the Creative Commons Attribution 4.0 License.
the Creative Commons Attribution 4.0 License.
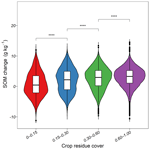
Quantification of the effects of long-term straw return on soil organic matter spatiotemporal variation: a case study in a typical black soil region
Yang Yan
Wenjun Ji
Baoguo Li
Guiman Wang
Songchao Chen
Dehai Zhu
Zhong Liu
The straw return practice is essential to soil organic matter (SOM) accumulation in the black soil area with high carbon sequestration potential. However, due to lacking accurate spatial distribution of straw return, few studies have carried out rigorous research on the impact of long-term straw return on SOM spatiotemporal variation on a regional scale. This study was carried out across an approximately 3000 km2 area in Lishu County, northeastern China, a typical agricultural plain. Based on a total of 619 soil samples and 16 environmental covariates, the study mapped the spatial distributions of SOM in 2006 and 2018 by random forest (RF) and evaluated the effects of the interaction of soil properties, land use, and straw return on SOM spatial–temporal variation. The results show that in the context of long-term straw return, the mean SOM content increased from 18.93 to 20.84 g kg−1 during 2006–2018. And 74.49 % of the region had a significant increase (maximum of 24.41 g kg−1) of SOM. The severest SOM loss occurred in the northwest due to the light texture and the transition from paddy fields to dryland. Nevertheless, for areas from paddy fields to dryland, the SOM loss decreased with the increased cumulative crop residue coverage (CRC). The SOM even increased by 1.79 g kg−1 when the cumulative crop residue coverage reached 0.60–1.00. In addition, soil with higher initial SOM and sand content had a lower response to straw return. The study revealed that straw return is beneficial to carbon sink in farmland and is a better way to prevent a carbon source caused by the conservation of paddy field to dryland.
- Article
(8705 KB) - Full-text XML
- BibTeX
- EndNote
Soil organic matter (SOM) profoundly impacts carbon contents, cationic exchange capacity, water-holding capacity, soil fertility, microorganisms, and soil structure (Ciais et al., 2011). Therefore, the SOM content's spatial–temporal variation was significant to global warming, soil quality, and ecosystem health (Ciais et al., 2011; Viscarra Rossel et al., 2014; Ondrasek et al., 2019), especially in black soil with rich SOM (Lugato et al., 2014; Amelung et al., 2020b). Recently, poor management practices have resulted in SOM loss in the black soil area. Previous studies have reported that soil fertility decreased in black soil areas in North America and eastern Europe (Russell et al., 2005; Fabrizzi et al., 2003). Meanwhile, a similar decline trend occurred in the black soil area in northeastern China (Wang et al., 2018). Therefore, rapidly and accurately quantifying the heterogeneity of SOM in the black soil region is necessary.
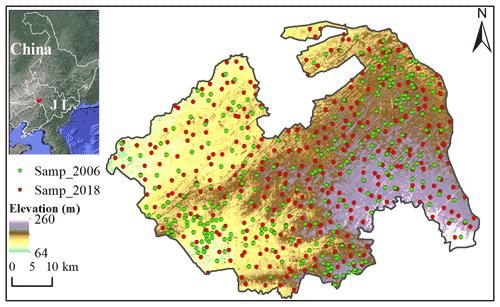
Figure 1Schematic diagram of the geographical position of the study area and sampling sites. The background is from © Google Earth and the distribution of elevation was derived from the Resource and Environment Science and Data Center.
Conventional mapping involves laboriously constructing maps by plane table and alidade (Ahrens, 2008). It is time-consuming and laborious and thus cannot satisfy the growing demand for the latest soil spatial information. Jenny (1994) described the soil as follows: soil property = f (climate, organism, relief, parent material, age) (Jenny, 1994). McBratney et al. (2003) proposed the SCORPAN function model and described the soil as follows: soil property = f (prior soil information, climate, organism, relief, parent material, age location) (Mcbratney et al., 2003). Based on soil-forming theory, digital soil mapping (DSM) uses statistical and geospatial techniques to model the relationship between soil properties and environmental covariates at a high spatial resolution. By analyzing the relationships between soil properties and environmental factors, DSM models can be developed to predict the soil properties of areas where no soil data exist. Therefore, it offers a promising solution for predicting soil properties with high precision and tremendous speed (Hengl et al., 2015; Dou et al., 2019; Liang et al., 2019; Schulze and Schütte, 2020). Moreover, DSM can also incorporate the temporal component in soil-property mapping by taking time as an index and comparing soil maps at two moments to identify changes in soil properties over time. This is particularly useful in understanding the impact of land use and management practices on soil properties and identifying areas where remediation may be necessary. Thus, the DSM method with environmental factors can accurately quantify the SOM spatial–temporal variation and measure the relationship between environmental covariates and SOM variation on a regional scale (Schillaci et al., 2017; Song et al., 2018; Zhou et al., 2019).
Nowadays, SOM variation under different land-use change and management practices has attracted increasing attention (Pan et al., 2010; Muñoz-Rojas et al., 2015). Some studies have explored reducing soil organic carbon (SOC) loss by straw return (West and Post, 2002; Liu et al., 2014; Wang et al., 2015; Amelung et al., 2020). Straw return is beneficial for retaining soil moisture and preventing soil wind erosion, especially in arid and semi-arid regions. In addition, the decomposition process of straw promotes the activity of microorganisms and is conducive to SOM accumulation (Chang et al., 2014; Lu et al., 2009; Wang et al., 2015). Conversely, previous scholars have reported that the influence of straw return on SOM accumulation is non-significant (Pittelkow et al., 2015; Poeplau et al., 2015; Powlson et al., 2011). This may be because adding organic matter to the soil has no effect on its chemical, chemical, and biological properties (Sosulski et al., 2011), or this practice may contribute to the SOM mineralization process and thus reduce SOM (Šimanský et al., 2019). The opposite result may be due to the various study areas with different soil properties, initial carbon content, land-use change, and straw return (Li et al., 2017; Berhane et al., 2020). In addition, these studies were mainly conducted on a field scale. On a regional scale, it is mostly through literature citation and policy enumeration to analyze the impact of straw return on SOM variation (Han et al., 2016; Zheng et al., 2015). However, few studies took the straw return as a variable to implement rigorous research on the effect of straw return on SOM variation due to lacking accurate spatial distribution of straw return.
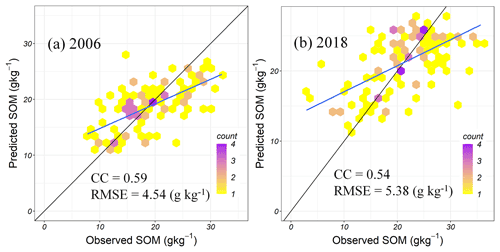
Figure 2Performances of the random forest with all environmental covariates (RF-all) on validation data in 2006 (a) and 2018 (b). RMSE, root mean square error; CC, Lin's concordance correlation coefficient; SOM, soil organic matter.
In the study, the overall objective was to take a typical black soil area as a case to quantify the relationship between SOM accumulation and straw return on a regional scale. This study area has a long-term straw return background. Specific objectives included (a) evaluating the performance of random forest (RF) models with different groups of factors to develop the most robust model; (b) analyzing the spatial–temporal variation of SOM during 2006–2018; and (c) discussing the effects of long-term straw return on SOM variation under different soil types, soil texture, and land-use change.
2.1 Study region
The study was conducted in Lishu County, Jilin Province, and its average elevation is 160 m (Fig. 1). The annual mean precipitation is 6.5∘, the annual mean temperature is 553.5 mm, and the average yearly duration of sunshine is 2541.4 h. The region's climate is classified as semi-humid. In Lishu County, the soil parent material gradually changes from weathered rocks and red sediments in the east to the loess-like sediment and loessal sub-sandy soil in the west, resulting in a regular distribution of soil type. Arenosols, Anthrosols, Phaezems, Luvisols, Cambisols, and Chernozems are the main soil types (World Reference Base for Soil Resources). Rainfall is the only source of water for crops growing in this region. The study area was located in the black soil region. However, the soil in this region was threatened by land degradation. In view of this, a research base was established in Lishu County, Jilin Province, China, in 2007, and the straw return technology was popularized.
2.2 Soil data
The straw return measure has been implemented in Lishu County since 2007, so the SOM in 2006 and 2018 was selected to quantify the SOM change under the long-term straw return background. By taking into account the sample sites in the second national soil survey, local landform, and soil types, a total of 300 sampling sites in 2006 were selected. Except for considering these factors, grid sampling was combined to select 319 sampling sites in 2018. These soil samples were collected on the surface (0–20 cm) from early October to mid-November in each year (from the harvest to the freezing). The corresponding longitude and latitude were also documented. The prediction error caused by the differences in sampling designs for the years 2006 and 2018 was not considered to make full use of legacy soil data (Ou et al., 2017; Sun et al., 2017; Nguemezi et al., 2021). A total of 3 kg soil at each sampling site was air-dried. During the process of air-drying, the soil samples were frequently turned over, and the intrusions outside the soil were removed. Sun exposure, acid, alkali, and dust pollution were strictly prohibited. After air-drying and grinding, the soil samples were thoroughly mixed and passed through a 2 mm mesh. The samples were ground and sieved to separate a particle size fraction (0.25 mm) to determine SOC concentration with the external heating potassium dichromate volumetric method, which can then be multiplied by a conversion factor of 1.724 to obtain the SOM amount (Liu et al., 1996).
2.3 Environmental covariates
The study collected the available grids on the website as environmental factors. A 30 m resolution digital elevation model (DEM) was derived from the Resource and Environment Science and Data Center (http://www.resdc.cn/, last access: 25 May 2020). Other terrain variables were calculated from DEM in the SAGA GIS software (Conrad et al., 2015), including terrain relief (TR), topographic wetness index (TWI), slope, aspect, profile curvature (PRC), multi-resolution valley bottom flatness (MrVBF) and plan curvature (PLC).
Landsat 5 TM and Landsat 8 TM were used to calculate the normalized difference vegetation index (NDVI) and enhanced vegetation index (EVI) in 2006 and 2018. The average reflectance of three image bands (B1 (blue), B3 (red), and B4 (near-infrared)) of Landsat 5 SR and Landsat 8 SR products spanning May to September were processed to calculate the NDVI by (B4 − B3)/(B4 + B3) and EVI by 2.5 × (B4 − B3)/(B4 + 6 × B3 − 7.5 × B1 × 1). The band calculation and image clipping were conducted in Google Earth Engine (GEE), and the images with less than 6 % of cloud coverage were selected.
Liu et al. (2021) provided a crop residue coverage map at a 10 m resolution in 2018 by combining radar indices and optical remote sensing indices. Crop residue cover refers to the ratio of the vertical projected area of crop residue in a field per unit area to the total surface area of this unit area, with a value ranging from 0 to 1. Firstly, the study divided the study area into a sandy soil area and a clay soil area to reduce the influence of soil properties on radar echo and spectral reflectance. Six radar indices and five optical remote sensing indices were then calculated from a Sentinel-1 SAR image and a Sentinel-2 optical image. Finally, the optimal subset regression based on these indices and 55 observations collected from 1–11 November 2018 was used to estimate the crop residue cover. The 55 observations were measured using the line–transect method. The best model shows high accuracy. Because Lishu County has implemented the straw return policy since 2007, the crop residue cover in 2006 can be regarded as 0. The difference between the crop residue cover in 2018 and 2006 was used as one of the variables (CRC) for modeling SOM in 2018 and to evaluate the effects of long-term straw return on SOM variation during 2006–2018. This study assumed that crop performance or crop types were the same except for CRC. The CRC was used to represent the straw return.
The resolution of land-use types is 30 m in 2005 and 2018. The land-use types in 2005 and 2018 are consistent with six major classes (farmland, woodland, grassland, waters, built-up land, and unused land) and 25 subclasses. The farmland includes upland and paddy land; the woodland includes forestland, shrubbery, open woodland, and other woodland; and the grassland includes high-, medium-, and low-coverage grass land. The land-use data were derived by manual visual interpretation of Landsat TM images. An electronic version of the soil-type map in Lishu County, offered by the Agricultural Extension Station in Lishu County, was digitized and delineated for this study. The map for soil clay content at 250 m resolution was obtained from SoilGrids250m products (Hengl et al., 2015) in the International Soil Reference and Information Center.
The National Earth System Science Data Center, National Science and Technology Infrastructure of China, provided annual mean precipitation (AMP) in 2006 and 2018. After multivariate regression analysis of 16 variables (Table 1) and SOM content, the variance inflation factors (VIFs) of independent variables were less than five, indicating multicollinearity did not exist among independent variables. These environmental covariates (Table 1) were resampled to 30 m using the bilinear method in ArcGIS 10.2.
Table 1Environmental covariates used for predicting soil organic matter (SOM).
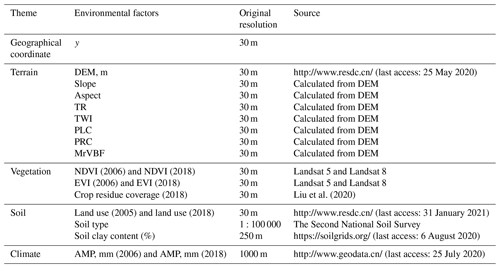
Notes: y, latitude; DEM, digital elevation model, m; TR, terrain relief index; TWI, topographic wetness index; PLC, plan curvature; PRC: profile curvature; MrVBF, multi-resolution valley bottom flatness; NDVI, normalized difference vegetation index; EVI, enhanced vegetation index; AMP, annual mean precipitation, mm. The number in parentheses is the year of the variable.
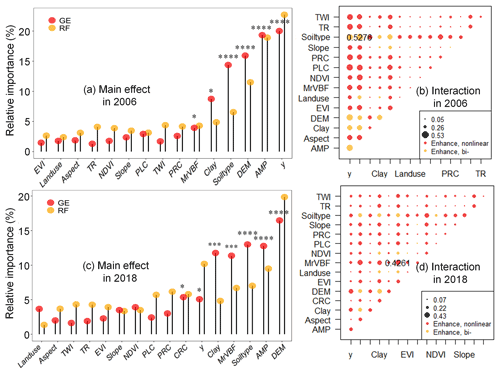
Figure 3Relative variables importance (a–c) and interaction (b–d) derived from the random forest (RF) and geographic detectors (GE) for soil organic matter (SOM) in 2006 and 2018. Notes: y, latitude; DEM, digital elevation model, m; TR, terrain relief index; TWI, topographic wetness index; PLC, plan curvature; PRC: profile curvature; MrVBF, multi-resolution valley bottom flatness; NDVI, normalized difference vegetation index; EVI, enhanced vegetation index; CRC, cumulative crop residue coverage; AMP, annual mean precipitation, mm. ; ; .
2.4 Spatial predictive modeling
2.4.1 Random forest (RF)
Many studies have successfully predicted various soil nutrient content by using the RF model (Wiesmeier et al., 2011; Guo et al., 2015; Zhang et al., 2017). First proposed by Breiman in 2001, RF (Breiman, 2001) is a tree-based ensemble model. Combined with the idea of feature selection, the approach can increase the diversity of individual decision trees and improve the generalization ability of the final RF model. The RF model combines the predictions of all the individual trees, either by taking the majority vote in the case of classification or averaging the outputs in the case of regression. This approach helps to smooth out the noise in the data and produce more accurate predictions. It includes the number of trees (ntree) and the number of variables available for selection in each split (mtry). We used 10-fold cross-validation to optimize the parameters of RF.
The study took SOM content and various environmental factors (Table 1) as the dependent and independent variables to build two RF models: consider all the variables as predictors (RF-all), consider the environmental variables without latitude as predictors (RF-(all-Y)), and consider the environmental variables without cumulative crop residue coverage (CRC) as predictors (RF-(all-CRC)).
2.4.2 Geographical detector (GE)
GE (Wang and Xu, 2017) is a statistical method used in geographical analysis to identify the factors that contribute to spatial patterns. It is based on the idea that the variation in a dependent variable across a geographical area can be explained by a set of independent variables and their interactions. GE is effective to quantify the spatial heterogeneity of attributes between layers. The method includes factor, interaction, risk, and ecological detector. We used the factor and interaction detector to explore the driving factors for SOM prediction in the study. In the factor detector, the q value (from 0 to 1) and whether it passes the significance test was given. The q value is proportional to the effect of the independent variable on the dependent variable. The interaction detector obtained five different results by comparing the q values (q1, q2) for each of two factors with the q value (q3) of the two factors' interaction, as presented in Table 2.
2.4.3 Model assessment
Independent verification was used to evaluate the performance of the model. The Lin's concordance correlation coefficient (CC) and the root mean square error (RMSE) were as evaluation metrics. The data were first randomly divided into a modeling set and a validation set according to the 7:3 ratio. In the modeling set, 10-fold cross-validation was used to obtain the best parameters of the model through RMSE as an index. The calculation of RMSE and CC is as follows:
where pi and oi are the predicted value and observed value, respectively. and are the average values of all predictions and observations, respectively. n is the number of soil samples. is the variances of predicted values, and is the variances of measured values. r is the correlation coefficient of predictions and observations. Model evaluation, descriptive analysis, and variance analysis were realized in R 4.0.2 (R Core Team, 2020). To satisfy the assumption of data independence, this study randomly selected of the total number of pixels using the sample function and then conducted pairwise significant difference analyses using the “wilcox.test” method in the ggviolin function with the stat_compare_means setting. The RF and GE models were implemented in the “caret” and “GD” libraries, respectively.
3.1 Descriptive statistics of SOM data
As shown in Table 3, from 2006 to 2018, the average SOM content increased from 18.93 to 20.84 g kg−1, and the coefficient of variations (CVs) rose slightly from 0.33 to 0.38. The CVs indicated moderate variation (Cambardella et al., 1994). The ascending CV could be attributed to more active human activities, such as popularized straw return technology in Lishu County, which is consistent with recent studies (Fan et al., 2020; Hu et al., 2014).
3.2 Model performance
Table 4 shows that the validation results considering all the variables as predictors (RF-all) (CC = 0.59, RMSE = 4.54 g kg−1, taking 2006 as an example) (Fig. 2) performed better than those considering the environmental variables without latitude as predictors (RF-(all-Y)) did. The result indicated that geographical coordinates were significant for SOM mapping. Compared with an RF-all model to predict nematode worm distribution, Ploton et al. (2020) obtained similar results by using an RF-XY (considering the longitude and latitude as predictors) model because the RF-all model mainly depends on geographic proximity (Van Den Hoogen et al., 2019). In areas dominated by agriculture, agricultural management practices (irrigation, tillage practices, farming systems, and residual management) increased the spatial heterogeneity of SOM, so the spatial distribution of SOM cannot be accurately reflected using individual vegetation indexes or topographic factors. However, few studies integrate these agricultural activities to explain the SOM spatial distribution due to the challenge of collecting them based on remote sensing technology. This study developed RF models with and without CRC. After removing CRC, CC was reduced by 14.9 %, and RMSE was increased by 0.23 g kg−1 (Table 4). The results demonstrated that the RF model with the CRC factor achieved a higher accuracy than the model using common environmental variables alone. Therefore, further research should consider the importance of geographic coordinates and long-term straw return in DSM.
Table 4The performance of the random forest model.
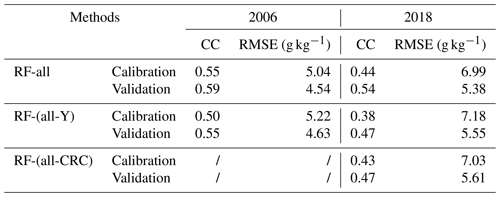
Notes: RF-all: consider all the variables as predictors; RF-(all-Y), consider the environmental variables without latitude as predictors; RF-(all-CRC), consider the environmental variables without cumulative crop residue coverage (CRC) as predictors; CC, Lin's concordance correlation coefficient; RMSE, root mean square error.
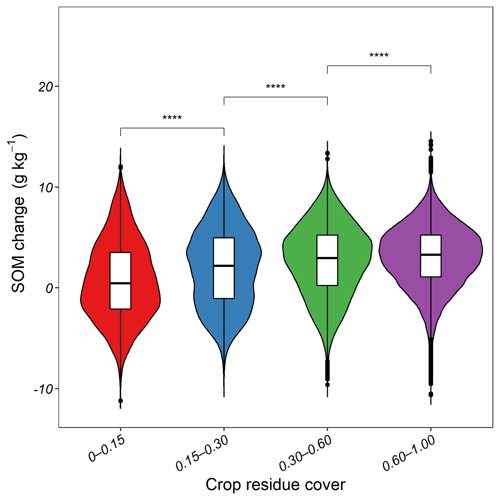
Figure 6The soil organic matter (SOM) change for different cumulative crop residue coverage (CRC). .
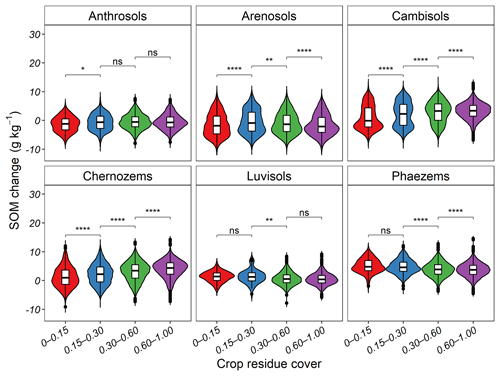
Figure 7The soil organic matter (SOM) change for different cumulative crop residue coverage (CRC) under soil type. ns, no significance; ; ; .
Table 5Models' performance in soil organic matter (SOM) prediction in plain farmland areas.
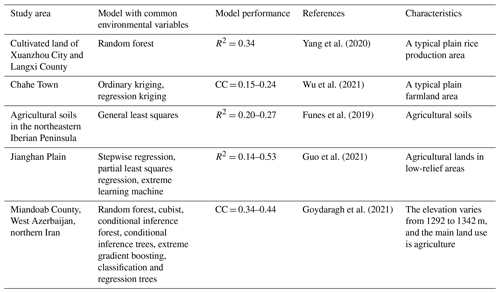
Notes: CC, Lins' concordance correlation coefficient.
The accuracy of the study's model was not high, which is the study's limitation. By reviewing the SOM or SOC modeling in flat farmland areas, we found that the models of these similar studies all show poor performance (Table 5). This may be because common environmental variables, such as topographic and remote sensing factors, are too homogeneous to effectively extract SOM information (Zeng et al., 2017). Compared with these studies in flat farmland areas, our prediction accuracy is comparable or even better (Table 5).
3.3 Importance of driving factors on SOM spatial distribution
As Fig. 3a and c presented, the relative importance of environmental factors obtained from the RF and GE methods was comparable, proving the feasibility of the two methods. For quantifying the relative importance, GE is based on spatial relationships, while RF is based on feature importance through decision tree splits. The geographical coordinates, soil type, precipitation, clay content, DEM, MrVBF, and CRC play key roles in SOM prediction (relative importance >5 %). The relative importance of the other factors was almost less than 5 %. Moreover, these factors passed the significance test in GE model. As Fig. 3b and d presented, the explanatory strength of 16 variables' interaction in pairs was stronger than that of the single factor through nonlinear enhancement or double factor enhancement (enhancement, bi). This finding proved that the complex interaction among different influencing factors led to the spatial distribution pattern of SOM. Figure 3b and d also proved that y, AMP, and DEM have the greatest influence on SOM. Similar to our results, Wang et al. (2017) found that precipitation was the key climatic variable that affects the spatial distribution of SOM in Liaoning, northeastern China. Many studies have revealed the importance of terrain parameters for predicting SOM in northeastern China (Wang et al., 2018; Ma et al., 2017). This may be because DEM-based terrain parameters cause the recombination and redistribution of temperature, water, light, soil, wind speed, and wind direction and thus affect the SOM content. At the same time, it shows the CRC was significant using the significance test with GE. CRC is not the main cause of spatial variation of SOM, but the CRC did contribute significantly to the spatial distribution of SOM.
3.4 Spatial variation of SOM over time
Figure 4 presents the spatial distributions of SOM (30 m resolution) in 2006 and 2018. The general spatial pattern was clear: the SOM decreased from southeast to northwest in Lishu County each year. In 2006, SOM concentration was highest in the south of Lishu County (22–28 g kg−1) and lowest in the western part (under 12 g kg−1). The highest SOM content was concentrated in the middle and east of the study area in 2018, while low SOM content (0–12 g kg−1) occupied a tiny area.
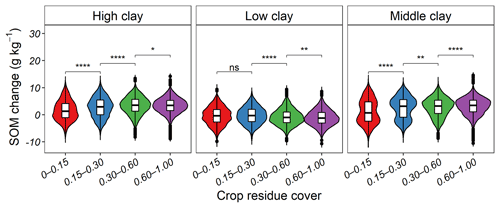
Figure 9The soil organic matter (SOM) change for different cumulative crop residue coverage (CRC) under soil texture. ns, no significance; ; ; .
The overall trend of SOM content was on the rise from 2006 to 2018. Consistent with the distribution of high CRC (0.30–1.00) (Fig. 5a), the SOM content in 74.49 % areas of Lishu County displayed a significant increasing trend, especially in the eastern part of the county (Fig. 4c) with a maximum increase of 24.41 g kg−1. Many studies have revealed that straw return contributes to carbon sink due to increased microbial biomass and biological activity (Han et al., 2016; Amelung et al., 2020a; Berhane et al., 2020).
The decrease in SOM mainly occurred in the northwest and south of Lishu County. The SOM reduction in southern Lishu County happened to be in the area with the high initial SOM concentration (2006) (Fig. 4c). Consistent with previous research, the reduction was severe in areas with higher initial concentrations of SOC (Zhou et al., 2019). In the northwest corner, the decrease in SOM was mainly distributed on the Arenosols and Anthrosols with a marked decline of 12.70 g kg−1 (Figs. 4c, 5b). The phenomenon may be related to the low CRC, light-texture soil, and the change from paddy land to dryland.
3.5 Effects of straw return on SOM variation
Figure 6 presents SOM increased by 0.74, 2.01, 2.68, and 3.08 g kg−1 when the CRC was 0–0.15, 0.15–0.30, 0.30–0.60, and 0.60–1.00, respectively. This result proved the SOM increment was proportional to the CRC. A previous study revealed that the effect of straw return on SOM content was closely linked to soil properties, initial SOM content, land-use change, and the straw return amount (Berhane et al., 2020). Therefore, variance analysis was conducted to explore the effects of straw return on SOM variation under different land-use change, soil types, and soil texture.
3.5.1 Effects of the straw return on SOM variation under different soil types
According to Fig. 7, for Anthrosols, Cambisols, and Chernozems, SOM increment was proportionate to the CRC. For Luvisols and Phaezems, SOM variation showed a decreasing trend with the boosted CRC. This phenomenon is relevant to the initial SOM contents. As displayed in Fig. 8, the initial SOM contents were lower in Anthrosols, Cambisols, and Chernozems and higher in Luvisols and Phaezems. Berhane et al. (2020) claimed that regardless of the soil type, the response of SOC change to carbon input was weak when the initial SOC content was high. Li et al. (2018) concluded that Phaezems with the highest initial SOM content had the lowest response rate to fertilization. A possible explanation for this might be that soils with low initial SOM are far away from their saturation levels and thus have a greater potential for carbon sequestration. Except for the SOM loss in Arenosols and Anthrosols, the SOM changes in other soil types were almost all positive (Fig. 7). This result verified that the degradation of Arenosols and Anthrosols resulted in the SOM reduction due to the light-texture soil and land-use change from the paddy land to dryland, respectively.
3.5.2 Effects of straw return on SOM variation under different soil texture
With the CRC increasing, SOM first increased and then decreased for soil with low clay content (Fig. 9). Nevertheless, SOM increases with the boosted CRC in soils with high and medium clay contents (Fig. 9), indicating that clay content was directly proportional to the response of SOM increment to CRC. The findings are consistent with previous research, which shows that straw return results in the highest carbon chelation when clay content is high (Xia et al., 2018). One possible explanation for this observation was that soils with higher clay content have greater potential to store organic carbon (Li et al., 2020), so the SOM accumulation in clayey soils is more responsive to straw return. Moreover, the SOM increment under sandy soil declined when the CRC was 0.60–1.00, which was because sandy soil cannot be protected by mineral particles (Xia et al., 2018) and is more susceptible to the influence of microorganisms.
3.5.3 Effects of straw return on SOM variation under different land-use change
The study considered only two kinds of land-use change: (a) the change from dryland to paddy land, and (b) the change from paddy land to dryland because the crop area accounted for over 70 % of the total sown area in Lishu County and all the samples were taken from farmland. In the two kinds of land-use change, variance analysis was carried out for the SOM variation under four levels of CRC. As shown in Fig. 10, the SOM increment increases with the boosted CRC under the change from dryland to paddy field. From paddy to dry land, the SOM dropped by 0.71 g kg−1 when the CRC was 0–0.15. However, with the increase in the CRC, the SOM loss gradually decreased. Even the SOM increased by 1.79 g kg−1 when the CRC was 0.60–1.00, indicating that straw return can reverse the carbon loss caused by the transformation of paddy to dryland. Previous studies have found that due to the transformation from anaerobic to aerobic, the conversion of paddy to dryland will cause carbon loss (Wang et al., 2014; Nishimura et al., 2008; Li et al., 2016). Some research pointed out that we can reduce the carbon loss by rewetting (Driessen et al., 2000) or cultivation of paddy fields continuously (Chen et al., 2017). The study pointed out that straw return is a way to prevent a C source caused by the conversion of the paddy field to dryland and can be carried out after that paddy is converted to dryland or when paddy is fallow.
The study estimated the surface SOM content and SOM variation between 2006 and 2018 by the RF model, quantified the spatial relationship between measured SOM and predictors, and further explored the effect of the interaction of soil properties, land use, and straw return on SOM change. The results of the study were as follows:
-
The RF model with all predictors (CC = 0.54, RMSE = 5.38 g kg−1 in 2018) performed better than RF-(all-Y) (CC = 0.47, RMSE = 5.55 g kg−1) and RF-(all-CRC) (CC = 0.47, RMSE = 5.61 g kg−1) in both 2006 and 2018. The result indicated that geographical coordinates and long-term straw return were significant for SOM mapping.
-
The SOM content decreased from southeast to northwest during both periods. Over time, SOM increased in 74.49 % of areas in Lishu County, with the eastern part showing a maximum increase of 24.41 g kg−1. The northwest and south corners of the study area aggregated the SOM loss, especially in the northwest, with a significant decline of 12.70 g kg−1.
-
Straw return played the main role in SOM variation. SOM increased by 0.74, 2.01, 2.68, and 3.08 g kg−1 with CRC of 0–0.15, 0.15–0.30, 0.30–0.60, and 0.60–1.00. The response rate of SOM to the CRC was inversely proportional to the initial SOM and the sand contents.
The study revealed that straw return is beneficial to carbon sink in farmland and is a better way to prevent a carbon source caused by the conservation of paddy fields to dryland, which can contribute to the development of strategies to ensure the sustainability of agricultural soils.
The data that support the findings of this study are available on request from the corresponding author.
Conceptualization, WJ; data curation, GW and ZL; methodology, WJ and YY; writing -original draft preparation, YY; writing – review and editing, WJ, SC, and DZ; supervision, WJ; project administration, WJ and BL; funding acquisition, WJ.
The contact author has declared that none of the authors has any competing interests.
Publisher’s note: Copernicus Publications remains neutral with regard to jurisdictional claims in published maps and institutional affiliations.
This study was supported by the National Natural Science Foundation of China (project no. 42001048), the National Key R&D Program of China (2021YFD1500201), the Open Fund of State Key Laboratory of Remote Sensing Science (OFSLRSS202121), the State Key Laboratory of Resources and Environmental Information System (2020), and the Chinese Universities Scientific Fund (2020TC205).
This paper was edited by Bas van Wesemael and reviewed by two anonymous referees.
Ahrens, R. J.: Digital soil mapping with limited data, PhD., Springer Science & Business Media, 978-1-4020-8591-8, 2008.
Amelung, W., Bossio, D., de Vries, W., Kögel-Knabner, I., Lehmann, J., Amundson, R., Bol, R., Collins, C., Lal, R., and Leifeld, J. J. N. C.: Towards a global-scale soil climate mitigation strategy, Nat. Commun., 11, 1–10, 2020.
Berhane, M., Xu, M., Liang, Z., Shi, J., Wei, G., and Tian, X.: Effects of long-term straw return on soil organic carbon storage and sequestration rate in North China upland crops: A meta-analysis, Global Change Biol., 26, 2686–2701, https://doi.org/10.1111/gcb.15018, 2020.
Breiman, L.: Random forests, Mach. Learn., 45, 5–32, 2001.
Cambardella, C. A., Moorman, T. B., Novak, J., Parkin, T., Karlen, D., Turco, R., and Konopka, A.: Field-scale variability of soil properties in central Iowa soils, Soil Sci. Soc. Am. J., 58, 1501–1511, 1994.
Chang, L., Meng, L., Jun, C., Bo, L., and Changming, F.: Effects of straw carbon input on carbon dynamics in agricultural soils: a meta-analysis, Global Change Biol., 20, 1336–1361, 2014.
Chen, A., Xie, X., Ge, T., Hou, H., Wang, W., Wei, W., and Kuzyakov, Y.: Rapid decrease of soil carbon after abandonment of subtropical paddy fields, Plant Soil, 415, 203–214, 2017.
Ciais, P., Gervois, S., Vuichard, N., Piao, S., and Viovy, N.: Effects of land use change and management on the European cropland carbon balance, Global Change Biol., 17, 320–338, 2011.
Conrad, O., Bechtel, B., Bock, M., Dietrich, H., Fischer, E., Gerlitz, L., Wehberg, J., Wichmann, V., and Böhner, J.: System for Automated Geoscientific Analyses (SAGA) v. 2.1.4, Geosci. Model Dev., 8, 1991–2007, https://doi.org/10.5194/gmd-8-1991-2015, 2015.
Dou, X., Wang, X., Liu, H., Zhang, X., Meng, L., Pan, Y., Yu, Z., and Cui, Y.: Prediction of soil organic matter using multi-temporal satellite images in the Songnen Plain, China, Geoderma, 356, 113896, https://doi.org/10.1016/j.geoderma.2019.113896, 2019.
Driessen, P., Deckers, J., Spaargaren, O., and Nachtergaele, F.: Lecture notes on the major soils of the world, PhD., 94, Food and Agriculture Organization (FAO), 9251046379, 2000.
Fabrizzi, K. P., Moron, A., and García, F. O.: Soil carbon and nitrogen organic fractions in degraded vs. non-degraded Mollisols in Argentina, Soil Sci. Soc. Am. J., 67, 1831–1841, 2003.
Fan, M. M., Margenot, A. J., Zhang, L. M., Lal, R., Wu, J. T., Chang, N. J., Shaukate, M., Chen, F. R., and Gao, C.: Soil organic carbon dynamics in intensively managed agricultural landscapes of eastern China, Arch. Agron. Soil Sci., 68, 503–515, 2020.
Funes, I., Savé, R., Rovira, P., Molowny-Horas, R., Alcañiz, J. M., Ascaso, E., Herms, I., Herrero, C., Boixadera, J., and Vayreda, J.: Agricultural soil organic carbon stocks in the north-eastern Iberian Peninsula: Drivers and spatial variability, Sci. Tot. Environ., 668, 283–294, 2019.
Goydaragh, M. G., Taghizadeh-Mehrjardi, R., Jafarzadeh, A. A., Triantafilis, J., and Lado, M.: Using environmental variables and Fourier Transform Infrared Spectroscopy to predict soil organic carbon, Catena, 202, 105280, https://doi.org/10.1016/j.catena.2021.105280, 2021.
Guo, L., Fu, P., Shi, T., Chen, Y., Zeng, C., Zhang, H., and Wang, S.: Exploring influence factors in mapping soil organic carbon on low-relief agricultural lands using time series of remote sensing data, Soil and Tillage Research, 210, 104982, https://doi.org/10.1016/j.still.2021.104982, 2021.
Guo, P. T., Li, M. F., Luo, W., Tang, Q. F., Liu, Z. W., and Lin, Z. M.: Digital mapping of soil organic matter for rubber plantation at regional scale: An application of random forest plus residuals kriging approach, Geoderma, 237, 49–59, https://doi.org/10.1016/j.geoderma.2014.08.009, 2015.
Han, P., Zhang, W., Wang, G., Sun, W., and Huang, Y.: Changes in soil organic carbon in croplands subjected to fertilizer management: a global meta-analysis, Sci. Rep., 6, 1–13, 2016.
Hengl, T., Heuvelink, G. B., Kempen, B., Leenaars, J. G., Walsh, M. G., Shepherd, K. D., Sila, A., MacMillan, R. A., Mendes de Jesus, J., and Tamene, L.: Mapping soil properties of Africa at 250 m resolution: Random forests significantly improve current predictions, PLoS One, 10, e0125814, https://doi.org/10.1371/journal.pone.0125814, 2015.
Hu, K., Wang, S., Li, H., Huang, F., and Li, B.: Spatial scaling effects on variability of soil organic matter and total nitrogen in suburban Beijing, Geoderma, 226, 54–63, 2014.
Jenny, H.: Factors of soil formation: a system of quantitative pedology, PhD., Courier Corporation,, 04-866-81289, 1994.
Li, X., Toma, Y., Yeluripati, J., Iwasaki, S., Bellingrath-Kimura, S. D., Jones, E. O., and Hatano, R.: Estimating agro-ecosystem carbon balance of northern Japan, and comparing the change in carbon stock by soil inventory and net biome productivity, Sci. Total Environ., 554, 293–302, 2016.
Li, Y., Li, Z., Chang, S. X., Cui, S., Jagadamma, S., Zhang, Q., and Cai, Y.: Residue retention promotes soil carbon accumulation in minimum tillage systems: Implications for conservation agriculture, Science of The Total Environment, 740, 140147, https://doi.org/10.1016/j.scitotenv.2020.140147, 2020.
Li, Y. E., Shi, S., Waqas, M. A., Zhou, X., Li, J., Wan, Y., Qin, X., Gao, Q., Liu, S., Wilkes, A.: Long-term (=20 years) application of fertilizers and straw return enhances soil carbon storage: a meta-analysis, Mittig. Adapt. Strat. Gl., 23, 603–619, 2018.
Liang, Z., Chen, S., Yang, Y., Zhao, R., Shi, Z., and Viscarra Rossel, R. A.: National digital soil map of organic matter in topsoil and its associated uncertainty in 1980's China, Geoderma, 335, 47–56, https://doi.org/10.1016/j.geoderma.2018.08.011, 2019.
Liu, C., Lu, M., Cui, J., Li, B., and Fang, C.: Effects of straw carbon input on carbon dynamics in agricultural soils: a meta-analysis, Global Change Biol., 20, 1366–1381, 2014.
Liu, G., Jiang, N., and Zhang, L.: Soil physical and chemical analysis & description of soil l profiles, PhD., Beiiing: Standards Press of China, 1996 (in Chinese).
Liu, Z., Liu, Z., Wan, W., Huang, J., Wang, J., and Zheng, M.: Estimation of maize residue cover on the basis of SAR and optical remote sensing image, Nat. Remote Sens. Bull., 25, 1308–1323, 2021.
Lu, F., Wang, X., Han, B., Ouyang, Z., Duan, X., Zheng, H., and Miao, H.: Soil carbon sequestrations by nitrogen fertilizer application, straw return and no-tillage in China's cropland, Global Change Biol., 15, 281–305, 2009.
Lugato, E., Panagos, P., Bampa, F., Jones, A., and Montanarella, L.: A new baseline of organic carbon stock in European agricultural soils using a modelling approach, Global Change Biol., 20, 313–326, 2014.
Ma, Y., Minasny, B. and Wu, C.: Mapping key soil properties to support agricultural production in Eastern China. Geoderma Regional, 10, 144–153, 2017.
McBratney, A. B., Santos, M. M., and Minasny, B.: On digital soil mapping, Geoderma, 117, 3–52, 2003.
Muñoz-Rojas, M., Doro, L., Ledda, L., Francaviglia, R., and Environment: Application of CarboSOIL model to predict the effects of climate change on soil organic carbon stocks in agro-silvo-pastoral Mediterranean management systems, Agr. Ecosyst. Environ., 202, 8–16, 2015.
Nguemezi, C., Tematio, P., Silatsa, F. B., Yemefack, M. J. S., and Research, T.: Spatial variation and temporal decline (1985–2017) of soil organic carbon stocks (SOCS) in relation to land use types in Tombel area, South-West Cameroon, Soil Till. Res., 213, 105114, https://doi.org/10.1016/j.still.2021.105114, 2021.
Nishimura, S., Yonemura, S., Sawamoto, T., Shirato, Y., Akiyama, H., Sudo, S., and Yagi, K.: Effect of land use change from paddy rice cultivation to upland crop cultivation on soil carbon budget of a cropland in Japan, Agr. Ecosyst. Environ., 125, 9–20, https://doi.org/10.1016/j.agee.2007.11.003, 2008.
Ondrasek, G., Begić, H. B., Zovko, M., Filipović, L., Meriño-Gergichevich, C., Savić, R., and Rengel, Z.: Biogeochemistry of soil organic matter in agroecosystems & environmental implications, Sci. Total Environ., 658, 1559–1573, 2019.
Ou, Y., Rousseau, A. N., Wang, L., and Yan, B.: Spatio-temporal patterns of soil organic carbon and pH in relation to environmental factors – A case study of the Black Soil Region of Northeastern China, Agr. Ecosyst., 245, 22–31, 2017.
Pan, G., Xu, X., Smith, P., Pan, W., and Lal, R.: An increase in topsoil SOC stock of China's croplands between 1985 and 2006 revealed by soil monitoring, Agr. Ecosyst. Environ., 136, 133–138, 2010.
Pittelkow, C. M., Liang, X., Linquist, B. A., Van Groenigen, K. J., Lee, J., Lundy, M. E., Van Gestel, N., Six, J., Venterea, R. T., and Van Kessel, C.: Productivity limits and potentials of the principles of conservation agriculture, Nature, 517, 365–368, 2015.
Poeplau, C., Kätterer, T., Bolinder, M. A., Börjesson, G., Berti, A., and Lugato, E. J.: Low stabilization of aboveground crop residue carbon in sandy soils of Swedish long-term experiments, Geoderma, 237, 246–255, 2015.
Powlson, D. S., Glendining, M. J., Coleman, K., and Whitmore, A. P.: Implications for Soil Properties of Removing Cereal Straw: Results from Long-Term Studies, Agron. J., 103, 279–287, 2011.
Russell, A. E., Laird, D., Parkin, T. B., and Mallarino, A. P.: Impact of nitrogen fertilization and cropping system on carbon sequestration in Midwestern Mollisols, Soil Sci. Soc. Am. J., 69, 413–422, 2005.
R Core Team R: A language and environment for statistical computing, R Foundation for Statistical Computing, Vienna, Austria, https://www.R-project.org/ (last access: 16 July 2020), [code], 2020.
Schillaci, C., Acutis, M., Lombardo, L., Lipani, A., Fantappie, M., Marker, M., and Saia, S.: Spatio-temporal topsoil organic carbon mapping of a semi-arid Mediterranean region: The role of land use, soil texture, topographic indices and the influence of remote sensing data to modelling, Sci. Total Environ., 601, 821–832, https://doi.org/10.1016/j.scitotenv.2017.05.239, 2017.
Schulze, R. E. and Schütte, S.: Mapping soil organic carbon at a terrain unit resolution across South Africa, Geoderma, 373, 114447, https://doi.org/10.1016/j.geoderma.2020.114447, 2020.
Šimanský, V., Juriga, M., Jonczak, J., Uzarowicz, L., and Stępień, W.: How relationships between soil organic matter parameters and soil structure characteristics are affected by the long-term fertilization of a sandy soil, Geoderma, 342, 75–84, 2019.
Song, X. D., Yang, F., Ju, B., Li, D. C., Zhao, Y. G., Yang, J. L., and Zhang, G. L.: The influence of the conversion of grassland to cropland on changes in soil organic carbon and total nitrogen stocks in the Songnen Plain of Northeast China, Catena, 171, 588–601, 2018.
Sosulski, T. and Korc, M.: Effects of different mineral and organic fertilization on the content of nitrogen and carbon in soil organic matter fractions, Ecol. Chem. Eng. A. 18, 601–609, 2011.
Sun, X.-L., Wang, H.-L., Zhao, Y.-G., Zhang, C., and Zhang, G.-L.: Digital soil mapping based on wavelet decomposed components of environmental covariates, Geoderma, 303, 118–132, https://doi.org/10.1016/j.geoderma.2017.05.017, 2017.
Van den Hoogen, J., Geisen, S., Routh, D., Ferris, H., Traunspurger, W., Wardle, D. A., de Goede, R. G. M., Adams, B. J., Ahmad, W., Andriuzzi, W. S., Bardgett, R. D., Bonkowski, M., Campos-Herrera, R., Cares, J. E., Caruso, T., Caixeta, L. D., Chen, X. Y., Costa, S. R., Creamer, R., Castro, J. M. D., Dam, M., Djigal, D., Escuer, M., Griffiths, B. S., Gutierrez, C., Hohberg, K., Kalinkina, D., Kardol, P., Kergunteuil, A., Korthals, G., Krashevska, V., Kudrin, A. A., Li, Q., Liang, W. J., Magilton, M., Marais, M., Martin, J. A. R., Matveeva, E., Mayad, E., Mulder, C., Mullin, P., Neilson, R., Nguyen, T. A. D., Nielsen, U. N., Okada, H., Rius, J. E. P., Pan, K., Peneva, V., Pellissier, L., da Silva, J. C. P., Pitteloud, C., Powers, T. O., Powers, K., Quist, C. W., Rasmann, S., Moreno, S. S., Scheu, S., Setala, H., Sushchuk, A., Tiunov, A. V., Trap, J., van der Putten, W., Vestergard, M., Villenave, C., Waeyenberge, L., Wall, D. H., Wilschut, R., Wright, D. G., Yang, J. I., and Crowther, T. W.: Soil nematode abundance and functional group composition at a global scale, Nature, 572, 194–198, 2019.
Viscarra Rossel, R. A., Webster, R., Bui, E. N., and Baldock, J. A.: Baseline map of organic carbon in Australian soil to support national carbon accounting and monitoring under climate change, Global Change Biol., 20, 2953–2970, https://doi.org/10.1111/gcb.12569, 2014.
Wang, H., Guan, D., Zhang, R., Chen, Y., Hu, Y., and Xiao, L.: Soil aggregates and organic carbon affected by the land use change from rice paddy to vegetable field, Ecol. Eng., 70, 206–211, 2014.
Wang, J. and Xu, C.: Geodetector: Principle and prospective, Ac. Geogr. Sin., 72, 116–134, 2017.
Wang, J., Wang, X., Xu, M., Feng, G., and Zhang, W.: Crop yield and soil organic matter after long-term straw return to soil in China, Nutr. Cyc. Agroecosyst., 102, 371–381, 2015.
Wang, S., Zhuang, Q., Jia, S., Jin, X., and Wang, Q.: Spatial variations of soil organic carbon stocks in a coastal hilly area of China, Geoderma, 314, 8–19, 2018.
Wang, S., Zhuang, Q., Wang, Q., Jin, X., and Han, C.: Mapping stocks of soil organic carbon and soil total nitrogen in Liaoning Province of China, Geoderma, 305, 250–263, 2017.
Wang, S., Zhao, Y., Wang, J., Zhu, P., Cui, X., Han, X., Xu, M., and Lu, C.: The efficiency of long-term straw return to sequester organic carbon in Northeast China's cropland, J. Int. Agr., 17, 436–448, https://doi.org/10.1016/s2095-3119(17)61739-8, 2018.
West, T. and Post, W.: Soil organic carbon sequestration rates by tillage and crop rotation: a global data analysis, Soil Sci. Soc. Am. J., 66, 1930–1946, 2002.
Wiesmeier, M., Barthold, F., Blank, B., and Kogel-Knabner, I.: Digital mapping of soil organic matter stocks using Random Forest modeling in a semi-arid steppe ecosystem, Plant Soil, 340, 7–24, https://doi.org/10.1007/s11104-010-0425-z, 2011.
Wu, Z., Liu, Y., Han, Y., Zhou, J., Liu, J., and Wu, J.: Mapping farmland soil organic carbon density in plains with combined cropping system extracted from NDVI time-series data, Sci. Total Environ., 754, 142120, https://doi.org/10.1016/j.scitotenv.2020.142120, 2021.
Xia, L., Lam, S. K., Wolf, B., Kiese, R., Chen, D., and Butterbach-Bahl, K.: Trade-offs between soil carbon sequestration and reactive nitrogen losses under straw return in global agroecosystems, Global Change Biol., 24, 5919–5932, 2018.
Yang, L., He, X., Shen, F., Zhou, C., Zhu, A. X., Gao, B., Chen, Z., and Li, M.: Improving prediction of soil organic carbon content in croplands using phenological parameters extracted from NDVI time series data, Soil Till. Res., 196, 104465, https://doi.org/10.1016/j.still.2019.104465, 2020.
Zeng, C., Zhu, A. X., Liu, F., Yang, L., Rossiter, D. G., Liu, J., and Wang, D.: The impact of rainfall magnitude on the performance of digital soil mapping over low-relief areas using a land surface dynamic feedback method, Ecol. Ind., 72, 297–309, 2017.
Zhang, H., Wu, P., Yin, A., Yang, X., Zhang, M., and Gao, C.: Prediction of soil organic carbon in an intensively managed reclamation zone of eastern China: A comparison of multiple linear regressions and the random forest model, Sci. Total Environ., 592, 704–713, https://doi.org/10.1016/j.scitotenv.2017.02.146, 2017.
Zheng, L., Wu, W., Wei, Y., and Hu, K.: Effects of straw return and regional factors on spatio-temporal variability of soil organic matter in a high-yielding area of northern China, Soil Till. Res., 145, 78–86, 2015.
Zhou, Y., Hartemink, A. E., Shi, Z., Liang, Z., and Lu, Y.: Land use and climate change effects on soil organic carbon in North and Northeast China, Sci. Total Environ., 647, 1230–1238, 2019.