the Creative Commons Attribution 4.0 License.
the Creative Commons Attribution 4.0 License.
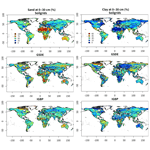
A review of the global soil property maps for Earth system models
Yongjiu Dai
Nan Wei
Qinchuan Xin
Hua Yuan
Shupeng Zhang
Shaofeng Liu
Xingjie Lu
Dagang Wang
Fapeng Yan
Soil is an important regulator of Earth system processes, but remains one of the least well-described data layers in Earth system models (ESMs). We reviewed global soil property maps from the perspective of ESMs, including soil physical and chemical and biological properties, which can also offer insights to soil data developers and users. These soil datasets provide model inputs, initial variables, and benchmark datasets. For modelling use, the dataset should be geographically continuous and scalable and have uncertainty estimates. The popular soil datasets used in ESMs are often based on limited soil profiles and coarse-resolution soil-type maps with various uncertainty sources. Updated and comprehensive soil information needs to be incorporated into ESMs. New generation soil datasets derived through digital soil mapping with abundant, harmonized, and quality-controlled soil observations and environmental covariates are preferred to those derived through the linkage method (i.e. taxotransfer rule-based method) for ESMs. SoilGrids has the highest accuracy and resolution among the global soil datasets, while other recently developed datasets offer useful compensation. Because there is no universal pedotransfer function, an ensemble of them may be more suitable for providing derived soil properties to ESMs. Aggregation and upscaling of soil data are needed for model use, but can be avoided by using a subgrid method in ESMs at the expense of increases in model complexity. Producing soil property maps in a time series still remains challenging. The uncertainties in soil data need to be estimated and incorporated into ESMs.
- Article
(2168 KB) - Full-text XML
- BibTeX
- EndNote
Soil or the pedosphere is a key component of the Earth system, and plays an important role in water, energy, and carbon balances and other biogeochemical processes. An accurate description of soil properties is essential in modelling the capability of Earth system models (ESMs) to predict land surface processes at the global and regional scales (Luo et al., 2016). Soil information is required by land surface models (LSMs), which are a component of ESMs. With the aid of computer-based geographic systems, many researchers have produced geographical databases to organize and harmonize large amounts of soil information generated from soil surveys during recent decades (Batjes, 2017; Hengl et al., 2017). However, soil datasets used in ESMs are not yet well updated or well utilized (Sanchez et al., 2009; FAO/IIASA/ISRIC/ISS-CAS/JRC, 2012). The popular soil datasets used in ESMs are outdated and have limited accuracies. Some soil properties, such as gravel (or coarse fragment) and depth to bedrock, are not utilized in most ESMs. The ESMs' schemes and structures must be changed to represent soil processes in a more realistic manner when utilizing new soil information (Oleson et al., 2013; Brunke et al., 2016; Luo et al., 2016). For example, Brunke et al. (2016) incorporated the depth-to-bedrock data into a land surface model using variable soil layers instead of the previous constant depth. Better soil information with a high resolution and better representation of soil in models has improved and will improve the performance of simulating the Earth system (e.g. Livneh et al., 2015; Dy and Fung, 2016; Kearney and Maino, 2018).
ESMs require detailed information on the physical, chemical, and biological properties of the soil. Site observations (called soil profiles) from soil surveys include soil properties such as soil depth, soil texture (sand, silt, and clay fractions), organic matter, coarse fragments, bulk density, soil colour, soil nutrients (carbon, C; nitrogen, N; phosphorus, P; potassium, K; and sulfur, S), amount of roots, and so on. The range of soil data collected during a soil survey varies with scale, country, or regional specifications and projected applications of the data (i.e. type of soil surveys, routine versus specifically designed surveys). As a result, the availability of soil properties differs in different soil databases. However, soil hydraulic and thermal parameters as well as biogeochemical parameters are usually not observed in soil surveys, which need to be estimated by pedotransfer functions (PTFs) (Looy et al., 2017). This review focuses on soil data (usually single-point observations at a given moment in time) from soil surveys, while variables such as soil temperature and soil moisture are beyond the scope of this paper.
Soil properties function in three aspects in ESMs.
-
Model inputs to estimate parameters. The soil thermal (soil heat capacity and thermal conductivity) and hydraulic characteristics (empirical parameters of the soil water retention curve and hydraulic conductivity) are usually obtained by fitting equations (PTFs) to easily measured and widely available soil properties, such as sand, silt, and clay fractions, organic matter content, rock fragments, and bulk density (Clapp and Hornberger, 1978; Farouki, 1981; Vereecken et al., 2010; Dai et al., 2013). Soil albedos are significantly correlated with the Munsell soil colour value (Post et al., 2000). For some ESMs, the parameters derived by PTFs are used as direct input instead of being calculated in the models.
-
Initial variables. The nutrient (C, N, P, K, S, and so on) amounts and the nutrients' associated parameters (pH, cation-exchange capacity, etc.) in soils can be used to initialize the simulations. Generally, their initial values are assumed to be at steady state by running the model over thousands of model years (i.e. spin-up) until there is no change trend in pool sizes (McGuire et al., 1997; Thornton and Rosenbloom, 2005; Doney et al., 2006; Luo et al., 2016). To initialize nutrient amounts using soil data derived from observations as background fields could largely reduce the times of model spin-up, and could avoid the possibility of a non-linear singularity evolution of the model, which means that the models may have multiple equilibria and then provide a better estimate of the true terrestrial nutrient state. The initial nutrient stocks' settings are major factors leading to model-to-model variation in simulation (Todd-Brown et al., 2014).
-
Benchmark data. Soil data, as measurements, could serve as a reference for model calibration, validation, and comparison. Soil carbon stock is one of the soil properties that is most frequently used as benchmark data (Todd-Brown et al., 2013). Other nutrient stocks, such as nitrogen stock, can also be used as benchmark data if an ESM simulated these properties.
Soil properties have great spatial heterogeneity both horizontally and vertically. As a result, ESMs usually incorporate soil property maps (i.e. horizontal spatial distribution) for multiple layers rather than a global constant or a single layer. ESMs, especially LSMs, are evolving towards hyper-resolutions of 1 km or finer with more detailed parameterization schemes to accommodate the land surface heterogeneity (Singh et al., 2015; Ji et al., 2017). Therefore, spatially explicit soil data at high resolutions are necessary to improve land surface representations and simulations. Because soil properties are observed at individual locations, soil mapping or spatial prediction models are needed to derive a 3-D representation of the soil distribution. The traditional method (i.e. the linkage method, also called the taxotransfer rule-based method) involves linking soil profiles and soil mapping units on soil-type maps, sometimes with ancillary maps such as topography and land use (Batjes, 2003; FAO/IIASA/ISRIC/ISS-CAS/JRC, 2012). In recent decades, various digital soil mapping technologies have been proposed by finding the relationships between soil and environmental covariates (usually remote sensing data), such as climate, topography, land use, or geology (McBratney et al., 2003).
There are many challenges related to the application of soil datasets in ESMs. First, soil datasets are usually not appropriately scaled or formatted for the use of ESMs, and some upscaling issues, which are the most frequently encountered, need to be addressed. The soil datasets produced by the linkage methods are polygon-based and need to be converted to fit the grid-based ESMs. This conversion can be performed by either the subgrid method or spatial aggregation. The up-to-date soil data are provided at a resolution of 1 km or finer, while the LSMs are mostly run at a coarser resolution. Therefore, soil data upscaling is necessary before it can be used by ESMs. Proper upscaling methods need to be chosen carefully to minimize the uncertainty introduced by these methods in the modelling results (Hoffmann et al., 2016; Kuhnert et al., 2017). Second, all the current global soil datasets represent the average state of the last decades, and the production of soil property maps in a time series is still challenging. Soil landscape and pedogenic models are developed to simulate soil formation processes and soil property changes, which can be incorporated into ESMs. The prediction of changing soil properties can also be performed by digital soil mapping using the changing climate and land use as covariates. Third, the uncertainty in the soil properties can be estimated, and adaptive surrogate modelling based on statistical regression and machine learning may be used to assess the uncertainty effects of soil properties on ESMs (Gong et al., 2015; Li et al., 2018). Finally, the layer schemes of soil datasets need to be converted for model use, and missing values for deeper soil layers need to be filled.
This paper is organized into the following sections. In Sect. 2, we first introduce soil datasets produced by the linkage method and digital soil mapping technology at global and national scales, and then we introduce the soil datasets that have already been incorporated into ESMs, and we also present PTFs that are used in ESMs to estimate soil hydraulic and thermal parameters. In Sect. 3, several global soil datasets are compared and evaluated with a global soil profile database. In Sect. 4, two issues regarding the model use of soil data are described and existing challenges related to the application of soil datasets in ESMs are discussed. In Sect. 5, a summary and the outlook of further improvements are provided.
2.1 Global and national soil datasets
Two kinds of soil data are generated from soil surveys: maps (usually in the form of polygon maps) representing the main soil types in landscape units and soil profiles with soil property measurements which are considered to be representative of the main component soils of the respective mapping units. ESMs usually require the spatial distribution of soil properties (i.e. soil property maps) rather than information about soil types. Two kinds of methods, i.e. the linkage method and the digital soil mapping method, are used to derive the soil property maps.
Soil maps (the term soil map refers to soil-type map in this paper) show the geographical distribution of soil types, which are compiled under a certain soil classification system. There are many soil mapping units (SMUs) in a soil map and an SMU is composed of more than one component (i.e. soil type) in most cases. At the global level, there is only one generally accepted global soil map, i.e. the FAO-UNESCO Soil Map of the World (SMW) (FAO, 1981). The SMW was made based on soil surveys conducted between the 1930s and 1970s and technology that was available in the 1960s. Several versions exist in digital format (FAO, 1995, 2003b; Zöbler, 1986) and these products are known to be outdated. The information on the initial SMW and DSMW has since been updated for large sections of the world in the Harmonized World Soil Database (HWSD) product (FAO/IIASA/ISRIC/ISS-CAS/JRC, 2012), which has recently been revised in WISE30sec (Batjes, 2016).
At the regional and national levels, there are many soil maps based on either national or international soil classifications. Some examples of major soil maps available in digital formats are as follows: the Soil and Terrain Database (SOTER) databases (Van Engelen and Dijkshoorn, 2012) for different regions, the European Soil Database (ESB, 2004), the 1:1 million Soil Map of China (National Soil Survey Office, 1995), the US General Soil Map (GSM), the 1:1 million Soil Map of Canada (Soil Landscapes of Canada Working Group, 2010), and the Australian Soil Resource Information System (ASRIS) (Johnston et al., 2003).
Soil profiles are composed of multiple layers called soil horizons. For each horizon, soil properties are observed (e.g. site data) or measured (e.g. pH, sand, silt, and clay content). At the global level, several soil profile databases exist. Here, we discuss only the two most comprehensive databases. The World Inventory of Soil Emission Potentials (WISE) database was developed as a homogenized set of soil profiles (Batjes, 2008). The newest version (WISE 3.1) contains 10 253 soil profiles and 26 physical and chemical properties. The soil profile database of the World Soil Information Service (WoSIS) contains the most abundant profiles (about 118 400) from national and global databases, including most of the databases mentioned below (Batjes et al., 2017), although only a selection of important soil properties (12) is included (Ribeiro et al., 2018). Data from WoSIS have been standardized, with special attention to the description and comparability of soil analytical methods worldwide. However, many countries, while having a large collection of soil profile data, are not yet sharing such data (Arrouays et al., 2017).
At the regional and national levels, there are many soil profile databases, usually with soil classifications corresponding to the local soil maps, and here are some examples: the USA National Cooperative Soil Survey Soil Characterization database (http://ncsslabdatamart.sc.egov.usda.gov/, last access: 3 July 2019), profiles from the USA National Soil Information System (http://soils.usda.gov/technical/nasis/, last access: 3 July 2019), the Africa Soil Profiles database (Leenaars, 2012), the ASRIS (Karssies, 2011), the Chinese National Soil Profile database (Shangguan et al., 2013), the soil profile archive from the Canadian Soil Information System (MacDonald and Valentine, 1992), soil profiles from SOTER (Van Engelen and Dijkshoorn, 2012), the soil profile analytical database for Europe (Hannam et al., 2009), the Mexico soil profile database (Instituto Nacional de Estadística y Geografía, 2016), and the Brazilian national soil profile database (Cooper et al., 2005).
The linkage method (called the taxotransfer rule-based method) involves linking soil maps (with SMUs or soil polygons) and soil profiles (with soil properties) according to taxonomy-based pedotransfer (taxotransfer for short; note that here pedotransfer here does not mean PTFs, which are a different thing) rules (Batjes, 2003). The criteria used in the linkage could be one or many factors, such as the following: soil class, soil texture class, depth zone, topographic class, distance between soil polygons, and soil profiles (Shangguan et al., 2012). Each soil type is represented by one or a group of soil profiles that meet the criteria, and, usually, the median or mean value of a soil property is assigned to the soil type. Because the linkage method assigned only one value or a statistical distribution to a soil type in the soil polygons (usually a polygon contains multiple soil types with their fractions), the intra-polygonal spatial variation is not considered. At the global level, many databases were derived by the linkage method: the FAO SMW with derived soil properties (FAO, 2003a), the Data and Information System of International Geosphere-Biosphere Programme (IGBP-DIS) database (Global Soil Data Task, 2000), the Soil and Terrain Database (Van Engelen and Dijkshoorn, 2012) for multiple regions and countries, the ISRIC-WISE-derived soil property maps (Batjes, 2006), the HWSD (FAO/IIASA/ISRIC/ISS-CAS/JRC, 2012), the Global Soil Dataset for Earth System Model (GSDE) (Shangguan et al., 2014), and WISE30sec (Batjes, 2016). The three most recent databases are HWSD, GSDE, and WISE30sec. HWSD was built by combining the existing regional and national soil information updates. GSDE, as an improvement of HWSD, incorporated more soil maps and more soil profiles related to the soil maps, with more soil properties. GSDE accomplished the linkage based on the local soil classification, which required no correlation between classification systems and avoided the error brought by the taxonomy reference. In addition, GSDE provides an estimation of eight layers to a depth of 2.3 m, while HWSD provides an estimation of two layers to a depth of 1 m. WISE30sec is another improvement of HWSD that incorporates more soil profiles with seven layers up to 200 cm depth and with uncertainty estimated by the mean ± standard deviation. WISE30sec used the soil map from HWSD with minor corrections and climate zone maps as categorical covariates. Many national and regional agencies around the world have organized their soil surveys by linking soil maps and soil profiles, including the USA State Soil Geographic Database (STATSGO2) (Soil Survey Staff, 2017), Soil Landscapes of Canada (Soil Landscapes of Canada Working Group, 2010), the ASRIS (Johnston et al., 2003), the Soil-Geographic Database of Russia (Shoba et al., 2008), the European Soil Database (ESB, 2004), and the China dataset of soil properties (Shangguan et al., 2013).
Digital soil mapping (McBratney et al., 2003) is the creation and population of a geographically referenced soil database, generated at a given resolution by using field and laboratory observation methods coupled with environmental data through quantitative relationships (http://digitalsoilmapping.org/, last access: 3 July 2019). Usually, the soil datasets derived by digital soil mapping provide grid-based spatially continuous estimation, while the soil datasets derived by the linkage method provide estimations with abrupt changes at the boundaries of soil polygons. GlobalSoilMap is a global consortium that aims to create global digital maps for key soil properties (Sanchez et al., 2009). This global effort takes a bottom–up framework and produces the best available soil map at a resolution of 3 arcsec (about 100 m) with 90 % confidence in the predictions. Soil properties will be provided for six soil layers (i.e. 0–5, 5–15, 15–30, 30–60, 60–100, and 100–200 cm). Many countries have produced soil maps following the GlobalSoilMap specifications (Odgers et al., 2012; Viscarra Rossel et al., 2015; Ballabio et al., 2016; Mulder et al., 2016; Arrouays et al., 2018; Ramcharan et al., 2018). The SoilGrids system (https://www.soilgrids.org, last access: 3 July 2019) is another global soil mapping project (Hengl et al., 2014, 2015, 2017). The newest version (Hengl et al., 2017) at a resolution of 250 m was produced by fitting an ensemble of machine-learning methods based on about 150 000 soil profiles and 158 soil covariates, which is currently the most detailed estimation of global soil distribution. A third global soil mapping project is the Global SOC (soil organic carbon) Map of the Global Soil Partnership, which focuses on country-specific soil organic carbon estimates (Guevara et al., 2018).
Because soil property maps are products that are derived based on soil measurements of soil profiles and spatially continuous covariates (including soil maps), it is necessary to discuss the sources of uncertainty, spatial uncertainty estimation, and accuracy assessment of these derived data (the last two are different aspects of uncertainty estimation). More attention should be given to this issue in ESM applications instead of taking soil property maps as observations without error. There are various uncertainty sources in the derivation of soil property maps, including uncertainty from soil maps, soil measurements, soil-related covariates, and the linkage method itself (Shangguan et al., 2012; Batjes, 2016; Stoorvogel et al., 2017). The following uncertainties are not a complete list of uncertainties, but the major uncertainties are listed. Uncertainties in soil maps are major sources of global datasets derived by the linkage methods. For these datasets, large sections of the world are incorporated into the coarse FAO SMW map, and the purity of soil maps (referring to the following website for the definition: https://esdac.jrc.ec.europa.eu/ESDB_Archive/ESDBv2/esdb/sgdbe/metadata/purity_maps/purity.htm, last access: 3 July 2019) is likely to be around 50 % to 65 % (Landon, 1991). Another important source of uncertainty is the limited comparability of different analytical methods for a given soil property when using soil profiles from various sources. A weak correlation or even a negative correlation was found between different analytical methods, although a strong positive correlation was revealed in most cases (McLellan et al., 2013). Both datasets of the linkage method and those by digital soil mapping are subject to this uncertainty. Although there are no straightforward mechanisms to harmonize the data, efforts have been undertaken to address this issue and provide quality assessment (Batjes, 2017; Pillar 5 Working Group, 2017). Another source of uncertainty comes from the geographic and taxonomic distribution of soil profiles, especially for the under-represented areas and soils (Batjes, 2016). The fourth source of uncertainty is from the linkage method itself. The linkage method does not represent the intra-polygon spatial variation and usually does not explicitly consider soil-related covariates like digital soil mapping, although there are cases where climate and topography are considered, and Stoorvogel et al. (2017) proposed a methodology to incorporate landscape properties into the linkage method. Finally, uncertainty from the covariates is minor because spatial prediction models such as machine learning in digital soil mapping can reduce its influences (Hengl et al., 2014), although a more comprehensive list of covariates with higher resolution and accuracy will improve the predicted soil property maps. Spatial uncertainty is estimated by different methods for the linkage method and digital soil mapping methods. For the linkage method, statistics such as standard derivation and percentiles can be used for the spatial uncertainty estimation, and these statistics are calculated for the population of soil profiles linked to a soil type or a land unit (Batjes, 2016). This estimation has some limitations because soil profiles are not taken probabilistically but are based on their availability, especially for the global soil datasets. Uncertainty will be underestimated when the sample size is not large enough to represent a soil type. For digital soil mapping, spatial uncertainty could be estimated by methods such as geostatistical methods and quantile regression forest (Vaysse and Lagacherie, 2017), which make sense of the statistics. The accuracy of the soil datasets derived by digital soil mapping is estimated by independent validation or cross-validation. However, this estimation is not trivial for those data derived by the linkage method due to the global scale and the support of the data and independent data (Stoorvogel et al., 2017), and most of these maps are validated by statistics such as the mean error and coefficient of determination. Instead, some datasets, including WISE and GSDE, use indictors such as the linkage level of soil class and sample size to offer quality control information (Shangguan et al., 2014; Batjes, 2016). A simple way to compare the accuracy of using datasets with both methods may be to use a global soil profile database as a validation dataset, though quite a number of these profiles were used when deriving these datasets and questions will be raised. We evaluated several global soil property maps in Sect. 3.
2.2 Soil dataset incorporated into ESMs
Table 1 shows ESMs (specifically, their LSMs) and their input soil datasets. The ESMs in Table 1 cover the CMIP5 (Coupled Model Intercomparison Project) list except those without information about the soil dataset inputs. LSMs are key tools to predict the dynamics of land surfaces under climate change and land use. Five datasets are widely used, i.e. the datasets by Wilson and Henderson-Sellers (1985), Zöbler (1986), Webb et al. (1993), Reynolds et al. (2000), the Global Soil Data Task (2000), and Miller and White (1998). Except for GSDE, HWSD, and STATSGO (Miller and White, 1998) for the USA in Table 1, these datasets were derived from the SMW (note that large sections of GSDE and HWSD still used this map as a base map because there are no available regional or national maps) (FAO, 1981) and limited soil profile data (no more than 5800 profiles), which gained popularity because of its simplicity and ease of use. However, these datasets are outdated and should no longer be used because much better soil information, as introduced in Sect. 2.1, can be incorporated (Sanchez et al., 2009; FAO/IIASA/ISRIC/ISS-CAS/JRC, 2012).
Table 1Lists of the soil dataset used by land surface models (LSMs) of Earth system models (ESMs) or climate models (CMs).
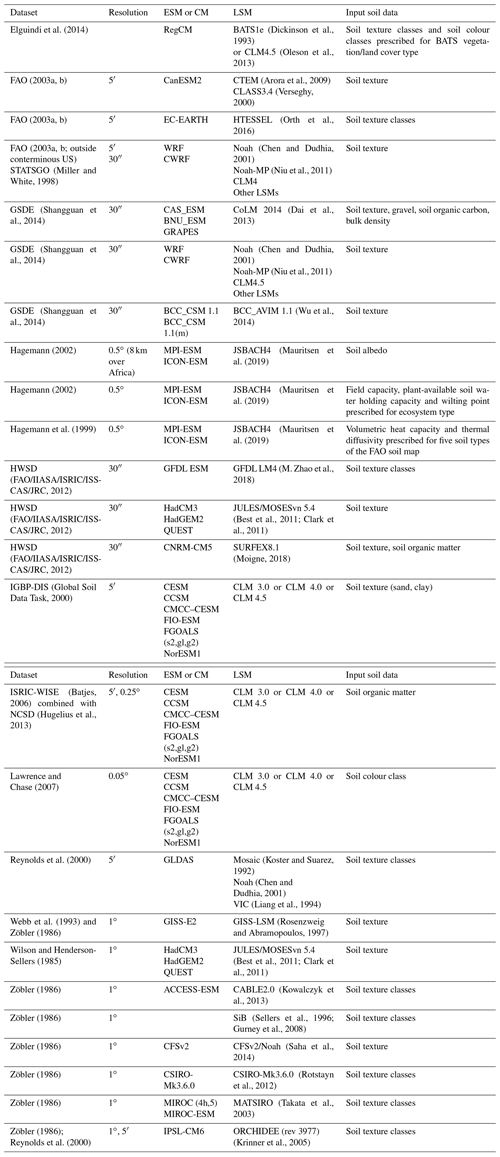
ACCESS: Australia Community Climate and Earth System Simulator; BATS: Biosphere-Atmosphere Transfer Scheme; BCC_CSM: Beijing Climate Center Climate System Model; BCC_AVIM: Beijing Climate Center Atmosphere and Vegetation Interaction Model; BNU_ESM: Beijing Normal University Earth System Model; CABLE: Community Atmosphere Biosphere Land Exchange; CanESM: Canadian Earth System Model; CAS_ESM: Chinese Academy of Sciences Earth System Model; CCSM: Community Climate System Model; CESM: Community Earth System Model; CFS: Climate Forecast System; CLASS: Canadian Land Surface Scheme; CLM: Community Land Model; CMCC–CESM: Euro-Mediterranean Centre on Climate Change Community Earth System Model; CNRM-CM: Centre National de Recherches Meteorologiques Climate Model; CoLM: Common Land Model; CSIRO-Mk: Commonwealth Scientific and Industrial Research Organization climate system model; CTEM: Canadian Terrestrial Ecosystem Model; EC-EARTH: European community Earth-System Model; FAO: Food and Agriculture Organization (FAO-UNESCO) digital Soil Map of the World (SMW) at a 1:5 million scale; FGOALS: Flexible Global Ocean-Atmosphere-Land System Model; FIO-ESM: First Institute of Oceanography Earth System Model; GRAPES: Global/Regional Assimilation Prediction System; GFDL: Geophysical Fluid Dynamics Laboratory; GISS: Goddard Institute for Space Studies; GLDAS: Global Land Data Assimilation System; GSDE: Global Soil Dataset for Earth System Model; HadCM: Hadley Centre Coupled Model; HadGEM2-ES: Hadley Global Environment Model 2 – Earth System; HTESSEL: Tiled ECMWF Scheme for Surface Exchanges over Land; HWSD: Harmonized World Soil Database; ICON-ESM: Icosahedral non-hydrostatic Earth System Model; IGBP-IDS: Data and Information System of International Geosphere-Biosphere Program; IPSL-CM: Institute Pierre Simon Laplace Climate Model; ISRIC-WISE: World Inventory of Soil Emission Potentials of International Soil Reference and Information Centre; JSBACH: Jena Scheme of Atmosphere Biosphere Coupling in Hamburg; JULES/MOSES= Joint UK Land Environment Simulator/Met Office Surface Exchange Scheme; MATSIRO: Minimal Advanced Treatments of Surface Interaction and Runoff; MIROC: Model for Interdisciplinary Research on Climate; MPI-ESM: Max Planck Institute for Meteorology Earth System Model; Noah-MP: Noah-multiparameterization; NorESM1: Norwegian Earth System Model; NCSD: Northern Circumpolar Soil Carbon Database; ORCHIDEE: Organising Carbon and Hydrology In Dynamic Ecosystems; QUEST: Quantifying and Understanding the Earth System; RegCM: Regional Climate Model; SiB: Simple Biosphere Model; STATSGO: State Soil Geographic Database; SURFEX: Surface Externalisée; WRF: Weather Research and Forecasting Model.
In recent years, efforts have been made to improve the soil data condition in ESMs. The Land-Atmosphere Interaction Research Group at Sun Yat-sen University (formerly at Beijing Normal University) has put much effort into this topic. Shangguan et al. (2012, 2013) developed a China soil property dataset for land surface modelling based on 8979 soil profiles and the Soil Map of China using the linkage method. Dai et al. (2013) derived soil hydraulic parameters using PTFs based on the soil properties by Shangguan et al. (2013). Shangguan et al. (2014) further developed a comprehensive global dataset for ESMs. The above soil datasets were widely used in the ESMs. Soil properties from these soil datasets, including soil texture fraction, organic carbon, bulk density, and derived soil hydraulic parameters, were implemented in the Common Land Model Version 2014 (CoLM2014, http://globalchange.bnu.edu.cn, last access: 3 July 2019). Li et al. (2017) showed that CoLM2014 was more stable than the previous version and had comparable performance to that of CLM4.5, which may be partially attributed to the new soil parameters being used as input. Wu et al. (2014) showed that soil moisture values are closer to the observations when simulated by CLM3.5 with the China dataset than those simulated with FAO. Zheng and Yang (2016) estimated the effects of soil texture datasets from FAO and BNU based on regional terrestrial water cycle simulations with the Noah-MP land surface model. Tian et al. (2012) used the China soil texture data in a land surface model (GWSiB) coupled with a groundwater model. Lei et al. (2014) used the China soil texture data in the CLM to estimate the impacts of climate change and vegetation dynamics on runoff in the mountainous region of the Haihe River basin. Zhou et al. (2015) estimated age-dependent forest carbon sinks with a terrestrial ecosystem model utilizing China soil carbon data. Dy and Fung (2016) updated the soil data for the Weather Research and Forecasting model (WRF).
Researchers have also put efforts into updating ESMs with other soil data. Lawrence and Chase (2007) used MODIS data to derive soil reflectance, which was used as a soil colour parameter in the Community Land Model 3.0 (CLM). De Lannoy et al. (2014) updated the NASA Catchment land surface model with soil texture and organic matter data from HWSD and STATSGO2. Livneh et al. (2015) evaluated the influence of soil textural properties on hydrologic fluxes by comparing the FAO data and STATSGO2. Folberth et al. (2016) evaluated the impact of soil input data on yield estimates in a globally gridded crop model. Slevin et al. (2017) utilized the HWSD to simulate global gross primary productivity in the JULES land surface model. Trinh et al. (2018) proposed an approach that can assimilate coarse global soil data by finer land use and coverage datasets, which improved the performance of hydrologic modelling at the watershed scale. Kearney and Maino (2018) incorporated the new generation of soil data produced by the digital soil mapping method into a climate model and found that, compared to the old soil information, the soil moisture simulation was improved at a fine spatial and temporal resolution over Australia. A dataset of globally gridded hydrologic soil groups (HYSOGs250m) was developed based on soil texture and depth to bedrock of SoilGrids (Hengl et al., 2017) and groundwater table depth (Fan et al., 2013) for curve-number-based runoff modelling of the U.S. Department of Agriculture (Ross et al., 2018).
Except for soil properties, the estimation of underground boundaries, including the groundwater table depth, the depth to bedrock (DTB), and depth to regolith, and its implementation in ESMs, is also a new focus. Fan et al. (2013) compiled global observations of water table depth and inferred the global patterns using a groundwater model. Pelletier et al. (2016) developed a global DTB dataset using process-based models for upland and an empirical model for lowland. This dataset was implemented in CLM4.5, and there were significant influences on the water and energy simulations compared to the default constant depth (Brunke et al., 2016). Shangguan et al. (2017) developed a global DTB by digital soil mapping based on about 1.7 million observations from soil profiles and water wells, which has a much higher accuracy than the dataset by Pelletier et al. (2016). Vrettas and Fung (2016) showed that weathered bedrock stores a significant fraction (more than 30 %) of the total water despite its low porosity. Jordan et al. (2018) estimated the global permeability of the unconsolidated and consolidated Earth for groundwater modelling. However, due to the lack of data, an accurate global estimation of depth to regolith is not feasible. Caution should be used when employing the so-called soil depth products in ESMs. Soil depth maps are usually estimated based on observations from soil surveys, and soil depth (or depth to the R horizon) is assumed to be equal to DTB. However, these observations are usually less than 2 m and usually do not reach the DTB (Shangguan et al., 2017). Thus, soil depth maps based on only soil profiles are significantly underestimated (1 order of magnitude lower) compared to the actual DTB and should not be taken as the lower boundary of ESMs.
2.3 Estimating secondary parameters using PTFs
Earth system modellers have employed different PTFs to estimate soil hydraulic parameters (SHPs), soil thermal parameters (STPs), and biogeochemical parameters (Dai et al., 2013; Looy et al., 2017) or used these parameters as model inputs. Nearly all ESMs incorporated SHPs and STPs estimated by PTFs, but not biogeochemical parameters. PTFs are the empirical, predictive functions that account for the relationships between certain soil properties (e.g. hydraulic conductivity) and more easily obtainable soil properties (e.g. sand, silt, clay, and organic carbon content). Direct measurement of these parameters is difficult, expensive, and in most cases impractical for obtaining sufficient samples to reflect spatial variation. Thus, most soil databases do not contain these parameters. PTFs provide an alternative means of estimating these parameters. In ESMs, SHPs and STPs are usually derived using simple PTFs, using only soil texture data as the input. As more soil properties become globally available, including gravel, soil organic matter, and bulk density, more sophisticated PTFs that use additional soil properties can be employed in ESMs.
PTFs can be expressed as either numerical equations or by machine-learning methodology, which is more flexible for simulating the highly non-linear relationship in analysed data. PTFs can also be developed based on soil processes. Most research has not indicated where the PTFs can potentially be used, and the accuracy of a PTF outside of its development dataset is essentially unknown (McBratney et al., 2011). PTFs are generally not portable from one region to another (i.e. locally or regionally validated). Therefore, PTFs should never be considered an ultimate source of parameters in soil modelling. Looy et al. (2017) reviewed PTFs extensively in Earth system science and emphasized that PTF development must go hand in hand with suitable extrapolation and upscaling techniques such that the PTFs correctly represent the spatial heterogeneity of soils in ESMs. Although the PTFs were evaluated, it is unclear which set of PTFs are the best for global applications. Due to these limitations, a better way to estimate these parameters may be to use an ensemble of PTFs, which can provide the parameter variability. Dai et al. (2013) derived a global soil hydraulic parameter database using the ensemble method. Selection of PTFs was carried out based on the following rules, including a consistent physical definition, an adequately large training sample, and positive evaluations that are comparable with other PTFs. The selected PTFs included not only those in equations, but also machine-learning PTFs. As a result, the modellers could use these parameters as inputs instead of calculating them in ESMs every time the model was run.
New generation soil information has already been utilized to derive SHPs and STPs in some studies. Montzka et al. (2017) produced a global map of SHPs at a 0.25∘ resolution based on the SoilGrids 1 km dataset. Tóth et al. (2017) calculated SHPs for Europe with EU-HYDI PTFs (Tóth et al., 2015) based on the SoilGrids 250 m dataset. Wu et al. (2018) used an integrated approach that ensembles PTFs to map the field capacity of China based on multi-source soil datasets.
The PTF performance in ESMs has been evaluated in many studies, although PTFs have not been fully exploited and integrated into ESMs (Looy et al., 2017). Some examples are as follows. Chen et al. (2012) incorporated soil organic matter to estimate soil porosity and thermal parameters for use in LSMs. H. Zhao et al. (2018) evaluated PTF performance to estimate SHPs and STPs for land surface modelling over the Tibetan Plateau. Zheng et al. (2018) developed PTFs to estimate the soil optical parameters to derive soil albedo for the Tibetan Plateau, and the PTFs that were incorporated into an eco-hydrological model improved the model simulation of a surface energy budget. Looy et al. (2017) envisaged two possible approaches to improve parameterization of ESMs by PTFs. One approach is to replace constant coefficients in current ESMs that have spatially distributed values with PTFs. The other approach is to develop spatially exploitable PTFs to parameterize specific processes using knowledge of environmental controls and variations in soil properties.
For the convenience of ESMs' application, we compared several available soil datasets and evaluated them with soil profiles from WoSIS for some of the key variables (sand, clay content, organic carbon, coarse fragment, and bulk density) used in ESMs. In addition to the most recently developed soil datasets, we also included one old dataset (i.e. IGBP) used in ESMs for the evaluation. It is not necessary to compare all the old datasets because they are based on similar, limited, and outdated source data as described in Sect. 2.2. These datasets have coarser resolutions (Table 1) than the newly developed soil datasets (Table 2).
We present basic descriptions of the new soil datasets in Tables 2 and 3. As described in Sect. 2.1, four available global soil datasets, i.e. HWSD, GSDE, WISE30sec, and SoilGrids, have been developed in the last several years (Table 2). These soil datasets are selected to be shown here because they have global coverage with key variables used by ESMs and were developed with relatively good data sources in recent years; these data are also freely available. Old versions of these datasets are not shown here. Table 3 shows the available soil properties of these soil datasets. Except for WISE30sec, none of these databases contains spatial uncertainty estimations. The explained soil property variance in SoilGrids is between 56 % and 83 %, while the other datasets do not offer quantitative accuracy assessments. GSDE has the largest number of soil properties, while SoilGrids currently contains 10 primary soil properties defined by the GlobalSoilMap consortium.
Table 3Derived soil properties considered in four global soil datasets.
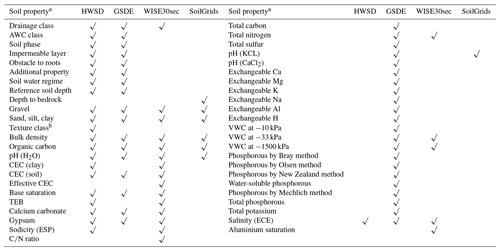
a CEC is cation exchange capacity. The base saturation measures the sum of exchangeable cations (nutrients) Na, Ca, Mg, and K as a percentage of the overall exchange capacity of the soil (including the same cations plus H and Al). TEB is the total exchangeable base including Na, Ca, Mg, and K. ESP is the exchangeable sodium percentage, which is calculated as Na*100/CEC (soil). ECE is electrical conductivity. AWC is the available water storage capacity. The first nine soil properties on the left, including the drainage class and AWC class, are available for each soil type, while the other properties are available for each layer. Notably, many different analytical methods have been used to derive a given soil property, which is a major source of uncertainty. b Texture class can be calculated using sand, silt, and clay content.
The accuracy of the newly developed soil datasets (SoilGrids, GSDE, and HWSD) and an old dataset (IGBP) are evaluated for five key variables using 94 441 soil profiles from WoSIS (Table 4), though quite a number of the WoSIS soil profiles were considered in the complication of these datasets, which means that this evaluation is not an independent validation. We used four statistics in the evaluation, including mean error (ME), root mean squared error (RMSE), coefficient of variation (CV), and coefficient of determination (R2). All soil datasets are evaluated for topsoil (0–30 cm) and subsoil (30–100 cm). The layer schemes of soil datasets are different (Table 1) and were converted to the two layers. Soil datasets are high in resolution and were converted to a resolution of 10 km by averaging. All datasets have a relatively small ME. In general, SoilGrids have much better accuracy than the other three due to RMSE, CV, and R2, and GSDE ranks second, followed by IGBP and HWSD. However, IGBP is slightly better than GSDE for bulk density and organic carbon content of topsoil. Notably, only the IGBP does not contain coarse fragments, which is needed when calculating soil carbon stocks. We did not evaluate the WISE30sec here to save time in data processing, because previous evaluation using WoSIS showed that WISE30sec had slightly better accuracy than HWSD (https://github.com/thengl/SoilGrids250m/tree/master/grids/HWSD, last access: 3 July 2019). This evaluation has some limitations. First, the datasets developed by the linkage method, which give the mean value of an SMU, resulted in an abrupt change between the boundaries of soil polygons, whereas the datasets developed by digital soil mapping simulated the soil as a continuum with a spatial continuous change in soil properties; thus, these datasets may not be comparable. Second, the original resolutions of soil datasets are different, which means that maps with higher resolutions provide more spatial details, and we should judge the map quality by not only the accuracy assessment, but also by the resolution. As a result, datasets with higher resolutions (i.e. HWSD, WISE30sec, and GSDE) are preferred to those with lower resolutions (i.e. IGBP) because the higher-resolution datasets have similar accuracy, especially when the LSMs are run at a high resolution such as 1 km. Third, the vertical variation is better represented by SoilGrids, GSDE, and WISE30sec, with more than two layers and a depth of over 2 m (Table 2), which will provide more useful information for ESMs, especially when they model deeper soils with multiple layers.
Table 4Evaluation statistics of soil datasets using soil profiles from the World Soil Information Service (WoSIS).
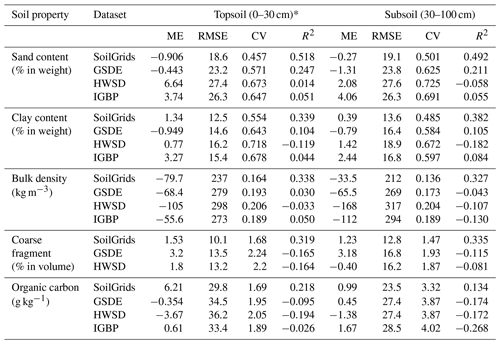
* Quite a number of WoSIS soil profiles were considered in the compilation of the four products. ME is the mean error. RMSE is the root mean squared error. CV is the coefficient of variation. R2 is the coefficient of determination.
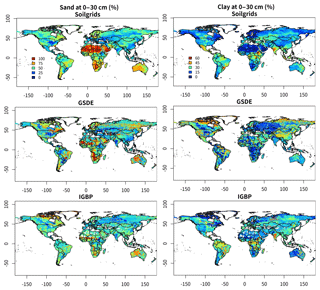
Figure 1Soil sand and clay fraction at the surface 0–30 cm layer from SoilGrids, IGBP, and GSDE. The difference among them will lead to different modelling results for ESMs. IGBP is the Data and Information System of the International Geosphere-Biosphere Program and GSDE is the Global Soil Dataset for Earth System Model.
The new generation soil dataset produced by the digital soil mapping method gave a very different distribution of soil properties from those produced by the linkage method. Figure 1 shows the soil sand and clay fractions at the surface 0–30 cm layer from SoilGrids, IGBP, and GSDE. Figure 2 shows the SOC and bulk density at the surface 0–30 cm layer from SoilGrids, IGBP, and GSDE. Significant differences are visible in these datasets. This difference will lead to different modelling results in ESMs. Tifafi et al. (2018) found that the global SOC stocks down to a depth of 1 m are 3400 Pg when estimated by SoilGrids and 2500 according to HWSD, and the estimates by SoilGrids are closer to the actual observations, although all datasets underestimated the soil carbon stocks. Figure 1 of Tifafi et al. (2018) shows the global distribution of soil carbon stocks by SoilGrids and HWSD.
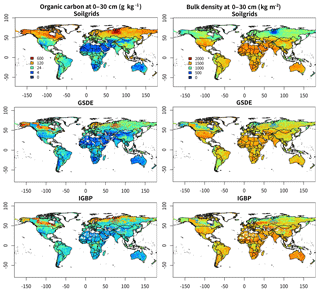
Figure 2Soil organic carbon and bulk density at the surface 0–30 cm layer from SoilGrids, GSDE, and IGBP.
In general, SoilGrids is preferred for ESMs' application because it currently has the highest accuracy and resolution. When soil properties are not available in SoilGrids, WISE30sec and GSDE offer alternative options. However, model sensitivity simulations need to be performed to investigate the effects of different soil datasets on ESMs in future studies.
4.1 Model use of soil data derived by the linkage method
Soil data by the linkage method are derived for each SMU or land unit and thus are polygon-based, while ESMs are usually grid-based. However, soil data derived by digital soil mapping are grid-based. Therefore, the compatibility between soil data derived by the linkage method and ESMs must be addressed. In the soil map, an SMU is composed of more than one component soil unit in most cases, and thus, a one-to-many relationship exists between the SMU and profile attributes of the respective soil units. This condition makes representing the attributes characterizing an SMU a nontrivial task. To keep the whole soil variation of an SMU, it is best to use the subgrid method in ESMs (Oleson et al., 2010), i.e. aggregate values of soil properties, and provide the area percentage of each value. This will bring about the problem of mapping the soil subgrids with land cover (or plant function type) subgrids. A possible solution is to classify the soil according to the soil properties and obtain a number of defined soil classes (n classes) such as land cover types (m classes), overlay the defined soil classes with land cover types, and obtain n by m combinations assuming the soil classes and land cover types are independent. However, this will increase the computing time and complexity of the ESMs' structures, which requires implementation the soil processes over each subgrid soil column within a grid instead of the entire model grid.
Usually, the compatibility issue is addressed by converting the SMU-based soil data to grid data using spatial aggregation. The ESMs uses grid data as input, and each grid cell has one unique value of a soil property. Three spatial aggregation methods were proposed to aggregate compositional attributes in an SMU to a representative value (Batjes, 2006; Shangguan et al., 2014). The area-weighting method (method A) obtains the area-weighting of soil attributes. The dominant type method (method D) obtains the soil attribute of the dominant type. The dominant binned method (method B) classifies the soil attributes into several preselected classes and obtains the dominant class. All three methods can be applied to quantitative data, while method D and method B can be applied to categorical data. The advantages and disadvantages of these methods have been discussed (Batjes, 2006; Shangguan et al., 2014). The choice should be made according to the specific applications (Hoffmann et al., 2016). Method B provides binned classes, which are not convenient for modelling, although method B is considered more appropriate to represent a grid cell. Method A maintains mass conservation, which can meet most model application demands. However, method A may be misleading in cases where extreme values appeared in an SMU. For the linkage method, the uncertainty is usually estimated by obtaining the 5 % and 95 % soil properties (or other statistics) of the soil profiles that are linked to an SMU. Because the frequency distribution of the soil properties within an SMU is usually not a normal distribution or any other typical statistical distribution, the application of statistics such as standard deviation to model use is not proper. This means that the uncertainty in the soil dataset derived by the linkage method cannot be incorporated into ESMs in a straightforward way, and technology such as bootstrap may be more suitable than methods that make assumptions on regarding the distribution.
The basic soil properties are often used to derive the secondary parameters, including SHPs and STPs by PTFs and soil carbon stock or other nutrient stocks by certain equations (Shangguan et al., 2014). This procedure could be performed either before or after the aggregation (referred to here as “aggregating after” and “aggregating first”). Because the relationship between the soil basic properties and the derived soil parameters is usually non-linear, the “aggregating first” method should be used. This was also proven by case studies (Romanowicz et al., 2005; Shangguan et al., 2014). However, some researchers have used the “aggregating after” method to produce misleading results (Hiederer and Köchy, 2012).
The aggregation smooths the variation in the soil properties between soil components within a given SMU (Odgers et al., 2012). To avoid aggregation, the spatial disaggregation of soil-type maps can be used to determine the location of the SMU components, although the location error may be high in some cases (Thompson et al., 2010; Stoorvogel et al., 2017). This method depends on the high density of soil profiles to establish soil and landscape relationships. Folberth et al. (2016) showed that the correct spatial allocation of the soil type to the present cropland was very important in global crop yield simulations. Currently, aggregation is still the practical method to use at the global scale due to lack of data.
4.2 Upscaling detailed soil data for model use
The updated soil datasets derived by both the linkage method and digital soil mapping are usually at a resolution from 1 km to 100 m, and upscaling or aggregation is required to derive lower-resolution datasets for model use. The aggregation methods mentioned above can be used. Moreover, there are many upscaling methods, such as the window median, variability-weighted methods (Wang et al., 2004), variogram method (Oz et al., 2002), fractal theory (Quattrochi et al., 2001), and the Miller–Miller scaling approach (Montzka et al., 2017). However, few studies have been devoted to determining the upscaling methods that are suitable for soil data. A preliminary effort was made by Shangguan (2014). Five upscaling methods were compared, including the window average, window median, window modal, arithmetic average variability-weighted method, and bilinear interpolation method. Differences between aggregation methods varied from 10 % to 100 % for different parameters. The upscaling methods affected the data derived by the linkage method more than the data derived by digital soil mapping. The window average, window median, and arithmetic average variability-weighted method performed similarly in upscaling. The RMSE increased rapidly when the window size was less than 40 pixels. Similarly to the aggregation of SMUs, the “aggregating first” method is recommended when secondary soil parameters are derived. Again, an alternative to avoid the aggregation into one single value for a grid cell is to use the subgrid method in ESMs.
The upscaling effect of soil data on the model simulation has been investigated in previous studies, with controversial conclusions. For example, Melton et al. (2017) used two linked algorithms to provide tiles of representative soil textures for subgrids in a terrestrial ecosystem model and found that the model is relatively insensitive to subgrid soil textures compared to a simple grid-mean soil texture at a global scale. However, the treatment without soil subgrid structure in JULES resulted in soil-moisture-dependent anomalies in simulated carbon flux (Park et al., 2018). Further research is necessary to investigate the upscaling effect on models.
4.3 The changing soil properties
There are no global soil property maps in the time series because we do not have enough available data. In all global soil property maps, all available soil observations in recent decades have been used in the development of soil property maps without considering the changing environment. Therefore these datasets should be considered to be an average state. The critical issue for mapping global soil properties in a time series is to establish a soil profile database with time stamps and then divide them into two or more groups of different periods such as the 1950s–1970s. This is still quite challenging at the global scale because the spatial coverage of soil profiles is quite uneven for different periods and the sample size may not be adequately large to derive maps with satisfactory accuracy.
Soil properties are changing, but we now usually consider them to be static in ESMs. As some ESMs already simulate the soil carbon, this may be considered in PTFs used to estimate soil hydraulic and thermal parameters. Other soil properties affecting soil hydraulic and thermal parameters include soil texture, bulk density, and soil structure, but the change is relatively slow. The effect of environmental change on soil properties is the topic of the quantitative modelling of soil-forming processes, i.e. soil landscape and pedogenic models (Gessler et al., 1995; Minasny et al., 2008). If we need to simulate the change in soil properties, a coupling of ESMs and soil landscape and pedogenic models will be needed. Otherwise, we need to predict the soil properties in the future using soil landscape and pedogenic models, which are small scale with high uncertainty. The prediction of changing soil properties may also be performed by digital soil mapping taken the changing (especially for the future) climate and land use as covariates, which may be easier and more feasible than dynamic models.
4.4 Incorporating the uncertainty of soil data into ESMs
Incorporating the uncertainty of soil data into ESMs is increasingly challenging. Except for WISE30sec, all the current global soil datasets do not have a corresponding uncertainty map for a soil property. However, the spatial uncertainty can be estimated by the methods mentioned in Sect. 2.1, and soil datasets with uncertainty maps will be made available sooner or later. It is too expensive to run multiple ESM simulations that combine the upper and lower bounds in all possible combinations to quantify the effect of soil data uncertainty on ESMs. Instead, adaptive surrogate modelling based on statistical regression and machine learning can be used to emulate the responses of ESMs to the variation of soil properties at each location, which uses much less computing time and proves to be effective and efficient (Gong et al., 2015; Li et al., 2018).
4.5 Layer schemes and lack of deep layer soil data
The layer scheme of a soil dataset needs to be converted to that of ESMs for model use. A simple method for this conversion is the depth-weighting method. When a more accurate conversion is needed, the equal-area quadratic smoothing spline functions can be used, which is advantageous in predicting the depth function of soil properties (Bishop et al., 1999). Mass conservation for a soil property of a layer is guaranteed by this method under the assumption of a continuous vertical variation in soil properties. This method may produce some negative values that should be set to zero.
The depth of soil observations in the soil survey is usually less than 2 m and thus results in missing values for the deep layers of ESMs. For the lack of deep soil data, there is no good solution other than extrapolating the values based on the observations of shallower layers, which will lead to higher uncertainty of soil properties for deep layers. The extrapolation can be performed by the abovementioned spline method or simply by assigning the soil properties of the last layer to the rest of the deeper soil layers. The DTB map (Shangguan et al., 2017) can be utilized to define the low boundary of soil layers, and a default set of thermal and hydraulic characteristics can be assigned for bedrocks.
In this paper, the status of soil datasets and their usage in ESMs is reviewed. Soil physical and chemical properties serve as model parameters, initial variables, or benchmark datasets in ESMs. Soil profiles, soil maps, and soil datasets derived by the linkage method and digital soil mapping are reviewed at national, regional, and global levels. The soil datasets derived by digital soil mapping are considered to provide a more realistic estimation of soils than those derived by the linkage method, because digital soil mapping provides spatially continuous estimations of soil properties using spatial prediction models with various soil-related covariates. Due to the evaluation of soil datasets by WoSIS, SoilGrids have the most accurate estimation of soil properties. However, other soil datasets, including GSDE and WISE30sec, can be considered compensation, and they provide more soil properties.
The popular soil datasets used in ESMs are outdated and there are updated soil datasets available. In recent years, efforts have been made to update the soil data in ESMs. The effects of updated soil properties which are used to estimate soil hydraulic and thermal parameters were evaluated. Other major updates include soil reflectance, groundwater tables, and DTB.
PTFs are employed to estimate secondary soil parameters, including soil hydraulic and thermal parameters, and biogeochemical parameters. PTFs can take more soil properties (i.e. SOC, bulk density) as input in addition to soil texture data. An ensemble of PTFs may be more suitable for providing secondary soil parameters as direct input to ESMs, because the ensemble method has a number of benefits and potential over a single PTF (Looy et al., 2017).
Soil data derived by the linkage methods and high-resolution data can be aggregated by different methods to be used in ESMs. The aggregation should be performed after the secondary parameters are estimated. However, the aggregation will omit the soil property variation. To avoid aggregation, the subgrid method in ESMs is an alternative that increases the model complexity. The effect of different upscaling methods on the performance of ESMs needs to be further investigated.
Because digital soil mapping has many advantages compared to the traditional linkage method, especially in representing spatial heterogeneity and quantifying uncertainty in the predictions, the new generation soil datasets derived by digital soil mapping need to be tested in ESMs, and some regional studies have shown that these datasets provide better modelling results than products by the linkage method (Kearney and Maino, 2018; Trinh et al., 2018). Moreover, many studies from digital soil mapping have identified that soil maps are not very important for predicting soil properties and are usually not used as a covariate in most studies (e.g. Hengl et al., 2014; Viscarra Rossel et al., 2015; Arrouays et al., 2018). However, the linkage method usually considers the soil map to be a base map, which essentially affects the accuracy of the derived soil property maps, especially for areas without detailed soil maps. As a data-driven method, digital soil mapping requires soil profile measurements and environmental covariates (in which the importance of soil maps is low), and by including more of these data in mapping will improve the global predictions (Hengl et al., 2017). More quality assessed data, analysed according to comparable analytical methods, are needed to support such efforts. The soil data harmonization is undertaken by the work of GSP Pillar 5 (Pillar 5 Working Group, 2017) and WoSIS (Batjes et al., 2017). Data derived from proximal sensing, although with higher uncertainty than traditional soil measurements, can be used in soil mapping (England and Viscarra Rossel, 2018). To avoid spatial extrapolation, soil profiles should have good geographical coverage. The temporal variation in global soil is quite challenging due to a lack of data. Soil image fusion is also needed to merge the local and global soil maps, and this fusion considers these maps to be soil variation components for ensemble predictions (Hengl et al., 2017). It may take years before a system for automated soil image fusion is fully functional in an operational system for global soil data fusion. Mapping the soil depth and DTB separately at the global level also remains challenging due to a lack of data and the understanding of relevant processes. Uncertainty estimation, especially spatial uncertainty estimation, should be included in the soil datasets developed in the future. However, incorporating the spatial uncertainty of the soil properties into ESMs is still challenging due to the cost, and an alternative may be to use adaptive surrogate modelling.
The gap is large between the amount of data that have been obtained in surveys and the amount of data freely available. The soil profiles included in global soil databases such as WoSIS comprise a very small fraction of the soil pits dug by human beings. For example, there are more than 100 000 soil profiles from the second national soil survey of China (Zhang et al., 2010) and no more than 9000 were used to produce the national soil property maps that are freely available (Shangguan et al., 2013). In the last century, national soil surveys have been widely accomplished, primarily for agriculture purposes. However, most of these legacy data are not digitalized and they are usually not made available to the science community even if digitalized. Obtaining these hidden soil data will require some mechanism such as government-mandated regulations and money investments to make these data available (Pillar 4 Working Group, 2014; Pillar 5 Working Group, 2017). Arrouays et al. (2017) reported that about 800 000 soil profiles have been obtained from the selected countries, although most of these are not yet freely available to the international community. In addition, investments in new soil samplings should be made, especially in the under-represented areas. A good example is the US, which has the most abundant soil data freely available (https://ncsslabdatamart.sc.egov.usda.gov/, last access: 3 July 2019), similar to many other data. Censored information produces censored maps and so on. If the hidden data could be made available in any way, science and all human beings will benefit. A true big data era is waiting for us. The data compatibility of different analysis methods and different description protocols including soil classifications is also an important issue and data harmonization is necessary when the data are made available to the public.
The underlying research data used can be accessed as follows: HWSD, GSDE, WISE30sec, SoilGrids, and WoSIS are freely available at http://www.iiasa.ac.at/web/home/research/researchPrograms/water/HWSD.html (last access: 27 June 2019) (IIASA, 2019), http://globalchange.bnu.edu.cn/research/data (last access: 3 July 2019) (Land-Atmosphere Interaction Research Group, 2019), https://www.isric.org/explore/wise-databases (last access: 3 July 2019) (ISRIC, 2019a), http://www.soilgrids.org (last access: 3 July 2019) (ISRIC, 2019b), and https://www.isric.org/explore/wosis (last access: 3 July 2019) (ISRIC, 2019c) respectively.
YD planned and led the work and wrote part of the paper, WS did the analysis and wrote most of the content of the work, and DW, NW, QX, HY, SZ, SL, XL, and FY suggested the study.
The authors declare that they have no conflict of interest
We thank two anonymous referees and the editors for their comments which helped us improve the paper much.
This research has been supported by the the National Key Research and Development Program of China under grants 2017YFA0604303 and 2016YFB0200801 and the Natural Science Foundation of China (under grants 41575072, 41730962, and U1811464).
This paper was edited by Axel Don and reviewed by two anonymous referees.
Arora, V. K., Boer, G. J., Christian, J. R., Curry, C. L., Denman, K. L., Zahariev, K., Flato, G. M., Scinocca, J. F., Merryfield, W. J., and Lee, W. G.: The Effect of Terrestrial Photosynthesis Down Regulation on the Twentieth-Century Carbon Budget Simulated with the CCCma Earth System Model, J. Climate, 22, 6066–6088, 2009.
Arrouays, D., Leenaars, J. G. B., Richer-de-Forges, A. C., Adhikari, K., Ballabio, C., Greve, M., Grundy, M., Guerrero, E., Hempel, J., Hengl, T., Heuvelink, G., Batjes, N., Carvalho, E., Hartemink, A., Hewitt, A., Hong, S.-Y., Krasilnikov, P., Lagacherie, P., Lelyk, G., Libohova, Z., Lilly, A., McBratney, A., McKenzie, N., Vasquez, G. M., Mulder, V. L., Minasny, B., Montanarella, L., Odeh, I., Padarian, J., Poggio, L., Roudier, P., Saby, N., Savin, I., Searle, R., Solbovoy, V., Thompson, J., Smith, S., Sulaeman, Y., Vintila, R., Rossel, R. V., Wilson, P., Zhang, G.-L., Swerts, M., Oorts, K., Karklins, A., Feng, L., Ibelles Navarro, A. R., Levin, A., Laktionova, T., Dell'Acqua, M., Suvannang, N., Ruam, W., Prasad, J., Patil, N., Husnjak, S., Pásztor, L., Okx, J., Hallett, S., Keay, C., Farewell, T., Lilja, H., Juilleret, J., Marx, S., Takata, Y., Kazuyuki, Y., Mansuy, N., Panagos, P., Van Liedekerke, M., Skalsky, R., Sobocka, J., Kobza, J., Eftekhari, K., Alavipanah, S. K., Moussadek, R., Badraoui, M., Da Silva, M., Paterson, G., Gonçalves, M. d. C., Theocharopoulos, S., Yemefack, M., Tedou, S., Vrscaj, B., Grob, U., Kozák, J., Boruvka, L., Dobos, E., Taboada, M., Moretti, L., and Rodriguez, D.: Soil legacy data rescue via GlobalSoilMap and other international and national initiatives, GeoResJ, 14, 1–19, https://doi.org/10.1016/j.grj.2017.06.001, 2017.
Arrouays, D., Savin, I., Leenaars, J., and McBratney, A.: GlobalSoilMap – Digital Soil Mapping from Country to Globe, CRC Press, London, UK, 2018.
Ballabio, C., Panagos, P., and Monatanarella, L.: Mapping topsoil physical properties at European scale using the LUCAS database, Geoderma, 261, 110–123, 2016.
Batjes, N. H.: A taxotransfer rule-based approach for filling gaps in measured soil data in primary SOTER databases, International Soil Reference and Information Centre, Wageningen, the Netherlands, 2003.
Batjes, N. H.: ISRIC-WISE derived soil properties on a 5 by 5 arc-minutes global grid. Report 2006/02, ISRIC- World Soil Information, Wageningen (with data set), the Netherlands, 2006.
Batjes, N. H.: ISRIC-WISE harmonized global soil profile dataset (ver. 3.1). Report 2008/02, ISRIC – World Soil Information, Wageningen, the Netherlands, 2008.
Batjes, N. H.: Harmonized soil property values for broad-scale modelling (WISE30sec) with estimates of global soil carbon stocks, Geoderma, 269, 61-68, https://doi.org/10.1016/j.geoderma.2016.01.034, 2016.
Batjes, N. H., Ribeiro, E., van Oostrum, A., Leenaars, J., Hengl, T., and Mendes de Jesus, J.: WoSIS: providing standardised soil profile data for the world, Earth Syst. Sci. Data, 9, 1–14, https://doi.org/10.5194/essd-9-1-2017, 2017.
Best, M. J., Pryor, M., Clark, D. B., Rooney, G. G., Essery, R. L. H., Ménard, C. B., Edwards, J. M., Hendry, M. A., Porson, A., Gedney, N., Mercado, L. M., Sitch, S., Blyth, E., Boucher, O., Cox, P. M., Grimmond, C. S. B., and Harding, R. J.: The Joint UK Land Environment Simulator (JULES), model description – Part 1: Energy and water fluxes, Geosci. Model Dev., 4, 677–699, https://doi.org/10.5194/gmd-4-677-2011, 2011.
Bishop, T. F. A., McBratney, A. B., and Laslett, G. M.: Modelling soil attribute depth functions with equal-area quadratic smoothing splines, Geoderma, 91, 27–45, 1999.
Brunke, M. A., Broxton, P., Pelletier, J., Gochis, D., Hazenberg, P., Lawrence, D. M., Leung, L. R., Niu, G.-Y., Troch, P. A., and Zeng, X.: Implementing and evaluating variable soil thickness in the Community Land Model version 4.5 (CLM4.5), J. Climate, 29, 3441–3461, https://doi.org/10.1175/JCLI-D-15-0307.1, 2016.
Chen, F. and Dudhia, J.: Coupling an advanced land surface-hydrology model with the Penn State-NCAR MM5 modeling system, Part I: Model implementation and sensitivity, Mon. Weather Revi., 129, 569–585, 2001.
Chen, Y., Yang, K., Tang, W., Qin, J., and Zhao, L.: Parameterizing soil organic carbon's impacts on soil porosity and thermal parameters for Eastern Tibet grasslands, Sci. China Earth Sci., 55, 1001–1011, https://doi.org/10.1007/s11430-012-4433-0, 2012.
Clapp, R. W. and Hornberger, G. M.: Empirical equations for some soil hydraulic properties, Water Resour. Res., 14, 601–604, 1978.
Clark, D. B., Mercado, L. M., Sitch, S., Jones, C. D., Gedney, N., Best, M. J., Pryor, M., Rooney, G. G., Essery, R. L. H., Blyth, E., Boucher, O., Harding, R. J., Huntingford, C., and Cox, P. M.: The Joint UK Land Environment Simulator (JULES), model description – Part 2: Carbon fluxes and vegetation dynamics, Geosci. Model Dev., 4, 701–722, https://doi.org/10.5194/gmd-4-701-2011, 2011.
Cooper, M., Mendes, L. M. S., Silva, W. L. C., and Sparovek, G.: A national soil profile database for brazil available to international scientists, Soil Sci. Soci. Am. J., 69, 649–652, 2005.
Cox, P. M., Betts, R. A., Bunton, C. B., Essery, R. L. H., Rowntree, P. R., and Smith, J.: The impact of new land surface physics on the GCM sensitivity of climate and climate sensitivity, Clim. Dynam., 15, 183–203, 1999.
Dai, Y., Zeng, X., Dickinson, R. E., Baker, I., Bonan, G. B., Bosilovich, M. G., Denning, A. S., Dirmeyer, P. A., Houser, P. R., Niu, G., Oleson, K. W., Schlosser, C. A., and Yang, Z.: The Common Land Model, B. Am. Meteorol. Soc., 84, 1013–1023, 2003.
Dai, Y., Shangguan, W., Duan, Q., Liu, B., Fu, S., and Niu, G.: Development of a China Dataset of Soil Hydraulic Parameters Using Pedotransfer Functions for Land Surface Modeling, J. Hydrometeorol., 14, 869–887, 2013.
De Lannoy, G. J. M., Koster, R. D., Reichle, R. H., Mahanama, S. P. P., and Liu, Q.: An updated treatment of soil texture and associated hydraulic properties in a global land modeling system, J. Adv. Model. Earth Sy., 6, 957-979, https://doi.org/10.1002/2014ms000330, 2014.
Dickinson, R. E., Henderson-Sellers, A., and Kennedy, P. J.: Biosphere-Atmosphere Transfer Scheme (BATS) Version 1e as Coupled to the NCAR Community Climate Model, NCAR-TN-387+STR, National Center for Atmospheric Research, Boulder, Colorado, USA, 88 pp., 1993.
Doney, S. C., Lindsay, K., Fung, I., and John, J.: Natural variability in a stable, 1000-yr global coupled climate-carbon cycle simulation, J. Climate, 19, 3033–3054, 2006.
Dy, C. Y. and Fung, J. C. H. C. J.: Updated global soil map for the Weather Research and Forecasting model and soil moisture initialization for the Noah land surface model, J. Geophys. Res.-Atmos., 121, 8777–8800, https://doi.org/10.1002/2015jd024558, 2016.
Elguindi, N., Bi, X., Giorgi, F., Nagarajan, B., Pal, J., Solmon, F., Rauscher, S., Zakey, A., O'Brien, T., Nogherotto, R., and Giuliani, G.: Regional climatic model RegCM Reference Manual version 4.6, 33, ITCP, Trieste, Italy, 2014.
England, J. R. and Viscarra Rossel, R. A.: Proximal sensing for soil carbon accounting, SOIL, 4, 101–122, https://doi.org/10.5194/soil-4-101-2018, 2018.
Fan, Y., Li, H., and Miguez-Macho, G.: Global Patterns of Groundwater Table Depth, Science, 339, 940–943, https://doi.org/10.1126/science.1229881, 2013.
Guevara, M., Olmedo, G. F., Stell, E., Yigini, Y., Aguilar Duarte, Y., Arellano Hernàndez, C., Arévalo, G. E., Arroyo-Cruz, C. E., Bolivar, A., Bunning, S., Bustamante Cañas, N., Cruz-Gaistardo, C. O., Davila, F., Dell Acqua, M., Encina, A., Figueredo Tacona, H., Fontes, F., Hernández Herrera, J. A., Ibelles Navarro, A. R., Loayza, V., Manueles, A. M., Mendoza Jara, F., Olivera, C., Osorio Hermosilla, R., Pereira, G., Prieto, P., Ramos, I. A., Rey Brina, J. C., Rivera, R., Rodríguez-Rodríguez, J., Roopnarine, R., Rosales Ibarra, A., Rosales Riveiro, K. A., Schulz, G. A., Spence, A., Vasques, G. M., Vargas, R. R., and Vargas, R.: No silver bullet for digital soil mapping: country-specific soil organic carbon estimates across Latin America, SOIL, 4, 173–193, https://doi.org/10.5194/soil-4-173-2018, 2018.
FAO: Soil Map of the World, Vol. 110, UNESCO, Paris, France, 1981.
FAO: Digitized Soil Map of the World and Derived Soil Properties, FAO, Rome, Italy, 1995.
FAO: Digital soil map of the world and derived soil properties, FAO, Land and Water Digital Media Series, CD-ROM, 2003a.
FAO: The Digitized Soil Map of the World Including Derived Soil Properties (version 3.6), FAO, Rome, Italy, 2003b.
FAO/IIASA/ISRIC/ISS-CAS/JRC: Harmonized World Soil Database (version1.2), FAO, Rome, Italy and IIASA, Laxenburg, Austria, 2012.
Farouki, O. T.: Thermal Properties of Soils. Monograph, No. 81-1, U.S. Army Cold Regions Research and Engineering Laboratory, Hanover, NH, USA, 1981.
Folberth, C., Skalský, R., Moltchanova, E., Balkovič, J., Azevedo, L. B., Obersteiner, M., and van der Velde, M.: Uncertainty in soil data can outweigh climate impact signals in global crop yield simulations, Nat. Commun., 7, 11872, https://doi.org/10.1038/ncomms11872, 2016.
Gessler, P. E., Moore, I. D., McKenzie, N. J., and Ryan, P. J.: Soil-landscape modelling and spatial prediction of soil attributes, Int. J. Geogr. Inf. Syst., 9, 421–432, 1995.
Global Soil DataTask: Global Soil Data Products CD-ROM (IGBP-DIS), International Geosphere-Biosphere Programme – Data and Information Services, Available online at from the ORNL Distributed Active Archive Center, Oak Ridge National Laboratory, Oak Ridge, Tennessee, USA, 2000.
Gong, W., Duan, Q., Li, J., Wang, C., Di, Z., Dai, Y., Ye, A., and Miao, C.: Multi-objective parameter optimization of common land model using adaptive surrogate modeling, Hydrol. Earth Syst. Sci., 19, 2409–2425, https://doi.org/10.5194/hess-19-2409-2015, 2015.
Gurney, K. R., Baker, D., Rayner, P., and Denning, S.: Interannual variations in continental-scale net carbon exchange and sensitivity to observing networks estimated from atmospheric CO2 inversions for the period 1980 to 2005, Global Biogeochem. Cy., 22, GB3025, https://doi.org/10.1029/2007GB003082, 2008.
Hagemann, S.: An Improved Land Surface Parameter Dataset for Global and Regional Climate Models, MPI Report No. 336, 28 pp., 2002.
Hagemann, S., Botzet, M., Dümenil, L., and Machenhauer, B.: Derivation of global GCM boundary conditions from 1 km land use satellite data, MPI Report No. 289, 34 pp., 1999.
Hannam, J. A., Hollis, J. M., Jones, R. J. A., Bellamy, P. H., Hayes, S. E., Holden, A., Van Liedekerke, M. H., and Montanarella, L.: SPADE-2: The soil profile analytical database for Europe, Version 2.0 Beta Version March 2009, unpublished Report, 27 pp., 2009.
Hengl, T., de Jesus, J. M., MacMillan, R. A., Batjes, N. H., Heuvelink, G. B. M., Ribeiro, E., Samuel-Rosa, A., Kempen, B., Leenaars, J. G. B., Walsh, M. G., and Gonzalez, M. R.: SoilGrids1km – Global Soil Information Based on Automated Mapping, PLoS ONE, 9, e105992, https://doi.org/10.1371/journal.pone.0105992, 2014.
Hengl, T., Heuvelink, G. B. M., Kempen, B., Leenaars, J. G. B., Walsh, M. G., Shepherd, K. D., Sila, A., MacMillan, R. A., Jesus, J. M. D., Tamene, L., and Tondoh, J. E.: Mapping Soil Properties of Africa at 250 m Resolution: Random Forests Significantly Improve Current Predictions, PLOS ONE, 10, e0125814, 2015.
Hengl, T., Mendes de Jesus, J., Heuvelink, G. B. M., Ruiperez Gonzalez, M., Kilibarda, M., Blagotic, A., Shangguan, W., Wright, M. N., Geng, X., Bauer-Marschallinger, B., Guevara, M. A., Vargas, R., MacMillan, R. A., Batjes, N. H., Leenaars, J. G. B., Ribeiro, E., Wheeler, I., Mantel, S., and Kempen, B.: SoilGrids250m: global gridded soil information based on Machine Learning, PLOS One, 12, e0169748, https://doi.org/10.1371/journal.pone.0169748, 2017.
Hiederer, R. and Köchy, M.: Global Soil Organic Carbon Estimates and the Harmonized World Soil Database, Publications Office of the European Union, Luxembourg, 79 pp., 2012.
Hoffmann, H., Zhao, G., Asseng, S., Bindi, M., Biernath, C., Constantin, J., Coucheney, E., Dechow, R., Doro, L., Eckersten, H., Gaiser, T., Grosz, B., Heinlein, F., Kassie, B. T., Kersebaum, K.-C., Klein, C., Kuhnert, M., Lewan, E., Moriondo, M., Nendel, C., Priesack, E., Raynal, H., Roggero, P. P., Rötter, R. P., Siebert, S., Specka, X., Tao, F., Teixeira, E., Trombi, G., Wallach, D., Weihermüller, L., Yeluripati, J., and Ewert, F.: Impact of Spatial Soil and Climate Input Data Aggregation on Regional Yield Simulations, PLOS One, 11, e0151782, https://doi.org/10.1371/journal.pone.0151782 2016.
Hugelius, G., Tarnocai, C., Broll, G., Canadell, J. G., Kuhry, P., and Swanson, D. K.: The Northern Circumpolar Soil Carbon Database: spatially distributed datasets of soil coverage and soil carbon storage in the northern permafrost regions, Earth Syst. Sci. Data, 5, 3–13, https://doi.org/10.5194/essd-5-3-2013, 2013.
IIASA: Harmonized World Soil Database (HWSD v 1.21), available at: http://www.iiasa.ac.at/web/home/research/researchPrograms/water/HWSD.html, last access: 27 June 2019.
Instituto Nacional de Estadística y Geografía: Conjunto de Datos de Perfiles de Suelos Escala 1 : 250 000 Serie II (Continuo Nacional), INEGI, Aguascalientes, Ags. Mexico, 2016.
ISRIC: WISE Soil Property Databases, available at: https://www.isric.org/explore/wise-databases, last access: 27 June 2019a.
ISRIC: SoilGrids, available at: http://www.soilgrids.org, last access: 27 June 2019b.
ISRIC: WoSIS, available at: https://www.isric.org/explore/wosis, last access: 27 June 2019c.
Ji, P., Yuan, X., and Liang, X.-Z.: Do Lateral Flows Matter for the Hyperresolution Land Surface Modeling?, J. Geophys. Res.-Atmos., 122, 12077–12092, https://doi.org/10.1002/2017JD027366, 2017.
Johnston, R. M., Barry, S. J., Bleys, E., Bui, E. N., Moran, C. J., Simon, D. A. P., Carlile, P., McKenzie, N. J., Henderson, B. L., Chapman, G., Imhoff, M., Maschmedt, D., Howe, D., Grose, C., and Schoknecht, N.: ASRIS: the database, Aust. J. Soil Res., 416, 1021–1036, 2003.
Jordan, H., Tom, G., Jens, H., and Janine, B.: Compiling and Mapping Global Permeability of the Unconsolidated and Consolidated Earth: GLobal HYdrogeology MaPS 2.0 (GLHYMPS 2.0), Geophys. Res. Lett., 45, 1897–1904, https://doi.org/10.1002/2017GL075860, 2018.
Karssies, L.: CSIRO National Soil Archive and the National Soil Database (NatSoil), No. v1 in Data Collection, CSIRO, Canberra, Australia, 2011.
Kearney, M. R. and Maino, J. L.: Can next-generation soil data products improve soil moisture modelling at the continental scale? An assessment using a new microclimate package for the R programming environment, J. Hydrol., 561, 662–673, https://doi.org/10.1016/j.jhydrol.2018.04.040, 2018.
Koster, R. D. and Suarez, M. J.: Modeling the land surface boundary in climate models as a composite of independent vegetation stands, J. Geophys. Res.-Atmos., 97, 2697–2715, https://doi.org/10.1029/91JD01696, 1992.
Kowalczyk, E., Stevens, L., Law, R., Dix, M., Wang, Y., Harman, I., Haynes, K., Srbinovsky, J., Pak, B., and Ziehn, T: The land surface model component of ACCESS: description and impact on the simulated surface climatology, Aust. Meteorol. Ocean., 63, 65–82, 2013.
Krinner, G., Viovy, N., de Noblet-Ducoudré, N., Ogée, J., Polcher, J., Friedlingstein, P., Ciais, P., Sitch, S., and Prentice, I. C.: A dynamic global vegetation model for studies of the coupled atmosphere-biosphere system, Global Biogeochem. Cy., 19, GB1015, https://doi.org/10.1029/2003gb002199, 2005.
Kuhnert, M., Yeluripati, J., Smith, P., Hoffmann, H., van Oijen, M., Constantin, J., Coucheney, E., Dechow, R., Eckersten, H., Gaiser, T., Grosz, B., Haas, E., Kersebaum, K.-C., Kiese, R., Klatt, S., Lewan, E., Nendel, C., Raynal, H., Sosa, C., Specka, X., Teixeira, E., Wang, E., Weihermüller, L., Zhao, G., Zhao, Z., Ogle, S., and Ewert, F.: Impact analysis of climate data aggregation at different spatial scales on simulated net primary productivity for croplands, Eur. J. Agron., 88, 41–52, https://doi.org/10.1016/j.eja.2016.06.005, 2017.
Land-Atmosphere Interaction Research Group: available at: http://globalchange.bnu.edu.cn/research/data, last access: 27 June 2019.
Landon, J. R.: Booker Tropical Soil Manual, Longman Scientific &Technical, New York, USA, 1991.
Lawrence, P. J. and Chase, T. N.: Representing a new MODIS consistent land surface in the Community Land Model (CLM 3.0), J. Geophys. Res., 112, G01023, https://doi.org/10.1029/2006JG000168, 2007.
Leenaars, J. G. B.: Africa Soil Profiles Database, Version 1.0. A compilation of geo-referenced and standardized legacy soil profile data for Sub Saharan Africa (with dataset), ISRIC report 2012/03, Africa Soil Information Service (AfSIS) project and ISRIC – World Soil Information, Wageningen, the Netherlands, 2012.
Lei, H., Yang, D., and Huang, M.: Impacts of climate change and vegetation dynamics on runoff in the mountainous region of the Haihe River basin in the past five decades, J. Hydrol., 511, 786–799, https://doi.org/10.1016/j.jhydrol.2014.02.029, 2014.
Li, C., Lu, H., Yang, K., Wright, J. S., Yu, L., Chen, Y., Huang, X., and Xu, S.: Evaluation of the Common Land Model (CoLM) from the Perspective of Water and Energy Budget Simulation: Towards Inclusion in CMIP6, Atmosphere, 8, 141, https://doi.org/10.3390/atmos8080141, 2017.
Li, J., Duan, Q., Wang, Y.-P., Gong, W., Gan, Y., and Wang, C.: Parameter optimization for carbon and water fluxes in two global land surface models based on surrogate modelling, Int. J. Climatol., 38, e1016–e1031, https://doi.org/10.1002/joc.5428, 2018.
Liang, X., Lettenmaier, D. P., Wood, E. F., and Burges, S. J.: A simple hydrologically based model of land surface water and energy fluxes for general circulation models, J. Geophys. Res.-Atmos., 99, 14415–14428, https://doi.org/10.1029/94JD00483, 1994.
Livneh, B., Kumar, R., and Samaniego, L.: Influence of soil textural properties on hydrologic fluxes in the Mississippi river basin, Hydrol. Process., 29, 4638–4655, https://doi.org/10.1002/hyp.10601, 2015.
Looy, K. V., Bouma, J., Herbst, M., Koestel, J., Minasny, B., Mishra, U., Montzka, C., Nemes, A., Pachepsky, Y. A., Padarian, J., Schaap, M. G., Tóth, B., Verhoef, A., Vanderborght, J., Ploeg, M. J., Weihermüller, L., Zacharias, S., Zhang, Y., and Vereecken, H.: Pedotransfer Functions in Earth System Science: Challenges and Perspectives, Rev. Geophys., 55, 1199–1256, https://doi.org/10.1002/2017RG000581, 2017.
Luo, Y., Ahlström, A., Allison, S. D., Batjes, N. H., Brovkin, V., Carvalhais, N., Chappell, A., Ciais, P., Davidson, E. A., Finzi, A., Georgiou, K., Guenet, B., Hararuk, O., Harden, J. W., He, Y., Hopkins, F., Jiang, L., Koven, C., Jackson, R. B., Jones, C. D., Lara, M. J., Liang, J., McGuire, A. D., Parton, W., Peng, C., Randerson, J. T., Salazar, A., Sierra, C. A., Smith, M. J., Tian, H., Todd-Brown, K. E. O., Torn, M., van Groenigen, K. J., Wang, Y. P., West, T. O., Wei, Y., Wieder, W. R., Xia, J., Xu, X., Xu, X., and Zhou, T. C. G. B.: Toward more realistic projections of soil carbon dynamics by Earth system models, Global Biogeochem. Cy., 30, 40–56, https://doi.org/10.1002/2015gb005239, 2016.
MacDonald, K. B. and Valentine, K. W. G.: CanSIS/NSDB, A general description (Centre for Land and Biological Resources Research), Research Branch, Agriculture Canada, Ottawa, Canada, 1992.
Mauritsen, T., Bader, J., Becker, T., Behrens, J., Bittner, M., Brokopf, R., Brovkin, V., Claussen, M., Crueger, T., Esch, M., Fast, I., Fiedler, S., Fläschner, D., Gayler, V., Giorgetta, M., Goll, D. S., Haak, H., Hagemann, S., Hedemann, C., Hohenegger, C., Ilyina, T., Jahns, T., Jimenez de la Cuesta Otero, D., Jungclaus, J., Kleinen, T., Kloster, S., Kracher, D., Kinne, S., Kleberg, D., Lasslop, G., Kornblueh, L., Marotzke, J., Matei, D., Meraner, K., Mikolajewicz, U., Modali, K., Möbis, B., Müller, W. A., Nabel, J. E. M. S., Nam, C. C. W., Notz, D., Nyawira, S.-S., Paulsen, H., Peters, K., Pincus, R., Pohlmann, H., Pongratz, J., Popp, M., Raddatz, T., Rast, S., Redler, R., Reick, C. H., Rohrschneider, T., Schemann, V., Schmidt, H., Schnur, R., Schulzweida, U., Six, K. D., Stein, L., Stemmler, I., Stevens, B., von Storch, J.-S., Tian, F., Voigt, A., de Vrese, P., Wieners, K.-H., Wilkenskjeld, S., Winkler, A., and Roeckner, E.: Developments in the MPI-M Earth System Model version 1.2 (MPI-ESM 1.2) and its response to increasing CO2, J. Adv. Model. Earth Sy., 11, 998–1038, 2019.
McBratney, A. B., Santos, M. L. M., and Minasny, B.: On digital soil mapping, Geoderma, 117, 3–52, https://doi.org/10.1016/s0016-7061(03)00223-4, 2003.
McBratney, A. B., Minasny, B., and Tranter, G.: Necessary meta-data for pedotransfer functions, Geoderma, 160, 627–629, 2011.
McGuire, A. D., Melillo, J. M., Kicklighter, D. W., Pan, Y. D., Xiao, X. M., Helfrich, J., Moore, B., Vorosmarty, C. J., and Schloss, A. L.: Equilibrium responses of global net primary production and carbon storage to doubled atmospheric carbon dioxide: sensitivity to changes in vegetation nitrogen concentration, Global Biogeochem. Cy., 11, 173–189, 1997.
McLellan, I., Varela, A., Blahgen, M., Fumi, M. D., Hassen, A., Hechminet, N., Jaouani, A., Khessairi, A., Lyamlouli, K., Ouzari, H.-I., Mazzoleni, V., Novelli, E., Pintus, A., Rodrigues, C., Ruiu, P. A., Pereira, C. S., and Hursthouse, A.: Harmonisation of physical and chemical methods for soil management in Cork Oak forests – Lessons from collaborative investigations, African Journal of Environmental Science and Technology, 7, 386–401, 2013.
Melton, J. R., Sospedra-Alfonso, R., and McCusker, K. E.: Tiling soil textures for terrestrial ecosystem modelling via clustering analysis: a case study with CLASS-CTEM (version 2.1), Geosci. Model Dev., 10, 2761–2783, https://doi.org/10.5194/gmd-10-2761-2017, 2017.
Miller, D. A. and White, R. A.: A conterminous United States multilayer soil characteristics dataset for regional climate and hydrology modeling, Earth Interact., 2, 1–26, https://doi.org/10.1175/1087-3562(1998)002<0001:ACUSMS>2.3.CO;2, 1998.
Minasny, B., McBratney, A. B. and Salvador-Blanes, S.: Quantitative models for pedogenesis – A review, Geoderma, 144, 140–157, 2008.
Moigne, P.: SURFEX scientific documentation, Centre National de Recherches Meteorologiques, Toulouse and Grenoble, France, 2018.
Montzka, C., Herbst, M., Weihermüller, L., Verhoef, A., and Vereecken, H.: A global data set of soil hydraulic properties and sub-grid variability of soil water retention and hydraulic conductivity curves, Earth Syst. Sci. Data, 9, 529–543, https://doi.org/10.5194/essd-9-529-2017, 2017.
Mulder, V. L., Lacoste, M., Richer-de-Forges, A. C., and Arrouays, D.: GlobalSoilMap France: High-resolution spatial modelling the soils of France up to two meter depth, Sci. Total Environ., 573, 1352–1369, https://doi.org/10.1016/j.scitotenv.2016.07.066, 2016.
National Soil Survey Office: Soil Map of China, China Map Press, Beijing, 1995 (in Chinese).
Niu, G.-Y., Yang, Z.-L., Mitchell, K. E., Chen, F., Ek, M. B., Barlage, M., Kumar, A., Manning, K., Niyogi, D., Rosero, E., Tewari, M., and Xia, Y.: The community Noah land surface model with multiparameterization options (Noah-MP): 1. Model description and evaluation with local-scale measurements, J. Geophys. Res.-Atmos., 116, D12110, https://doi.org/10.1029/2010JD015139, 2011.
Odgers, N. P., Libohova, Z., and Thompson, J. A.: Equal-area spline functions applied to a legacy soil database to create weighted-means maps of soil organic carbon at a continental scale, Geoderma, 189–190, 153–163, 2012.
Oleson, K. W., Lawrence, D. M., Bonan, G. B., Drewniak, B., Huang, M., Koven, C. D., Levis, S., Li, F., Riley, W. J., Subin, Z. M., Swenson, S.C., Thornton, P. E., Bozbiyik, A., Fisher, R., Kluzek, E., Lamarque, J.-F., Lawrence, P. J., Leung, L. R., Lipscomb, W., Muszala, S., Ricciuto, D. M., Sacks, W., Sun, Y., Tang, J., and Yang, Z.-L.: Technical Description of version 4.5 of the Community Land Model (CLM). Ncar Technical Note NCAR/TN-503+STR, National Center for Atmospheric Research, Boulder, CO, USA, 420 pp., 2013.
Orth, R., Dutra, E., and Pappenberger, F.: Improving Weather Predictability by Including Land Surface Model Parameter Uncertainty, Mon. Weather Rev., 144, 1551–1569, 2016.
Oz, B., Deutsch, C. V., and Frykman, P.: A visualbasic program for histogram and variogram scaling, Comput. Geosci., 28, 21–31, https://doi.org/10.1016/S0098-3004(01)00011-5, 2002.
Park, J., Kim, H.-S., Lee, S.-J., and Ha, T.: Numerical Evaluation of JULES Surface Tiling Scheme with High-Resolution Atmospheric Forcing and Land Cover Data, SOLA, 14, 19–24, https://doi.org/10.2151/sola.2018-004, 2018.
Pelletier, J. D., Broxton, P. D., Hazenberg, P., Zeng, X., Troch, P. A., Niu, G.-Y., Williams, Z., Brunke, M. A., and Gochis, D.: A gridded global data set of soil, immobile regolith, and sedimentary deposit thicknesses for regional and global land surface modeling, J. Adv. Modeling Earth Sy., 8, 41–65, https://doi.org/10.1002/2015MS000526, 2016.
Pillar 5 Working Group: Implementation Plan for Pillar Five of the Global Soil Partnership, FAO, Rome, Italy, 2017.
Pillar 4 Working Group: Plan of Action for Pillar Four of the Global Soil Partnership, FAO, Rome, Italy, 2014.
Post, D. F., Fimbres, A., Matthias, A. D., Sano, E. E., Accioly, L., Batchily, A. K., and Ferreira, L. G.: Predicting Soil Albedo from Soil Color and Spectral Reflectance Data, Soil Sci. Soc. Am. J., 64, 1027–1034, 2000.
Quattrochi, D. A., Emerson, C. W., Lam, N. S.-N., and Qiu, H.-L.: Fractal Characterization of Multitemporal Remote Sensing Data, in: Modelling Scale in Geographical Information System, edited by: Tate, N. and Atkinson, P., John Wiley & Sons, London, UK, 13–34, 2001.
Ramcharan, A., Hengl, T., Nauman, T., Brungard, C., Waltman, S., Wills, S., and Thompson, J.: Soil Property and Class Maps of the Conterminous United States at 100-Meter Spatial Resolution, Soil Sci. Soc. Am. J., 82, 186–201, https://doi.org/10.2136/sssaj2017.04.0122, 2018.
Ribeiro, E., Batjes, N. H., and Oostrum, A. V.: World Soil Information Service (WoSIS) – Towards the standardization and harmonization of world soil data, ISRIC – World Soil Information, Wageningen, the Netherlands, 2018.
Reynolds, C. A., Jackson, T. J., and Rawls, W. J.: Estimating soil water-holding capacities by linking the Food and Agriculture Organization Soil map of the world with global pedon databases and continuous pedotransfer functions, Water Resour. Res., 36, 3653–3662, 2000.
Romanowicz, A. A., Vanclooster, M., Rounsevell, M., and Junesse, I. L.: Sensitivity of the SWAT model to the soil and land use data parametrisation: a case study in the Thyle catchment, Belgium, Ecol. Model., 187, 27–39, 2005.
Rosenzweig, C. and Abramopoulos, F.: Land surface model development for the GISS GCM, J. Climate, 10, 2040–2054, 1997.
Ross, C. W., Prihodko, L., Anchang, J., Kumar, S., Ji, W., and Hanan, N. P.: HYSOGs250m, global gridded hydrologic soil groups for curve-number-based runoff modeling, Scientific Data, 5, 180091, https://doi.org/10.1038/sdata.2018.91, 2018.
Rotstayn, L. D., Jeffrey, S. J., Collier, M. A., Dravitzki, S. M., Hirst, A. C., Syktus, J. I., and Wong, K. K.: Aerosol- and greenhouse gas-induced changes in summer rainfall and circulation in the Australasian region: a study using single-forcing climate simulations, Atmos. Chem. Phys., 12, 6377–6404, https://doi.org/10.5194/acp-12-6377-2012, 2012.
Saha, S., Moorthi, S., Wu, X., Wang, J., Nadiga, S., Tripp, P., Behringer, D., Hou, Y.-T., Chuang, H.-y., Iredell, M., Ek, M., Meng, J., Yang, R., Mendez, M.P., Dool, H.v.d., Zhang, Q., Wang, W., Chen, M., and Becker, E.: The NCEP Climate Forecast System Version 2, J. Climate 27, 2185–2208, 2014.
Sanchez, P. A., Ahamed, S., Carré, F., Hartemink, A. E., Hempel, J., Huising, J., Lagacherie, P., McBratney, A. B., McKenzie, N. J., Mendonça-Santos, M. d. L., Budiman Minasny, L. M., Okoth, P., Palm, C. A., Sachs, J. D., Shepherd, K. D., Vågen, T.-G., Vanlauwe, B., Walsh, M. G., Winowiecki, L. A., and Zhang, G.-L.: Digital soil map of the world, Science, 325, 680–681, 2009.
Sellers, P. J., Randall, D. A., Collatz, G. J., Berry, J. A., Field, C. B., Dazlich, D. A., Zhang, C., Collelo, G. D., and Bounoua, L.: A revised land surface parameterization (SiB2) for atmospheric GCMs, Part I: model formulation, J. Climate, 9, 676–705, 1996.
Shangguan, W.: Comparison of aggregation ways on soil property maps, 20th World Congress of Soil Science, 8–13 June 2019, Jeju, Korea, 2014.
Shangguan, W., Dai, Y., Liu, B., Ye, A., and Yuan, H.: A soil particle-size distribution dataset for regional land and climate modelling in China, Geoderma, 171–172, 85–91, 2012.
Shangguan, W., Dai, Y., Liu, B., Zhu, A., Duan, Q., Wu, L., Ji, D., Ye, A., Yuan, H., Zhang, Q., Chen, D., Chen, M., Chu, J., Dou, Y., Guo, J., Li, H., Li, J., Liang, L., Liang, X., Liu, H., Liu, S., Miao, C., and Zhang, Y.: A China dataset of soil properties for land surface modeling, J. Adv. Model. Earth Sy., 5, 212–224, https://doi.org/10.1002/jame.20026, 2013.
Shangguan, W., Dai, Y., Duan, Q., Liu, B., and Yuan, H.: A global soil data set for earth system modeling, J. Adv. Model. Earth Sy., 6, 249–263, 2014.
Shangguan, W., Hengl, T., Mendes de Jesus, J., Yuan, H., and Dai, Y.: Mapping the global depth to bedrock for land surface modeling, J. Adv. Model. Earth Sy., 9, 65–88, https://doi.org/10.1002/2016ms000686, 2017.
Shoba, S. A., Stolbovoi, V. S., Alyabina, I. O., and Molchanov, E. N.: Soil-geographic database of Russia, Eurasian Soil Sci., 41, 907–913, https://doi.org/10.1134/s1064229308090019, 2008.
Singh, R. S., Reager, J. T., Miller, N. L., and Famiglietti, J. S.: Toward hyper-resolution land-surface modeling: The effects of fine-scale topography and soil texture on CLM4.0 simulations over the Southwestern U.S., Water Resour. Res., 51, 2648–2667, https://doi.org/10.1002/2014WR015686, 2015.
Slevin, D., Tett, S. F. B., Exbrayat, J.-F., Bloom, A. A., and Williams, M.: Global evaluation of gross primary productivity in the JULES land surface model v3.4.1, Geosci. Model Dev., 10, 2651–2670, https://doi.org/10.5194/gmd-10-2651-2017, 2017.
Soil Landscapes of Canada Working Group: Soil Landscapes of Canada version 3.2., Agriculture and Agri-Food Canada, Ottawa, Ontario, Canada, 2010.
Soil Survey Staff (NRCS): United States Department of Agriculture: Web Soil Survey, available at: http://websoilsurvey.nrcs.usda.gov/, last access: 1 January 2017.
Stoorvogel, J. J., Bakkenes, M., Temme, A. J. A. M., Batjes, N. H., and Brink, B. J. E.: S-World: A Global Soil Map for Environmental Modelling, Land Degrad. Dev., 28, 22–33, https://doi.org/10.1002/ldr.2656, 2017.
Takata, K., Emori, S., and Watanabe, T.: Development of the minimal advanced treatments of surface interaction and runoff, Global Planet. Change, 38, 209–222, 2003.
Thompson, J. A., Prescott, T., Moore, A. C., Bell, J., Kautz, D. R., Hempel, J. W., Waltman, S. W., and Perry, C. H.: Regional approach to soil property mapping using legacy data and spatial disaggregation techniques, 19th World Congress of Soil Science, Brisbane, Queensland, Australia, 2010,
Thornton, P. E. and Rosenbloom, N. A.: Ecosystem model spin-up: estimating steady state conditions in a coupled terrestrial carbon and nitrogen cycle model, Ecol. Model., 189, 25–48, 2005.
Tian, W., Li, X., Wang, X.-S., and Hu, B. X.: Coupling a groundwater model with a land surface model to improve water and energy cycle simulation, Hydrol. Earth Syst. Sci. Discuss., 9, 1163–1205, https://doi.org/10.5194/hessd-9-1163-2012, 2012.
Tifafi, M., Guenet, B., and Hatté, C.: Large Differences in Global and Regional Total Soil Carbon Stock Estimates Based on SoilGrids, HWSD, and NCSCD: Intercomparison and Evaluation Based on Field Data From USA, England, Wales, and France, Global Biogeochem. Cy., 32, 42–56, https://doi.org/10.1002/2017GB005678, 2018.
Todd-Brown, K. E. O., Randerson, J. T., Post, W. M., Hoffman, F. M., Tarnocai, C., Schuur, E. A. G., and Allison, S. D.: Causes of variation in soil carbon simulations from CMIP5 Earth system models and comparison with observations, Biogeosciences, 10, 1717–1736, https://doi.org/10.5194/bg-10-1717-2013, 2013.
Todd-Brown, K. E. O., Randerson, J. T., Hopkins, F., Arora, V., Hajima, T., Jones, C., Shevliakova, E., Tjiputra, J., Volodin, E., Wu, T., Zhang, Q., and Allison, S. D.: Changes in soil organic carbon storage predicted by Earth system models during the 21st century, Biogeosciences, 11, 2341–2356, https://doi.org/10.5194/bg-11-2341-2014, 2014.
Tóth, B., Weynants, M., Nemes, A., Makó, A., Bilas, G., and Tóth, G.: New generation of hydraulic pedotransfer functions for Europe, Eur. J. Soil Sci., 66, 226–238, https://doi.org/10.1111/ejss.12192, 2015.
Tóth, B., Weynants, M., Pásztor, L., and Hengl, T.: 3-D soil hydraulic database of Europe at 250 m resolution, Hydrol. Process., 31, 2662–2666, https://doi.org/10.1002/hyp.11203, 2017.
Trinh, T., Kavvas, M. L., Ishida, K., Ercan, A., Chen, Z. Q., Anderson, M. L., Ho, C., and Nguyen, T.: Integrating global land-cover and soil datasets to update saturated hydraulic conductivity parameterization in hydrologic modeling, Sci. Total Environ., 631–632, 279–288, https://doi.org/10.1016/j.scitotenv.2018.02.267, 2018.
Van Engelen, V. and Dijkshoorn, J.: Global and National Soils and Terrain Digital Databases (SOTER), Procedures Manual, version 2.0. ISRIC Report 2012/04, ISRIC – World Soil Information, Wageningen, the Netherlands, 2012.
Vaysse, K. and Lagacherie, P.: Using quantile regression forest to estimate uncertainty of digital soil mapping products, Geoderma, 291, 55–64, https://doi.org/10.1016/j.geoderma.2016.12.017, 2017.
Vereecken, H., Weynants, M., Javaux, M., Pachepsky, Y., Schaap, M. G., and Genuchten, M. T. V.: Using pedotransfer functions to estimate the van Genuchten-Mualem soil hydraulic properties: a review, Vadose Zone J., 9, 795–820, 2010.
Viscarra Rossel, R., Chen, C., Grundy, M., Searle, R., Clifford, D., and Campbell, P.: The Australian three-dimensional soil grid: Australia's contribution to the GlobalSoilMap project, Soil Res., 53, 845–864, 2015.
Verseghy, D.: The Canadian land surface scheme (CLASS): Itshistory and future, Atmos. Ocean, 38, 1–13, 2000.
Vrettas, M. D. and Fung, I. Y.: Toward a new parameterization of hydraulic conductivity in climate models: Simulation of rapid groundwater fluctuations in Northern California, J. Adv. Model. Earth Sy., 7, 2105–2135, https://doi.org/10.1002/2015ms000516, 2016.
Wang, G., Gertner, G., and Anderson, A. B.: Up-scaling methods based on variability-weighting and simulation for inferring spatial information across scales, Int. J. Remote Sens., 25, 4961–4979, 2004.
Webb, R. S., Rosenzweig, C. E., and Levine, E. R.: Specifying land surface characteristics in general circulation models: Soil profile data set and derived water-holding capacities, Global Biogeochem. Cy., 7, 97–108, 1993.
Wilson, M. F. and Henderson-Sellers, A.: A global archive of land cover and soils data for use in general circulation climate models, J. Climatol., 5, 119–143, 1985.
Wu, L., Wang, A., and Sheng, Y.: Impact of Soil Texture on the Simulation of Land Surface Processes in China, Climatic and Environmental Research, 19, 559–571, https://doi.org/10.3878/j.issn.1006-9585.2013.13055, 2014 (in Chinese).
Wu, T., Song, L., Li, W., Wang, Z., Zhang, H., Xin, X., Zhang, Y., Zhang, L., Li, J., Wu, F., Liu, Y., Zhang, F., Shi, X., Chu, M., Zhang, J., Fang, Y., Wang, F., Lu, Y., Liu, X., Wei, M., Liu, Q., Zhou, W., Dong, M., Zhao, Q., Ji, J., Li, L., and Zhou, M: An overview of BCC climate system model development and application for climate change studies, J. Meteorol. Res., 28, 34–56, 2014.
Wu, X., Lu, G., Wu, Z., He, H., Zhou, J., and Liu, Z.: An Integration Approach for Mapping Field Capacity of China Based on Multi-Source Soil Datasets, Water, 10, 728, https://doi.org/10.3390/w10060728, 2018.
Zhang, W. L., Xu, A. G., Ji, H. J., Zhang, R. L., Lei, Q. L., Zhang, H. Z., Zhao, L. P., and Long, H. Y.: Development of China digital soil map at 1 : 50 000 scale, 19th World Congress of Soil Science, Soil Solutions for a Changing World, 1–6 August 2010, Brisbane, Australia, 2010,
Zhao, H., Zeng, Y., Lv, S., and Su, Z.: Analysis of soil hydraulic and thermal properties for land surface modeling over the Tibetan Plateau, Earth Syst. Sci. Data, 10, 1031–1061, https://doi.org/10.5194/essd-10-1031-2018, 2018.
Zhao, M., Golaz, J.-C., Held, I. M., Guo, H., Balaji, V., Benson, R., Chen, J.-H., Chen, X., Donner, L. J., Dunne, J. P., Dunne, K., Durachta, J., Fan, S.-M., Freidenreich, S. M., Garner, S. T., Ginoux, P., Harris, L. M., Horowitz, L. W., Krasting, J. P., Langenhorst, A. R., Liang, Z., Lin, P., Lin, S.-J., Malyshev, S. L., Mason, E., Milly, P. C. D., Ming, Y., Naik, V., Paulot, F., Paynter, D., Phillipps, P., Radhakrishnan, A., Ramaswamy, V., Robinson, T., Schwarzkopf, D., Seman, C. J., Shevliakova, E., Shen, Z., Shin, H., Silvers, L. G., Wilson, J. R., Winton, M., Wittenberg, A. T., Wyman, B., and Xiang, B.: The GFDL Global Atmosphere and Land Model AM4.0/LM4.0: 2. Model Description, Sensitivity Studies, and Tuning Strategies, J. Adv. Model. Earth Sy., 10, 735–769, https://doi.org/10.1002/2017MS001209, 2018.
Zheng, G., Yang, H., Lei, H., Yang, D., Wang, T., and Qin, Y.: Development of a Physically Based Soil Albedo Parameterization for the Tibetan Plateau, Vadose Zone J., 17, https://doi.org/10.2136/vzj2017.05.0102, 2018.
Zheng, H. and Yang, Z. L.: Effects of soil type datasets on regional terrestrial water cycle simulations under different climatic regimes, J. Geophys. Res.-Atmos., 121, 14387–14402, https://doi.org/10.1002/2016jd025187, 2016.
Zhou, T., Shi, P. J., Jia, G. S., Dai, Y. J., Zhao, X., Shangguan, W., Du, L., Wu, H., and Luo, Y. Q.: Age-dependent forest carbon sink: Estimation via inverse modeling, J. Geophys. Res.-Biogeo., 120, 2473–2492, https://doi.org/10.1002/2015jg002943, 2015.
Zöbler, L.: A world soil file for global climate modeling, NASA Tech. Memo. 87802, NASA, New York, USA, 33 pp., 1986.
- Abstract
- Introduction
- General methodology of deriving soil datasets for ESMs
- Comparison of available global soil datasets
- Soil data usage in ESMs and existing challenges
- Summary and outlook
- Data availability
- Author contributions
- Competing interests
- Acknowledgements
- Financial support
- Review statement
- References
- Abstract
- Introduction
- General methodology of deriving soil datasets for ESMs
- Comparison of available global soil datasets
- Soil data usage in ESMs and existing challenges
- Summary and outlook
- Data availability
- Author contributions
- Competing interests
- Acknowledgements
- Financial support
- Review statement
- References