the Creative Commons Attribution 4.0 License.
the Creative Commons Attribution 4.0 License.
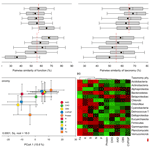
Lower functional redundancy in “narrow” than “broad” functions in global soil metagenomics
Kayan Ma
Yu Huang
Qi Fu
Yingbo Qiu
Jiajiang Lin
Christopher W. Schadt
Hao Chen
Understanding the relationship between soil microbial taxonomic compositions and functional profiles is essential for predicting ecosystem functions under various environmental disturbances. However, even though microbial communities are sensitive to disturbance, ecosystem functions remain relatively stable, as soil microbes are likely to be functionally redundant. Microbial functional redundancy may be more associated with “broad” functions carried out by a wide range of microbes than with “narrow” functions in which specific microorganisms specialize. Thus, a comprehensive study to evaluate how microbial taxonomic compositions correlate with broad and narrow functional profiles is necessary. Here, we evaluated soil metagenomes worldwide to assess whether functional and taxonomic diversities differ significantly between the five broad and the five narrow functions that we chose. Our results revealed that, compared with the five broad functions, soil microbes capable of performing the five narrow functions were more taxonomically diverse, and thus their functional diversity was more dependent on taxonomic diversity, implying lower levels of functional redundancy in narrow functions. Co-occurrence networks indicated that microorganisms conducting broad functions were positively related, but microbes specializing in narrow functions were interacting mostly negatively. Our study provides strong evidence to support our hypothesis that functional redundancy is significantly different between broad and narrow functions in soil microbes, as the association of functional diversity with taxonomy was greater in the five narrow than in the five broad functions.
- Article
(4649 KB) - Full-text XML
-
Supplement
(1285 KB) - BibTeX
- EndNote
Microbial communities often exhibit incredible taxonomic diversity, with one gram of soil harboring millions of microbial species (Gans et al., 2005). However, how such diversity governs microbial functional potential and ecosystem processes is largely unknown. Though microbial taxonomic composition is generally sensitive to disturbance and often does not rapidly recover (Allison and Martiny, 2008), it is unclear how changes in microbial community composition would regulate ecosystem functioning. A mechanistic understanding of microbial systems, including microbial taxonomic compositions and functional potential, is essential for predicting ecosystem functioning under various environmental disturbances (Mcgill et al., 2006; Torsvik and Øvreås, 2002; Wellington et al., 2003).
Though microbial community composition usually shifts in response to disturbance, ecosystem functions could remain relatively stable due to functional redundancy (Allison and Martiny, 2008). Microbial functional redundancy is an inevitable emergent property of microbial systems (Louca et al., 2018), as some metabolic functions can be performed by multiple species, which may thus be substitutable in certain ecosystem processes (Rosenfeld, 2002), implying that microbial taxonomy and function can be decoupled (Louca et al., 2016, 2017). Functional redundancy can involve either “strict redundancy”, meaning that microorganisms sharing the exact same set of functions can easily substitute each other, or “partial redundancy”, denoting that microbes show similarities in certain functions but still harbor differences in other functions, leading to partially dissimilar ecological requirements or environmental preferences (Galand et al., 2018). In addition, microbial functional redundancy may be caused by not only metabolic processes but also other mechanical responses to environmental disturbance, such as different foraging strategies, particle attachment and biofilm formation, nitrogen source usage, and resistance to antibiotics, which are difficult to thoroughly evaluate in the current approach that mostly focuses on metabolic redundancy (Louca et al., 2018).
Microbial functional redundancy has been mainly observed in “broad” ecosystem processes (Yin et al., 2000; Rousk et al., 2009; Banerjee et al., 2016), but is perhaps less significant in “narrow” functions in which certain microorganisms specialize (Schimel, 1995; Balser et al., 2002). However, some studies that simulated microbial diversity reduction and physiological processes have challenged the hypothesis of microbial redundancy in soil microbes (Delgado-Baquerizo et al., 2016b; Philippot et al., 2013; Peter et al., 2011). Microbial functional redundancy is inevitable when a high-dimensional trait space is projected into a lower-dimensional function space of interest (Louca et al., 2018). Such apparent contradictory results suggest that the degree of functional redundancy may arise from the definition of redundancy in different studies and our limitations in measuring the factors controlling niche space, and – more importantly – may depend on the function of interest. Microbes conducting broad metabolic functions, such as carbon decomposition, are likely to distribute across most taxa (Crowther et al., 2019) and associate with a high level of functional redundancy (Rivett and Bell, 2018; Beier et al., 2017). Narrow functions, such as nitrification or methanogenesis, may be restricted to a few phylogenetic clades (Schimel and Gulledge, 1998), and are hypothesized to exhibit less redundancy than broad functions (Schimel, 1995; Rocca et al., 2015). Today, multifunctionality (Hector and Bagchi, 2007) has to be accounted for to avoid overestimating functional redundancy (Gamfeldt et al., 2008). By assessing multiple functions, the relationship between microbial diversity in the soil and ecosystem function can be better quantified (Delgado-Baquerizo et al., 2016a; Bastida et al., 2016).
Nowadays, metagenomics is increasingly being used as a promising comparative tool (Tringe et al., 2005) to study the relationship between functional and taxonomic diversities (Fierer et al., 2012, 2013; Souza et al., 2015; Pan et al., 2014; Leff et al., 2015). The growing wealth of soil metagenome data is thus well poised to aid in the generalization of global patterns of microbial attributes and the standardization of frameworks for the consistent representation of microbial communities (Xu et al., 2021). However, a synthetic metagenomic analysis to assess how general microbial taxonomic and functional beta diversities differ between broad and narrow functions across the globe is still lacking.
Here, we constructed soil metagenomic datasets of the taxonomic and functional beta diversities of five broad and five narrow functions across 17 climate zones. We typically chose the SEED Subsystems database (Overbeek et al., 2013), which has a diverse classification at level 1, allowing us to conduct a comparison between broad and narrow functions. We selected five narrow functions, namely N (nitrogen metabolism), P (phosphorus metabolism), K (potassium metabolism), S (sulfur metabolism), and Fe (iron acquisition and metabolism). These are typical functional categories of specific nutrient cycling in subsystems level 1, and are only performed by certain groups of soil microbes (Schimel, 1995). The five broad functions selected were AAD (amino acids and derivatives), CHO (carbohydrates), CBS (clustering-based subsystems), CVPGP (cofactors, vitamins, prosthetic groups, pigments), and Protein (protein metabolism), which are the most abundant functional categories in subsystems level 1, and represent broad-scale functions acquired by a relatively large group of diverse soil microbes (Balser et al., 2002). We further constructed the pairwise similarity of function and taxonomy based on the relative abundances of functional and taxonomic compositions, respectively, for the five broad and the five narrow functions. We hypothesized that the taxonomic similarity of soil microbes would be more linearly correlated to the functional similarity for the five narrow functions in comparison to the five broad functions. Therefore, using these global soil metagenomes, our objective was to test whether the taxonomic compositions of soil microbes that conduct the five narrow functions are more dependent on the functional compositions, leading to a lower level of functional redundancy in the narrow functions than the broad functions.
2.1 Data collection
To ensure that the quality and completeness of the metagenomes analyzed were of a sufficient standard, we carefully selected soil metagenomes in the MG-RAST server that have been published in peer-reviewed journals. We searched peer-reviewed publications from 2012 to 2018 in the Web of Science database using search terms such as “soil metagenome”, “shotgun sequencing”, and “MG-RAST” to trace the metagenomic data used in this study to their source publications. We included soil metagenomes that are publicly available in the MG-RAST database and were generated using shotgun sequencing without amplification or were directly deposited by peer-reviewed studies into the MG-RAST database. We then extracted a data matrix of taxonomic and functional compositions of soil metagenomes from the MG-RAST public server (https://www.mg-rast.org/, last access: 10 September 2019) based on the study ID and/or MG-RAST ID reported in the publications. Details of each soil metagenome extracted from publications and the MG-RAST database are given in Table S1 in the Supplement.
The functional database that we used in this study, SEED Subsystems, is a categorization system that organizes gene functional categories into a hierarchy with three levels of resolution (levels 3, 2, and 1; Overbeek et al., 2013). To download the taxonomic compositions of soil microbes that conduct broad and narrow functions, in the “Analysis” function of the MG-RAST server (https://www.mg-rast.org/mgmain.html?mgpage=analysis, last access: 10 September 2019), we loaded SEED subsystems (levels 3, 2, and 1) as functional profiles and RefSeq (Tatusova et al., 2013) databases (genus, family, order, class, and phylum levels) as taxonomic compositions (Xu et al., 2021) for each soil metagenome. The detailed protocols of the MG-RAST server were followed to analyze the metagenomic functions (Meyer et al., 2008; Wilke et al., 2017). To obtain the taxonomic compositions of soil microbes that conduct the selected broad and narrow functions, we chose “RefSeq” as the source and “genus” as the level, and in “function filter” we added the functional categories in subsystems level 1 that we were interested in, including the five broad functions of AAD (amino acids and derivatives), CHO (carbohydrates), CBS (clustering-based subsystems), CVPGP (cofactors, vitamins, prosthetic groups, pigments), and Protein (protein metabolism), for which the relative abundances were 5 %–13 %. AAD, CHO, CBS, CVPGP, and Protein were the most abundant functional categories in subsystems level 1, and were used to represent the broad-scale functions acquired by a large group of diverse soil microbes. Correspondingly, five narrow functions were chosen, namely N (nitrogen metabolism), P (phosphorus metabolism), K (potassium metabolism), S (sulfur metabolism), and Fe (iron acquisition and metabolism), for which the relative abundances were 0.8 %–1.4 %, as these are typical functional categories of specific nutrient cycling in subsystems level 1, and are only performed by certain groups of soil microbes. The genus level was used as the taxonomic classification level across different datasets. Following the default setting in MG-RAST, species that were classified into higher classification levels than genus but could not be identified at the genus level were assigned to “unclassified” groups. Across the various studies, 2.16 ± 0.85 % of the sequences belonged to the unclassified groups, showing that most of the taxonomic groups were classified to the genus level. The total hits for a particular taxonomic composition of soil microbes that conduct a particular function at subsystems level 1 was calculated as the sum of the hits for all genera (at the RefSeq genus level) comprising that taxonomic composition.
Comparative metagenomic analyses were performed using default settings (maximum e-value cutoff = , minimum identity cutoff = 60 %, and minimum alignment length = 50; Meyer et al., 2008). We then merged the taxonomic compositions of the data matrix for each function extracted from different studies to generate new datasets of microbial taxonomic compositions annotated by the RefSeq database. The reason why we chose the Subsystems database for functional grouping rather than the KEGG Orthology (KO; Kanehisa et al., 2015), Clusters of Orthologous Groups (COG; Galperin et al., 2014), and Non-supervised Orthologous Groups (NOG; Huerta-Cepas et al., 2015) databases was that Subsystems had more diverse classification at level 1, allowing us to conduct a direct comparison between broad and narrow functions. We chose the RefSeq database rather than traditional ribosomal RNA databases such as the RDP (Ribosomal Database Project; Cole et al., 2008), Greengenes (Desantis et al., 2006), or Silva LSU/SSU (Pruesse et al., 2007) because taxonomic hits in the RefSeq database were over 1000-fold higher than in the rRNA databases, rendering the resolution comparable to that for functional hits when comparing broad and narrow functions. To increase the coverage of our datasets, soil metagenomes with and without assembly were both included.
The geographic coordinates – latitude (LAT) and longitude (LONG) – of each soil metagenome were directly obtained from publications. Based on the LAT and LONG, climate data on the mean annual temperature (MAT) and precipitation (MAP) at each study site for each soil metagenome were extracted from the WorldClim dataset (Fick and Hijmans, 2017) using the R package “raster” (Hijmans et al., 2015). To examine how the microbial taxonomic beta diversities of broad and narrow functions differ globally, soil metagenomic data were classified into 17 climate zones based on the main Köppen–Geiger climatic zone classification (Kottek et al., 2006) using the R package “kgc” (Bryant et al., 2017).
2.2 Statistical analyses
To minimize bias caused by different sequencing depths and read lengths among studies, we standardized the hits for each taxonomic (or functional) category in each sequence to the relative abundance by dividing those hits by the total number of hits. To calculate the pairwise similarity of taxonomy based on the relative taxonomic abundances at genus level of the microbes conducting the five broad and five narrow functions, we calculated the Bray–Curtis similarity following log transformation of the compositional taxonomic data by constructing a pairwise Bray–Curtis similarity matrix between each pair of samples for each functional category in the Subsystems database at level 1, in which matrices were further transformed into lists of pairwise Bray–Curtis similarities ordered by sample pair names in PRIMER 7 (Plymouth Routines in Multivariate Ecological Research Statistical Software, v7.0.13, PRIMER-E Ltd, UK; Clarke and Gorley, 2015). To calculate the pairwise similarity of function based on the functional abundance at function gene level within each of the five broad and five narrow functions, we calculated the Bray–Curtis similarity following log transformation of the compositional functional data by constructing a pairwise Bray–Curtis similarity matrix between each pair of samples for each functional category in the Subsystems database at level 1, in which matrices were further transformed into lists of pairwise Bray–Curtis similarities ordered by sample pair names in PRIMER 7. To examine the relationship between functional and taxonomic beta diversities, Pearson's correlations were constructed between the transformed lists of pairwise Bray–Curtis similarities of soil metagenomes annotated using the Subsystems database at level 3 (function) and the RefSeq database at genus level (taxonomy). The approaches used for processing the relative abundance of compositional data follow the requirements of compositional data analysis in microbiome datasets (Gloor et al., 2017). To analyze the taxonomic composition structures of soil metagenomes annotated using the RefSeq database at genus level (taxonomy) for the five broad and five narrow functions, PCoA (principal coordinates analysis) and PERMANOVA (permutational multivariate analysis of variance) were conducted using the pairwise Bray–Curtis similarity matrix in PRIMER 7.
To compare the microbial taxonomic compositions of the five broad and the five narrow functions, one-factor PERMANOVA was conducted using the main test and pairwise test in PRIMER 7 with P values and square root of estimates of components of variation reported. Pearson correlations were constructed to assess the relationships between functional and taxonomic beta diversities in the broad and narrow functions with the adjusted P2 reported. A RELATE analysis was also performed to evaluate the relatedness among broad and narrow functions by calculating Spearman's rho correlation coefficient in PRIMER 7. To examine the relative abundances of dominant microbes at phylum and class levels (mean >1 %) among the five broad and five narrow functions, heatmaps were constructed using HeatMapper (Babicki et al., 2016). One-way analysis of variance (ANOVA) with P values adjusted by Bonferroni correction for multiple comparisons was conducted using SPSS 22.0 software (Chicago, IL, USA) to evaluate differences in the relative abundances of dominant taxonomic compositions (mean >1 %) among climate zones after the normality of residues and homogeneity of variance were checked using the Shapiro–Wilk and Levene test, respectively. The significance level was set at α=0.05 unless otherwise stated. To calculate the statistical difference between the relative abundances of dominant microbial taxonomic groups (mean >1 %) in the broad and narrow functions, the LEfSe (linear discriminant analysis effect size) method was used (http://huttenhower.sph.harvard.edu/lefse/, last access: 6 December 2019; Segata et al., 2011). Venn diagrams were constructed to visualize the amount of dominant microbial taxonomic groups at genus level or the network nodes shared between the five broad and the five narrow functions using InteractiVenn (Heberle et al., 2015).
To find potential interactions of microbial taxonomic compositions between broad and narrow functions across the globe, co-occurrence network analysis was performed using the Molecular Ecological Network Analyses Pipeline (http://ieg4.rccc.ou.edu/MENA/, last access: 1 November 2019; Deng et al., 2012; Zhou et al., 2011). To make the minimum observed value close to but no less than 1, as required by the pipeline, the relative abundance data were multiplied by 106, which did not change the correlation coefficients. The transformed data matrix was uploaded to construct the network with default settings, including (1) only keeping species that were present in more than half of all samples, (2) only filling 0.01 into blanks with paired valid values, (3) taking the logarithm of the recommended similarity matrix of Pearson's correlation coefficient; and (4) setting the parameter “calculation order” to “decrease the cutoff from top” and the parameter “scan speed” to “regress Poisson distribution only”. A default cutoff value (similarity threshold, St) for the similarity matrix was used to create a link between the pair of species. After that, the global network properties, the centrality of individual nodes, and the module separation and modularity were analyzed based on default settings using greedy modularity optimization. Network files were exported and visualized using Cytoscape software (Shannon et al., 2003). Scatter plots of within-module connectivity (Zi) and among-module connectivity (Pi) were constructed to show the network node distribution of module-based topological roles of taxonomic compositions for the broad and narrow functions. The threshold values of Zi and Pi used for categorization were 2.5 and 0.62, respectively (Guimerà and Nunes Amaral, 2005; Guimerà et al., 2007; Olesen et al., 2006). An overview of the data acquisition, transformation, and analysis processes used in this study is given in Fig. S1 in the Supplement.
3.1 Microbial taxonomic compositions
This study included 845 soil metagenomes across 17 climate zones around the world, which were extracted from 56 MG-RAST studies published in 51 peer-reviewed papers. They resulted in 356 090 pairwise comparisons of Bray–Curtis similarity in functional (subsystems level 3) and taxonomic (RefSeq genus) beta diversity for the five broad and five narrow functions, which were analyzed to find out whether the correlations in function and taxonomy were greater in the five narrow functions. Overall, for the five narrow functions, the positive correlations of the pairwise similarity of taxonomy and function between two samples (r2=0.36–0.49) were greater than those for the five broad functions (r2=0.23–0.29; Fig. 1). This suggests that rare phylotypes could be more associated with narrow ecosystem processes than broad-scale functions, supporting the notion that the abundances of particular specialists could influence narrow functional measures (Rivett and Bell, 2018; Peter et al., 2011), leading to the association of a lower degree of functional redundancy with narrow functions, such as the nutrient cycling examined in this study.
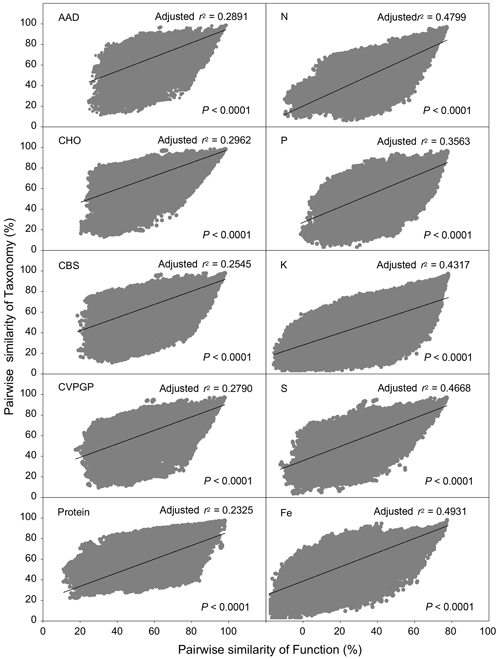
Figure 1Relations between functional and taxonomic beta diversities for broad and narrow functions. Pearson's correlations between the pairwise Bray–Curtis similarity of microbial taxonomic composition and the pairwise Bray–Curtis similarity of functional composition for broad and narrow functions annotated using Subsystems at the function level (function) and RefSeq at the genus level (taxonomy) are shown. For each correlation, r2 and P values are given. Broad functions include AAD (amino acids and derivatives), CHO (carbohydrates), CBS (clustering-based subsystems), CVPGP (cofactors, vitamins, prosthetic groups, pigments), and Protein (protein metabolism). Narrow functions include N (nitrogen metabolism), P (phosphorus metabolism), K (potassium metabolism), S (sulfur metabolism), and Fe (iron acquisition and metabolism).
Table 1Summary of key properties of co-occurrence networks for the five broad and the five narrow functions.
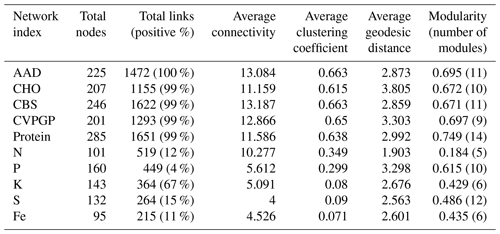
Several soil metagenomic studies have reported a linear relationship between functional and taxonomic diversities (Fierer et al., 2012, 2013; Leff et al., 2015), indicating a dependency of microbial functional profiles on taxonomic composition. This dependency, however, does not necessarily imply an absence of microbial functional redundancy. In fact, all of those studies showed a lower variation of beta diversity in metagenomic function than in taxonomy (Fierer et al., 2012, 2013; Souza et al., 2015; Pan et al., 2014) or a higher similarity in composition of functional profiles than in taxonomic composition (Leff et al., 2015). Those findings suggest that microbial functions are more stable than taxonomy in response to ecological and environmental perturbations. In this study, the five broad and the five narrow functions had relative abundances of 5 %–13 % and 0.8 %–1.4 %, respectively. Thus, the five broad functions are more abundant than the five narrow functions. In addition, the numbers of genes within the categories of the five broad functions were also greater than those for the narrow functions. As the diversities of the microbes conducting the five broad functions were also greater than those conducting the narrow functions, we calculated the relationship between the diversities of taxonomy and function for each of the five broad and five narrow functions and compared the resulting relationships. Our study further evidenced a lower extent of functional redundancy in the five narrow functions compared to the five broad functions, despite the linear correlations found in our study.
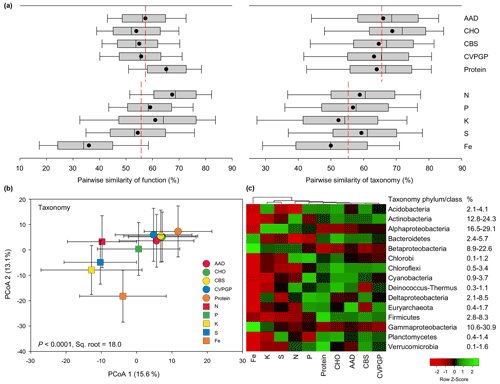
Figure 2Functional and taxonomic diversities for broad versus narrow functions. (a) Box plots and mean values of pairwise Bray–Curtis similarity of microbial functional diversity and of pairwise Bray–Curtis similarity of taxonomic diversity for broad versus narrow functions. (b) PCoA (principal coordinates analysis) showing microbial taxonomic beta diversity for broad and narrow functions annotated using RefSeq at genus level (taxonomy). The error bars represent standard deviations of data ranges. Variations (by percentage) explained by the two principal coordinate dimensions are given in parentheses. The P value and the square root of PERMANOVA are also given. (c) Heatmap showing the relative abundances of dominant microbial taxonomic compositions (mean >0.5 %) for broad and narrow functions annotated using RefSeq at the phylum/class level (taxonomy). Broad functions include AAD (amino acids and derivatives), CHO (carbohydrates), CBS (clustering-based subsystems), CVPGP (cofactors, vitamins, prosthetic groups, pigments), and Protein (protein metabolism); narrow functions include N (nitrogen metabolism), P (phosphorus metabolism), K (potassium metabolism), S (sulfur metabolism), and Fe (iron acquisition and metabolism).
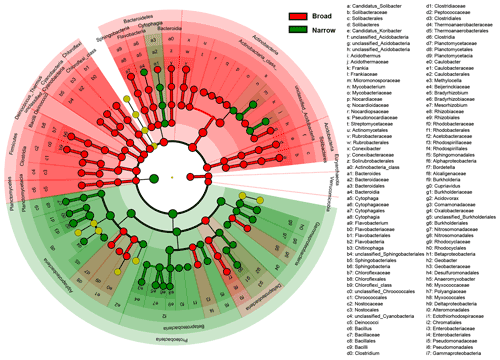
Figure 3Difference in taxonomic compositions between broad and narrow functions. LEfSe (linear discriminant analysis effect size) results are presented that show the significant differences in the relative abundances of dominant microbial taxonomic groups (mean >0.5 %) between broad (red) and narrow (green) functions annotated using RefSeq (taxonomy). From the center outward, each circle represents the domain, phylum, class, order, family, and genus level, respectively. The taxonomic groups with significant differences are labeled by color.
To compare the similarity ranges of these two compositions related to the five broad functions versus the five narrow functions, boxplots were constructed based on the pairwise similarity of function and taxonomy. For the functional compositions at specific function gene levels, the average similarity of the five broad functions (58 %) was comparable to that of the five narrow functions (56 %; Fig. 2a). However, the pairwise similarities of the five narrow functions showed larger variation, with the Fe function showing the lowest similarity of 36 % and the N function showing the highest similarity of 69 %. On the contrary, the taxonomic similarities of the five broad functions were consistently greater (63 %–69 %) than those of the five narrow functions (50 %–59 %). The PERMANOVA pairwise test was conducted to find the difference in taxonomic similarity between the microbes conducting the five broad functions and those conducting the five narrow functions, based on the relative abundance. Our results indicated that the microbial taxonomic compositions of the five broad functions were more phylogenetically different from those of the five narrow functions (13 %–22 %) than they were from each other (8 %–13 %; Table S2 in the Supplement). The RELATE test was also conducted to evaluate the relationship between the taxonomic compositions of the microbes conducting the five broad functions and the corresponding relationship for the five narrow functions. Our results confirmed that the microbial taxonomic compositions of the five broad functions were more correlated with each other (0.97–0.99) than those of the five narrow functions (0.77–0.94; Table S3 in the Supplement). When the microbial taxonomic compositions of the 10 functional categories were combined in a PCoA analysis, the resulting scatter plot showed that the five broad functions were grouped closely together and separated from the five narrow functions (Fig. 2b). The grouping of the 10 functions generally explained up to 18.0 % of the community difference, with the five narrow functions being more distinct from each other. These results together suggest that the taxonomic composition was more conserved for soil microbes conducting the five broad functions than for those conducting the five narrow functions. However, it should be noted that the current analysis has some limitations, as the metagenomics datasets consisted of sequencing data that were phylogenetically classified and assigned based on certain taxonomic and functional databases. Thus, our results may to some extent depend on the databases chosen, since the classification and assignment may contain potential bias. Future studies should continue to test this hypothesis using regional samples and individual datasets.
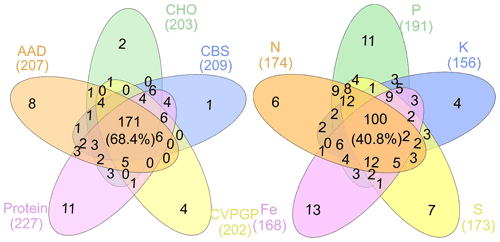
Figure 4Taxonomic compositions shared among broad and narrow functions. The Venn diagram shows the dominant microbial taxonomic groups (mean >0.1 %), annotated using RefSeq at the genus level (taxonomy), that are shared among broad and narrow functions.
To investigate how microbial taxonomic diversity differs globally, the taxonomic compositions of soil microbes that conduct the five broad and the five narrow functions were analyzed among the 17 climate zones based on the PCoA analysis. Across the climate zones, the microbial taxonomic compositions of the five narrow functions (square root = 15.2–18.8) were more distinct than those of the five broad functions (square root = 13.4–15.1), based on the PERMANOVA analysis (Fig. S2 in the Supplement). This suggests that microorganisms that perform broad functions are similar to each other in taxonomy, because broad functions are more broadly distributed across most taxa, whereas soil microbes performing narrow functions are more phylogenetically diverse due to the specialty of narrow functions. Thus, though microbial metabolic functions can be strongly coupled to elemental cycles and certain environmental factors, the decoupling of microbial taxonomic and functional profiles is still inevitable when a low-dimensional functional space is projected into a high-dimensional taxonomic space (Louca et al., 2018), especially for broad functions. Moreover, certain environmental factors (such as the extreme environment of an ice cap) may have significant effects on the coupling of taxonomy and function due to the selective pressure they exert, and thus future research can focus on comparing the relationship between function and taxonomy among terrestrial ecosystems with different selective pressure levels.
The taxonomic compositions of microbes conducting the five broad functions showed greater abundances of most major phyla, such as Acidobacteria, Actinobacteria, Bacteroidetes, and Firmicutes, while the taxonomic compositions of microbes conducting the five narrow functions showed greater abundances of Proteobacteria, especially Alphaproteobacteria and Betaproteobacteria (Fig. 2c). Other studies also found that some bacteria that conduct N cycling, such as ammonia oxidizers and rhizobia for N fixation, mainly belong to Alphaproteobacteria or Betaproteobacteria (Moulin et al., 2001; Stephen et al., 1996).
To find the dominant microbial groups that were statistically different between the five broad and the five narrow functions, LEfSe analysis was conducted based on the relative abundances at the taxonomic levels of domain, phylum, class, order, family, and genus. In particular, among the Proteobacteria conducting the five narrow functions, Bacillaceae from Bacilli, Clostridium, Peptococcaceae, and Thermoanaerobacteraceae from Clostridia, Methylocella, Bradyrhizobium, Bradyrhizobiaceae, and Rhizobiaceae from Rhodospirillaceae, and Cupriavidus from Comamonadaceae had higher relative abundances than the others (Fig. 3). Venn diagrams indicated that the taxonomic compositions of soil microbes that perform the broad functions shared 68 % of the dominant genera among the five functional categories, while the proportion was reduced to only 41 % for the five narrow functions (Fig. 4). However, it should be stated that all the analyses performed in our study were based on relative abundance data that are compositional, so it is difficult to directly compare taxonomic diversities among samples and/or datasets. Despite the presence of differences in the identification protocol and quantification of soil metagenomes, we deem the effects of these differences to be trivial for our analyses, as we intended to understand the general patterns of microbial taxonomic and functional linkages rather than to simply compare soil community structures across samples. By uncovering universal patterns of these relationships within the microbial community, we can then further establish a potential linkage framework to account for the microbial contributions to major biogeochemical cycles.
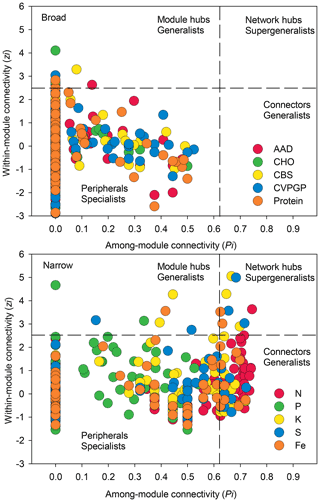
Figure 5Network information on taxonomic compositions for broad and narrow functions. The figure shows node distributions of module-based topological roles of taxonomic compositions for broad and narrow functions determined by scatter plots of within-module connectivity (Zi) versus among-module connectivity (Pi). The threshold values of Zi and Pi for categorization were 2.5 and 0.62, respectively.
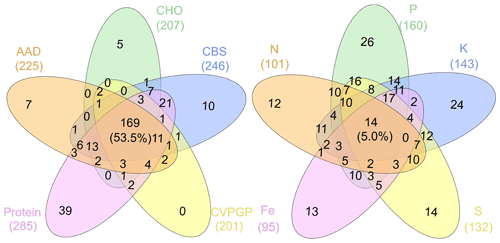
Figure 6Venn diagrams showing the microbial taxonomic network nodes shared among broad and narrow functions.
Because of the functional redundancy of soil microbes, understanding which types of functions have more significant associations with microbial taxonomy can be critical for the accurate prediction of microbial metabolic activity and flexibility across space and time. As the microbial taxonomic composition and diversity play a critical role in maintaining ecosystem function (Allison and Martiny, 2008), our results suggest that taxonomic information alone is of limited utility for predicting basic metabolic capabilities, but may be capable of forecasting biogeochemical transformations or changes in the rate of a biogeochemical process at ecosystem level (Hall et al., 2018). Investigating functional redundancy with respect to functions associated with elemental cycles provides useful information for guiding the development of explicit microbial biogeochemical prediction, and delving further into the major pathways of C and N cycles will be a fruitful approach for scrutinizing the functional potentials of microbes.
3.2 Microbial taxonomic co-occurrence networks
To identify potential interaction patterns of microbial groups that conduct the five broad and the five narrow functions, co-occurrence networks of taxonomic compositions were generated based on the taxonomic composition at the genus level across the globe. Network graphs with submodule structures indicated distinct taxonomic network topologies for the broad and narrow functions (Table 1, Figs. S3 and S4 in the Supplement). Compared to the narrow functions, the broad functions harbored larger and more complex networks with more nodes (201–285 vs. 95–160) and links (1293–1651 vs. 215–519), along with higher average connectivities (11.2–13.2 vs. 4.0–10.3) and average clustering coefficients (0.64–0.66 vs. 0.07–0.35). The broad function network had 99 %–100 % positive links, while the narrow function network had 33 %–96 % negative links. This significant difference in network properties between broad and narrow functions suggests that the taxonomic composition of narrow functions has both facilitative and inhibitive interactions, while the taxonomic compositions of the broad functions are all cooperative (Faust and Raes, 2012). Thus, soil microbes with broad functions tended to respond to the environment in a similar way, indicating functional sharing and association, while the distinct microorganisms that conduct narrow functions competitively interact with each other, reflecting regulatory or suppression relationships (Delgado-Baquerizo et al., 2018).
In addition, network modularity was greater for the broad functions, indicating that significant correlations between the taxonomic compositions of microbes that conduct the five broad functions occur mainly within similar taxonomic groups. No node could be classified as a connector in the five broad function networks (Fig. 5), reaffirming that the broad function networks had links mainly within modules of similar species. In the co-occurrence network of taxonomic composition of the narrow functions, 13 % of the nodes were identified as connectors linking several modules (high Pi), while 3 % were identified as module hubs that connected other nodes within their own modules (high Zi), as indicated by the Zi–Pi plot (Deng et al., 2012; Olesen et al., 2007). Significantly fewer nodes were identified as module hubs in the co-occurrence network of the taxonomic composition of the broad functions, indicting that fewer correlations were found among different modules. This is expected, given that each module comprised genera that were mainly from the same phylogenetic groups. This difference was consistent with Venn diagrams showing that significantly more nodes were shared among the five functional categories representing the broad functions (54 %) than among the five narrow function networks (5 %; Fig. 6). Environmental conditions likely determine the microbial taxonomic composition, and microbial phylotypes with similar habitat preferences tend to co-occur (Delgado-Baquerizo et al., 2018; Ramírez-Flandes et al., 2019). We emphasize that this analysis is a combination of snapshots of microbial communities compared across space, so environmental conditions (at the same geographic location) may vary and the levels of functional redundancy may change depending on the mechanisms selecting specific functions and the phylogenetic distribution of those functions (Louca et al., 2018).
By analyzing and generalizing microbial taxonomic and functional profiles, we provide strong evidence that the degree of soil microbial functional redundancy differs significantly between broad and narrow functions across the globe. The level of functional redundancy varies depending on the functions of interest. Here, by contrasting the five broad metabolic functions with the five narrow functions that are important for elemental cycles, we found that lower levels of functional redundancy are associated with the five narrow functions of biogeochemical cycling, despite the fact that, even for the five narrow functions, there is still a high level of functional redundancy in the soil communities. Although there is a caveat concerning the direct comparison of metagenomic data, the present study demonstrated the use of comparative metagenome and co-occurrence network analysis in generalizing patterns of microbial characteristics regulating the biogeochemical cycling of major elements. With the increasing advancement of sequencing techniques and data coverage, future sequencing efforts will likely increase our confidence in comparative metagenomes and provide time-series information to further identify to what extent microbial functional redundancy regulates dynamic ecological fluxes across space and time.
The data that support the findings of this study are available from the corresponding author upon request. All metagenomic data used in this study are publicly accessible on the MG-RAST server with the study and MG-RAST IDs reported in the Supplement.
The supplement related to this article is available online at: https://doi.org/10.5194/soil-8-297-2022-supplement.
HuC conceived the study, performed the data analysis, interpreted the results, and drafted the paper. JL, CWS, and HaC secured the research funding. KM, YH, QF, YQ, and HaC critically assessed and interpreted the findings. All authors discussed the results and commented on, edited, revised, and approved the paper.
The contact author has declared that neither they nor their co-authors have any competing interests.
Publisher's note: Copernicus Publications remains neutral with regard to jurisdictional claims in published maps and institutional affiliations.
We thank the authors of the publications included in our study, without which this global metagenomic analysis would not be possible.
This study is supported by the National Natural Science Foundation of China (grant nos. 41977106 and 31872691) and the Basic and Applied Basic Research Foundation of Guangdong Province (grant no. 2022A1515010861).
This paper was edited by Ashish Malik and reviewed by two anonymous referees.
Allison, S. D. and Martiny, J. B.: Resistance, resilience, and redundancy in microbial communities, P. Natl. Acad. Sci. USA, 105, 11512–11519, 2008.
Babicki, S., Arndt, D., Marcu, A., Liang, Y., Grant, J. R., Maciejewski, A., and Wishart, D. S.: Heatmapper: web-enabled heat mapping for all, Nucleic Acids Res., 44, W147–W153, 2016.
Balser, T. C., Kinzig, A. P., and Firestone, M. K.: Linking soil microbial communities and ecosystem functioning, in: The functional consequences of biodiversity: empirical progress and theoretical extensions, edited by: Kinzig, A. P., Pacala, S., and Tilman, D., Princeton University Press, 265–293, https://doi.org/10.1515/9781400847303.265, 2002.
Banerjee, S., Kirkby, C. A., Schmutter, D., Bissett, A., Kirkegaard, J. A., and Richardson, A. E.: Network analysis reveals functional redundancy and keystone taxa amongst bacterial and fungal communities during organic matter decomposition in an arable soil, Soil Biol. Biochem., 97, 188–198, 2016.
Bastida, F., Torres, I. F., Moreno, J. L., Baldrian, P., Ondoño, S., Ruiz-Navarro, A., Hernández, T., Richnow, H. H., Starke, R., and García, C.: The active microbial diversity drives ecosystem multifunctionality and is physiologically related to carbon availability in Mediterranean semi-arid soils, Mol. Ecol., 25, 4660–4673, 2016.
Beier, S., Shen, D., Schott, T., and Jürgens, K.: Metatranscriptomic data reveal the effect of different community properties on multifunctional redundancy, Mol. Ecol., 26, 6813–6826, 2017.
Bryant, C., Wheeler, N., Rubel, F., and French, R.: kgc: Koeppen–Geiger climatic zones, R package version 1.0.0.2, https://cran.r-project.org/package=kgc (last access: 10 September 2019), 2017.
Clarke, K. and Gorley, R.: Getting started with PRIMER v7, PRIMER-E: Plymouth, Plymouth Marine Laboratory, http://updates.primer-e.com/primer7/manuals/Getting_started_with_PRIMER_7.pdf (last access: 10 September 2019), 2015.
Cole, J. R., Wang, Q., Cardenas, E., Fish, J., Chai, B., Farris, R. J., Kulam-Syed-Mohideen, A., McGarrell, D. M., Marsh, T., and Garrity, G. M.: The Ribosomal Database Project: improved alignments and new tools for rRNA analysis, Nucleic Acids Res., 37, D141–D145, 2008.
Crowther, T. W., Van den Hoogen, J., Wan, J., Mayes, M. A., Keiser, A., Mo, L., Averill, C., and Maynard, D. S.: The global soil community and its influence on biogeochemistry, Science, 365, eaav0550, https://doi.org/10.1126/science.aav0550, 2019.
Delgado-Baquerizo, M., Maestre, F. T., Reich, P. B., Jeffries, T. C., Gaitan, J. J., Encinar, D., Berdugo, M., Campbell, C. D., and Singh, B. K.: Microbial diversity drives multifunctionality in terrestrial ecosystems, Nat. Commun., 7, 10541, https://doi.org/10.1038/ncomms10541, 2016a.
Delgado-Baquerizo, M., Giaramida, L., Reich, P. B., Khachane, A. N., Hamonts, K., Edwards, C., Lawton, L. A., and Singh, B. K.: Lack of functional redundancy in the relationship between microbial diversity and ecosystem functioning, J. Ecol., 104, 936–946, 2016b.
Delgado-Baquerizo, M., Oliverio, A. M., Brewer, T. E., Benavent-González, A., Eldridge, D. J., Bardgett, R. D., Maestre, F. T., Singh, B. K., and Fierer, N.: A global atlas of the dominant bacteria found in soil, Science, 359, 320–325, 2018.
Deng, Y., Jiang, Y.-H., Yang, Y., He, Z., Luo, F., and Zhou, J.: Molecular ecological network analyses, BMC Bioinformatics, 13, 113, https://doi.org/10.1186/1471-2105-13-113, 2012.
Desantis, T. Z., Hugenholtz, P., Larsen, N., Rojas, M., Brodie, E. L., Keller, K., Huber, T., Dalevi, D., Hu, P., and Andersen, G. L.: Greengenes, a chimera-checked 16S rRNA gene database and workbench compatible with ARB, Appl. Environ. Microbiol., 72, 5069–5072, 2006.
Faust, K. and Raes, J.: Microbial interactions: from networks to models, Nat. Rev. Microbiol., 10, 538, https://doi.org/10.1038/ismej.2011.159, 2012.
Fick, S. E. and Hijmans, R. J.: WorldClim 2: new 1-km spatial resolution climate surfaces for global land areas, Int. J. Climatol., 37, 4302–4315, 2017.
Fierer, N., Lauber, C. L., Ramirez, K. S., Zaneveld, J., Bradford, M. A., and Knight, R.: Comparative metagenomic, phylogenetic and physiological analyses of soil microbial communities across nitrogen gradients, ISME J., 6, 1007, https://doi.org/10.1038/nrmicro2832, 2012.
Fierer, N., Ladau, J., Clemente, J. C., Leff, J. W., Owens, S. M., Pollard, K. S., Knight, R., Gilbert, J. A., and McCulley, R. L.: Reconstructing the microbial diversity and function of pre-agricultural tallgrass prairie soils in the United States, Science, 342, 621–624, 2013.
Galand, P. E., Pereira, O., Hochart, C., Auguet, J. C., and Debroas, D.: A strong link between marine microbial community composition and function challenges the idea of functional redundancy, ISME J., 12, 2470–2478, https://doi.org/10.1038/s41396-018-0158-1, 2018.
Galperin, M. Y., Makarova, K. S., Wolf, Y. I., and Koonin, E. V.: Expanded microbial genome coverage and improved protein family annotation in the COG database, Nucleic Acids Res., 43, D261–D269, 2014.
Gamfeldt, L., Hillebrand, H., and Jonsson, P. R.: Multiple functions increase the importance of biodiversity for overall ecosystem functioning, Ecology, 89, 1223–1231, 2008.
Gans, J., Wolinsky, M., and Dunbar, J.: Computational improvements reveal great bacterial diversity and high metal toxicity in soil, Science, 309, 1387–1390, 2005.
Gloor, G. B., Macklaim, J. M., Pawlowsky-Glahn, V., and Egozcue, J. J.: Microbiome datasets are compositional: and this is not optional, Front. Microbiol., 8, 2224, https://doi.org/10.3389/fmicb.2017.02224, 2017.
Guimerà, R. and Nunes Amaral, L. A.: Functional cartography of complex metabolic networks, Nature, 433, 895–900, https://doi.org/10.1038/nature03288, 2005.
Guimerà, R., Sales-Pardo, M., and Amaral, L. A. N.: Classes of complex networks defined by role-to-role connectivity profiles, Nat. Phys., 3, 63–69, https://doi.org/10.1038/nphys489, 2007.
Hall, E. K., Bernhardt, E. S., Bier, R. L., Bradford, M. A., Boot, C. M., Cotner, J. B., del Giorgio, P. A., Evans, S. E., Graham, E. B., and Jones, S. E.: Understanding how microbiomes influence the systems they inhabit, Nat. Microbiol., 3, 977–982, 2018.
Heberle, H., Meirelles, G. V., da Silva, F. R., Telles, G. P., and Minghim, R.: InteractiVenn: a web-based tool for the analysis of sets through Venn diagrams, BMC Bioinformatics, 16, 169, https://doi.org/10.1186/s12859-015-0611-3, 2015.
Hector, A. and Bagchi, R.: Biodiversity and ecosystem multifunctionality, Nature, 448, 188–190, https://doi.org/10.1038/nature05947, 2007.
Hijmans, R. J., Van Etten, J., Cheng, J., Mattiuzzi, M., Sumner, M., Greenberg, J. A., Lamigueiro, O. P., Bevan, A., Racine, E. B., and Shortridge, A.: Package “raster”, R package, 734, https://cran.r-project.org/package=raster (last access: 10 September 2019), 2015.
Huerta-Cepas, J., Szklarczyk, D., Forslund, K., Cook, H., Heller, D., Walter, M. C., Rattei, T., Mende, D. R., Sunagawa, S., and Kuhn, M.: eggNOG 4.5: a hierarchical orthology framework with improved functional annotations for eukaryotic, prokaryotic and viral sequences, Nucleic Acids Res., 44, D286–D293, 2015.
Kanehisa, M., Sato, Y., Kawashima, M., Furumichi, M., and Tanabe, M.: KEGG as a reference resource for gene and protein annotation, Nucleic Acids Res., 44, D457–D462, 2015.
Kottek, M., Grieser, J., Beck, C., Rudolf, B., and Rubel, F.: World map of the Köppen-Geiger climate classification updated, Meteorol. Z., 15, 259–263, 2006.
Leff, J. W., Jones, S. E., Prober, S. M., Barberán, A., Borer, E. T., Firn, J. L., Harpole, W. S., Hobbie, S. E., Hofmockel, K. S., and Knops, J. M.: Consistent responses of soil microbial communities to elevated nutrient inputs in grasslands across the globe, P. Natl. Acad. Sci. USA, 112, 10967–10972, 2015.
Louca, S., Parfrey, L. W., and Doebeli, M.: Decoupling function and taxonomy in the global ocean microbiome, Science, 353, 1272–1277, 2016.
Louca, S., Jacques, S. M., Pires, A. P., Leal, J. S., Srivastava, D. S., Parfrey, L. W., Farjalla, V. F., and Doebeli, M.: High taxonomic variability despite stable functional structure across microbial communities, Nat. Ecol. Evol., 1, 0015, https://doi.org/10.1038/s41559-016-0015, 2017.
Louca, S., Polz, M. F., Mazel, F., Albright, M. B., Huber, J. A., O'Connor, M. I., Ackermann, M., Hahn, A. S., Srivastava, D. S., and Crowe, S. A.: Function and functional redundancy in microbial systems, Nat. Ecol. Evol., 2, 936–943, https://doi.org/10.1038/s41559-018-0519-1, 2018.
McGill, B. J., Enquist, B. J., Weiher, E., and Westoby, M.: Rebuilding community ecology from functional traits, Trend. Ecol. Evol., 21, 178–185, 2006.
Meyer, F., Paarmann, D., D'Souza, M., Olson, R., Glass, E. M., Kubal, M., Paczian, T., Rodriguez, A., Stevens, R., and Wilke, A.: The metagenomics RAST server – a public resource for the automatic phylogenetic and functional analysis of metagenomes, BMC Bioinformatics, 9, 386, https://doi.org/10.1186/1471-2105-9-386, 2008.
Moulin, L., Munive, A., Dreyfus, B., and Boivin-Masson, C.: Nodulation of legumes by members of the β-subclass of Proteobacteria, Nature, 411, 948–950, https://doi.org/10.1038/35082070, 2001.
Olesen, J. M., Bascompte, J., Dupont, Y. L., and Jordano, P.: The smallest of all worlds: Pollination networks, J. Theor. Biol., 240, 270–276, https://doi.org/10.1016/j.jtbi.2005.09.014, 2006.
Olesen, J. M., Bascompte, J., Dupont, Y. L., and Jordano, P.: The modularity of pollination networks, P. Natl. Acad. Sci. USA, 104, 19891–19896, 2007.
Overbeek, R., Olson, R., Pusch, G. D., Olsen, G. J., Davis, J. J., Disz, T., Edwards, R. A., Gerdes, S., Parrello, B., and Shukla, M.: The SEED and the Rapid Annotation of microbial genomes using Subsystems Technology (RAST), Nucleic Acids Res., 42, D206–D214, 2013.
Pan, Y., Cassman, N., de Hollander, M., Mendes, L. W., Korevaar, H., Geerts, R. H., van Veen, J. A., and Kuramae, E. E.: Impact of long-term N, P, K, and NPK fertilization on the composition and potential functions of the bacterial community in grassland soil, FEMS Microbiol. Ecol., 90, 195–205, 2014.
Peter, H., Beier, S., Bertilsson, S., Lindström, E. S., Langenheder, S., and Tranvik, L. J.: Function-specific response to depletion of microbial diversity, ISME J., 5, 351–361, https://doi.org/10.1038/ismej.2010.119, 2011.
Philippot, L., Spor, A., Hénault, C., Bru, D., Bizouard, F., Jones, C. M., Sarr, A., and Maron, P.-A.: Loss in microbial diversity affects nitrogen cycling in soil, ISME J., 7, 1609–1619, https://doi.org/10.1038/ismej.2013.34, 2013.
Pruesse, E., Quast, C., Knittel, K., Fuchs, B. M., Ludwig, W., Peplies, J., and Glöckner, F. O.: SILVA: a comprehensive online resource for quality checked and aligned ribosomal RNA sequence data compatible with ARB, Nucleic Acids Res., 35, 7188–7196, 2007.
Ramírez-Flandes, S., González, B., and Ulloa, O.: Redox traits characterize the organization of global microbial communities, P. Natl. Acad. Sci., 116, 3630–3635, 2019.
Rivett, D. W. and Bell, T.: Abundance determines the functional role of bacterial phylotypes in complex communities, Nat. Microbiol., 3, 767–772, https://doi.org/10.1038/s41564-018-0180-0, 2018.
Rocca, J. D., Hall, E. K., Lennon, J. T., Evans, S. E., Waldrop, M. P., Cotner, J. B., Nemergut, D. R., Graham, E. B., and Wallenstein, M. D.: Relationships between protein-encoding gene abundance and corresponding process are commonly assumed yet rarely observed, ISME J., 9, 1693–1699, https://doi.org/10.1038/ismej.2014.252, 2015.
Rosenfeld, J. S.: Functional redundancy in ecology and conservation, Oikos, 98, 156–162, 2002.
Rousk, J., Brookes, P. C., and Bååth, E.: Contrasting soil pH effects on fungal and bacterial growth suggest functional redundancy in carbon mineralization, Appl. Environ. Microbiol., 75, 1589–1596, 2009.
Schimel, J.: Ecosystem consequences of microbial diversity and community structure, in: Arctic and alpine biodiversity: patterns, causes and ecosystem consequences, Springer, 239–254, https://doi.org/10.1007/978-3-642-78966-3_17, 1995.
Schimel, J. P. and Gulledge, J.: Microbial community structure and global trace gases, Glob. Change Biol., 4, 745–758, 1998.
Segata, N., Izard, J., Waldron, L., Gevers, D., Miropolsky, L., Garrett, W. S., and Huttenhower, C.: Metagenomic biomarker discovery and explanation, Genome Biol., 12, R60, https://doi.org/10.1186/gb-2011-12-6-r60, 2011.
Shannon, P., Markiel, A., Ozier, O., Baliga, N. S., Wang, J. T., Ramage, D., Amin, N., Schwikowski, B., and Ideker, T.: Cytoscape: a software environment for integrated models of biomolecular interaction networks, Genome Res., 13, 2498–2504, 2003.
Souza, R. C., Hungria, M., Cantão, M. E., Vasconcelos, A. T. R., Nogueira, M. A., and Vicente, V. A.: Metagenomic analysis reveals microbial functional redundancies and specificities in a soil under different tillage and crop-management regimes, Appl. Soil Ecol., 86, 106–112, 2015.
Stephen, J. R., McCaig, A. E., Smith, Z., Prosser, J. I., and Embley, T. M.: Molecular diversity of soil and marine 16S rRNA gene sequences related to beta-subgroup ammonia-oxidizing bacteria, Appl. Environ. Microbiol., 62, 4147–4154, 1996.
Tatusova, T., Ciufo, S., Fedorov, B., O'Neill, K., and Tolstoy, I.: RefSeq microbial genomes database: new representation and annotation strategy, Nucleic Acids Res., 42, D553–D559, 2013.
Torsvik, V. and Øvreås, L.: Microbial diversity and function in soil: from genes to ecosystems, Curr. Opin. Microbiol., 5, 240–245, 2002.
Tringe, S. G., Von Mering, C., Kobayashi, A., Salamov, A. A., Chen, K., Chang, H. W., Podar, M., Short, J. M., Mathur, E. J., and Detter, J. C.: Comparative metagenomics of microbial communities, Science, 308, 554–557, 2005.
Wellington, E. M., Berry, A., and Krsek, M.: Resolving functional diversity in relation to microbial community structure in soil: exploiting genomics and stable isotope probing, Curr. Opin. Microbiol., 6, 295–301, 2003.
Wilke, A., Gerlach, W., Harrison, T., Paczian, T., Trimble, W. L., and Meyer, F.: MG-RAST manual for version 4, revision 3, Lemont, IL, Argonne National Laboratory, https://help.mg-rast.org/user_manual.html (last access: 10 September 2019), 2017.
Xu, X., Qiu, Y., Zhang, K., Yang, F., Chen, M., Luo, X., Yan, X., Wang, P., Zhang, Y., and Chen, H.: Climate warming promotes deterministic assembly of arbuscular mycorrhizal fungal communities, Glob. Change Biol., 28, 1147–1161, 2021.
Yin, B., Crowley, D., Sparovek, G., De Melo, W. J., and Borneman, J.: Bacterial functional redundancy along a soil reclamation gradient, Appl. Environ. Microbiol., 66, 4361–4365, 2000.
Zhou, J., Deng, Y., Luo, F., He, Z., and Yang, Y.: Phylogenetic molecular ecological network of soil microbial communities in response to elevated CO2, MBio, 2, e00122–00111, 2011.