the Creative Commons Attribution 4.0 License.
the Creative Commons Attribution 4.0 License.
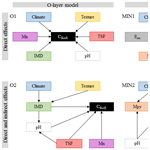
Boreal-forest soil chemistry drives soil organic carbon bioreactivity along a 314-year fire chronosequence
Benjamin Andrieux
David Paré
Julien Beguin
Pierre Grondin
Yves Bergeron
Following a wildfire, organic carbon (C) accumulates in boreal-forest soils. The long-term patterns of accumulation as well as the mechanisms responsible for continuous soil C stabilization or sequestration are poorly known. We evaluated post-fire C stock changes in functional reservoirs (bioreactive and recalcitrant) using the proportion of C mineralized in CO2 by microbes in a long-term lab incubation, as well as the proportion of C resistant to acid hydrolysis. We found that all soil C pools increased linearly with the time since fire. The bioreactive and acid-insoluble soil C pools increased at a rate of 0.02 and 0.12 MgC ha−1 yr−1, respectively, and their proportions relative to total soil C stock remained constant with the time since fire (8 % and 46 %, respectively). We quantified direct and indirect causal relationships among variables and C bioreactivity to disentangle the relative contribution of climate, moss dominance, soil particle size distribution and soil chemical properties (pH, exchangeable manganese and aluminum, and metal oxides) to the variation structure of in vitro soil C bioreactivity. Our analyses showed that the chemical properties of podzolic soils that characterize the study area were the best predictors of soil C bioreactivity. For the O layer, pH and exchangeable manganese were the most important (model-averaged estimator for both of 0.34) factors directly related to soil organic C bioreactivity, followed by the time since fire (0.24), moss dominance (0.08), and climate and texture (0 for both). For the mineral soil, exchangeable aluminum was the most important factor (model-averaged estimator of −0.32), followed by metal oxide (−0.27), pH (−0.25), the time since fire (0.05), climate and texture (∼0 for both). Of the four climate factors examined in this study (i.e., mean annual temperature, growing degree-days above 5 ∘C, mean annual precipitation and water balance) only those related to water availability – and not to temperature – had an indirect effect (O layer) or a marginal indirect effect (mineral soil) on soil C bioreactivity. Given that predictions of the impact of climate change on soil C balance are strongly linked to the size and the bioreactivity of soil C pools, our study stresses the need to include the direct effects of soil chemistry and the indirect effects of climate and soil texture on soil organic matter decomposition in Earth system models to forecast the response of boreal soils to global warming.
- Article
(1028 KB) - Full-text XML
-
Supplement
(448 KB) - BibTeX
- EndNote
Soil is the largest terrestrial carbon (C) reservoir (Scharlemann et al., 2014) and a major source of uncertainty in ecosystem C predictions (Shaw et al., 2014). Therefore, an advanced mechanistic understanding of soil C processes needs to be investigated and integrated into forecast models to reduce uncertainties in global C cycle feedback projections and to better predict the effects of global change on the soil C reservoir (Bradford et al., 2016; Schmidt et al., 2011). The maintenance of the vast soil C reservoir is partly under microbial control (Cotrufo et al., 2013) and could respond to variations in environmental conditions (Davidson and Janssens, 2006). Hence, the C quality-temperature hypothesis states that more “recalcitrant” soil organic matter should have a higher temperature sensitivity (Craine et al., 2010; Fierer et al., 2005). According to this hypothesis, it is important to distinguish the recalcitrant portion of the soil organic matter from the active portion in order to predict the impact of a rise in temperature on soil heterotrophic respiration. Furthermore, the bioreactive C fraction of soil organic matter is cycled on timescales relevant to global warming. Thus, quantifying the size of the bioreactive soil C reservoir and understanding the controlling factors of soil C bioreactivity (CBioR) are key to inform models of the C cycle in the face of and to better anticipate global warming.
Wildfire is a major natural disturbance in boreal forests that drives the ecosystem C balance (Bond-Lamberty et al., 2007; Kurz et al., 2013) and is known to impact several soil properties, including organic matter quantity and quality (Certini, 2005; Knicker, 2007). Key soil properties, some evolving following a fire (e.g., soil acidity) and some not (e.g., particle size distribution), interact with climate and vegetation composition in complex causal direct and indirect relationships to regulate post-fire soil C accumulation (Andrieux et al., 2018). A saturation of soil C accumulation, especially for its recalcitrant portion, is often observed in soils when the rates of organic matter input to the soil are increased (Stewart et al., 2007; Hassink, 1996). Saturation of recalcitrant C is believed to come from the finite capacity of stabilization mechanisms in soils, such as chemical protection from the decomposition of soil organic matter by clay surfaces (Six et al., 2002). At a steady state and when the accumulation of physicochemically stabilized soil C occurs, soil C can only accumulate as nonprotected C (Castellano et al., 2015) that is more prone to decompose quickly. However, the long-term patterns of change in soil C quality and the accumulation pattern of recalcitrant and bioreactive C pools as well as the mechanisms responsible for continuous accumulation or the stabilization of soil C reservoirs are poorly known and have not been explicitly integrated into soil biogeochemistry (Luo et al., 2016). Most models of soil C dynamics divide soil organic matter into several conceptual pools and simulate decomposition as a first-order decay process (Luo et al., 2016). As part of this study, we characterized the acid-insoluble and bioreactive soil organic C pools (Cslow and Cfast, respectively; expressed as stocks) that accumulate following a wildfire. The acid-insoluble soil C fraction is assumed to be recalcitrant or resistant to biological degradation (Paul et al., 2006; Xu et al., 1997). Hereafter, we define CBioR as the proportion of C mineralized in CO2 by microbes at a constant temperature and constant water content over a long period of time as a relative measure of soil C lability (Laganière et al., 2015; Xu et al., 1997). Assessing the sizes of resistant and bioreactive soil C fractions through a fire chronosequence would help modelers to enhance the current and future C balance of landscapes prone to wildfires.
Besides its direct role in C cycling, climate has been shown to be an indirect predictor of soil C storage (quantity and accessibility for microbial decomposition) through its effects on geochemistry (Doetterl et al., 2015). In addition, vegetation types determine the quantity, quality and vertical distribution of soil litter inputs and so lead to differential mechanisms of soil C protection and stabilization (Jobbagy and Jackson, 2000; Laganière et al., 2017), with the moss stratum being a major source of soil C inputs in boreal ecosystems (Preston et al., 2006). Although many of these processes have been investigated separately, we are not aware of any empirical study so far that has quantified all these processes simultaneously and assessed the relative contribution of climate, time since fire (TSF), vegetation attributes and soil physicochemistry to soil CBioR.
The objectives of this study are to fill these knowledge gaps by quantifying changes in boreal-forest soil bioreactive C stock with TSF (from 2 to 314 years) and disentangling the direct and indirect relative contributions of climate, moss dominance, soil particle size distribution and soil chemical properties (pH; exchangeable manganese, Mn, and aluminum, Al; and metal oxides) to soil CBioR across the spruce–feather moss bioclimatic domain in eastern North America. Focusing on the complex interplay between climatic and non-climatic factors and their direct or indirect influence on soil CBioR, we addressed the following questions. (i) Does soil CBioR reservoir change with the soil organic C accumulation observed with TSF? (ii) To what extent do direct and indirect relationships among TSF, climate, physicochemical soil properties and bryophyte dominance influence soil CBioR? We framed our study within the state factor model of ecosystems (Amundson and Jenny, 1997), which emphasizes soil physicochemical properties understood to be important to the pedogenesis of podzolic soils (Schaetzl and Anderson, 2005) that occur on the sampled sites. From there, our general hypotheses are that once site factors such as overstory composition, surficial deposits and soil drainage are accounted for, as they were in the present study, (1) soil CBioR increases as forest stands get older, leading to a buildup of soil bioreactive C stock under the cold conditions of the boreal forest; (2) alternatively, if the bioreactive soil C stock reaches a new equilibrium because of rapid turnover, the proportion of bioreactive C stock should decline as total soil C stocks increases with TSF; and (3) soil CBioR is primarily controlled by TSF and moss dominance in the O layer and by soil physicochemistry in the mineral soil (see Sect. 2.3 for detailed hypotheses).
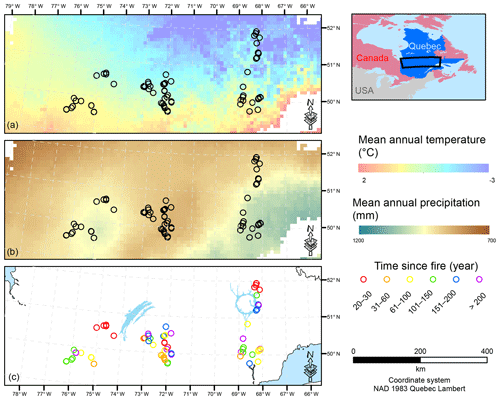
Figure 1Map of the study area showing the location of the sample plots. Mean annual temperature (a) and mean annual precipitation (b) are interpolations from the 1981–2010 Canadian climate normals on a 10×10 km pixel grid (Chaste et al., 2018). The lower panel presents the location of the sample plots in relation to the time since fire (year). NAD: North American Datum.
2.1 Site selection, sampling design and fieldwork
To account for the effects of TSF and climate on soil C pools, we established sample plots across both a chronosequence and a climosequence (Fig. 1; see Andrieux et al., 2018, for a description of the study area). Using numeric forest inventory maps compiled by the Ministère des Forêts, de la Faune et des Parcs du Québec (MFFPQ), we selected stands with as many similarities as possible in terms of canopy composition (black spruce – Picea mariana – stands), surficial deposits (thick till) and mesic drainage conditions. The soils that develop under these cool and humid climate conditions with acidic litter inputs typically belong to the podzolic order (Table 1). In boreal forests, podzolic soils often have a thick organic surface horizon (O layer), an eluviated A horizon (Ae) from where leached materials accumulate in the illuviated B horizon (Fig. S1). Within mesic drainage conditions, soil texture was quite variable (Table 1). These stands were overlaid with fire maps produced by the MFFPQ and other published dendrochronological surveys (Belisle et al., 2011; Bouchard et al., 2008; Cyr et al., 2012; Frégeau et al., 2015; Le Goff et al., 2007, 2008; Portier et al., 2016) to establish the chronosequence. We assumed that the canopy composition of black spruce did not change significantly with time and that the forest cyclically returned to the dominance of black spruce after a fire, in so-called recurrent dynamics, as previously described for these forests in a paleological survey (Frégeau et al., 2015). Then, while studying this ecosystem with a single and cyclic successional trajectory and low vegetation diversity and by carefully selecting stable permanent site conditions, we guarded against pitfall conclusions associated with space–time substitutions when using a chronosequence approach (Walker et al., 2010; Kenkel et al., 1997).
For field inventory and soil sampling, we followed Canada's National Forest Inventory ground plot guidelines (Canadian Forest Service, 2008). Stand biophysical description and soil sampling took place in a single 314 m2 circular plot (10 m radius) in each stand. Slope inclination and orientation were recorded from the center of each plot with a clinometer and a compass, respectively. Every 2 m along two orthogonal transects oriented following the main cardinal directions and for a total of 20 records per plot, the thickness of the O layer was measured on a sample taken with a soil auger, and the dominant moss types (Sphagnum spp. or feather mosses) were identified using 400 cm2 microplots (Fig. S1 in the Supplement). After litter and green living mosses were removed, the O layer was sampled at the edge of the plot in three 400 cm2 microplots that were spaced 15 m from each other, from which we extracted volumetric mineral soil samples (top 15 cm) with a metallic cylinder (diameter of 4.7 cm; height of 15 cm). One soil pit was dug at the plot edge and at the same location where we sampled one of the three O-layer samples, down to the bottom of the podzolic B horizon or to the bedrock when possible, for a soil description and to collect the mineral soil from 15 to 35 cm under the forest-floor–mineral-soil boundary, as well as in the top 15 cm B horizon with a metallic cylinder (diameter of 4.7 cm). The significant stone content at one site prevented us from sampling the mineral soil from 15 to 35 cm; thus, no analyses could be provided for this layer of soil. Samples were kept in the dark and brought to the lab within 15 d for each region. They were kept in the dark at 2 ∘C until processing during the fall.
The fieldwork was conducted in 2015, from 15 June to 8 September. The sampling effort covered 72 sites in black-spruce-dominated stands where a fire had burned 2 to 314 years ago. Climate data were interpolated at the plot level using BioSim v10.3.2 (Régnière et al., 2013) together with 1981–2010 climate normal series (http://climat.meteo.gc.ca/, last access: 12 November 2018) from surrounding weather stations and considering local slope attributes measured in the field as correcting factors (Régnière, 1996). Soil characteristics are summarized below (Table 1).
2.2 Laboratory analyses
2.2.1 Soil preparation
First, we prepared a composite of soil materials obtained from every sampled microplot (N=3), by plot and soil layer (O layer or the top 15 cm of mineral soil), to create representative samples for each layer in each of the 72 sample plots. O-layer samples were sieved through a 6 mm mesh before being oven-dried (60 ∘C), whereas mineral soil samples (either the top 15 cm of the mineral soil, mineral soil from 15 to 35 cm or the top 15 cm of the B horizon) were dried by air and passed through a 2 mm sieve. Bulk density was determined after weighing the dried samples, assuming there were no coarse fragments in the O layer, and corrected for fragments >2 mm for the mineral soil. A part of each sample was retained for soil incubation. We used the <2 mm fraction to determine pH, exchangeable cation and texture (mineral soil only for the latter). Finely ground subsamples (<0.5 mm) were used for C concentration, pyrophosphate-extractable Fe and Al (top 15 cm of the B horizon only), and acid hydrolysis analyses.
2.2.2 Soil physicochemistry
C concentration of each sample was analyzed by dry combustion (Skjemstad and Baldock, 2007) using a LECO TruMac (LECO Corp, St. Joseph, MI, USA). Exchangeable cations were extracted using a Mehlich 3 solution and were analyzed by inductively coupled plasma atomic emission spectroscopy (Ziadi and Sen Tran, 2007), using an Optima 7300 DV (Perkin Elmer Inc., Waltham, MA, USA). Pyrophosphate-extractable Fe and Al (i.e., organically complexed metals; Mpy, hereafter defined as metal oxides) were extracted with a 0.1 N Na4P2O7 solution before analysis with the Optima 7300 DV (Courchesne and Turmel, 2007). O-layer and mineral soil pH were determined in a soil : water solution by weights of 1:10 and 1:2 (Hendershot and Lalande, 2007), respectively, using a pH meter (Orion 2 Star). Particle size distribution of the mineral soil was assessed using a standard hydrometer method (Kroetsch and Wang, 2007).
2.2.3 Incubation settings
Soil incubation followed the method described in Paré et al. (2011). We prepared a total of 215 microcosms (72 sites for three soil layers minus one sample in the 15–35 cm mineral soil). We used 9 g of oven-dried O-layer and 50 g of air-dried mineral soil. Dried soil was used to ensure that the initial incubation moisture conditions were similar. Soil samples were placed in 100 mL bottom-perforated plastic containers. The containers were previously filled with glass wool (to avoid material losses during moisture adjustments) and prewashed with HCl (0.1 M) followed by deionized water. The microcosms were saturated with deionized water, drained for 24 h at 2 ∘C and weighed to determine their water-holding capacity. Over approximately 50 weeks of experiments, microcosms were placed under constant air temperature (26 ∘C) and humidity (100 %) in a growth chamber, and, when necessary, deionized water was periodically added to adjust the soil moisture to 85 % of the water-holding capacity. Except during CO2 production measurements, each microcosm was stored in a 500 mL Mason jar kept open to maintain aerobic conditions and to prevent CO2 accumulation to toxic levels. A rubber septum was installed on the metal lid for gas sampling when measuring CO2 production.
2.2.4 CO2 production measurements
CO2 produced by each microcosm was measured periodically (at days 20, 26, 48, 64, 108, 126, 154, 227, 264 and 340 for the O layer and at days 8, 14, 21, 29, 36, 43, 51, 57, 72, 79, 86, 101, 113, 140, 203, 238 and 358 for mineral soil layers; Fig. S2), using a LI-6400 portable photosynthesis system (LI-COR, Lincoln, NE, USA) connected to an N2 carrier gas (LI-COR application note 134). The flow rate of the carrier gas was set to 100 mL min−1 using the gas flow meter FMA1812A (Omega Engineering, Inc., Norwalk, CO, USA). Initial CO2 measurements were taken directly after hermetically sealing a jar with a metal lid, and final CO2 measurements were taken after 4 to 24 h, depending on the soil layer and the progress of the experiment; these measurements were carried out using 2.5 or 10 mL (for O-layer or mineral soil, respectively) of air volume, extracted from the jar headspace with a syringe through the rubber septum. This gas sample was injected through the carrier gas into the LI-6400 infrared analyzer. The CO2 concentration (µmol mol−1) was predicted using the linear regression of a sample's measured CO2 peak against calibration curves obtained from a benchmark gas (CO2 at 800 and 3000 ppm). The first measurement (initial CO2 concentration) accounted for the CO2 concentration of the ambient air when closing the jars. This value was subtracted from the final CO2 concentration to account solely for the CO2 produced by the microcosm.
All data were subsequently standardized to a 24 h period to provide a daily respiration rate and to calculate cumulative C mineralization (Fig. S2; Paré et al., 2006). In short, we applied the gas law to convert CO2 concentration (µmol mol−1) to a C mass basis, using a constant pressure at 101.3 kPa and the specific head space volume of each sample (total volume of the jar minus soil volume and container). Cumulative respiration was calculated according to the following Eq. (1):
where Mt (mg CO2-C) is the cumulative mass of C mineralized at time t, Rt (mg CO2-C d−1) is the daily respiration rate at time t and t is the Julian day (d). Mt was divided by the initial C mass (g) of each sample to compute the specific respiration rate (Rs; mg CO2-C g−1 Corg). Then, dividing Rs by 10 gave the percentage of the initial mass of soil C lost through microbial respiration or CBioR.
2.2.5 Acid hydrolysis
We used acid hydrolysis as an index of biologically recalcitrant soil C (Xu et al., 1997), which has been proposed as an indicator of a slowly cycling soil C pool (Paul et al., 2006). Hydrolysis was carried out by refluxing 2 g of soil with 50 mL HCl (6 M) brought to the boiling point on a hot plate. We used a 2 h reaction time because the majority of soil organic matter is hydrolyzed during the first 2 h, and longer reaction times do not significantly change C release (Silveira et al., 2008; Xu et al., 1997). Acid-insoluble residues were separated from hydrolysates by filtering the solution on inert paper filters, rinsed three times with 50 mL of deionized water to remove any chlorine residues, oven-dried at 60 ∘C overnight and weighed before C concentration analysis by dry combustion (see Sect. 2.3.2). Based on the total C concentration of the acid-insoluble residues and mass loss of the samples during the treatments, the hydrolysability (Plante et al., 2006) of a sample was calculated based on the following Eq. (2):
where CAI is the percentage of the acid-insoluble C (%), [CAI] and [Ci] are the C concentration of the acid-insoluble residues and of the initial soil (%), and MAI and Mi are the masses of the acid-insoluble residues and of the initial soil sample (g), respectively.
2.3 Ecological a priori hypotheses
According to our third general hypothesis and to address the complex interplay among climatic and non-climatic factors, we first selected the following environmental variables documented in the literature as being important drivers of soil CBioR and the pedogenesis of podzolic soils: climate (temperature and water supply), soil texture, TSF, dominance of the moss functional type, soil pH, and the concentration of metal oxides and of exchangeable elements (Mn and Al; Wiesmeier et al., 2019; Schaetzl and Anderson, 2005). As in other ecosystems (Fierer et al., 2003; Salomé et al., 2010), the boreal-forest soil microbial community as well as the chemical and physical environment change with soil depth (Clemmensen et al., 2013; Hynes and Germida, 2013), suggesting different drivers of the decomposition process in the O layer and in the mineral soil layer. Hence, for each of these layers, we built two separate sets of a priori ecological hypotheses expressed as direct acyclic graphs (DAGs) representing different causal relationships among environmental variables and soil CBioR (Fig. 2). Therein, we tested the validity of four competing a priori ecological hypotheses represented by a DAG. This hypothetico-deductive approach, in which each a priori hypothesis was supported by ecological knowledge, allows for testing an alternative causal explanation in a falsifiable form regarding the underlying mechanisms of soil CBioR in the two soil layers. For each soil layer, the first hypothesis assumed only direct relationships between environmental variables and soil CBioR (baseline hypotheses for the O layer and the mineral layer, O1 and MIN1 in Fig. 2) such that they mirrored the widespread assumptions used in soil C prediction models based on multiple regression or ANOVA analyses. In addition, framed within Jenny's factor model of soil formation (Jenny, 1994), these baseline hypotheses assumed independence among environmental variables. Alternatively, we formulated a priori competing hypotheses in which both direct and indirect effects among variables and soil CBioR were explicit (alternative hypotheses for the O layer and the mineral layer, O2 and MIN2 in Fig. 2). Justifications for each a priori ecological hypothesis are listed below.
2.3.1 Baseline hypothesis for the O layer (O1)
This hypothesis assumes that soils that have developed under cooler conditions limiting microbial activity should have greater CBioR (Laganière et al., 2015) once temperature constraints have been removed. Rainfall under good drainage conditions (such as in this study) should promote greater decomposition rates and hence lower CBioR. Because wildfire induces polymerization and polycondensation of organic compounds, resulting in residues that are more resistant to biological degradation (Certini, 2005; Gonzalez-Perez et al., 2004; Knicker, 2007), TSF is expected to have a direct and positive effect on CBioR which was anticipated to increase with TSF. Soil pH also had a direct effect on soil bioreactivity because it regulates the microbial community (Fierer and Jackson, 2006) and is a key determinant of the decomposition process (Prescott et al., 2000; Zhang et al., 2008). We expected decreasing CBioR with decreasing pH because acidic soil conditions limit the activity of soil decomposers. Compared to Sphagnum spp., feather mosses are more palatable to microbes (Fenton et al., 2010; Lang et al., 2009), so we expected lower CBioR with more Sphagnum. Also, Mn availability has been shown to be a good predictor of boreal-soil C stocks (Stendahl et al., 2017); hence, with Mn being a co-metabolic compound of lignin degradation, we assumed that Mn has a direct positive effect on CBioR.
2.3.2 Alternative hypothesis for the O layer (O2)
As in hypothesis O1, TSF, pH, moss functional type and Mn had direct effects on C bioreactivity. However, this hypothesis differed from O1 in that TSF and moss dominance also had indirect effects on CBioR through changes in pH conditions. We expected decreasing pH with increasing Sphagnum spp. dominance because some physiological characteristics of these organisms lead to environment acidification (Andrus, 1986). Also, in the short term, fire modifies pH through the liming effect (Gonzalez-Perez et al., 2004; Knicker, 2007). In the long term, soils acidify with TSF as a result of vegetation regrowth, which involves the exchange of protons against cations to maintain the physiological electroneutrality of the vegetation (Driscoll and Likens, 1982). Contrary to hypothesis O1, which postulated that climate and soil texture had direct effects on CBioR, hypothesis O2 assumed that these drivers had only indirect effects on CBioR through their influence on moss dominance. Indeed, we expected that Sphagnum spp. would dominate over feather mosses under wetter conditions induced by greater precipitation, fined-texture soils holding more water or both because of their greater dependence to high soil water content.
2.3.3 Baseline hypothesis for the mineral soil (MIN1)
This hypothesis assumes that there are only direct effects of environmental variables on CBioR in the mineral soil. Climate and pH directly control the decomposition process. As the binding of organic matter with the mineral phase has been recognized as an important mechanism of C protection against decomposition (Doetterl et al., 2015; Kaiser et al., 2002; Porras et al., 2017), we assumed that there would be direct effects of soil texture and metal oxide contents on CBioR. In the first years following a fire, the slow incorporation of charred residues from upper soil layers into the mineral soil could decrease the organic matter quality (Johnson and Curtis, 2001), resulting in a decrease of CBioR with increasing TSF. Mn availability could directly modulate CBioR (see O1), and exchangeable Al could impede microbial decomposition when in excess (Kunito et al., 2016).
2.3.4 Alternative hypothesis for the mineral soil (MIN2)
As an alternative to hypothesis MIN1, this hypothesis assumes that only TSF, pH, metal oxides, and Mn and Al have direct effects on CBioR. Additionally, pH is assumed to decrease with TSF because of the imbalance in nutrient uptake caused by aggrading vegetation. Also, exchangeable cations are dependent on pH (Sanborn et al., 2011), and the decrease in pH favors the creation of organometallic complexes impeding microbial decomposition (Buurman and Jongmans, 2005; Porras et al., 2017). Contrary to hypothesis MIN1, which assumed direct effects of climate and soil texture on CBioR, this hypothesis assumes that climate and soil texture have only indirect effects on CBioR. The indirect effect of climate on CBioR is mediated through its effect on mineral weathering (Doetterl et al., 2015) and the quantity of metal oxides leached from the upper soil layers (Schaetzl and Anderson, 2005). Compared to coarse-textured soils, fine-textured soils have more reactive surface sites that can bind additional Mn and Al ions (Petersen et al., 1996).
2.4 Calculations and data analyses
2.4.1 Index of moss dominance
In order to account for the effects of moss functional traits on CBioR of the O layer, we differentiated between Sphagnum spp. and feather mosses, since they have different ecophysiological characteristics (Bisbee et al., 2001); e.g., feather mosses decompose faster than Sphagnum spp. (Fenton et al., 2010; Lang et al., 2009). Based on Nalder and Wein (1999), we calculated an index of moss dominance (IMD) using Eq. (3):
where O is the sum of occurrence of a species in the 20 microplots (see Sect. 2.1), sph is Sphagnum spp., pl is Pleurozium schreberi (Brid.) Mitt., h is Hylocomium splendens (Hedw.) Schimp. and pt is Ptilium crista-castrensis (Hedw.).
Feather mosses dominate the moss stratum when the IMD tends toward 0, whereas Sphagnum spp. dominates the moss stratum when the IMD tends toward 1. Some sites (n=5) that recently had fires did not have any moss species regrowth at the time of the fieldwork. For these sites, we set the IMD to 0.
2.4.2 Soil C quality and bioreactivity
First, we wanted to estimate variation in the size of the bioreactive and recalcitrant soil C pools (Cfast and Cslow, respectively, expressed as stocks) with TSF. For each soil layer (O layer, the top 15 cm of mineral soil and 15 to 35 cm of mineral soil), we scaled up to the plot scale of the cumulative proportion of C mineralized at the end of the incubations and the proportion of acid-insoluble C using Eqs. (4) and (5):
where Cfast and Cslow are the bioreactive and recalcitrant soil C pools (Mg ha−1), CBioR is the percentage of the initial mass of soil C lost through microbial respiration (%), C is soil C content (%), DB is the bulk density (g cm−3), h is the soil depth (i.e., mean depth based on 20 measurements per plot for the O layer; cm) and CAI is the acid-insoluble C fraction (%). Hereafter, the total C stock (Ctot), Cfast or Cslow pool size represents, within each plot, the sum of each C pool across all soil layers.
Secondly, in order to express the qualitative (relative) changes in soil C in relation to environmental variables, we used the proportion of the initial mass of soil C lost through microbial respiration as an index of C lability (see Sect. 2.2.4). In the statistical analyses, we considered the whole mineral soil (in the top 15 cm and from 15 to 35 cm) as a single soil layer by calculating the weighted mean by depth for all mineral soil variables.
2.4.3 Statistical analyses
First, we evaluated post-fire C stock changes in functional reservoirs (bioreactive versus recalcitrant) using the linear regression of C stocks against TSF. Preliminary analyses with generalized additive models and piecewise regressions did not show any significant nonlinear or segmented relationships. Secondly, we quantified direct and indirect causal relationships among variables and CBioR using confirmatory path analysis with directional-separation tests (Shipley, 2000a), according to the set of alternative a priori hypotheses (Fig. 2). Path analysis was used together with Fisher's C test (Shipley, 2000b) as a simultaneous test of independence for a model basis set (i.e., all nonadjacent pairs of variables defined as claims of independence). This led us to quantify how our data supported each hypothetical DAG and to identify whether some hypotheses would be rejected based on a robust statistical test (Shipley, 2009). Fisher's C statistic was compared with a χ2 distribution with 2 k degrees of freedom (where k is the number of claims of independence in a model basis set). We rejected a causal model at the significance level α=0.05 when p<α. Prior to analyses, we standardized (reduced and centered) all variables to quantify their relative contribution to the variability of soil CBioR.
The fit of each DAG for every soil layer (O and mineral soil) was compared using a model selection approach together with the second-order Akaike information criterion (AICc) in order to account for small sample sizes (Shipley, 2013). For model selection, we used the relative AICc difference with the “best” model or relative weight (Symonds and Moussalli, 2010). To avoid having latent variables in the models and because we had no a priori knowledge about which specific climate, texture or exchangeable elements should be used for testing the validity of each hypothesis, we used the cross product of four climatic variables (mean annual temperature: MAT; growing degree-day above 5 ∘C: GDD5; mean annual precipitation: MAP; and water balance: WB), three soil texture variables (sand percentage, silt percentage and clay percentage) and two exchangeable elements (Al and Mn, only for mineral soil). Therefore, we tested 12 and 24 model combinations for each hypothesis and DAG involving the O layer and the mineral soil, respectively. Given that each soil layer had two alternative causal hypotheses, we then compared 24- and 48-candidate DAG models using a model selection procedure for the O layer and mineral soil, respectively. Model-averaged estimates were calculated by multiplying each estimate within each model with the corresponding Akaike weight and by summing the resulting values across all models; this allowed all models to influence model-averaged estimates. By doing so, we guarded against making arbitrary decisions about which model should be considered. We used the “ggm” package to compute Fisher's C statistic (Marchetti et al., 2015). All calculations and statistics were made using the R software version 3.4.3 (R Core Team, 2017).
Table 2Post-fire soil C pool size and accumulation rates.
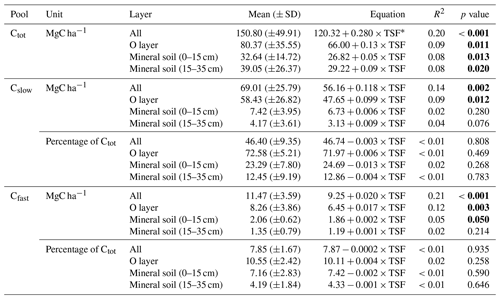
∗ TSF: time since fire (year). p values <0.05 in bold.
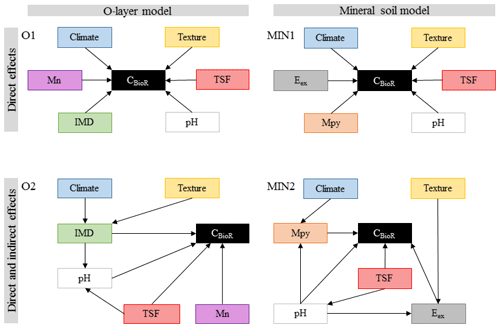
Figure 2Path models for each of the multivariate causal hypotheses. Arrows indicate direct causal relationships. Climate: climate variable; texture: texture variable; TSF: time since fire; pH: pH of the O layer (O1 and O2) or of the top 35 cm of mineral soil (MIN1 and MIN2); IMD: index of moss dominance; Mn: exchangeable manganese of the O layer; Mpy: pyrophosphate-extractable metals; Eex: exchangeable element (Al or Mn) of the mineral soil.
3.1 Post-fire soil C pool size
Total soil C stock (Ctot, i.e., the sum of O-layer and mineral C stocks in the top 35 cm), the size of the recalcitrant C pool and the size of the bioreactive C pool (Cslow and Cfast, respectively) all increased linearly with TSF (Fig. 3a). A minimum Ctot value of 63 MgC ha−1 was observed for a 100-year-old stand, which is close to the Ctot value of 66 MgC ha−1 of the youngest (2-year-old) stand. A maximum Ctot value of 305 MgC ha−1 was observed for a 283-year-old stand. On average, the Cslow size was 6-fold bigger than the Cfast size (Table 2). Cfast values ranged from 5 to 25 MgC ha−1 for a 100-year-old stand and for a 91-year-old stand, respectively. Cslow values were 29 and 175 MgC ha−1 for a 2-year-old stand and for a 91-year-old stand, respectively.
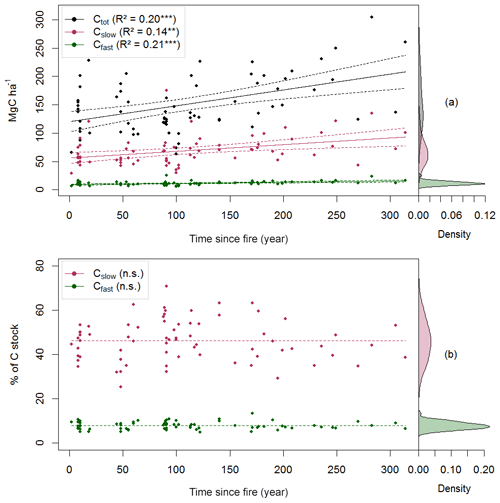
Figure 3Carbon quality as a function of the time since fire (TSF). The upper panel shows the total soil C reservoir (Ctot), the recalcitrant C pool (Cslow) and the bioreactive C pool (Cfast) sizes as a function of TSF (a, on the left), and the kernel density for each pool (a, on the right). The lower panel shows the proportion of Cslow and Cfast relative to Ctot as a function of TSF (b, on the left) and their kernel density (b, on the right). p<0.001; p<0.01; n.s. p>0.05.
Using these simple linear trends, Ctot accumulated faster than Cslow and Cfast (F, p<0.001; Table 2 and Fig. 3b). Our data indicate that the overall soil C quality did not vary quantitatively with TSF (R2<0.01, p≥0.81 for both C pools; Table 2) because the proportion of Cslow and Cfast remained constant over the time span of the fire chronosequence (Fig. 3b). These general trends are mostly influenced by the size of the Cslow and Cfast pools of the O layer, being 5 times and 2.4 times larger than the top 35 cm of the mineral soil one (i.e., sum of the two mineral soil layers), respectively (Table 2; Fig. S3). Cslow and Cfast decrease with soil layers from the surface soil horizon (O layer) to the deeper mineral soil, both in absolute size and proportion (Table 2; Fig. S3). Consistently with the whole dataset, the proportion of Cslow and Cfast do not vary quantitatively with TSF for all the soil layers analyzed separately (Table 2). The size of the Cslow pool increases linearly with TSF in the O layer only (R2=0.09, p=0.01), not in mineral soil layers (p>0.07 for both mineral soil layers). The size of the Cfast pool increases linearly with TSF in the O layer (R2=0.12, p=0.003) and in the top 15 cm of the mineral soil (R2=0.05, p<0.05), not in the deepest mineral soil layer from 15 to 35 cm (p>0.21).
Table 3Model fitness to the data for a priori hypotheses for the O layer. Models are sorted by the increasing second-order Akaike information criterion (AICc).
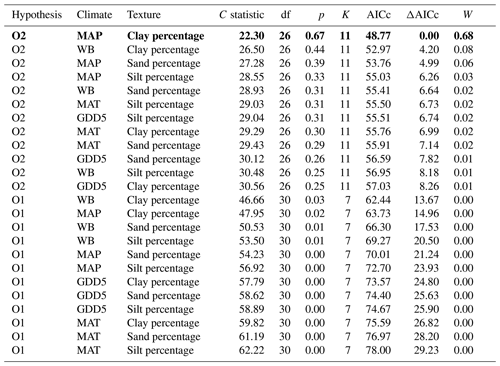
Hypothesis: model name; climate: climate variable; texture: texture variable; C statistic: statistic for Fisher's C test; df: degree of freedom; p: p value for Fisher's C test (when p<0.05, the model is not supported by the data); K: number of free parameters; AICc: second-order Akaike information criterion; ΔAICc: relative AICc difference with the model that best fitted the data (in bold); W: Akaike weight. MAT: mean annual temperature; GDD5: growing degree-day above 5 ∘C; MAP: mean annual precipitation; WB: water balance.
3.2 O-layer path analysis and model selection
The causal structure of the O1 baseline hypothesis, which assumes that there are only direct effects of covariates on CBioR in the O layer, was rejected for all candidate models (Fisher's C statistic >45, p<0.05; Table 3). Instead, the data better supported the causal structure of the O2 alternative hypothesis for all models (Fisher's C statistic <31, p>0.25; Table 3), which indicates that indirect effects among covariates and CBioR in the O layer need to be accounted for in order to properly assess the variation structure in the data. The model selection procedure revealed that the data were best explained by one leading model (hereafter, the best model; Fisher's C statistic = 22.3, p=0.67; Table 3); this model was associated with O2, with MAP as the climate variable and clay content as the texture variable. The Akaike weight for this model (68 %) was about 8 times greater than the weight of the second-most supported model (8 %). The model-averaging procedure revealed that exchangeable Mn and pH of the O layer were the two covariates that had the strongest direct and positive effects on CBioR of the O layer (both with an averaged path coefficient of pc = 0.34, p<0.01; Fig. 4 and Table S1). TSF was the second-most important relative driver with a significant direct and positive effect on CBioR (pc = 0.24, p<0.05; Fig. 4 and Table S1 in the Supplement). Moss dominance had no significant direct effects on CBioR (pc = 0.08, p>0.41; Fig. 4 and Table S1). In addition, both TSF and moss dominance had indirect effects on CBioR through their influences on pH (TSF→pH: pc = −0.32, p<0.01; moss dominance→pH: pc = 0.30, p<0.01; Fig. 4 and Table S1). In addition, the model contained an indirect effect of climate (MAP) on CBioR through its direct and negative effect on moss dominance (pc = −0.25, p<0.05; Fig. 4 and Table S1). We detected no any effect of texture (clay content) of the mineral soil on moss dominance (−0.01 < pc < 0.05., p>0.43).
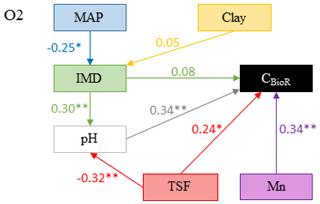
Figure 4Model that best fitted the data to explain carbon bioreactivity (CBioR) in the O layer. Arrows indicate direct causal relationships. The numbers are standardized averaged path coefficients obtained using model averaging (see Table S1 and text for further details). MAP: mean annual precipitation; clay: clay content in the top 35 cm of mineral soil; Mn: exchangeable manganese; pH: pH of the O layer; TSF: time since fire; IMD: index of moss dominance. ; .
By allowing all of the models (O1 and O2) to influence coefficient estimates, the model-averaging procedure indicated that the most important variables exerting a direct control over CBioR of the O layer were as follows, by decreasing importance: pH and Mn, TSF, and moss dominance (Table S1). Moreover, we could not detect any direct effect of climatic and texture variables tested in this study on CBioR in the O layer.
Table 4Model fitness to the data for a priori hypotheses for the mineral soil. Models are sorted by the increasing second-order Akaike information criterion (AICc).
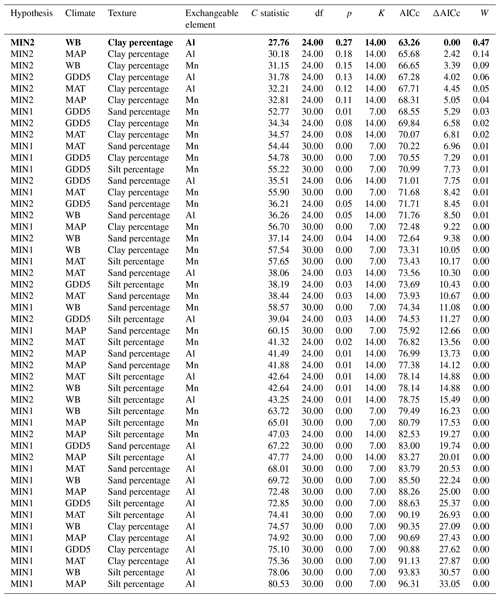
Hypothesis: model name; climate: climate variable; texture: texture variable; exchangeable element: exchangeable-element variable; C statistic: statistic for Fisher's C test; df: degree of freedom; p: p value for Fisher's C test (when p<0.05, the model is not supported by the data); K: number of free parameters; AICc: second-order Akaike information criterion; ΔAICc: relative AICc difference with the model that best fitted the data (in bold); W: Akaike weight. MAT: mean annual temperature; GDD5: growing degree-day above 5 ∘C; MAP: mean annual precipitation; WB: water balance.
3.3 Mineral soil path analysis and model selection
The causal structure of the MIN1 baseline hypothesis, which assumed that there were only direct effects of covariates on the CBioR of the mineral soil, was rejected (Fisher's C statistic >52, p<0.05; Table 4). Instead, the data best supported the causal structure implied by the MIN2 alternative hypothesis indicating that, similar to the O layer, indirect effects among covariates need to be accounted for in order to properly assess variation in the CBioR of the mineral soil. The model selection procedure revealed that the data were best explained by one leading model (hereafter, the best model; Fisher's C statistic = 27.76, p=0.27; Table 4). This model was associated with MIN2, with WB as the climate variable, clay content as the texture variable and Al as the exchangeable-element variable. The Akaike weight for this model (47 %) was about 3 times greater than for the second-most supported model (14 %). The model-averaging procedure revealed that exchangeable Al had the strongest direct and negative effect on the CBioR of the mineral soil (pc = −0.32, p<0.001; Fig. 5). Metal oxide content (pc = −0.27, p<0.05) and pH (pc = −0.25, p<0.05) were the second-most influential drivers with significant and negative direct effects on the CBioR of the mineral soil. TSF had a small positive direct effect on the CBioR of the mineral soil, but this relationship was not significant (pc = 0.05, p>0.36). In addition, pH induced two indirect effects on the CBioR of the mineral soil, i.e., through its negative and direct effects on Al and Mpy (pH→Al: pc = −0.24, p<0.01; pH→Mpy: pc = −0.34, p<0.01). Clay content had an indirect effect on the CBioR of the mineral soil, through its direct and positive effect on exchangeable Al (pc = 0.17, p<0.05). Also, water balance had a weak indirect effect on the CBioR of the mineral soil through its direct effect on Mpy (pc = 0.11, p=0.07).
By allowing all the models (MIN1 and MIN2) to influence estimates, the model-averaging procedure indicated that the most important variables tested in this study and exerting direct control over the CBioR of the mineral soil were as follows, by decreasing importance: exchangeable Al, metal oxide contents, pH and TSF (Table S2). Moreover, we failed to detect any direct effect of climate or mineral soil texture on CBioR.
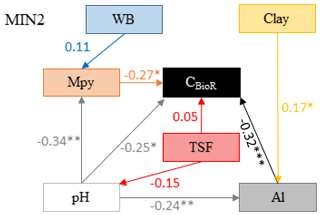
Figure 5Model that best fitted the data to explain the carbon bioreactivity (CBioR) in the top 35 cm of the mineral soil. Arrows indicate direct causal relationships. The numbers are standardized averaged path coefficients obtained using model averaging (see Table S2 and text for further details). Al: exchangeable aluminum in the top 35 cm of the mineral soil; pH: pH of the top 35 cm of the mineral soil; TSF: time since fire; Mpy: metal oxide content in the top 15 cm of the B horizon; WB: water balance; clay: clay content of the top 35 cm of the mineral soil. ; ; .
4.1 Post-fire soil C quality
Most of the studies on post-fire soil C have focused on immediate or short-term responses and found that fire affects soil C quality by creating profound changes in the structure of soil organic matter compounds through thermal oxidation (Certini, 2005; Gonzalez-Perez et al., 2004). By using a long-term chronosequence of TSF ranging from 2 to 314 years, our study provides new insights into the understanding of the trajectory of changes in soil C quality following a fire, over hundreds of years. Our estimates of the size of fast- and slow-cycling soil C pools and our results indicate that (i) both pools accumulate with TSF and (ii) the proportion of each C pool remains constant with TSF relative to total soil C stock (Fig. 2 and Table 1). These results do not necessarily imply that fire has no effect on soil C functional pools, because our chronosequence has a low resolution for the first few years following a fire, but they rather suggest that such changes, if present, are not long-lasting. Our results also highlight that the accumulation process of the bioreactive soil C reservoir does not reach an equilibrium, at least not in the first 3 centuries following a fire. Instead, environmental conditions limiting decomposition, such as cold temperatures under a thickening O layer which developed with TSF, could have slowed down labile C degradation and allowed its accumulation (Kane et al., 2005). Our results also emphasize that changes in the size of the soil functional reservoirs with TSF are stratified within the soil profile. This pattern is consistent with the fact that the impact of a fire on soil C stock is limited to surface soil horizons (Andrieux et al., 2018).
4.2 Control mechanisms of the soil carbon bioreactivity
This study shows that soil CBioR is driven by several climatic and non-climatic variables, some being common both for the O layer and mineral soil and others not, suggesting that different mechanisms may be involved in the control of the decomposition process in the O layer and in the mineral soil (Shaw et al., 2015; Ziegler et al., 2017).
4.2.1 Soil carbon bioreactivity in the O layer
Our results suggested that pH and exchangeable Mn are important drivers of CBioR in the O layer. Boreal evergreen coniferous species generate high-lignin litter and forest floor layers (Laganière et al., 2017). This is reflected in the high proportion of acid-insoluble C of O-layer samples (among all the O-layer samples, mean ± SD = 73±5 %; Fig. S3). Therefore, soil C cycling in boreal forests depends on the capacity of microbes to depolymerize lignin. Microorganisms in the acidic soils of this ecosystem are dominated by fungi that use metalloenzymes – such as Mn peroxidases – to metabolize lignin (Pollegioni et al., 2015) or are white-rot fungi (Basidiomycota) equipped with enzymes that oxidize lignin (Cragg et al., 2015). Soil C stocks in the boreal-forest humus layer have been found to be negatively correlated with exchangeable Mn availability (Stendahl et al., 2017). In our study, exchangeable Mn of the O layer was positively correlated with CBioR, suggesting that increasing Mn availability stimulates organic matter breakdown and that an Mn bottleneck in soil C cycling may be present (Kranabetter, 2019). We also observed direct and positive causal relationships between pH and CBioR of the O layer, indicating that acidic soil conditions limit soil C mineralization (Prescott et al., 2000). Bacterial respiration and microbial community composition were found to be strongly determined by soil pH in the forest soil (Bååth and Anderson, 2003). We found that pH of the O layer decreased with TSF. Alongside the direct and positive effect of TSF on the CBioR of the O layer, our results indicate that dynamic processes constrained by chemical soil properties shifting with stand development after burning (e.g., pH) drive the nature of soil organic matter and potentially the rate of C losses by heterotrophic respiration from boreal-forest soils.
Altogether, these results emphasize the need to include both soil chemistry and biological mechanisms in models of soil C cycling to better anticipate the role played by the boreal forest in C cycle climate feedbacks. Soil C cycling in mechanistic models of forest C dynamics often assumes that climate drives decay and the transfer rate of and between soil C pools (see Deluca and Boisvenue, 2012). Based on our results, we argue that chemical drivers of soil organic matter decomposition, such as exchangeable Mn concentrations and pH, might also be used to modulate soil C dynamics in such models, and we especially advise accounting for temporal shifts in soil pH occurring with stand development.
We did not detect any direct effect of climate on soil CBioR in the O layer. This finding is consistent with the results of unchanged soil C stocks with in situ experimental warming worldwide (van Gestel et al., 2018). Furthermore, when synthesizing data of in situ experimental warming, Carey et al. (2016) found no change in the soil respiration rate for warmed compared to control plots at the global scale, whereas changes were found to be significant for the boreal biome. The cumulative C mineralization of incubated soils in our study was not modulated by in situ temperature, which supports the results of Carey et al. (2016) for their entire dataset but not for the boreal biome-restricted dataset. However, Carey et al. (2016) did not study soils from the Canadian Boreal Shield.
4.2.2 Soil carbon bioreactivity in the mineral soil
As in the O layer, our results highlight the role of pH as a regulator of CBioR in the mineral soil. In addition to having a direct effect on CBioR, pH also had two indirect effects. The first indirect effect is through the stimulation of metal oxide production with increasing acidic conditions. We observed that low-pH conditions correlated positively with higher metal oxide contents, which in turn correlated negatively with CBioR in the mineral soil. This result is consistent with previous findings showing the role played by pH in mineral weathering and the preservation of C from decomposition through organometal complexation (Andrieux et al., 2018). The second indirect effect of pH on CBioR in the mineral soil is mediated through exchangeable Al only, not through Mn (Table S2). Microbes are vertically stratified within the soil column (Clemmensen et al., 2013; Ekschmitt et al., 2008; Hynes and Germida, 2013), with fungi populating the upper soil layers because of their higher metabolic-oxygen demand compared with bacteria, which can more easily dwell in the less-oxygenated deeper soil layers. Our results suggest that, contrary to the O layer, oxidative depolymerization of lignin compounds mediated by Mn peroxidases may not be a major process for C cycling in the mineral soil (see above). Instead, the negative effect of pH on exchangeable Al, together with the negative effect of exchangeable Al on CBioR in the mineral soil, indicates that low-pH conditions favor a greater abundance of exchangeable Al, which in turn impedes organic matter decomposition. These findings are consistent with the observed pH-dependent Al toxicity that slowed microbial catabolic activities in acidic forest soils in Japan (Kunito et al., 2016) and in laboratory experiments (Wood, 1995). Our study goes one step further in that we show that the content of exchangeable Al is directly related to soil texture (especially clay content) in these podzolic soils. This supports the hypothesis that exchangeable Al bound to fine mineral particles, such as clay, might act as a source of stored Al that can be mobilized and complexed with C to impede decomposition.
Contrary to the O layer, we found that TSF was only weakly correlated with mineral soil CBioR and pH, and these relationships were not significant. We also found that the indirect effect of climate (correlation between water balance and metal oxides) on CBioR was marginal. These results indicate that effects of TSF (direct and indirect) and water availability (indirect) on CBioR are restricted to surface organic horizons. Our results support the idea that properties of the organic layer are more likely to be affected by fire because they are directly exposed to surface heating (De Bano, 1990) and that the thick humus layer of boreal-forest soils (Table 1) protects deeper soil layers from shifts in environmental conditions. The fact that roots of black spruce mostly develop in the top soil (Yuan and Chen, 2010) could explain why we did not observe shifts in pH of the mineral soil with TSF.
4.3 Implication for carbon cycling and research needs
Theoretically, soil C dynamics can be predicted through a knowledge of soil C pool sizes, changes in inputs and sensitivity to environmental factors (Luo et al., 2016). Understanding the bioreactivity of the large boreal-forest soils C reservoir is key to predicting the future global C cycle in the face of global warming. As illustrated in our study, some factors may act as C stabilization agents of soil C (i.e., metal oxides binding organic matter), while others may contribute to accelerating or slowing down the rate of soil organic matter biological processing (i.e., exchangeable Mn as a co-metabolic compound of lignin degradation; when in excess, toxicity of exchangeable Al for microbes; soil acidity regulating the microbial activity), and finally, others are related to the quality of organic matter inputs to the soil (i.e., type of moss flora; low-quality organic materials left after a fire) or simply to the time required by the system to adjust and reach the steady state (i.e., causal relationships between TSF and pH or pH and metal oxides). Our best models showed that the climatic conditions experienced in situ, expressed here as temperature and water availability, had no direct effect on in vitro soil CBioR. Moreover, the indirect effects of climate on soil CBioR are limited to water supply factors, not to temperature. Best models also reveal direct and indirect effects on CBioR of both site properties and factors that evolve with TSF. Understanding and predicting changes in the soil chemistry is therefore a key challenge that remains to be addressed in future works in order to improve our understanding of soil C balance with global change. Our results are in agreement with Davidson and Janssens (2006) and Davidson (2015), suggesting that improvements to Earth system models (ESMs) may arise from integrating the long-term effects of climate on soil properties with the environmental constraints on microbiological degradation of soil organic matter.
The results of this study identified new pathways for the control mechanisms of soil CBioR that could help to predict the response of boreal-forest soils to global change. While Earth system models commonly focus on a temperature dependence of soil C decomposition (Bradford et al., 2016), our study showed, in agreement with Rasmussen et al. (2018), that key soil properties, because of their relationship to soil C bioreactivity, could improve ESMs for modeling soil C dynamics in relation to climate change. In particular, our study shows that predictive models need to include the direct effects of soil chemistry and the indirect effects of climate and soil texture on soil CBioR. Moreover, while some factors (metal oxides and TSF) were found to affect both soil CBioR (this study) and soil C stocks (Andrieux et al., 2018), at the same time, other factors did not have such effects (types of mosses and pH). For example, moss dominance had a direct effect on C stock (Andrieux et al., 2018) but not on CBioR (this study) in the O layer.
The path analyses and model selection procedure used in our study have made it possible to distinguish direct from indirect effects of ecological drivers on soil C dynamics. We found that the local climate shaped soil CBioR indirectly through effects on moss dominance and on metal oxides and that of the four climatic variables examined, only the variables related to water supply – and not temperature – significantly but indirectly affected soil CBioR. This suggests that the forecasted increase of 11 % precipitation by the end of this century in eastern North America (IPCC, 2013) would indirectly modulate soil C stocks (Andrieux et al., 2018) and soil CBioR (this study), together with the indirect effects of climate on the mechanisms of soil C stock and bioreactivity. How the boreal-ecosystem C balance will evolve in the context of global change might be assessed through further research focusing on the changes in soil physicochemical reactions pertaining to the mechanisms of soil organic matter decomposition and stabilization (Thornley and Canell, 2001).
Our study aimed to quantify the long-term post-fire changes occurring in the functional soil C pools and to disentangle the direct and indirect relative contribution of climate, moss dominance, soil particle size distribution and soil chemistry (pH, exchangeable Mn and Al, and metal oxides) on soil CBioR. Using a chronosequence approach, we show that labile and recalcitrant soil C pools both increased continually from 2 to 314 years after a fire. Changes in the size of the bioreactive and of the acid-insoluble C pools with TSF were found to be stratified within the soil profile and reveal that the impact of a fire on soil functional C reservoirs is limited to surface soil horizons. The main drivers of CBioR varied with the soil layer considered. The breakdown of organic matter in the O layer was constrained by pH and exchangeable Mn, while that of the mineral soil was dependent on the availability of exchangeable Al, metal oxide content and pH. Moreover, our results suggest that for both soil layers, the complex interplay among biogeochemical covariates needs to be accounted for to assess the variation structure of CBioR, with climate (water supply parameters only and not temperature) having only indirect effects. We argue that the direct and indirect effects that covariates have on the bioreactivity of soil organic carbon need to be integrated in models simulating C dynamics. This is key to forecasting the response of the enormous boreal-forest soil carbon pool to global warming.
All data presented in this paper can be accessed online free of charge on Canada's Open Government website (https://doi.org/10.23687/611911cf-e58a-4efa-9acf-c19bd0767e10, Andrieux et al., 2020).
The supplement related to this article is available online at: https://doi.org/10.5194/soil-6-195-2020-supplement.
BA, DP, YB and PG participated in writing the funding application and in designing the study. BA and PG did the fieldwork. BA and DP contributed to the lab work. BA and JB analyzed the data. BA prepared the paper with contributions from all coauthors.
The authors declare that they have no conflict of interest.
We would like to thank Catherine Bruyère, Cécile Remy, Arnaud Guillemard and Eric Beaulieu for field assistance. We are grateful to Danielle Charron and Pierre Clouâtre for helping with field logistics. We warmly thank Véronique Poirier, Jean Noël and Emeline Chaste for help with geomatics work and Serge Rousseau for laboratory analyses as well as Cindy Shaw for insightful comments.
This research has been supported by Mitacs Acceleration (grant nos. IT05018 and FR11062 to FR11067).
This paper was edited by Stefan Doerr and reviewed by two anonymous referees.
Amundson, R. and Jenny, H.: On a state factor model of ecosystems, BioScience, 47, 536–543, https://doi.org/10.2307/1313122, 1997.
Andrieux, B., Beguin, J., Bergeron, Y., Grondin, P., and Paré, D.: Drivers of post-fire organic carbon accumulation in the boreal forest, Global Change Biol., 24, 4797–4815, https://doi.org/10.1111/gcb.14365, 2018.
Andrieux, B., Paré, D., Beguin, J., Grondin, P., and Bergeron, Y.: Soil organic carbon bioreactivity in the spruce feathermoss forests of Quebec (Canada), https://doi.org/10.23687/611911cf-e58a-4efa-9acf-c19bd0767e10, last access: 12 May 2020.
Andrus, R. E.: Some aspects of Sphagnum ecology, Can. J. Botany, 64, 416–426, https://doi.org/10.1139/b86-057, 1986.
Bååth, E. and Anderson, T. H.: Comparison of soil fungal/bacterial ratios in a pH gradient using physiological and PLFA-based techniques, Soil Biol. Biochem., 35, 955–963, https://doi.org/10.1016/s0038-0717(03)00154-8, 2003.
Belisle, A. C., Gauthier, S., Cyr, D., Bergeron, Y., and Morin, H.: Fire regime and old-growth boreal forests in central Quebec, Canada: An ecosystem management perspective, Silva Fenn, 45, 889–908, 2011.
Bisbee, K., Gower, S., Norman, J., and Nordheim, E.: Environmental controls on ground cover species composition and productivity in a boreal black spruce forest, Oecologia, 129, 261–270, https://doi.org/10.1007/s004420100719, 2001.
Bond-Lamberty, B., Peckham, S. D., Ahl, D. E., and Gower, S. T.: Fire as the dominant driver of central Canadian boreal forest carbon balance, Nature, 450, 89–92, https://doi.org/10.1038/nature06272, 2007.
Bouchard, M., Pothier, D., and Gauthier, S.: Fire return intervals and tree species succession in the North Shore region of eastern Quebec, Can. J. Forest Res., 38, 1621–1633, https://doi.org/10.1139/x07-201, 2008.
Bradford, M. A., Wieder, W. R., Bonan, G. B., Fierer, N., Raymond, P. A., and Crowther, T. W.: Managing uncertainty in soil carbon feedbacks to climate change, Nat. Clim. Change, 6, 751–758, https://doi.org/10.1038/nclimate3071, 2016.
Buurman, P. and Jongmans, A. G.: Podzolisation and soil organic matter dynamics, Geoderma, 125, 71–83, https://doi.org/10.1016/j.geoderma.2004.07.006, 2005.
Carey, J. C., Tang, J., Templer, P. H., Kroeger, K. D., Crowther, T. W., Burton, A. J., Dukes, J. S., Emmett, B., Frey, S. D., Heskel, M. A., Jiang, L., Machmuller, M. B., Mohan, J., Panetta, A. M., Reich, P. B., Reinsch, S., Wang, X., Allison, S. D., Bamminger, C., Bridgham, S., Collins, S. L., de Dato, G., Eddy, W. C., Enquist, B. J., Estiarte, M., Harte, J., Henderson, A., Johnson, B. R., Larsen, K. S., Luo, Y., Marhan, S., Melillo, J. M., Penuelas, J., Pfeifer-Meister, L., Poll, C., Rastetter, E., Reinmann, A. B., Reynolds, L. L., Schmidt, I. K., Shaver, G. R., Strong, A. L., Suseela, V., and Tietema, A.: Temperature response of soil respiration largely unaltered with experimental warming, P. Natl. Acad. Sci. USA, 113, 13797–13802, https://doi.org/10.1073/pnas.1605365113, 2016.
Castellano, M. J., Mueller, K. E., Olk, D. C., Sawyer, J. E., and Six, J.: Integrating plant litter quality, soil organic matter stabilization, and the carbon saturation concept, Global Change Biol., 21, 3200–3209, https://doi.org/10.1111/gcb.12982, 2015.
Certini, G.: Effects of fire on properties of forest soils: a review, Oecologia, 143, 1–10, https://doi.org/10.1007/s00442-004-1788-8, 2005.
Chaste, E., Girardin, M. P., Kaplan, J. O., Portier, J., Bergeron, Y., and Hély, C.: The pyrogeography of eastern boreal Canada from 1901 to 2012 simulated with the LPJ-LMfire model, Biogeosciences, 15, 1273–1292, https://doi.org/10.5194/bg-15-1273-2018, 2018.
Clemmensen, K. E., Bahr, A., Ovaskainen, O., Dahlberg, A., Ekblad, A., Wallander, H., Stenlid, J., Finlay, R. D., Wardle, D. A., and Lindahl, B. D.: Roots and associated fungi drive long-term carbon sequestration in boreal forest, Science, 339, 1615–1618, 10.1126/science.1231923, 2013.
Cotrufo, M. F., Wallenstein, M. D., Boot, C. M., Denef, K., and Paul, E.: The Microbial Efficiency-Matrix Stabilization (MEMS) framework integrates plant litter decomposition with soil organic matter stabilization: do labile plant inputs form stable soil organic matter?, Global Change Biol., 19, 988–995, https://doi.org/10.1111/gcb.12113, 2013.
Courchesne, F. and Turmel, M.-C.: Extractable Al, Fe, Mn, and Si, in: Soil Sampling and Methods of Analysis, Second Edition, edited by: Carter M. R., and Gregorich, E. G., CRC Press, Boca Raton, FL, 1262 pp., 2007.
Cragg, S. M., Beckham, G. T., Bruce, N. C., Bugg, T. D., Distel, D. L., Dupree, P., Etxabe, A. G., Goodell, B. S., Jellison, J., McGeehan, J. E., McQueen-Mason, S. J., Schnorr, K., Walton, P. H., Watts, J. E., and Zimmer, M.: Lignocellulose degradation mechanisms across the Tree of Life, Curr. Opin. Chem. Biol., 29, 108–119, https://doi.org/10.1016/j.cbpa.2015.10.018, 2015.
Craine, J. M., Fierer, N., and McLauchlan, K. K.: Widespread coupling between the rate and temperature sensitivity of organic matter decay, Nat. Geosci., 3, 854–857, https://doi.org/10.1038/ngeo1009, 2010.
Cyr, D., Gauthier, S., and Bergeron, Y.: The influence of landscape-level heterogeneity in fire frequency on canopy composition in the boreal forest of eastern Canada, J. Veg. Sci., 23, 140–150, https://doi.org/10.1111/j.1654-1103.2011.01338.x, 2012.
Davidson, E. A.: Soil carbon in a beer can, Nat. Geosci., 8, 748–749, https://doi.org/10.1038/ngeo2522, 2015.
Davidson, E. A. and Janssens, I. A.: Temperature sensitivity of soil carbon decomposition and feedbacks to climate change, Nature, 440, 165–173, https://doi.org/10.1038/nature04514, 2006.
De Bano, L. F.: The effect of fire on soil properties, Management and Productivity of Western-Montane Forest Soils, Boise, 151–155, 1990.
Deluca, T. H. and Boisvenue, C.: Boreal forest soil carbon: distribution, function and modelling, Forestry, 85, 161–184, https://doi.org/10.1093/forestry/cps003, 2012.
Doetterl, S., Stevens, A., Six, J., Merckx, R., Van Oost, K., Casanova Pinto, M., Casanova-Katny, A., Muñoz, C., Boudin, M., Zagal Venegas, E., and Boeckx, P.: Soil carbon storage controlled by interactions between geochemistry and climate, Nat. Geosci., 8, 780–783, https://doi.org/10.1038/ngeo2516, 2015.
Driscoll, C. T. and Likens, G. E.: Hydrogen ion budget of an aggrading forested ecosystem, Tellus, 34, 283–292, https://doi.org/10.1111/j.2153-3490.1982.tb01817.x, 1982.
Ekschmitt, K., Kandeler, E., Poll, C., Brune, A., Buscot, F., Friedrich, M., Gleixner, G., Hartmann, A., Kästner, M., Marhan, S., Miltner, A., Scheu, S., and Wolters, V.: Soil-carbon preservation through habitat constraints and biological limitations on decomposer activity, J. Plant. Nutr. Soil. Sc., 171, 27–35, https://doi.org/10.1002/jpln.200700051, 2008.
Fenton, N. J., Bergeron, Y., and Paré, D.: Decomposition rates of bryophytes in managed boreal forests: influence of bryophyte species and forest harvesting, Plant Soil, 336, 499–508, https://doi.org/10.1007/s11104-010-0506-z, 2010.
Fierer, N., Allen, A. S., Schimel, J. P., and Holden, P. A.: Controls on microbial CO2 production: a comparison of surface and subsurface soil horizons, Global Change Biol., 9, 1322–1332, https://doi.org/10.1046/j.1365-2486.2003.00663.x, 2003.
Fierer, N., Craine, J. M., McLauchlan, K., and Schimel, J. P.: Litter Quality and the Temperature Sensitivity of Decomposition, Ecology, 86, 320–326, https://doi.org/10.1890/04-1254, 2005.
Fierer, N. and Jackson, R. B.: The diversity and biogeography of soil bacterial communities, P. Natl. Acad. Sci. USA, 103, 626–631, https://doi.org/10.1073/pnas.0507535103, 2006.
Frégeau, M., Payette, S., and Grondin, P.: Fire history of the central boreal forest in eastern North America reveals stability since the mid-Holocene, The Holocene, 25, 1912–1922, https://doi.org/10.1177/0959683615591361, 2015.
Gonzalez-Perez, J. A., Gonzalez-Vila, F. J., Almendros, G., and Knicker, H.: The effect of fire on soil organic matter – a review, Environ. Int., 30, 855–870, https://doi.org/10.1016/j.envint.2004.02.003, 2004.
Hassink, J.: Preservation of Plant Residues in Soils Differing in Unsaturated Protective Capacity, Soil Sci. Soc. Am. J., 60, 487–491, https://doi.org/10.2136/sssaj1996.03615995006000020021x, 1996.
Hendershot, W. H. and Lalande, H.: Soil Reaction and Exchangeable Acidity, in: Soil Sampling and Methods of Analysis, Second Edition, edited by: Carter M. R. and Gregorich, E. G., CRC Press, Boca Raton, FL, 1262 pp., 2007.
Hynes, H. M. and Germida, J. J.: Impact of clear cutting on soil microbial communities and bioavailable nutrients in the LFH and Ae horizons of Boreal Plain forest soils, Forest Ecol. Manage., 306, 88–95, https://doi.org/10.1016/j.foreco.2013.06.006, 2013.
IPCC: Climate change 2013: the physical science basis. Contribution of working group I to the fifth assessment report of the intergovernmental panel on climate change, Cambridge University Press, Cambridge, UK and New York, NY, 1535 pp., 2013.
IUSS Working Group WRB: World reference base for soil ressources 2014, update 2015, International soil classification system for naming soils and creating legends for soil maps, World Soil Ressources Reports, FAO, Rome, 203 pp., 2015.
Jenny, H.: Factors of soil formation: a system of quantitative pedology, Dover Publications Inc., New York, NY, 191 pp., 1994.
Jobbagy, E. G. and Jackson, R. B.: The vertical distribution of soil organic carbon and its relation to climate and vegetation, Ecol. Appl., 10, 423–436, https://doi.org/10.2307/2641104, 2000.
Johnson, D. W. and Curtis, P. S.: Effects of forest management on soil C and N storage: meta analysis, Forest Ecol. Manage., 140, 227–238, https://doi.org/10.1016/S0378-1127(00)00282-6, 2001.
Kaiser, K., Eusterhues, K., Rumpel, C., Guggenberger, G., and Kögel-Knabner, I.: Stabilization of organic matter by soil minerals – investigations of density and particle-size fractions from two acid forest soils, J. Plant. Nutr. Soil. Sc., 165, 451, https://doi.org/10.1002/1522-2624(200208)165:4<451::aid-jpln451>3.0.co;2-b, 2002.
Kane, E. S., Valentine, D. W., Schuur, E. A., and Dutta, K.: Soil carbon stabilization along climate and stand productivity gradients in black spruce forests of interior Alaska, Can. J. Forest Res., 35, 2118–2129, https://doi.org/10.1139/x05-093, 2005.
Kenkel, N. C., Walker, D. J., Watson, P. R., Caners, R. T., and Lastra, R. A.: Vegetation dynamics in boreal forest ecosystems, Coenoses, 12, 97–108, 1997.
Knicker, H.: How does fire affect the nature and stability of soil organic nitrogen and carbon? A review, Biogeochemistry, 85, 91–118, https://doi.org/10.1007/s10533-007-9104-4, 2007.
Kranabetter, J. M.: Increasing soil carbon content with declining soil manganese in temperate rainforests: is there a link to fungal Mn?, Soil Biol. Biochem., 128, 179–181, https://doi.org/10.1016/j.soilbio.2018.11.001, 2019.
Kroetsch, D. and Wang, C.: Particle Size Distribution, in: Soil Sampling and Methods of Analysis, Second Edition, edited by: Carter M. R. and Gregorich, E. G., CRC Press, Boca Raton, FL, 1262 pp., 2007.
Kunito, T., Isomura, I., Sumi, H., Park, H.-D., Toda, H., Otsuka, S., Nagaoka, K., Saeki, K., and Senoo, K.: Aluminum and acidity suppress microbial activity and biomass in acidic forest soils, Soil Biol. Biochem., 97, 23–30, https://doi.org/10.1016/j.soilbio.2016.02.019, 2016.
Kurz, W. A., Shaw, C. H., Boisvenue, C., Stinson, G., Metsaranta, J., Leckie, D., Dyk, A., Smyth, C., and Neilson, E. T.: Carbon in Canada's boreal forest – A synthesis, Environ. Rev., 21, 260–292, https://doi.org/10.1139/er-2013-0041, 2013.
Laganière, J., Podrebarac, F., Billings, S. A., Edwards, K. A., and Ziegler, S. E.: A warmer climate reduces the bioreactivity of isolated boreal forest soil horizons without increasing the temperature sensitivity of respiratory CO2 loss, Soil Biol. Biochem., 84, 177–188, https://doi.org/10.1016/j.soilbio.2015.02.025, 2015.
Laganière, J., Boèa, A., Van Miegroet, H., and Paré, D.: A tree species effect on soil that is consistent across the species' range: The case of aspen and soil carbon in North America, Forests, 8, 113, https://doi.org/10.3390/f8040113, 2017.
Lang, S. I., Cornelissen, J. H. C., Klahn, T., van Logtestijn, R. S. P., Broekman, R., Schweikert, W., and Aerts, R.: An experimental comparison of chemical traits and litter decomposition rates in a diverse range of subarctic bryophyte, lichen and vascular plant species, J. Ecol., 97, 886–900, https://doi.org/10.1111/j.1365-2745.2009.01538.x, 2009.
Le Goff, H., Flannigan, M. D., Bergeron, Y., and Girardin, M. P.: Historical fire regime shifts related to climate teleconnections in the Waswanipi area, central Quebec, Canada, Int. J. Wildland Fire, 16, 607–618, https://doi.org/10.1071/Wf06151, 2007.
Le Goff, H., Girardin, M. P., Flannigan, M., and Bergeron, Y.: Dendroclimatic inference of wildfire activity in Quebec over the 20th century and implication for natural disturbance-based forest management at the northern limit of the commercial forest, Int. J. Wildland Fire, 17, 348–362, 2008.
Luo, Y., Ahlström, A., Allison, S. D., Batjes, N. H., Brovkin, V., Carvalhais, N., Chappell, A., Ciais, P., Davidson, E. A., Finzi, A., Georgiou, K., Guenet, B., Hararuk, O., Harden, J. W., He, Y., Hopkins, F., Jiang, L., Koven, C., Jackson, R. B., Jones, C. D., Lara, M. J., Liang, J., McGuire, A. D., Parton, W., Peng, C., Randerson, J. T., Salazar, A., Sierra, C. A., Smith, M. J., Tian, H., Todd-Brown, K. E. O., Torn, M., van Groenigen, K. J., Wang, Y. P., West, T. O., Wei, Y., Wieder, W. R., Xia, J., Xu, X., Xu, X., and Zhou, T.: Toward more realistic projections of soil carbon dynamics by Earth system models, Global Biogeochem. Cy., 30, 40–56, https://doi.org/10.1002/2015gb005239, 2016.
Nalder, I. A. and Wein, R. W.: Long-term forest floor carbon dynamics after fire in upland boreal forests of western Canada, Global Biogeochem. Cy., 13, 951–968, https://doi.org/10.1029/1999gb900056, 1999.
Canadian Forest Service: Canada's National Forest Inventory ground sampling guidelines: specifications for ongoing measurement. Natural Resources Canada, Canadian Forest Service, Pacific Forestry Centre, Victoria, British Colmbia, 271 pp., available at: https://nfi.nfis.org/en/ (last access: 12 May 2020) 2008.
Paré, D., Boutin, R., Larocque, G. R., and Raulier, F.: Effect of temperature on soil organic matter decomposition in three forest biomes of eastern Canada, Can. J. Soil Sci., 86, 247–256, https://doi.org/10.4141/s05-084, 2006.
Paré, D., Banville, J. L., Garneau, M., and Bergeron, Y.: Soil Carbon Stocks and Soil Carbon Quality in the Upland Portion of a Boreal Landscape, James Bay, Quebec, Ecosystems, 14, 533–546, https://doi.org/10.1007/s10021-011-9429-7, 2011.
Paul, E. A., Morris, S. J., Conant, R. T., and Plante, A. F.: Does the Acid Hydrolysis – Incubation Method Measure Meaningful Soil Organic Carbon Pools?, Soil Sci. Soc. Am. J., 70, 1023, https://doi.org/10.2136/sssaj2005.0103, 2006.
Petersen, L. W., Moldrup, P., Jacobsen, O. H., and Rolston, D. E.: Relations between Specific Surface Area and Soil Physical and Chemical Properties, Soil Sci., 161, 9–21, https://doi.org/10.1097/00010694-199601000-00003, 1996.
Plante, A. F., Conant, R. T., Paul, E. A., Paustian, K., and Six, J.: Acid hydrolysis of easily dispersed and microaggregate-derived silt- and clay-sized fractions to isolate resistant soil organic matter, Eur. J. Soil Sci., 57, 456–467, https://doi.org/10.1111/j.1365-2389.2006.00792.x, 2006.
Pollegioni, L., Tonin, F., and Rosini, E.: Lignin-degrading enzymes, FEBS J., 282, 1190–1213, https://doi.org/10.1111/febs.13224, 2015.
Porras, R. C., Hicks Pries, C. E., McFarlane, K. J., Hanson, P. J., and Torn, M. S.: Association with pedogenic iron and aluminum: effects on soil organic carbon storage and stability in four temperate forest soils, Biogeochemistry, 133, 333–345, https://doi.org/10.1007/s10533-017-0337-6, 2017.
Portier, J., Gauthier, S., Leduc, A., Arseneault, D., and Bergeron, Y.: Fire regime along latitudinal gradients of continuous to discontinuous coniferous boreal forests in eastern Canada, Forests, 7, 211, 10.3390/f7100211, 2016.
Prescott, C. E., Maynard, D. G., and Laiho, R.: Humus in northern forests: friend or foe?, Forest Ecol. Manage., 133, 23–36, https://doi.org/10.1016/s0378-1127(99)00295-9, 2000.
Preston, C. M., Bhatti, J. S., Flanagan, L. B., and Norris, C.: Stocks, chemistry, and sensitivity to climate change of dead organic matter along the Canadian boreal forest transect case study, Clim. Change, 74, 223–251, https://doi.org/10.1007/s10584-006-0466-8, 2006.
Rasmussen, C., Heckman, K., Wieder, W. R., Keiluweit, M., Lawrence, C. R., Berhe, A. A., Blankinship, J. C., Crow, S. E., Druhan, J. L., Hicks Pries, C. E., Marin-Spiotta, E., Plante, A. F., Schädel, C., Schimel, J. P., Sierra, C. A., Thompson, A., and Wagai, R.: Beyond clay: towards an improved set of variables for predicting soil organic matter content, Biogeochemistry, 137, 297–306, https://doi.org/10.1007/s10533-018-0424-3, 2018.
Régnière, J.: Generalized Approach to Landscape-Wide Seasonal Forecasting with Temperature-Driven Simulation Models, Environ. Entomol., 25, 869–881, https://doi.org/10.1093/ee/25.5.869, 1996.
Régnière, J., Saint-Amant, R., and Béchard, A.: BioSIM 10 user's manual, Natural Resources Canada, Québec, QC, 76 pp., 2013.
Salomé, C., Nunan, N., Pouteau, V., Lerch, T. Z., and Chenu, C.: Carbon dynamics in topsoil and in subsoil may be controlled by different regulatory mechanisms, Global Change Biol., 16, 416–426, https://doi.org/10.1111/j.1365-2486.2009.01884.x, 2010.
Sanborn, P., Lamontagne, L., and Hendershot, W.: Podzolic soils of Canada: Genesis, distribution, and classification, Can. J. Soil Sci., 91, 843–880, https://doi.org/10.4141/Cjss10024, 2011.
Schaetzl, R. and Anderson, S.: Soils: genesis and geomorphology 2 Edn., Cambridge University Press, New York, 817 pp., 2005.
Scharlemann, J. P. W., Tanner, E. V. J., Hiederer, R., and Kapos, V.: Global soil carbon: understanding and managing the largest terrestrial carbon pool, Carbon Manage., 5, 81–91, https://doi.org/10.4155/cmt.13.77, 2014.
Schmidt, M. W., Torn, M. S., Abiven, S., Dittmar, T., Guggenberger, G., Janssens, I. A., Kleber, M., Kogel-Knabner, I., Lehmann, J., Manning, D. A., Nannipieri, P., Rasse, D. P., Weiner, S., and Trumbore, S. E.: Persistence of soil organic matter as an ecosystem property, Nature, 478, 49–56, https://doi.org/10.1038/nature10386, 2011.
Shaw, C. H., Hilger, A. B., Metsaranta, J., Kurz, W. A., Russo, G., Eichel, F., Stinson, G., Smyth, C., and Filiatrault, M.: Evaluation of simulated estimates of forest ecosystem carbon stocks using ground plot data from Canada's National Forest Inventory, Ecol. Modell., 272, 323–347, https://doi.org/10.1016/j.ecolmodel.2013.10.005, 2014.
Shaw, C. H., Bona, K. A., Kurz, W. A., and Fyles, J. W.: The importance of
tree species and soil taxonomy to modeling forest soil carbon stocks in
Canada, Geoderma, 4, 114–125,
doi10.1016/j.geodrs.2015.01.001, 2015.
Shipley, B.: Cause and correlation in biology: a user's guide to path analysis, structural equations and causal inference, Cambridge University Press, Cambridge, UK, 331 pp., 2000a.
Shipley, B.: A new inferential test for path models based on directed acyclic graphs, Struct. Equ. Modeling, 7, 206–218, https://doi.org/10.1207/s15328007sem0702_4, 2000b.
Shipley, B.: Confirmatory path analysis in a generalized multilevel context, Ecology, 90, 363–368, https://doi.org/10.1890/08-1034.1, 2009.
Shipley, B.: The AIC model selection method applied to path analytic models compared using a d-separation test, Ecology, 94, 560–564, https://doi.org/10.1890/12-0976.1, 2013.
Silveira, M. L., Comerford, N. B., Reddy, K. R., Cooper, W. T., and El-Rifai, H.: Characterization of soil organic carbon pools by acid hydrolysis, Geoderma, 144, 405–414, https://doi.org/10.1016/j.geoderma.2008.01.002, 2008.
Six, J., Conant, R. T., Paul, E. A., and Paustian, K.: Stabilization mechanisms of soil organic matter: Implications for C-saturation of soils, Plant Soil, 241, 155–176, https://doi.org/10.1023/a:1016125726789, 2002.
Skjemstad, J. O. and Baldock, J. A.: Total and Organic Carbon, in: Soil Sampling and Methods of Analysis, Second Edition, edited by: Carter M. R., and Gregorich, E. G., CRC Press, Boca Raton, FL, 1262 pp., 2007.
Stendahl, J., Berg, B., and Lindahl, B. D.: Manganese availability is negatively associated with carbon storage in northern coniferous forest humus layers, Sci. Rep., 7, 15487, https://doi.org/10.1038/s41598-017-15801-y, 2017.
Stewart, C. E., Paustian, K., Conant, R. T., Plante, A. F., and Six, J.: Soil carbon saturation: concept, evidence and evaluation, Biogeochemistry, 86, 19–31, https://doi.org/10.1007/s10533-007-9140-0, 2007.
Symonds, M. R. E. and Moussalli, A.: A brief guide to model selection, multimodel inference and model averaging in behavioural ecology using Akaike's information criterion, Behav. Ecol. Sociobiol., 65, 13–21, https://doi.org/10.1007/s00265-010-1037-6, 2010.
Thornley, J. H. M. and Canell, M. G. R.: Soil carbon storage response to temperature: an hypothseis, Ann. Bot-London, 87, 591–598, https://doi.org/10.1006/anbo.2001.1372, 2001.
van Gestel, N., Shi, Z., van Groenigen, K. J., Osenberg, C. W., Andresen, L. C., Dukes, J. S., Hovenden, M. J., Luo, Y., Michelsen, A., Pendall, E., Reich, P. B., Schuur, E. A. G., and Hungate, B. A.: Predicting soil carbon loss with warming, Nature, 554, 4–5, https://doi.org/10.1038/nature25745, 2018.
Walker, L. R., Wardle, D. A., Bardgett, R. D., and Clarkson, B. D.: The use of chronosequences in studies of ecological succession and soil development, J. Ecol., 98, 725–736, https://doi.org/10.1111/j.1365-2745.2010.01664.x, 2010.
Wiesmeier, M., Urbanski, L., Hobley, E., Lang, B., von Lützow, M., Marin-Spiotta, E., van Wesemael, B., Rabot, E., Ließ, M., Garcia-Franco, N., Wollschläger, U., Vogel, H.-J., and Kögel-Knabner, I.: Soil organic carbon storage as a key function of soils – A review of drivers and indicators at various scales, Geoderma, 333, 149–162, https://doi.org/10.1016/j.geoderma.2018.07.026, 2019.
Wood, M.: A mechanism of aluminium toxicity to soil bacteria and possible ecological implications, Plant Soil, 171, 63–69, https://doi.org/10.1007/bf00009566, 1995.
Xu, J. M., Cheng, H. H., Koskinen, W. C., and Molina, J. A. E.: Characterization of potentially bioreactive soil organic carbon and nitrogen by acid hydrolysis, Nutr. Cycl. Agroecosys., 49, 267–271, https://doi.org/10.1023/a:1009763023828, 1997.
Yuan, Z. Y. and Chen, H. Y. H.: Fine Root Biomass, Production, Turnover Rates, and Nutrient Contents in Boreal Forest Ecosystems in Relation to Species, Climate, Fertility, and Stand Age: Literature Review and Meta-Analyses, Crc. Cr. Rev. Plant Sci., 29, 204–221, https://doi.org/10.1080/07352689.2010.483579, 2010.
Zhang, D., Hui, D., Luo, Y., and Zhou, G.: Rates of litter decomposition in terrestrial ecosystems: global patterns and controlling factors, J. Plant Ecol., 1, 85–93, https://doi.org/10.1093/jpe/rtn002, 2008.
Ziadi, N. and Sen Tran, T.: Mehlich 3-Extractable Elements, in: Soil Sampling and Methods of Analysis, Second Edition, edited by: Carter M. R., and Gregorich, E. G., CRC Press, Boca Raton, FL, 1262 pp., 2007.
Ziegler, S. E., Benner, R., Billings, S. A., Edwards, K. A., Philben, M., Zhu, X., and Laganière, J.: Climate warming can accelerate carbon fluxes without changing soil carbon stocks, Front. Earth Sci., 5, 2, https://doi.org/10.3389/feart.2017.00002, 2017.