The initial mass of the tea bag contents was determined on 20 randomly selected bags for each tea type from different boxes; the tea bags were oven-dried at 70 °C for 48 h and weighed separately. The tea bag content mean dry mass for green tea was 1.717±0.048 g and that for rooibos tea was 1.835±0.027 g. For both countries, close to seeding of annual crops, from end of April to mid-June depending on location, each tea bag was properly identified and buried in the soil at 8 cm depth.
For Austria, four bags of each tea were used; they were placed side by side at a distance of 2 to 3 cm. Each tea bag was properly identified and buried in the soil at 8 cm depth. The TBI incubation period from placement to last retrieval averaged 80±13 d (Table 3) due to logistic issues regarding collection. After collection and following the removal of adhering soil particles according to the standardized protocol by Keuskamp et al. (2013), the tea bags were oven-dried at 70 °C for 48 h. After drying, the tea bags were opened and the tea content was weighted. The ash content was not determined.
The same TBI protocol was used for the Swedish sites. As in Austria, four bags of each tea were used per experimental unit, and they were placed side by side at a distance of 2 to 3 cm. Each tea bag was properly identified and buried in the soil at 8 cm depth. The mean TBI incubation period from placement to last retrieval averaged 91±1 d (Table 3). Following the removal of adhering soil particles, the ash content was also determined (i.e., for both green and rooibos tea on mixed samples of the four replicates) in a muffle oven at 550 °C for 16 h. The rational for measuring the ash content was that three of the Swedish sites had a high clay content (Table S1), meaning that the complete removal of adhering soil particles may be more difficult. However, the ash content was quite low on average, representing 15±6 % and 10±4 % for the green and rooibos tea bags, respectively (data not shown).
After measuring the remaining dry matter, the decomposition rate (k) and stabilization factor (S) for both countries were calculated according to the TBI, as presented by Keuskamp et al. (2013). This standardized method, which uses single measurements after an incubation period of 90 d in the soil, has received some criticism. For instance, Mori (2022) and Mori et al. (2023) showed that a 90 d incubation period is not always long enough for the mass loss of green tea to reach a plateau, and they further suggested that a mass loss data time series of rooibos tea is also required to respect the underlying assumptions of the TBI method. Time series (15, 30, 60 and 90 d) of both green and rooibos tea were available for all of the Swedish sites but only at one Austrian site (16, 26, 62 and 91 d at AT16). The incubation period was always 90 d for the Swedish sites, and only shorter than that (i.e., about a 60 d incubation period) for a few of the Austrian sites (Table 3). To have comparisons that were as uniform as possible between the two datasets, we only used the last measurement for both countries to calculate k and S. Using the time series to test the underlying TBI assumptions was beyond the scope of this paper.
The daily climate data for Austria were retrieved from the Central Institution for Meteorology and Geodynamics (ZAMG). For Sweden, the daily climate data were gathered from official data from the closest LantMet climate stations as well as from the Swedish Meteorological and Hydrological Institute (SMHI). The climate variables used in this study were air temperature, precipitation, solar radiation, wind speed and air humidity (Table 3).
For Austrian soils, pH was measured electrochemically (pH/mV 340i pocket pH meter; WTW, Weilheim, Germany) in 0.01 M CaCl2 at a soil-to-solution ratio of 1:5 (ÖNORM L1083). Total soil organic C (TOC) concentrations were analyzed by dry combustion in a LECO RC-612 TruMac CN (LECO Corp., St. Joseph, MI, USA) at 650 °C (ÖNORM L1080). Total N (Ntot) was determined according to ÖNORM L1095 with elemental analysis using a CNS (carbon, nitrogen and sulfur) 2000 SGA-410–06 at 1250 °C. Texture was determined according to ÖNORM L1061-1 and L1062-2. For Sweden, the data were gathered from recent archived analysis protocols (pH was measured in water). Clay content, C content, C:N ratio and pH measured at each site in both countries are shown in Table S1.
2.3 Data analysis
An analysis of variance (ANOVA) was performed for each experiment category (i.e., CB, SF, TS, CMP and ROT) to analyze the effects of the treatments and the differences between sites for k and S separately for both countries. When the treatments were identical within the same experiment category, sites were used as a random effect with a mixed ANOVA to test the average treatment effect, while mean values were used as replicates to test the differences between sites. A Tukey test (p<0.05) was used to compare the same treatments and the same sites with R software (version 4.2.2). We treated the data from different years at the same sites in Austria as independent observations, in the sense that they are not a measurement time series. Interactions between site and treatment were considered.
We calculated a climate-dependent soil biological activity parameter (Reclim) using the mean daily air temperature, total precipitation and potential evapotranspiration (PET) data in the pedotransfer, soil water balance and biological activity functions. Compared with raw climatic data alone, this parameter integrates the effects of climate, soil and crop properties. It is calculated as the product of a soil temperature (Retemp) and relative water content (Rewat) factor with a daily time step (i.e., Re), which is thereafter averaged to give an estimate of soil biological activity for a given time period. These two factors are derived from soil temperature and soil moisture response functions expressing the activity of decomposers and their relative effect on the decay rates of organic materials in the arable layer of agricultural soils. Briefly, Retemp is calculated from the air temperature and leaf area index using an empirical model (Kätterer and Andrén, 2009), whereas Rewat is calculated using pedotransfer functions to simulate the soil water balance and a function to estimate PET. In addition to the air temperature and leaf area index, calculations of Rewat also involve the use of daily climatic data for precipitation, wind speed, air humidity and solar radiation; crop type and yield data; soil texture information; and SOC content data. For details, the reader is referred to Bolinder et al. (2008), Fortin et al. (2011), and information in the package documentation of the following R package used for the calculations: https://github.com/ilmenichetti/reclim (last access: 20 June 2022). The Reclim concept can be used to quantify regional differences in soil biological activity alone (Bolinder et al., 2013; Andrén et al., 2007) or it can be integrated as a parameter in the Introductory Carbon Balance Model (ICBM) SOC model. In the latter case, it adjusts the decomposition rates of both C inputs to soil from crop residue (e.g., straw) and those of the more stable SOC (Andrén and Kätterer, 1997; Andrén et al., 2004). In this study, we used the concept of Reclim and the Retemp and Rewat factors to test (1) if the product of soil temperature and relative water content better explained the variation in k and S compared with soil temperature and relative water content separately and (2) if they also better explained this variation compared with using only raw climatic data.
We calculated a simple correlation between the following variables using a Pearson correlation: mean annual temperature (MAT), mean temperature during the incubation period (MATTBI), total annual precipitation (TAP), total precipitation during the incubation period (TPTBI), potential evapotranspiration (PET), potential evapotranspiration during the incubation period (PETTBI), the aridity index (AI), the aridity index during the incubation period (AITBI), a temperature and precipitation factor (T×P), Reclim, Rewat, Retemp, pH, SOC, clay and the C:N ratio. For more accurate results, we applied random forest (RF) regression, using the randomForest R package, in order to rank the importance of variables for k and S (Liaw and Wiener, 2002). RF is a machine learning technique based on decision trees that predicts a certain variable from a set of other variables via a series of binary splits of the data, where the variables are either continuous or categorical. For example, in the case of a continuous variable, it consists of all of the data points above or below a certain threshold, whereas it consists of all of the data points belonging to or not belonging to a specific class for a categorical variable. All of these subsequent splits constitute a decision tree. A random forest is a set of decision trees; therefore, it is an ensemble technique. This allowed us to utilize treatment and crop variables (including N fertilizer) without having to convert them into a ranking. Another useful asset of an RF regression is that it evaluates the importance of each variable in defining the predicted variable. There are various possible measurements to do this, but they are all based on measuring the effectiveness of each subsequent split in each node of a decision tree with respect to sorting the information. In our study, we used a measurement based on the Gini index called node purity, which expresses the probability of one split of the data (i.e., one node of the tree) defining the predicted variable. The total node purity of a certain variable in a tree is the sum of all of the node purity measurements for each node considering that particular variable; the higher the node purity, the more important the variable.
We used the following models to predict the two TBI parameters, k and S:
Here, the soil (continuous) variables were TN (total N, g kg−1), SOC (g kg−1), CN (C:N ratio), cl (clay content, grams per 100 g) and pH. Categorical variables were N (N fertilizer application factor with 4 levels), cr (crop factor, e.g., barley, ley (establishment), ley (production), oat, spring oilseeds, sugar beet, maize and winter wheat) and tr (a treatment factor with 30 levels). The climatic variables (PETTBI, PET, TPTBI, TAP, MATTBI, MAT, AITBI and AI) are as defined in Table 3. The climate response variables Reclim, Rewat and Retemp are as described above.
As many of variables in our model are likely to be correlated and carry similar information, we applied the recursive feature elimination algorithm implemented in the caret R package (Kuhn et al., 2016), which assesses the optimal set of predicting variables (features) to be utilized by the RF model in subsequent iterations. The procedure starts by fitting an RF model with all variables, ranking them by importance and discarding the least important. The algorithm then iterates. The optimal number and set of features are then defined by a fitness metric (in our case, the model R2), selecting the set with the best model fitness. The selected models were used to compute the variables' relative importance.
In order to better understand the similarities between the sites, we run a principal component analysis (PCA) in the space composed by the following variables: pH, SOC, TN, C:N, cl, TAP, MAT, PET, AI and T×P. The analysis was run with the R prcomp command (Venables and Ripley, 2002) from the base R installation (stats package). Variables were all standardized by rescaling them to zero mean and unit variance.
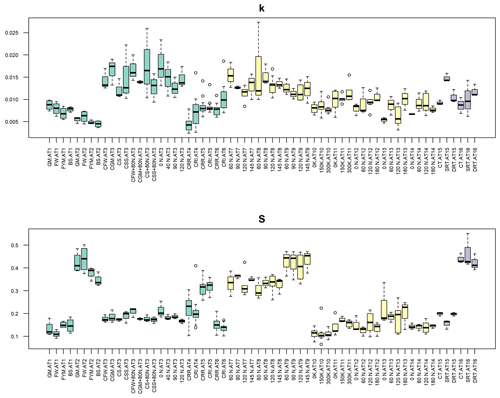
Figure 2Average decomposition rate (k) and stabilization factor (S) for each treatment and site in Austria. The extents of the box indicate the 25th and 75th percentiles, the lines represent the 50th percentile, whiskers represent the 10th and 90th percentiles, and outliers are given as open symbols. The figure is color-coded as follows: green boxes – carbon balance (CB) experiment; yellow boxes – soil fertility (SF) experiment; purple boxes – tillage system (TS) experiment. Site AT1 shows results from 2014; sites AT2, AT3, AT5, AT7, AT9 and AT15 show results from 2015; and sites AT4, AT6, AT8, AT10, AT11, AT12, AT13, AT14 and AT16 show results from 2016.
3.1 Effect of management practices
3.1.1 Austria
Both of the TBI parameters, k and S, varied between treatments and sites in Austria, and they even varied between years at the same site within the C balance category (Fig. 2, Table S2). In general, all treatments at AT1, which had a lucerne crop grown under wetter conditions (2014), showed a higher k and lower S than at AT2, which had a wheat crop grown under dryer conditions (2015), and the treatment with municipal compost and green manure (FW) had the highest S in 2015. The AT3 site did not show significant differences between the treatments. The crop residue incorporation treatment (CRI) had a higher k than the crop residue removal treatment (CRR) at the AT4 and AT6 sites, and AT6 showed a higher k than at AT4. Comparing years for the same experiment site and type with different crops, AT5 (2015; with wheat) had a higher S than AT6 (2016; with maize).
For the soil fertility experiment category (Table 1), AT12 had the highest k and AT13 had the highest S. Sites receiving NPK fertilizer application (AT7, AT8 and AT9) had a higher k and S (even at different N doses) than at the AT12, AT13 and AT14 sites receiving only N (i.e., without P and K). Stabilization was significantly higher at AT9 than at AT7. For potassium trials, AT11 showed a significantly higher k and S than AT10. Regarding sites receiving only N addition (AT12, AT13 and AT14), maximum doses (180 kg N) showed the highest k (0.0095), and no N addition had the lowest k (0.0066) (Fig. 2, Table S2).
With respect to the tillage system experiment category (AT15 and AT16; Table 1), k was significantly higher in the shallow reduced tillage treatment (SRT), whereas S was higher in the deep reduced tillage treatment (DRT) in 2015 (AT15), but no significant differences between treatments were found in 2016 (AT16). Site AT16 had a significantly higher S than site AT15 (Fig. 2, Table S2).
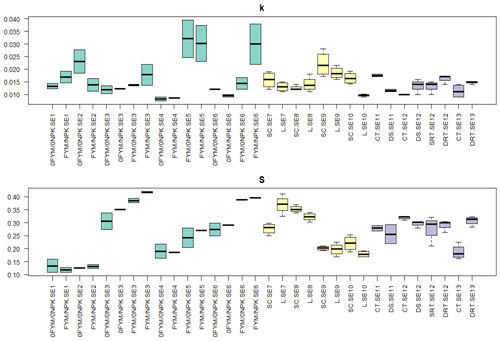
Figure 3Average decomposition rate (k) and stabilization factor (S) for each site and treatment in Sweden. The extents of the box indicate the 25th and 75th percentiles, the lines represent the 50th percentile, and whiskers represent the 10th and 90th percentiles. The figure is color-coded as follows: green boxes – combined management practice (CMP) experiment; yellow boxes – rotation (ROT) experiment; purple boxes – tillage system (TS) experiment.
3.1.2 Sweden
At the Swedish sites (Table 2), the TBI measurements for the combined management practices experiment category showed that both k and S were significantly higher for the maximum amount of farmyard manure and maximum doses of NPK (FYM/NPK) treatments (Fig. 3, Table S3) compared with the control treatments (0 FYM/0 NPK). Comparing sites, SE5 and SE6 had the highest k and SE4 had the lowest k, while SE3 showed the highest S followed by SE6, SE5 and SE4. The lowest S was found for SE1 and SE2 (Table S3).
Regarding the rotation experiments, the continuous spring cereal rotation showed higher k than for ley, but there was no significant difference in S. Comparing sites, SE9 showed higher k than SE7, SE8 and SE10, whereas SE7 and SE8 had the highest S.
For the tillage system experiment category, conventional tillage (CT) had the lowest k and S, whereas deep reduced tillage (DRT) had the highest k and S. The highest S was observed for the SE12 site, followed by SE11 and SE13. Sites did not show significant differences with respect to k.
Comparing tillage system experiments in Austria and Sweden (2016) for sites (AT16, SE11, SE12 and SE13) for the treatments that were the same in both countries (CT, SRT and DRT), deep reduced tillage had the highest k, while shallow reduced tillage had the highest S. The Austrian sites showed the lowest k and the highest S compared with the Swedish sites, the latter of which did not show significant differences among them (Table S3).
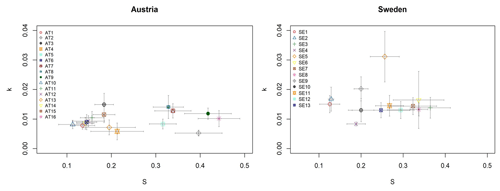
Figure 4Distribution of the mean decomposition rate constant (k) and the stabilization factor (S) for each site in Austria and Sweden. Error bars represent standard deviation.
Table 4Mean decomposition rate (k) and stabilization factor (S) values for the TBI approach after the incubation period.
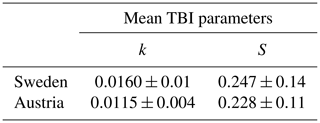
The field application of the TBI found a clear discrimination with respect to both k and S values between the two countries. The mean k and S values were both higher in Sweden (Fig. 4, Table 4). In general, the variation in the k and S values was lower in Austria. Indeed, mean k by site in Austria varied between 0.0058 and 0.0128, while mean S varied between 0.113 and 0.442 (Table S2). In contrast, mean k by site in Sweden varied between 0.0084 and 0.0301, while mean S varied between 0.118 and 0.361 (Table S3). All values for k and S were within the range (0.005–0.04 for k; 0.05–0.55 for S) of a previous global TBI investigation by Sandén et al. (2020).
3.1.3 Influence of climate and soil properties
Using the combined dataset for Austria and Sweden resulted in a significant negative correlation between k and MAT, TAP, PET, the T×P factor, Reclim and Retemp as well as a significant positive correlation with the C:N ratio (Table S4). Stabilization factors in Austria and Sweden combined correlated negatively with the MATTBI period, Reclim and Retemp.
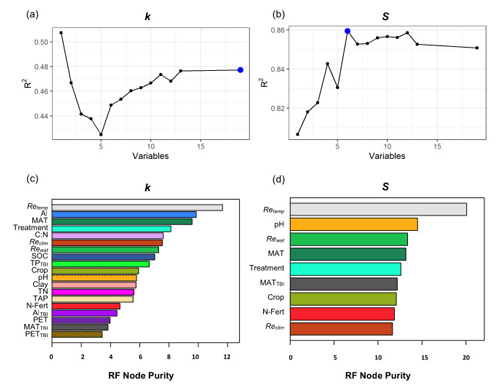
Figure 5(a, b) Variable selection procedure to identify the optimal number of variables to explain the variance in k and S with a random forest model. The blue point represents the optimal model. (c, d) Relative importance of the variables used by each optimized random forest model to predict the variance in the k and S parameters in Austria and Sweden jointly. The higher the node purity, the higher the importance of the variable.
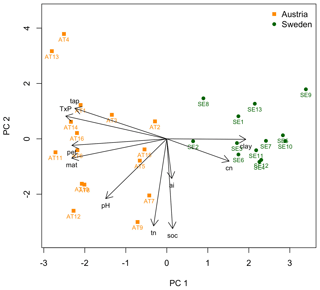
Figure 6The principal component analysis showing how the sites in Austria and Sweden differ based on the variables. PC1 and PC2 are the first two components, explaining most variance. The loadings (black arrows) are the weight of each variable in defining each principal component. The sizes of the arrows denote how much they contribute to defining this space, while the direction is their contribution on each axis. The abbreviations used in the figure are as follows: tap – total annual precipitation; T×P – temperature × precipitation factor; pet – potential evapotranspiration; mat – mean annual temperature; tn – total soil nitrogen; soc – total soil organic carbon; cn – soil C:N ratio; ai – aridity index.
The variable selection procedure with the random forest models (Fig. 5) identified fewer variables explaining k and S values for the combined dataset. The variable explaining k is low, but the overall predictive power of the model decreased substantially.
According to the optimized random forest model, most of the variance in k was accounted for by climatic variables (Fig. 5), with Retemp ranking the highest, followed by AI and MAT. It was also found that treatment, C:N ratio and SOC were important factors. Moreover, S was influenced by climate-related variables, with Retemp leading the ranking, followed by pH and more agronomic variables, such as treatment, crop and N fertilizer application.
The principal component analysis (Fig. 6) revealed that the data are well divided into two groups that represent the two countries; this enables us to say that edapho-climatic characteristics are indeed different between Austria and Sweden. PC1 showed that points in Austria are positively related to climatic characteristics, such as MAT, TAP and PET. On the other hand, points in Sweden are positively influenced by the high clay content and high C:N ratio. A high C:N ratio enhancement is related to low TAP and T×P. High clay contents are related to low MAT and PET, which can be due to the historical influence of soil weathering. PC2 was dominated by the SOC and TN contents, mainly at two points in Austria (AT7 and AT9: two sites testing soil fertility with NPK and relatively low TAP and TPTBI compared with other sites). Austria showed more divergent data, with more heterogeneous sites than in Sweden.
4.1 Influence of climate and soil properties
In previous studies using the TBI approach, it has been shown that climate plays a significant role on decomposition in a temperate biome (Djukic et al., 2018); however, when comparing several different biomes, climatic conditions were of relatively low importance (Fanin et al., 2020). In boreal soils, Althuizen et al. (2018) found that increased temperatures enhanced k, whereas increased precipitation decreased k across years. Many studies have shown a positive correlation between precipitation and decomposition rates (Pimentel et al., 2019; García Palacios et al., 2016); however, precipitation did not show a correlation with k according to the random forest analysis. On the other hand, Rewat showed great importance (Fig. 5). This is because Rewat includes nonlinearities due to its shape, according to which decomposition increases with soil moisture and then decreases at a high soil water content due to the oxygen limitation of microorganisms (Moyano et al., 2013).
In general, higher k values were observed when the aridity index was lower, and the AI was identified by the random forest regression model as being an important variable affecting the rate of decomposition (Fig. 5). This may be related to the observations that the biological processes driving SOC dynamics at more arid and warmer sites are impaired, possibly resulting in decreasing soil C and N stocks (Kerr and Ochsner, 2020; Ontl and Schulte, 2012; Jiao et al., 2016; Reynolds et al., 2007).
For practical reasons, the tea bags in our study were buried in the soil during the growing season, corresponding to a period when the soil biological activity is highest (Bolinder et al., 2013). When burying the tea bags during the growing season, the difference in climatic variables between sites is attenuated, in particular with respect to air temperature. For example, the MAT at the most northerly (SE5 and SE9) and southerly (SE2) sites in Sweden are 4.1 and 9.0 °C, respectively, whereas the corresponding mean air temperatures during the incubation period (MATTBI) were 14.3 and 16.2 °C, respectively.
The TBI S parameter was also dependent on climate. Indeed, the random forest model identified climatic parameters as the main factors affecting S in both Austria and Sweden during all of the evaluated periods. In particular, Reclim and Retemp often showed significant negative correlations, implying a negative impact of air temperature on S. However, raw climatic variables, such as precipitation and temperature, were only weakly correlated with S. This is probably due to nonlinear processes, which are considered in the ICBM climate-dependent soil biological activity calculations, such as Rewat (as discussed above). Furthermore, as litter decomposition dynamics is influenced by multiple factors that interact and change over time (Bradford et al., 2016), the relationships are not always linear. The random forest models that we fitted to the data are more efficient at capturing such combinations and interactions of factors, and they can detect relationships that would not be detectable by linear approaches.
4.2 Effect of management practices
Our results revealed that a large number of management practices significantly affected both the decomposition rate (k) and stabilization factor (S), according to the TBI approach, in several LTEs in Austria and Sweden (Figs. 2, 3, 5). This is in contrast with studies by Djukic et al. (2018) and Saint-Laurent and Arsenault-Boucher (2020), who did not find any significant effect of land use or management practice on early-stage litter decomposition in a temperate biome.
In the C balance trials in Austria, soils receiving green manure and municipal compost had a higher S than soil receiving a biogas slurry in the second year (Table S2). This is in agreement with studies indicating that compost can improve SOC stabilization over time (Mekki et al., 2019; Eshetu et al., 2013; Ceccanti et al., 2007). The higher k in the treatments incorporating crop residue (AT4 and AT6; Table S2) can be attributed to the fact that the incorporation of crop residue into the soil can increase the decomposition rate by stimulating microbial activity. During the early stages of decomposition, soluble C is rapidly utilized by soil biota (Werth and Kuzyakov, 2010). The higher k and lower S values at AT6 compared with AT4 were likely due to the loamy texture and lower potential evapotranspiration, resulting in a lower aridity index at the AT4 site (Tables 3, S1), as corroborated by the PCA analysis (Fig. 6).
There were no significant differences in k and S found among treatments in the soil fertility trials in Austria with NPK addition. However, there was a trend towards a higher S at AT9 compared with AT7, which was likely related to the higher SOC content at AT9, as the climatic conditions and soil texture were quite similar for both areas, suggesting that a higher SOC content may have increased S. Site AT11 had higher k and S values than AT10. Possible explanations for this trend are that AT10 had a lower clay content and lower precipitation, resulting in higher PET (corroborated by the PCA analysis) and AI, contributing to a lower soil moisture content and, thus, lower decomposition and stabilization.
Nitrogen supply may favor microbial activity and, therefore, litter decomposition (Raiesi, 2004). This was reflected in the treatments in which only N was added, as the high dose of 180 kg N ha−1 (AT12, AT13 and AT14) induced a significantly higher k (Table S2) compared with the treatments with no N addition (which also had the lowest k). Furthermore, the significant difference between sites (AT12 had the highest k) could at least partly be explained by a higher SOC and higher pH at the site (corroborated by the PCA analysis).
With respect to the tillage system experiment at Fuchsenbigl in Austria in 2015 (AT15; with maize) and 2016 (AT16; with wheat), the shallow reduced tillage only showed significantly higher k values than deep reduced tillage and conventional tillage in 2015, indicating that shallow soil tillage stimulated decomposition in that particular year. This was likely due to climatic conditions, as 2015 was slightly drier and warmer; furthermore, maize straw has a lower C:N ratio than wheat straw and tends to decompose faster. Some studies showed faster decomposition under conventional tillage than under reduced tillage conditions (e.g., Lupwayi et al., 2004). However, Kainiemi et al. (2015) found a decrease in soil respiration for conventional tillage compared with shallow tillage in temperate regions, which directly implies lower decomposition (and lower k). These differences between tillage treatments are attributable to indirect effects on soil moisture and temperature profiles. We attribute the significantly higher S in 2016 to the fact that this year was moister and less warm than 2015, resulting in a lower aridity index during the TBI incubation period (AITBI).
In the Swedish combined management practices trials, treatments receiving organic and mineral fertilizer application had higher k and S values (FYM/NPK; Table S3), likely due to the increase in microbial diversity and activity favored by nutrient and C supply (Stark et al., 2007). Sites SE5 and SE6 showed the highest k: SE5 had low evapotranspiration and a low aridity index, resulting in more moisture; SE6 also had a high S, due to a high clay content and low PET (corroborated by the PCA analysis). Site SE3 had a high S, which could be related to a higher C:N ratio, as suggested in another TBI experiment by Althuizen et al. (2018), who showed that the C:N ratio is positively correlated with S. The SE1 and SE2 sites had a lower S than SE3, despite similar climatic conditions, which was probably related to the type of crops growing at these sites (i.e., sugar beet at SE1 and SE2; grass–clover ley at SE3), as these two crop types have different effects on soil temperature and moisture.
In the Swedish rotation system trials, spring cereal (SC) had a higher k than ley (Table S3). Site SE9 had a higher k and lower S; this low stabilization may be caused by a low clay content, low pH and high solar radiation, leading to low SOC (corroborated by the PCA analysis). The highest S values were found at sites SE7 and SE8, where the former showed a high clay and SOC content, while the latter had high precipitation and low PET.
For the tillage system treatments in Sweden, similar to the Austrian sites, conventional tillage showed the lowest k as well as the lowest S. Even when comparing tillage systems in Sweden and Austria jointly (Table S3), we noticed that conventional tillage showed the lowest k, whereas deep reduced tillage showed the highest values.
As expected, the decomposition rate (k) was mostly affected by climatic conditions, such as temperature and aridity or moisture. However, agronomic and edaphic factors (i.e., soil management choice (treatment), soil C:N ratio, SOC, crop type, pH, clay content, soil nitrogen and others) proved to be of great relevance for k. The stabilization factor (S) expresses the degree to which the labile fraction of the plant material is decomposed. We observed that the influence of pH was the most important edaphic factor and that the agronomic factors, such as the soil management treatment, crop type and N fertilizer application, were also good predictors. A study conducted by Fu et al. (2021) suggested that pH, nutrient availability and soil compaction were the main factors contributing to the differences in litter decomposition. The net effect of pH is not clear, as it modifies both SOC decay kinetics and productivity simultaneously (Paradelo et al., 2015), with a maximum effect at around neutral pH (Liao et al., 2016). Nevertheless, the impact of pH on litter decomposition using the TBI approach seems clear in our study.
Our results show that both the TBI k and S parameters are sensitive to management practices in agroecosystems in Austria and Sweden. We observed significant differences for some of the treatments in all LTE categories. Notably, for the effect of crop residue incorporation, organic amendment and N fertilizer application, crop type, and tillage systems. In the Austrian LTEs, the application of green manure and municipal compost showed a higher S compared with the application of other organic amendments. The incorporation of crop residue instead of crop residue removal as well as high-N fertilizer instead of low or no N application increased the decomposition rate (k). In the Swedish LTEs, it was shown that combined management practices with both farmyard manure and mineral NPK resulted in higher k and S values compared with no manure and no NPK application, whereas growing spring cereals instead of leys increased k but did not change S. For both countries, tillage systems with deep reduced tillage practices had higher k values, whereas shallow reduced tillage had higher S values. However, these effects were also dependent on site or year within a given country. Climatic conditions had the most important impact on the decomposition rate (k) and the stabilization factor (S), but pH, treatment, crop type, SOC, C:N ratio and clay content were also good predictors of the TBI parameters. Generally, the correlations with raw climatic variables, such as precipitation and temperature, were quite poor. Better relationships were found when nonlinearities due to interactions between climatic and edaphic conditions were accounted for. The results highlight how, in addition to soil and climatic conditions, a wide range of management practices used in agroecosystems affect TBI parameters. This also suggests that the TBI k and S parameters could serve as indicators of how different agricultural management practices influence the global carbon cycle via decomposition. This is a matter requiring further in-depth investigation.
Data are available from the authors upon reasonable request.
The supplement related to this article is available online at: https://doi.org/10.5194/soil-10-407-2024-supplement.
MRG, MAB and TS wrote and prepared the manuscript draft; MAB, TK and TS supervised and led the research; MAB, OA, HS, JKF, AnS, AgS and TS developed the methodology; and MRG and LM analyzed the data. All authors reviewed and edited the manuscript.
The contact author has declared that none of the authors has any competing interests.
Publisher’s note: Copernicus Publications remains neutral with regard to jurisdictional claims made in the text, published maps, institutional affiliations, or any other geographical representation in this paper. While Copernicus Publications makes every effort to include appropriate place names, the final responsibility lies with the authors.
The authors are grateful to the Swedish Faculty of Natural Resources and Agricultural Sciences and the Austrian Agency for Health and Food Safety (AGES), for providing resources to maintain the long-term field experiments, and to present and former colleagues, for their engagement in these experiments.
In Austria, this research has partly been supported by the European Union's Horizon 2020 Research and Innovation program (grant no. 862695); the MUBIL trial (AT1 and AT2) was financially supported by the Austrian Ministry of Agriculture, Forestry, Regions and Water Management. In Sweden, financial support was provided by the Swedish Farmers' Foundation for Agricultural Research (grant no. O-18-23-141).
The publication of this article was funded by the Swedish Research Council, Forte, Formas and Vinnova.
This paper was edited by Ingrid Lubbers and reviewed by Ute Hamer, Moritz Laub, and two anonymous referees.
Aichberger, K. and Söllinger, J.: Use of biocompost in agriculture – results of a long-term field trial, in: Realising the ETAP in the management of waste from farms, edited by: Spiegel, H. and Zonno, V., Proceedings of the second AQUAGRIS workshop Vienna, 19 June 2009, Vienna, AGES, 6–8, 2009.
Althuizen, I. H. J., Lee, H., Sarneel, J. M., and Vandvik, V.: Long-term climate regime modulates the impact of short-term climate variability on decomposition in Alpine grassland soils, Ecosystems, 21, 1580–1592, https://doi.org/10.1007/s10021-018-0241-5, 2018.
Andrén, O. and Kätterer, T.: ICBM: The introductory carbon balance model for exploration of soil carbon balances, Ecol. Appl., 7, 1226–1236, https://doi.org/10.2307/2641210, 1997.
Andrén, O., Kätterer, T., and Karlsson, T.: ICBM regional model for estimations of dynamics of agricultural soil carbon pools, Nutr. Cycl. Agroecosys., 70, 231–239, https://doi.org/10.1023/B:FRES.0000048471.59164.ff, 2004.
Andrén, O., Kihara, J., Bationo, A., Vanlauwe, B., and Kätterer, T.: Soil climate and decomposer activity in Sub-Saharan Afrika estimated from standard weather station data: A simple climate index for soil carbon balance calculations, Ambio, 36, 379–386, https://doi.org/10.1579/0044-7447(2007)36[379:scadai]2.0.co;2, 2007.
Arvidsson, J. and Håkansson, I.: Response of different crops to soil compaction–Short-term effects in Swedish field experiments, Soil Till. Res., 138, 56–63, https://doi.org/10.1016/j.still.2013.12.006, 2014.
Barel, J. M., Kuyper, T. W., Paul, J., de Boer, W., Cornelissen, J. H. C., and De Dein, G. B.: Winter cover crop legacy effects on litter decomposition act through litter quality and microbial community changes, J. Appl. Ecol., 56, 132–143, https://doi.org/10.1111/1365-2664.13261, 2019.
Bergkvist, G. and Öborn, I.: Long-term field experiments in Sweden–what are they designed to study and what could they be used for, Aspects of Applied Biology, 113, 75–85, 2011.
BMLFUW: Richtlinien für die sachgerechte Düngung. Bundesministerium für Land- und Forstwirtschaft, Umwelt und Wasserwirtschaft, 2017.
Bocock, K. L. and Gilbert, O. J. W.: The disappearance of leaf litter under different woodland conditions, Plant Soil, 9, 179–185, https://doi.org/10.1007/BF01398924, 1957.
Bolinder, M. A., Andrén, O., Kätterer, T., and Parent, L. E.: Soil organic carbon sequestration potential for Canadian Agricultural Ecoregions calculated using the Introductory Carbon Balance Model, Can. J. Soil Sci., 88, 451–460, https://doi.org/10.4141/CJSS07093, 2008.
Bolinder, M. A., Fortin, J. G., Anctil, F., Andrén, O., Kätterer, T., de Jong, R., and Parent, L. E.: Spatial and temporal variability of soil biological activity in the Province of Québec, Canada (45–58 °N, 1960–2009) – calculations based on climate records, Climatic Change, 117, 739–755, https://doi.org/10.1007/s10584-012-0602-6, 2013.
Bolinder, M. A., Janzen, H. H., Gregorich, E. G., Angers, D. A., and VandenBygaart, A. J.: An aproach for estimating net primary productivity and annual carbon inputs to soil for common agricultural crops in Canada, Agr. Ecosyst. Environ., 118, 29–42, https://doi.org/10.1016/j.agee.2006.05.013, 2007.
Bradford, M. A., Berg, B., Maynard, D. S., Wieder, W. R., and Wood, S. A.: Understanding the dominant controls on litter decomposition, J. Ecol., 104, 229–38, https://doi.org/10.1111/1365-2745.12507, 2016.
Buchholz, J., Querner, P., Paredes, D., Bauer, T., Strauss, P., Guernion, M., Scimia, J., Cluzeau, D., Burel, F., Kratchmer, S., Winter, S., Pothhof, M., and Zaller, J. G.: Soil biota in vineyards are more influenced by plants and soil quality than by tillage intensity or the surrounding landscape, Sci. Rep.-UK, 7, 17445, https://doi.org/10.1038/s41598-017-17601-w, 2017.
Bünemann, E. K., Bongiorno, G., Bai, Z., Creamer, R., de Deyn, G. B., Goede, R., Fleskens, L., Geissen, V., Kuyper, T. W., Mäder, P., Pulleman, M., Sukkel, W., van Groenigen, J. W., and Brussaard, L.: Soil quality – A critical review, Soil Biol. Biochem., 120, 105–125, https://doi.org/10.1016/j.soilbio.2018.01.030, 2018.
Burgess, M. S., Mehuys, G. R., and Madramootoo, C. A.: Decomposition of grain-corn residues (Zea mays L.): A litterbag study under three tillage systems, Can. J. Soil Sci., 82, 127–138, https://doi.org/10.4141/S01-013, 2002.
Carlgren, K. and Mattsson, L.: Swedish soil fertility experiments, Acta Agr. Scand., 51, 49–76, https://doi.org/10.1080/090647101753483787, 2001.
Ceccanti, B., Masciandaro, G., and Macci, C.: Pyrolysis-gas chromatography to evaluate the organic matter quality of a mulched soil, Soil Till. Res., 97, 71–78, https://doi.org/10.1016/j.still.2007.08.011, 2007.
Cleveland, C. C., Reed, S. C., Keller, A. B., Nemergut, D. R., O'Neill, S. P., Ostertag, R., and Vitousek, P. M.: Litter quality versus soil microbial community controls over decomposition: a quantative analysis, Oecologia, 174, 283–294, https://doi.org/10.1007/s00442-013-2758-9, 2014.
Costantini, E. A. C., Castaldini, M., and Diago, M. P.: Effects of soil erosion on agroecosystem services and soil functions: A multidisciplinary study in nineteen organically farmed European and Turkish vineyards, J. Environ. Manage., 223, 614–624, https://doi.org/10.1016/j.jenvman.2018.06.065, 2018.
Daebeler, A., Petrová, E., Kinz, E., Grausenburger, S., Berthold, H., Sandén, T., Angel, R., and the high-school students of biology project groups I, II, and III from 2018–2019: Pairing litter decomposition with microbial community structures using the Tea Bag Index (TBI), SOIL, 8, 163–176, https://doi.org/10.5194/soil-8-163-2022, 2022.
Davidson, E. A., Janssens, I. A., and Luo, Y.: On the variability of respiration in terrestrial ecosystems: moving beyond Q10, Glob. Change Biol., 12, 154–164, https://doi.org/10.1111/j.1365-2486.2005.01065.x, 2006.
Djukic, I., Kopfer-Rojas, S., Schmidt, I. K., Larsen, K. S., Beier, C., Berg, B., and Verheyen, K.: Early stage litter decomposition across biomes, Sci. Total Environ., 628–629, 1369–1394, https://doi.org/10.1016/j.scitotenv.2018.01.012, 2018.
Dossou-Yovo, W., Parent, S. E., Ziadi, N., Parent, E., and Parent, L. E.: Tea Bag Index to assess carbon decomposition rate in cranberry agroecosystems, Soil Syst., 5, 44, https://doi.org/10.3390/soilsystems5030044, 2022.
Eshetu, B., Baum, C., and Leinweber, P.: Compost of different stability affects the molecular composition and mineralization of soil organic matter, Open J. Soil Sci., 3, 58–69, https://doi.org/10.4236/ojss.2013.31007, 2013.
Fanin, N., Bezaud, S., Sarneel, J. M., Cecchini, S., Nicolas, M., and Augusto, L.: Relative importance of climate, soil and plant functional traits during the early decomposition stage of standardized litter, Ecosystems, 23, 1004–1018, https://doi.org/10.1007/s10021-019-00452-z, 2020.
Fortin, J. G., Bolinder, M. A., Anctil, F., Kätterer, T., Andrén, O., and Parent, L. E.: Effects of climatic data low-pass filtering on the ICBM temperature- and moisture-based soil biological activity factors in a cool and humid temperate climate, Ecol. Model., 222, 3050–3060, https://doi.org/10.1016/j.ecolmodel.2011.06.011, 2011.
Fu, Y., Jonge, L. W., Greve, M. H., Arthur, E., Moldrup, P., Norgaard, T., Paradelo, M.: Linking litter decomposition to soil physicochemical properties, gas transport, and land use, Soil physics and hydrology, Soil Sci. Soc. Am. J., 86, 34–46, https://doi.org/10.1002/saj2.20356, 2021.
García Palacios, P., Shaw, E. A., Wall, D. H., and Hättenschwiler, S.: Temporal dynamics of biotic and abiotic drivers of litter decomposition, Ecol. Lett., 19, 554–563, https://doi.org/10.1111/ele.12590, 2016.
Gholz, H. L., Wedin, D. A., Smitherman, S. M., Harmon, M. E., and Parton, W. J.: Long-term dynamics of pine and hardwood litter in contrasting environments: toward a global model of decomposition, Glob. Change Biol., 6, 751–765, https://doi.org/10.1046/j.1365-2486.2000.00349.x, 2000.
IPCC: Agriculture, forestry and other land use, IPCC guidelines for national greenhouse gas inventories, edited by:: Eggelston, S., Buendia, L., Miwa, K., Ngara, T., and Tanebe, K., Institute for Global Environmental Strategies, prepared by the National Greenhouse Gas Inventories Programme, Hayama, Kanagawa, Japan, ISBN 4-88788-032-4, 2006.
Janzen, H. H.: Beyond carbon sequestration: soil as conduit of solar energy, Eur. J. Soil Sci., 66, 19–32, https://doi.org/10.1111/ejss.12194, 2015.
Jiao, F., Shi, X. R., Han, F. P., and Yuan Z. Y.: Increasing aridity, temperature and soil pH induce soil C-N-P imbalance in grasslands, Sci. Rep.-UK, 6, 19601, https://doi.org/10.1038/srep19601, 2016.
Kainiemi, V., Arvidsson, J., and Kätterer, T.: Effects of autumn tillage and residue management on soil respiration in a long-term field experiment in Sweden, J. Plant Nutr. Soil Sci., 178, 189–198, https://doi.org/10.1002/jpln.201400080, 2015.
Kampichler, C. and Bruckner, A.: The role of microarthropods in terrestrial decomposition: a meta-analysis of 40 years of litterbag studies, Biol. Rev., 84, 375–89, https://doi.org/10.1111/j.1469-185X.2009.00078.x, 2009.
Kätterer, T. and Andrén, O.: Predicting daily soil temperature profiles in arable soils in cold temperate regions from air temperature and leaf area index, Acta Agr. Scand., 59, 77–86, https://doi.org/10.1080/09064710801920321, 2009.
Kätterer, T. and Bolinder, M. A.: Chapter 15: Agriculture practices to improve soil carbon sequestration in upland soil, in: Understanding and fostering soil carbon sequestration, edited by: Rumpel Dr., C., https://doi.org/10.19103/AS.2022.0106.15, 2022.
Kätterer, T., Bolinder, M. A., Berglund, K., and Kirchmann, H. J.: Strategies for carbon sequestration in agricultural soils in northern Europe, Acta Agr. Scand., 62, 181–198, https://doi.org/10.1080/09064702.2013.779316, 2012.
Kerr, D. D. and Ochsner, T. E.: Soil organic carbon more strongly related to soil moisture than soil temperature in temperate grassland, Soil Sci. Soc. Am. J., 84, 587–596, https://doi.org/10.1002/saj2.20018, 2020.
Keuskamp, J. A., Dingemans, B. J., Lehtinen, T., Sarneel, J. M., and Hefting, M. M.: Tea bag index: a novel approach to collect uniform decomposition data across ecosystems, Methods Ecol. Evol., 4, 1070–1075, https://doi.org/10.1111/2041-210X.12097, 2013.
Kuhn, M., Wing J., Weston, S., Williams, A., Keefer, C., Engelhardt, A., Cooper, T., Mayer, Z., Kenkel, B., Benesty, M., Lescarbeau, R., Ziem, A., Scrucca, L., Tang, Y., and Candan, C.: Classification and Regression Training, R package version 6.0-71, https://CRAN.R-project.org/package=caret (last access: 20 June 2022), 2016.
Lal, R.: Soil carbon sequestration impacts on global climate change and food security, Science, 304, 1623–1627, https://doi.org/10.1126/science.1097396, 2004.
Lehtinen, T., Dersch, G., Söllinger, J., Baumgarten, A., Schlatter, N., Aichberger, K., and Spiegel, H.: Long-term amendment of four different compost types on a loamy silt Cambisol: impact on soil organic matter, nutrients and yields, Arch. Agron. Soil Sci., 63, 663–673, https://doi.org/10.1080/03650340.2016.1235264, 2017.
Lehtinen, T., Schlatter, N., Baumgarten, A., Bechini, L., Krüger, J., Grignani, C., Zavattaro, L., Costamagna, C., and Spiegel, H.: Effect of crop residue incorporation on soil organic carbon and greenhouse gas emissions in European agricultural soils, Soil Use Manage., 30, 524–538, https://doi.org/10.1111/sum.12151, 2014.
Liao, K., Wu, S., and Zhu, Q.: Can Soil pH Be Used to Help Explain Soil Organic Carbon Stocks?, Clean Soil Air Water, 44, 1685–1689, https://doi.org/10.1002/clen.201600229, 2016.
Liaw, A. and Wiener, M.: Classification and Regression by randomForest, R News, 2, 18–22, https://CRAN.R-project.org/doc/Rnews/Liaw and Wiener (last access: 20 June 2022), 2002.
Lupwayi, N. Z., Clayton, G. W., O'Donovan, J. T., Harker, K. N., Turkington, T. K., and Rice, W. A.: Decomposition of crop residues under conventional and zero tillage, Can. J. Soil Sci., 84, 403–410, https://doi.org/10.4141/S03-082, 2004.
Mekki, A., Aloui, F., and Sayadi, S.: Influence of biowaste compost amendment on soil organic carbon storage under arid climate, Japca J Air Waste Ma, 69, 867–877, https://doi.org/10.1080/10962247.2017.1374311, 2019.
Minasny, B., Malone, B. P., McBratney, A. B., Angers, D. A., Arrouays, D., Chambers, A., Chaplot, V., Chen, Z-S., Cheng, K., Das, B. S., Field, D. J., Gimona, A., Hedley, C. B., Hong, S. Y., Mandak, B., Marchant, B. P., Martin, M., McConkey, B. G., Mulder, V. L., O’Rourke, S., and Winowiecki, L.: Soil carbon 4 per mille, Geoderma, 292, 59–86, https://doi.org/10.1016/j.geoderma.2017.01.002, 2017.
Mori, T.: Validation of the Tea Bag Index as a standard approach for assessing organic matter decomposition: A laboratory incubation experiment, Ecol. Indic., 141, 109077, https://doi.org/10.1016/j.ecolind.2022.109077, 2022.
Mori, T., Ono, K., and Sakai, Y.: Testing the Tea Bag Index as a potential indicator for assessing litter decomposition in aquatic ecosystems, Ecol. Indic., 152, 110358, https://doi.org/10.1016/j.ecolind.2023.110358, 2023.
Moyano, F. E., Manzoni, S., and Chenu, C.: Responses of soil heterotrophic respiration to moisture availability: An exploration of processes and models, Soil Biol. Biochem., 59, 72–85, https://doi.org/10.1016/j.soilbio.2013.01.002, 2013.
Ontl, T. A. and Schulte, L. A.: Soil carbon storage, Nature Education Knowledge, 3, 35, 2012.
Paradelo, R., Virto, I., and Chenu, C.: Net effect of liming on soil organic carbon stocks: A review, Agr. Ecosyst. Environ., 202, 98–107, https://doi.org/10.1016/j.agee.2015.01.005, 2015.
Paustian, K., Lehmann, J., Ogle, S., Reay, D., Robertson, G. P., and Smith, P.: Climate-smart soils, Nature, 532, 49–57, https://doi.org/10.1038/nature17174, 2016.
Pimentel, L. G., Cherubin, M. R., Oliveira, D. M., Cerri, C. E., and Cerri, C. C.: Decomposition of sugarcane straw: Basis for management decisions for bioenergy production, Biomass Bioenerg., 122, 133–144, https://doi.org/10.1016/j.biombioe.2019.01.027, 2019.
Poeplau, C., Kätterer, T., Bolinder, M. A., Börjesson, G., Berti, A., and Lugato, E.: Low stabilization of aboveground crop residue carbon in sandy soils of Swedish long-term experiments, Geoderma, 237, 246–255, https://doi.org/10.1016/j.geoderma.2014.09.010, 2015.
Poeplau, C., Zopf, D., Greiner, B., Geerts, R., Korvaar, H., Thumm, U., Don, A., Heidkamp, A., and Flessa, H.: Why does mineral fertilization increase soil carbon stocks in temperate grasslands?, Agr. Ecosyst. Environ., 265, 144–155, https://doi.org/10.1016/j.agee.2018.06.003, 2018.
Raiesi, F.: Soil properties and N application effects on microbial activities in two winter wheat cropping systems, Biol. Fert. Soils, 40, 88–92, https://doi.org/10.1007/s00374-004-0741-7, 2004.
Reynolds, J. F., Smith, D. M. S., Lambin, E. F., Turner, B. L., Mortimore, M., Battendury, S. P. J., Downing, T. E., Dowlatabadi, H., Fernández, R. J., Herrick, J. E., Huber-Sannwald, E., Jiang, H., Leemans, R., Lynam, T., Maestre, F. T., Ayarza, M., and Walker, B.: Global desertification: Building a science for dryland development, Science, 316, 847–851, https://doi.org/10.1126/science.1131634, 2007.
Saint-Laurent, D. and Arsenault-Boucher, L.: Soil properties and rate of organic matter decomposition in riparian woodlands using the TBI protocol, Geoderma, 358, 113976, https://doi.org/10.1016/j.geoderma.2019.113976, 2020.
Sandén, T., Spiegel H., Stüger, H. P., Schlatter, N., Haslmayr, H. P., Zavattaro, L., Grignani, C., Bechini, L., D'Hose, T., Molendijk, L., Pecio, A., Jarosz, Z., Guzmán, G., Vanderlinden, K., Giráldez, J. V., Mallast, J., and ten Berge, H.: European long-term field experiments: knowledge gained about alternative management practices, Soil Use Manage., 34, 167–176, https://doi.org/10.1111/sum.12421, 2018.
Sandén, T., Spiegel, H., Wenng, H., Schwarz, M., and Sarneel, J. M.: Learning science during teatime: Using a citizen science approach to collect data on litter decomposition in Sweden and Austria, Sustainability, 12, 29–39, https://doi.org/10.3390/su12187745, 2020.
Sandén, T., Wawra, A., Berthold, H., Miloczki, J., Schweinzer, A., Gschmeidler, B., Spiegel, H., Debeljak, M., and Trajanov, A.: TeaTime4Schools: Using Data Mining Techniques to Model Litter Decomposition in Austrian Urban School Soils, Front. Ecol. Evolut., 9, 432, https://doi.org/10.3389/fevo.2021.703794, 2021.
Sievers, T. and Cook, R. L.: Aboveground and root decomposition of cereal rye and hairy vetch cover crops, Soil Sci. Soc. Am. J., 82, 147–155, https://doi.org/10.2136/sssaj2017.05.0139, 2018.
Spiegel, H., Dersch, G., Hösch, J., and Baumgarten, A.: Tillage effects on soil organic carbon and nutrient availability in a long-term field experiment in Austria, Bodenkultur, 58, 1–4, 2007.
Spiegel, H., Mosleitner, T., Sandén, T., and Zaller, J. G.: Effects of two decades of organic and mineral fertilization of arable crops on earthworms and standardized litter decomposition, Die Bodenkultur: Journal of Land Management, Food and Environment, 69, 17–28, https://doi.org/10.2478/boku-2018-0003, 2018.
Stark, C., Condron, L. M., Stewart, A., Di, H. J., and O'Callaghan, M.: Influence of organic and mineral amendments on microbial soil properties and processes, Appl. Soil Ecol., 35, 79–93, https://doi.org/10.1016/j.apsoil.2006.05.001, 2007.
Struijk, M., Whitmore, A. P., Mortimer, S., Shu, X., and Sizmur, T.: Absence of a home-field advantage within a short-rotation arable cropping system, Plant Soil, 26, 1–7, https://doi.org/10.1007/s11104-022-05419-z, 2022.
Tatzber, M., Schlatter, N., Baumgarten, A., Dersch, G., Körner, R., Lehtinen, T., Unger, G., Mifek, E., and Spiegel, H.: KMnO4 determination of active carbon for laboratory routines: three long-term field experiments in Austria, Soil Res., 53, 190–204, https://doi.org/10.1071/SR14200, 2015.
Tiefenbacher, A., Sandén, T., Haslmayr, H-P., Miloczki, J., Wenzel, W., and Spiegel, H.: Optimizing carbon sequestration in croplands: a synthesis, Agronomy, 11, 882, https://doi.org/10.3390/agronomy11050882, 2021.
Tóth, Z., Táncsics, A., Kriszt, B., Kröel-Dulay, G., Ónodi, G., and Hornung, E.: Extreme effects of drought on decomposition of the soil bacterial community and decomposition of plant tissue, Eur. J. Soil Sci., 68, 504–513, https://doi.org/10.1111/ejss.12429, 2017.
Treharne, R., Bjerke, J. W., Tømmervik, H., Stendardi, L., and Phoenix, G. K.: Arctic browning: Impacts of extreme climatic events on heathland ecosystem CO2 fluxes, Glob. Change Biol., 25, 489–503, https://doi.org/10.1111/gcb.14500, 2019.
Tresch, S., Moretti, M., Le-Bayon, R. C., Mäder, P., Zanetta, A., Frey, D., Stehle, B., Kuhn, A., Munyangabe, A., and Fliessbach, A.: Urban soil quality assessment – A comprehensive case study dataset of urban garden soils, Front Environ. Sci., 6, 136, https://doi.org/10.3389/fenvs.2018.00136, 2018.
Venables, W. N. and Ripley, B. D.: Modern Applied Statistics with S, Springer-Verlag, 2002.
Werth, M. and Kuzyakov, Y.: 13C Fractionation at the Root-Microorganisms-Soil Interface: A Review and Outlook for Partitioning Studies, Soil Biol. Biochem., 42, 1372–1384, https://doi.org/10.1016/j.soilbio.2010.04.009, 2010.
Zaller, J. G., König, N., Tiefenbacher, A., Muraoka, Y., Querner, P., Ratzenböch, A., Bonkowski, M., and Koller, R.: Pesticide seed dressings can affect the activity of various soil organisms and reduce decomposition of plant material, BMC Ecol., 16, 37, https://doi.org/10.1186/s12898-016-0092-x, 2016.