the Creative Commons Attribution 4.0 License.
the Creative Commons Attribution 4.0 License.
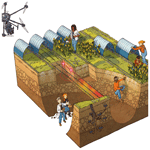
Closing the phenotyping gap with non-invasive belowground field phenotyping
Guillaume Blanchy
Waldo Deroo
Tom De Swaef
Peter Lootens
Paul Quataert
Isabel Roldán-Ruíz
Roelof Versteeg
Sarah Garré
Breeding climate-robust crops is one of the needed pathways for adaptation to the changing climate. To speed up the breeding process, it is important to understand how plants react to extreme weather events such as drought or waterlogging in their production environment, i.e. under field conditions in real soils. Whereas a number of techniques exist for aboveground field phenotyping, simultaneous non-invasive belowground phenotyping remains difficult. In this paper, we present the first data set of the new HYDRAS (HYdrology, Drones and RAinout Shelters) open-access field-phenotyping infrastructure, bringing electrical resistivity tomography, alongside drone imagery and environmental monitoring, to a technological readiness level closer to what breeders and researchers need. This paper investigates whether electrical resistivity tomography (ERT) provides sufficient precision and accuracy to distinguish between belowground plant traits of different genotypes of the same crop species. The proof-of-concept experiment was conducted in 2023, with three distinct soybean genotypes known for their contrasting reactions to drought stress. We illustrate how this new infrastructure addresses the issues of depth resolution, automated data processing, and phenotyping indicator extraction. The work shows that electrical resistivity tomography is ready to complement drone-based field-phenotyping techniques to accomplish whole-plant high-throughput field phenotyping.
- Article
(10974 KB) - Full-text XML
- BibTeX
- EndNote
Alongside actions to mitigate climate change, the agricultural sector needs solutions to adapt to the increased occurrence of weather extremes such as drought or waterlogging. In this sense, breeding climate-robust crops is one of the needed pathways for adaptation to climate change (Snowdon et al., 2020). In a typical breeding programme, the selection of a new variety ready for the market takes more than a decade (Voss-Fels et al., 2019). To speed up this process and to breed climate-robust crops more efficiently, it is important to understand how plants respond to extreme weather events such as drought or waterlogging and to identify which traits should be targeted in selection programmes. Recent advances in phenotyping have resulted in powerful tools to screen plant traits in large collections of plants in different settings and under various conditions.
While methods for evaluation under controlled conditions in growth chambers or greenhouses remain important in plant phenotyping, a weak correlation has often been reported between responses in a controlled environment and those in a production environment (Langstroff et al., 2021). Consequently, in-field evaluation, in combination with remote sensing techniques, is increasingly being deployed (Araus and Cairns, 2014). Nevertheless, few options are currently available to phenotype belowground in-field conditions in undisturbed and living soils. Most techniques for soil–root investigation in the field are invasive and destructive (Das et al., 2015; Trachsel et al., 2010). These techniques cannot monitor a given plant, or plot, throughout its entire growth period. Installation of minirhizotron tubes with cameras offers a less invasive approach (Svane et al., 2019) but delivers only local information on the (partly disturbed) zone surrounding the tubes (Rajurkar et al., 2022; Vamerali et al., 2011). Geophysical imaging techniques allow for the monitoring of a bigger soil volume in a minimally invasive way.
Electrical resistivity tomography (ERT) (also denoted as electrical resistivity imaging (ERI) or direct-current resistivity (DCR)) is a commonly used geophysical technique to inject a current into a pair of electrodes and to measure the voltage in another pair, obtaining the electrical resistance of the bulk soil in the measurement volume. The measurement volume varies with the distance between the four electrodes. This procedure is repeated over many combinations of electrodes along a transect or a grid or even using borehole electrodes. This results in a series of apparent electrical resistivities of different volumes of bulk soil. The data set of measured resistances is then inverted to reconstruct a plausible distribution of electrical resistivity in the subsurface below the transect or grid (i.e. to create an image of the subsurface). Since the inversion problem is ill-posed, the obtained distribution of electrical resistivity should always be considered to be an estimation with which uncertainty is associated. The electrical resistivity of bulk soil is determined by soil variables (e.g. soil moisture, temperature, salinity) and properties (e.g. clay content, porosity) which may change simultaneously. To monitor a variable of interest, such as soil moisture, other variables or properties are typically measured with independent methods so that their effect on resistivity can be predicted and eliminated using calibration relationships. The experimental setup can also keep other variables of interest constant, or one can neglect their influence if the effect is much smaller than the one of the variable of interest. Time lapse ERT allows for the removal of the effect of constant properties of the subsurface since only changes in electrical resistivity over time can be considered. More information on the theoretical basis of ERT, inversion, and its applications can be found in Binley and Slater (2020).
ERT integrates the entire soil volume under a plant, row, or plot and is sensitive to changes in soil moisture. This makes the technique suitable to monitor the impact of crop root systems on soil water depletion, which is related to static or dynamic root system traits (McGrail et al., 2020; Atkinson et al., 2019; Ehosioke et al., 2020). The most important advantage is that ERT does not disturb root system structures, architecture, and functions within the rhizosphere and bulk soil environments, and so the rhizosphere can be monitored as a “holistic phenotype”. This could lead as far as the discovery of new traits to be targeted by breeders and is essential for researchers investigating the functioning of the soil–plant system. ERT has been used before to assess static and dynamics properties of the root zone in the context of agronomic, ecological, and engineering studies. In the agronomic context, Michot et al. (2003) were amongst the first to use ERT to observe root water uptake patterns of maize in the field. In the following years, the potential of ERT to monitor soil water depletion in the root zone was further demonstrated by various authors using a range of crops, soils, and climates (these include, amongst others, Amato et al., 2009; Srayeddin and Doussan, 2009; Cassiani et al., 2012; Garré et al., 2013; Blanchy et al., 2020c). Whalley et al. (2017) were the first to bring the technique explicitly to the breeding context by testing its ability to discriminate between the soil moisture profiles under 13 wheat varieties over 3 years. Amongst the tested techniques, ERT provided the best discrimination among wheat lines. They found inter-genotype differences in the depth of water uptake and in the extent of surface drying, paving the way for ERT as a technique for belowground plant phenotyping, complementing aboveground high-throughput field phenotyping (HTFP).
Despite its clear potential, past studies highlighted a few remaining challenges in using ERT as a fully fledged belowground phenotyping technique. In agronomic applications, ERT is mostly implemented as a surface transect or grid. To maximize the resolution in the root zone, small electrode spacings (0.2–0.5 m) should be adopted. Nevertheless, the resolution and sensitivity of the imaging declines with depth. Obtaining a high spatial resolution throughout the root zone while attaining sufficient depth penetration is a significant challenge (Zhao et al., 2019). The resolution of ERT is typically limited to the decimetre range in the field, especially when targeting (the effects of) roots at greater depths. The effect of small uncertainties in electrode positions quickly inflates when electrode spacings are reduced and when surface electrodes are combined with buried or borehole electrodes (Wilkinson et al., 2008; Ochs et al., 2022). In addition, the choice of electrode configuration significantly influences the quality of ERT data and the sensitivity distribution (Garre et al., 2021). Optimizing electrode layouts and measurement arrays for specific phenotyping objectives (balancing space and time resolution) is therefore key to getting robust and accurate phenotyping data sets (Uhlemann et al., 2018).
Another challenge is that apparent resistivity measurements need to be converted to resistivity distributions by an inversion process if spatially explicit information is required. The classical geophysical inversion is ill-posed, and choosing the most appropriate inversion parameters still requires significant expert knowledge. In addition, the quality of the inversion results is sensitive to several factors, which may sometimes change during the growing season (e.g. changing contact resistances due to drought). Developing robust inversion algorithms with clear descriptions of the used approaches and applied regularization strengths remains important. Improving the transparency and accuracy of the inversion process is necessary to develop standardized processing pipelines and resulting indicators for breeders. Different types of inversion strategies should be explored, such as joint or coupled inversion, machine learning, or other types of hybrid algorithms (Wagner and Uhlemann, 2021). As a geophysical inversion is ill-posed, ERT data inversion results are inevitably associated with uncertainties. Understanding and quantifying these uncertainties (Tso et al., 2021; Linde et al., 2017) and developing strategies to manage and communicate them are important for the reliable interpretation of resistivity distributions and derived phenotyping indicators.
Finally, establishing standardized (meta-)data formats, procedures for ERT measurement for phenotyping, and calibration and data processing is crucial for ensuring consistency, comparability, and interoperability of data across different studies and locations. Currently, typically, very little information about the exact settings of the measurement device, electrode array, and data processing is shared in research publications. Some initiatives, such as REDA (https://geophysics-ubonn.github.io/reda/index.html, last access: 16 January 2025), try to address this but are currently not widely adopted. Well-documented, open-access ERT data sets for agriculture and, in particular, phenotyping are rare (CAGS, https://agrogeophy.github.io/datasets/, last access: 16 January 2025). There are some efforts for unified data models in the phenotyping community (e.g. MIAPPE, https://www.miappe.org/, last access: 16 January 2025), in environmental monitoring (e.g. eLTER, https://zenodo.org/records/6373410, last access: 16 January 2025, ENVRI-FAIR, Wohner et al., 2022), and in geoscience (e.g. ODM2, ODMX, https://odmx.org/, last access: 16 January 2025), but those remain limited to certain sub-communities or projects. In addition, there is no clear, broadly accepted standard for the ERT data storage, processing, and sharing in the framework of belowground phenotyping. This hampers the wide use and application of the technique and/or resulting data sets.
HYDRAS (HYdrology, Drones and RAinout Shelters) is an open-access field-phenotyping infrastructure, located near Ghent in Belgium. It was designed to address these challenges and to bring electrical resistivity tomography, alongside drone imagery and environmental monitoring, to a technological readiness level closer to what breeders and researchers of the soil–plant–atmosphere system need. The objective of this work was to investigate whether electrical resistivity tomography (ERT) provides sufficient precision and accuracy to distinguish between belowground plant traits of different genotypes of the same crop species. We address the issues of depth resolution; automated data processing; phenotyping indicator extraction; and open, interoperable data sets. Using the data of a proof-of-concept (POC) experiment conducted in 2023, we illustrate steps towards addressing these challenges and highlight potential further developments.
The HYDRAS open field-phenotyping infrastructure comprises fields with mobile rainout shelters used to generate precise drought periods and neighbouring control fields. Regular drone flights with RGB and multispectral cameras generate high-throughput phenotyping indicators characterizing the aboveground part of the plant at key phenological stages. Continuous electrical resistivity tomography (ERT) monitoring provides indicators representing the plant below the ground. Measurement methods are calibrated and validated with independent soil and plant sensors. In this work, we present the results of the belowground phenotyping activities during the proof-of-concept (POC) experiment conducted in 2023, with three distinct soybean genotypes known for their contrasting reactions to drought stress: Glycine max (L.) Merr. cv. Lenka, Glycine max (L.) Merr. cv. Hermes, and Glycine max (L.) Merr. cv. Pro-1. The Lenka genotype is known to be more resistant to drought thanks to the slow canopy drought trait (Ye et al., 2019). Pro-1 and Hermes do not have this trait, but the Pro-1 genotype has a more compact habitus that can potentially make it more drought tolerant.
2.1 Site description
HYDRAS is located in Melle, Belgium (50.99281° N, 3.78602° E), on a sandy soil classified as a Eutric Stagnic Glossic Retisol according the WRB (2022) or as Sbc according to the Belgian soil classification system. Each drought and control block consists of three fields undergoing a 3-year crop rotation, with six blocks in total (see Fig. 1). The size of each field is 30 m × 10 m. The drought blocks have an additional parking for the shelters, which is not used for experiments. The year 2023 was the first year of operation of the infrastructure. Two weather stations are present: one underneath a shelter and one in the open air. HYDRAS is equipped with a calibration pit. In this pit, soil moisture, soil temperature, and electrical resistivity sensors monitor the soil status continuously in each horizon (see below for details). This allows us to establish a robust field-specific pedophysical relationship by curve-fitting to the soil moisture–electrical conductivity data from the pit. The pit also provides continuous validation data characterizing the field status. In addition, soil moisture and water potential sensors are installed near the soil surface in the experimental fields to validate the ERT measurements during the growing season.
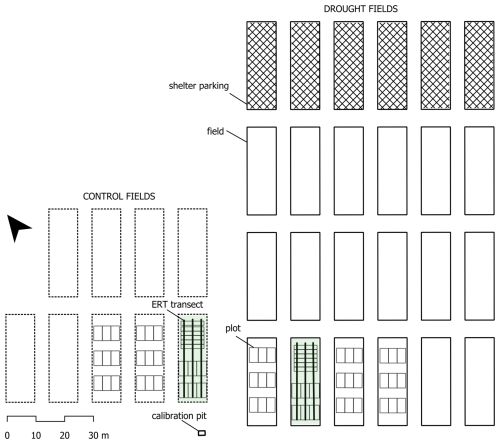
Figure 1Layout of the HYDRAS field-phenotyping infrastructure during the POC2023 experiment. The control and drought field equipped with ERT in 2023 are indicated in shaded green. Black lines indicate the location of an ERT transect constituted by surface electrodes and electrodes buried at 0.5 m depth. The polygons inside the fields represent the plots sown with soybean.
2.2 Data acquisition
2.2.1 Electrical resistivity tomography
In HYDRAS, electrical resistivity tomography (ERT) is used to identify differences in the root system functioning of a panel of plant genotypes by monitoring the impact of each genotype on soil water depletion patterns. We start the growing season with well-watered soil all along the ERT transect. The electrical resistivity of the soil increases (or the conductivity decreases) where water disappears through the process of root water uptake, amongst others. In the electrical resistivity profile, this appears as a series of root water uptake bulbs or as a drying front along the transect if the plant rows are close to each other. Upon an irrigation event, new water infiltrates into the profile, affecting the drying patterns. The root water uptake is therefore most visible in the time lapse ERT data over a dry period. The effect of root-induced soil moisture changes is generally much larger than other, direct effects of the growing root biomass (Ehosioke et al., 2020).
ERT quantifies the bulk electrical resistivity of the soil between a series of electrodes. The bulk electrical resistivity corresponds to the combined resistivity of soil particles, pore water, and air. A basic measurement system consists of four electrodes (A, B, M, N), often referred to as a “quadrupole”. A direct current of known intensity (I) is sent between electrodes A and B, while a potential difference (V) is measured between electrodes M and N. The resistivity meter switches the polarity of the current using a square wave to avoid polarization of the injection electrodes. It then computes a transfer resistance (R, Ω) for each combination of four electrodes based on Ohm's law: R = V I. Based on the distances between the four electrodes, a geometric factor K can be computed to transform this transfer resistance into a bulk apparent electrical resistivity (ρa, Ω m): ρa . This resistivity is “apparent” because it represents the resistivity of a homogeneous isotropic ground with the same transfer resistance. Electrical conductivity (EC, mS m−1) is the inverse of the resistivity.
For the POC experiment, we sowed the three soybean genotypes on 24 May 2023 (for more information on the timing of different agronomic and experimental events, see Appendix A). We then equipped one field in the control block and one in the drought block with three ERT transects crossing the soybean genotype plots (the shaded fields in Fig. 1). Each transect was 26.4 m long (excluding the borders of the field) and consisted of a surface cable and a cable buried at 0.5 m depth. The buried cable increases the resolution in the bottom part of the root zone. The surface electrode spacing was 0.3 m, whereas the electrode spacing along the buried cable was 0.6 m (see Fig. 2a and b). This resulted in a total of 426 electrodes per field connected to the ERT base unit. The surface electrodes had a diameter of 0.01 m and a length of 0.1 m and were connected to the multicore cables. The cable take-outs of the buried cables served directly as electrodes. In the HYDRAS installation, the buried cables stay in place permanently since they are installed under the plough depth. The surface cables are put in place after sowing, stay there for the entire growth period, and are removed at harvest.
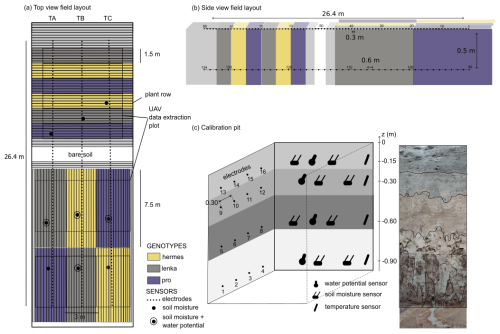
Figure 2(a) Top-view scheme of the ERT-equipped fields in HYDRAS during the POC2023 experiment. Colours represent genotypes. Small dots represent electrodes of the three ERT transects: TA, TB, and TC. (b) Side-view scheme of the ERT-equipped fields, highlighting electrode numbering. (c) Calibration pit with sensors, electrode locations, and soil profiles.
We performed the ERT measurements with a single-channel, multi-electrode autonomous resistivity system developed and sold by Subsurface Insights (Hanover, NH, United States) and Metinco (Wapenveld, The Netherlands). These systems can support thousands of electrodes. The two systems installed in HYDRAS have 420 electrodes each. These systems are specifically designed for unattended long-term monitoring efforts and, apart from needing external power, are fully self-contained. An internal single-board computer controls the data acquisition and communicates with a cloud server for data storage and further processing. Users can control acquisition and can access collected data through a web interface and a software API. The system can inject currents of up to 120 mA or, alternatively, can apply a fixed transmitter (Tx, between electrodes A and B) voltage between 0 and 150 V. In the POC experiment, we applied a Tx voltage of 20 V. This Tx voltage was found to be adequate to have a good signal-to-noise ratio for our electrode spacing and soil type. The measurement sequence for the POC experiment contained dipole–dipole and gradient quadrupoles on the same line and between surface and buried lines (full sequence available in the Appendix). We collected reciprocals of all quadrupoles for error assessment. One ERT measurement sequence of 2118 quadrupoles took about 1.5 h and was repeated continuously from 21 June 2023 (just after sowing) to 30 September 2023 (just before harvest). There is an obvious trade-off between acquisition (slower for a single-channel device than for a multi-channel device) and instrument price (cheaper for a single-channel device than for a multi-channel devices). The cheaper instrument price of the SSI single-channel device allows for multiple devices in the field and for simultaneous data acquisition from multiple fields.
As Fig. 2a shows, we adopted two types of plant row orientations in this POC experiment (along and perpendicular to the ERT transects) to assess whether this orientation influences the measurements. The orientation did not affect the ERT-derived indicators considerably, confirming that the 2.5 D assumption (homogeneity in the Y direction) holds for both row orientations in this trial. Analysis of the yield data of the trial revealed that the yield in the plots with rows perpendicular to the ERT transects was systematically lower than in the plots with rows established along the ERT transects. This was most likely due to more edge effects and more of an impact by tractor wheel tracks (data not shown).
2.2.2 Environmental monitoring
Four soil water potential sensors (TEROS 21, METER Group) and nine soil moisture sensors (CS616, Campbell Scientific) were installed vertically in the soil surface. Figure 2a shows the location of those sensors in the field. They are all, at maximum, two plant rows away from the ERT transects. The site has a calibration pit equipped with soil moisture, soil water potential, and soil temperature sensors (T107, Campbell Scientific) and four electrodes in each of the four soil horizons up to 1 m depth (see Fig. 2c). Sensors and electrical resistivity measurements are continuously logged throughout the year to establish the field-scale soil hydraulic functions, thermal characteristics, and pedophysical calibration functions. Figure 3b shows significant variability near the soil surface, but at 0.6 and 0.9 m depth, the soil moisture remained rather constant throughout the season. As the soil pit was bare and because the fields were sown with soybean, the evapotranspiration was higher in the fields than in the pit, which resulted in lower soil moisture contents in the field than in the pit. The pit should therefore not be used to assess the field status of soil variables but rather as a field-scale source of data on soil hydraulic, thermal, and pedophysical functions.
Meteorological variables (solar radiation, precipitation, wind direction, wind speed, air temperature, water vapour pressure, atmospheric pressure) were measured using a ATMOS 41 weather station (METER Group). One station was mounted in open air, and the other was mounted under the rainout shelter when drought was applied to assess the impact of the shelter on the microclimate.
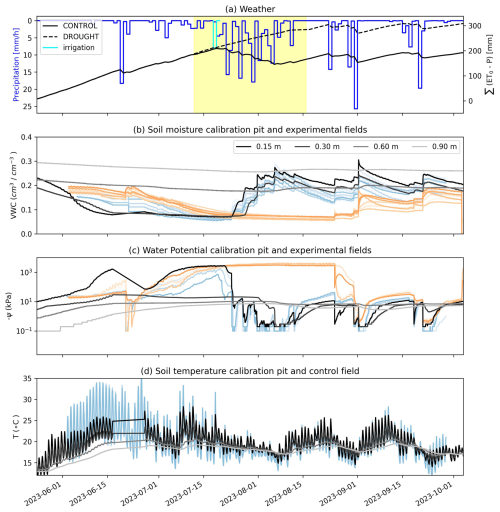
Figure 3Overview of environmental conditions in the calibration pit, drought, and control fields. (a) Precipitation and cumulative precipitation deficit () under drought and control treatments. The cyan irrigation event was only applied on the control plots. The yellow band indicates the length of the applied drought treatment with the rainout shelter. (b) Soil moisture in the calibration pit at four depths (grey hues) and in the drought (orange hues) and control fields (blue hues) at 0.15 m depth (different hues indicate different locations in the field). (c) Soil water potential in the calibration pit at four depths (grey hues) and in the drought (0–0.10 m depth) and control fields (vertical installation depth: 0–0.10 m). (d) Soil temperature in the calibration pit at four depths (grey hues) and in the control treatment (vertical installation depth: 0–0.10 m).
2.2.3 Aboveground phenotyping using drone imaging
Drone-based high-throughput field phenotyping was executed in analogy with Borra-Serrano et al. (2020) and Pranga et al. (2021). In total, 14 flights were performed using a UAV DJI Matrice 600 Pro (DJI, Shenzhen, China) equipped with an RGB camera (a6400, Sony Corporation, Tokyo, Japan), a 10-band multispectral camera (MX dual-camera system, MicaSense, Seattle, USA), and a thermal camera (WIRIS Pro, Workswell, Czech Republic). For this article, only multispectral data were used. Flight speed and flight altitude were 2.7 m s−1 and 30 m for the multispectral sensor. This resulted in a ground sampling distance of 2.0 cm per pixel. All flights were conducted within 2 h of solar noon and with 80 %–80 % side and front overlap. Multispectral images were processed, and geo-referenced orthophotos were created using PIX4Dmapper 4.7.5 (Pix4D S.A., Switzerland). Several plant indices were calculated, but, here, we only show the soil-adjusted vegetation index (SAVI). This index minimizes the influence of soil brightness using a correction factor. NIR represents the pixel values from the near-infrared band, Red represents the pixel values from the near-red band, and L represents the amount of green vegetation cover.
Generally, in areas with no green vegetation cover, L=1; in areas of moderate green vegetative cover, L=0.5; and in areas with very high vegetation cover, L=0 (which is equivalent to the normalized difference vegetation index (NDVI) method). This index outputs values between −1.0 and 1.0.
2.3 Processing and quality control
Figure 4 shows an overview of the data processing workflow. The workflow is divided into four different steps: preprocessing, quality assessment, inversion, and computing indicators. More explanations of some of these steps are given below.
2.3.1 Quality assessment
From the field ERT unit, the data are continuously uploaded to a server, where a basic quality assessment at the level of the quadrupoles is executed. Users are alerted when the server does not receive data or when the raw data do not reach defined thresholds (e.g. very low injection current). Figure 5 shows the evolution of the raw metrics for each measured quadrupole over the entire monitoring period: current (I), measured voltage (Vmn), contact resistance (cR, estimated resistance between the electrode and the soil), apparent resistivity (ρa), standard deviation from stacking (SD), and relative difference in apparent resistivity to the reference background image () from the first survey (ρa0). cR is calculated from the same measurement data that are used for resistivity. This is given for an electrode pair by dividing the voltage applied across the electrodes by the current and then dividing by 2 to determine approximate resistance per electrode. This overview enables us to spot any irregularities in the system or sudden environmental changes, such as heavy rainfall after a dry period (e.g. end of August), allowing for real-time alerting during the monitoring period.
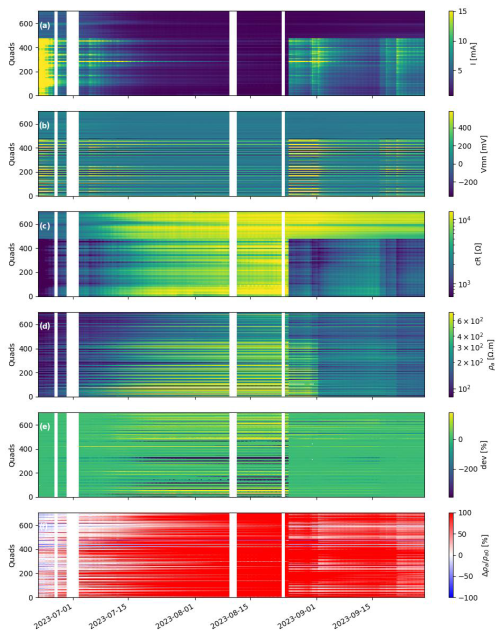
Figure 5Evolution of raw metrics at quadrupole level (Quads) measured by the ERT system, featuring injected current (I), measured voltage (Vmn), contact resistances (cR), apparent resistivity (ρa), standard stacking deviation (SD), and relative change in apparent resistivity from the first survey (). Vertical white bands show no-data time. The data are shown here for transect A of the ERT field to which the drought treatment was applied (see Fig. 2 for transect location within the field).
Quadrupoles with electrodes often associated with high contact resistances (>50 k Ω) were removed from the data sets. Negative apparent resistivities, as well as quadrupoles with large (> 50 %) standard deviations, were also removed before further processing. In addition, quadrupoles with injection electrode pairs buried and voltage electrode pairs at the surface (or the opposite) were removed from the data set. After preliminary inspection of the raw data, we noticed that these quadrupoles lead to significant artefacts around the electrodes, possibly caused by small inaccuracies in the position of the surface cable with respect to the buried cable. From synthetic studies, we found that 2 cm misplacement of electrodes (longitudinal or lateral to the transect) can reproduce the artefacts we observed if all data are used to invert the data set (data not shown). Given the relatively small electrode spacing of the survey and the difficulty in positioning the surface electrode right on top of the buried ones with centimetric precision, we decided to remove these quadrupoles from the inversion. More background on the effect of electrode misplacement on ERT accuracy can be found in, amongst others, Wilkinson et al. (2010), Uhlemann et al. (2018), and Oldenborger et al. (2005).
The pipeline computes reciprocal errors, and Fig. 6 shows the evolution of their distribution as a function of time for each transect. The reciprocal errors tend to increase as the soil dries out because this also increases the contact resistance of the electrodes at the surface (see Fig. 5). In this study, the reciprocal errors were not used for filtering as most outliers were already removed by the previous filters. However, filtering on reciprocal error might be needed for a noisier survey. We fitted a power-law error model for each survey on a transect using the binned reciprocal errors (Koestel et al., 2008). This approach ensures a sufficient number of data points to obtain a robust error model while allowing the error model to vary throughout the season. The implementation of the data processing can be found in the accompanying Jupyter notebook.
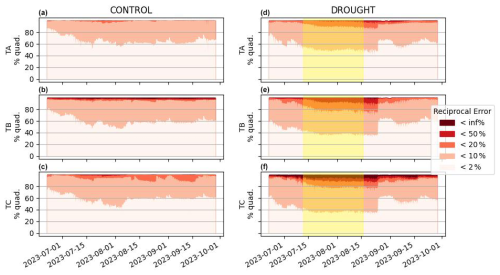
Figure 6Evolution of the distribution of reciprocal errors () during the growing season of the POC23 experiment for all ERT transects (TA, TB, and TC) in the control (subplots a, b, c) and drought (subplots d, e, f) conditions. Reciprocal errors are distributed in classes for easier visualization. The percentage of quadrupoles in each class is indicated on the vertical axis.
2.3.2 Data inversion
The ERT data were processed using the ResIPy software v3.5.1 (Blanchy et al., 2020a) that makes use of the Occam-based R2 inversion code (Binley, 2015). Each survey was inverted and compared to the first recorded survey following the difference inversion method (LaBrecque and Yang, 2001) (reg_mode =2 in ResIPy). The difference inversion consists of first inverting a reference survey (in our case, the first survey collected on 21 June 2023) and then computing, for each subsequent survey, the difference with respect to this reference survey. For a given survey, the response (i.e. transfer resistances for each quadrupole) from the inverted model of the reference survey is computed and added to the difference between the measured transfer resistances of the reference and the given survey. This new data set of transfer resistances is then inverted. This approach highlights differences between survey dates and suppresses the systematic errors that might arise due to electrode placement. It is well suited when electrodes are kept in place between surveys, as was the case in this study. The inversion procedure was done using a triangular mesh. The objective function to be minimized consisted of a data misfit (weighted by the errors computed from the fitted error model) and a model misfit (smooth L2 regularization). Inversions typically converged within five iterations and reached a final weighted root-mean-square error close to 1. More details on the inversion can be found in Binley and Slater (2020). Appendix C shows several of these inversion quality indicators as a function of time for all transects.
2.3.3 Temperature correction and conversion to soil moisture
After inversion, resistivity data were temperature-corrected and converted to soil moisture using a site-specific pedophysical relationship, established using multi-sensor data in the HYDRAS calibration pit (see Fig. 3d). The resistivity data of this study are corrected for temperature using the following relationship (Ma et al., 2010; Campbell et al., 1949): EC, with α=0.02. The soil temperature profile during the measurements was assumed to be equal to the temperature measured in the different soil horizons of the calibration pit, which is a simplification of reality.
Figure 7 shows the sensor data from the calibration pit for the four soil horizons. As the deepest layers never reached dry conditions, the in-field pedophysical relationship for those horizons cannot be established in the dry range at the moment. Further monitoring in coming years will improve the number of data in the dryer range. For illustration, we fit the simplified Waxman–Smits model to the data of each soil horizon. The data largely follow the expected trend but also exhibit significant scatter. Since we want to investigate the pedophysical relationship further in future experiments, we have not used it in the current article to compute indicators based on estimated soil moisture.
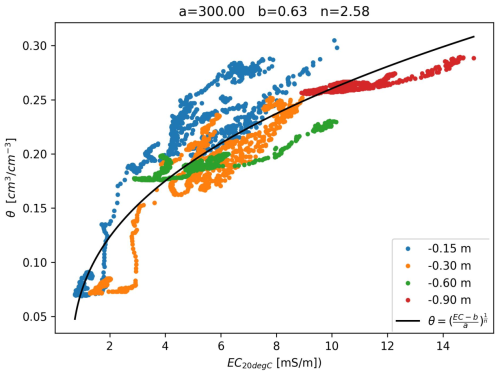
Figure 7Evolution of volumetric soil moisture θ with respect to the bulk temperature-corrected electrical conductivity (ECb,20) based on the data collected at the different depths in the control pit. For illustration, the simplified Waxman–Smits model was fit to the data of each soil horizon, as shown in Garré et al. (2013) (black line on top of the data points).
2.3.4 Data dissemination: from geophysicists to plant scientists and breeders
While profiles of inverted electrical conductivity are certainly interesting to geophysicists, they have less meaning for plant scientists or breeders who use the HYDRAS open-access infrastructure. As such, we translated the geophysical results into phenotyping indicators that are more interpretable for crop breeders and researchers. Table 1 shows four of those indicators with their potential. We computed indicators along the inverted profile and the profile of difference compared to the background. Figure 8 illustrates how these indicators are computed in EC or Δ EC profiles. The shape of the inverted EC profile often takes the form of a sigmoid (Shanahan et al., 2015): EC = . The parameters of the sigmoid summarize information about the shape of the soil moisture profile. Fitting parameter a defines an offset from zero soil moisture, and b controls the width of the sigmoid (magnitude of the drying), d controls the steepness of the curve, and c defines the depth of inflection in the soil profile. Parameters c and d influence the drying depth. The integration of the negative difference in EC with depth (green-shaded zone in Fig. 8b) gives an estimation of the extent of the water depletion caused primarily by root water uptake. Similarly, the 10th percentile of the depths at which a negative difference larger than a threshold of 20 % change occurs (vertical dashed line in Fig. 8b) is an indicator for the advancement of the drying depth in the soil profile (horizontal orange line). The pedophysical relationship shown above allows us to translate EC to soil moisture (not done here).
Table 1Description of the proposed phenotyping indicators derived from the average electrical conductivity profiles.
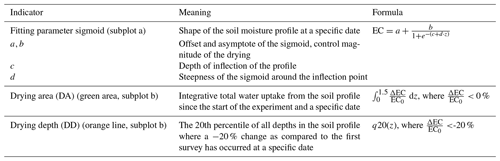
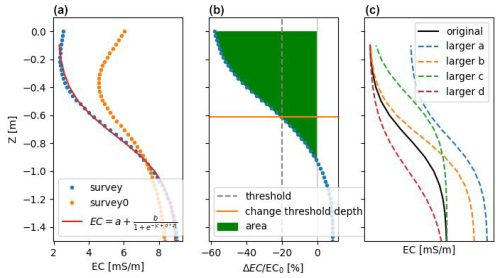
Figure 8Example of indicators computed on either (a) electrical conductivity (EC) profile or (b) its relative difference () from the reference survey (survey0). Subplot (c) shows how the sigmoid function changes with respect to an increase in each of its parameters.
To enable users to interact with the data set and to explore and compare profiles in space, depth, and time, all processed data from the ERT pipeline were summarized into an HTML report with interactive Bokeh (https://docs.bokeh.org/en/latest/#, v3.4.0, last access: 25 March 2024) figures (available in the GitLab repository).
3.1 Apparent resistivities
The first question of the POC experiment was whether the electrical resistivity measurements are sensitive enough to detect subtle differences in water depletion patterns and strategies between contrasting genotypes of the same crop species. Figure 9 shows the pseudo-section of the ERT transect B in the control field. Raw apparent resistivities reflect consistent patterns related to different root water uptake patterns of the three investigated genotypes in different plots along the transect. For instance, Hermes and Pro_1 take up more water earlier in the growing season than Lenka. We observe similar trends in the part of the transect with plant rows that are longitudinal to the ERT transect as in the part with rows crossing the transect.
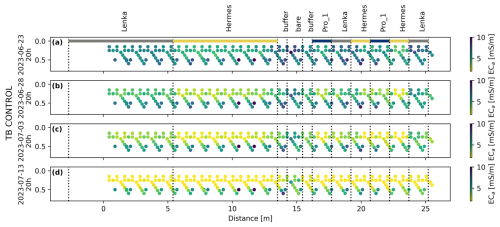
Figure 9Evolution of pseudo-section for transect B in the control field. Note that the depth on the vertical axis is not the actual depth of the reading but rather an estimation of the depth above which most of the signal originates (a pseudo-depth) based on the electrode location and measurement geometry.
To further detect the subtle differences between genotypes, we take advantage of the statistical design of the study and look at the apparent electrical conductivity distributions. In Fig. 10, the impact of the drought treatment is clearly visible in the apparent conductivity distributions. This confirms the capability of ERT to monitor the dynamic impact of both treatment and genotype on bulk soil electrical properties. This means that, even without further inversion or other data processing, a statistical test can be executed to discriminate between genotypes in terms of the impact of drought on belowground behaviour. Figure 10 shows the distribution of the apparent conductivity values of each treatment–genotype combination. We performed an ANOVA (analysis of variance) with the statsmodels Python package v0.14.0 (Seabold and Perktold, 2010) considering two factors: genotype (Hermes, Lenka, Pro-1) and treatment (drought, control). The effect of drought is significant (p value < 0.05) for shallow pseudo-depths at all four time points and for the deepest pseudo-depth only in mid-July. The genotypes show significant differences for shallow pseudo-depths but not for the deeper depths. A root sampling campaign, just after harvest, has shown that there are few roots present at depths below −0.5 m in any of the treatment–genotype combinations, with Pro-1 showing the most roots in the deepest layer, especially under drought conditions (data not shown). This might explain why we did not find a significant difference in apparent electrical conductivity below that depth.
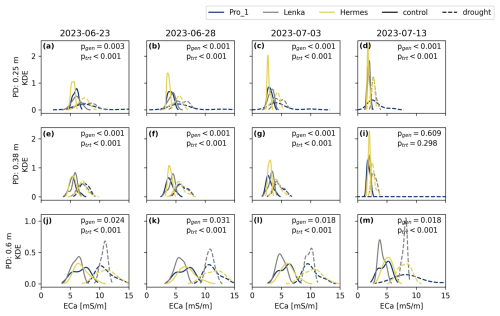
Figure 10Kernel density function of all apparent conductivities for three different pseudo-depths (PDs) along with ANOVA p values for the genotype factor (pgen) and for the drought or control factor (ptrt). All p values are rounded to three decimal places. The interaction term was not significant (> 0.05) for all cases. The subplots at different depths do not share the same vertical axis.
Apparent conductivity data do not disclose information about specific depths of water depletion (but rather of pseudo-depths). For example, large drying or wetting at the surface will decrease or increase the apparent conductivity in the surface but also, to a lesser extent, for quadrupoles with deeper measurement volumes. This makes it difficult to relate the data to plant traits such as rooting depth, root density, or depths of water uptake. To obtain depth-explicit information, a data inversion or other advanced data processing such as coupled modelling and/or machine learning is necessary. In addition, it is not possible to use the pedophysical relationships from the control pit to convert apparent EC to depth-specific soil water content. Indeed, the apparent resistivities are depth-weighted integrative measurements, while soil water content from sensors is depth-specific. Only inverting the apparent resistivities will enable us to obtain depth-specific EC values which can then be converted to soil moisture with a pedophysical relationship.
3.2 Inverted transects
Figure 11 shows the inverted transect B (control) at three moments in June during a period with little rain. Differences in soil water depletion between genotypes are apparent. For instance, Lenka took up less water than Pro-1 and Hermes. The figure also illustrates how the drying front tends to increase with time. Water depletion is mainly caused by the crop as the bare plot in the middle does not show the deep drying pattern.
3.3 Profile evolution
From the inverted section, we extracted average profiles for each plot and then merged per genotype by taking the average profile of all plots (see Fig. 12). By subtracting the average background profile from this, we can depict the percentage change occurring over the profile as compared to the background. Drying under the control is faster during the early natural drought but is more limited in time than for the drought treatment in which an artificial drought was generated using the rainout shelter. After the rainfall events of July, the control field becomes wetter than at the start (blue band starting in August, Fig. 12), and only superficial drying was observed after. From the colour gradient, differences between genotypes remain difficult to see, which is why indicators were derived.
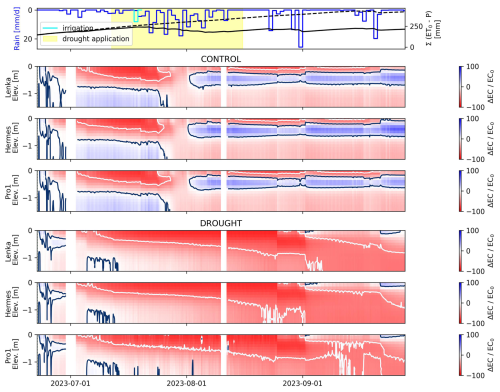
Figure 12Evolution of profiles of inverted, temperature-corrected electrical conductivity (EC) for the three genotypes under both treatments (control and drought) during the growth season. The rainfall deficit is shown in subplot (a) for both the control (plain black line) and drought (dashed black line) fields. The period of the drought application is indicated by the yellow-shaded area. Missing data are indicated by white bars at that date. The white line shows a −50 % change, while the dark-blue line shows a 0 % change.
3.4 Indicators
Figure 13c–f show the evolution of four selected indicators computed from the inverted electrical conductivity profile and the profile of change in conductivity with respect to the background for different genotypes. We present the indicators alongside meteorological conditions (Fig. 13a) and crop development as represented by the UAV-based phenotyping SAVI (Fig. 13b). The indicators differ clearly between the control and the drought treatments. From the end of July onwards, genotypes submitted to the drought treatment exhibit larger drying patterns than in the control. This is apparent from the drying area (DA) (see Table 1 for definitions of indicators), which resides for a much longer period in a zone of great water depletion (large negative value) in the drought conditions than in the control, and this is the case for all genotypes. This is because, in the control, rainfall replenishes the soil moisture profile. The effect of crop water uptake is therefore partially undone. The DA even becomes positive at some moments in time, which means that the soil was wetter then than at the start of the experiment. The difference between treatments decreases from September onwards. Since the shelters were removed, both treatments were receiving rain again, and the crop reached physiological maturity. Where DA is a robust indicator which is smooth over time because it represents an integration of the whole profile, the other indicators are more noisy. The drying depth (DD), for example, represents one specific point in the profile intersecting with the 20 % threshold, which is much more sensitive to slight changes in the profile. We performed ANOVA tests on all time steps of all four indicators and indicated at which time during the year the genotype effect and/or the treatment effect is significant using grey dots at the top and bottom edges of the subplots. Significant differences between genotypes (dark grey) are mostly observed in the beginning of the growing season, which correlates with the pseudo-sections shown in Fig. 9. Just like with vegetation indices, curves could be fitted to the time evolution of these belowground indicators to assess rates of drying or similar properties.
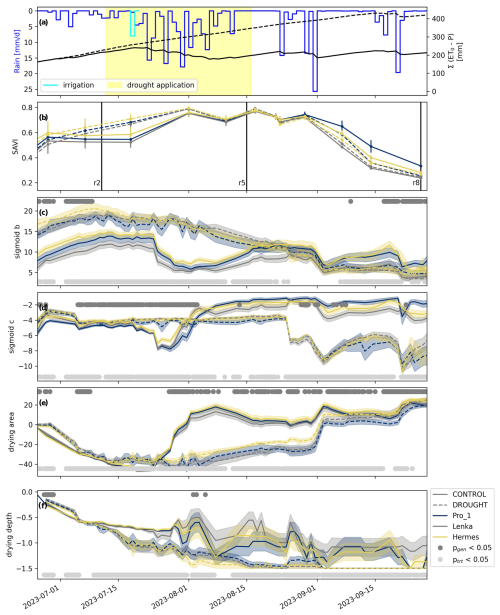
Figure 13Example indicators and their evolution for the three genotypes as a function of the treatment applied (plain line: control, dashed line: drought). (a) Rainfall and precipitation deficit, (b) SAVI (soil-adjusted vegetation index) and important growth stages (reproductive stages r2, r5, and r8), (c)–(d) parameters b and c of the sigmoid fitted to the electrical conductivity profile, (d) drying-area indicator, and (f) drying-depth indicator. For the subplots with indicators (c)–(f), an ANOVA was performed. The grey and light-grey dots show when the p value for the genotype factor and the drought or control factor was below 0.05, respectively.
In Fig. 14, we zoom in on the genotype differences in our four selected indicators at two specific moments in the crop growth: flowering (r2), just before the drought application, and seed filling (r5), just after the drought application. The control is shown in blue, and the drought is shown in orange. In sigmoid c, we see the clearest genotype effect on 11 July. This parameter reflects the inflection point of the sigmoid, which is therefore related to the drying depth.
The proof-of-concept experiment with the HYDRAS infrastructure in 2023 established a data set at a high spatial and temporal resolution. This data set characterized differences in belowground behaviour between control and drought treatments and soybean genotypes under realistic field conditions in a real, living soil. We have shown that ERT is not only capable of detecting differences between crops or treatments, as previously done in the literature, but also has sufficient precision to distinguish between genotypes of the same crop. We highlighted the potential to automatically derive phenotyping indicators related to dynamic belowground plant traits. To our knowledge, the only permanent infrastructure which is capable of monitoring water depletion and root system activity at the same spatio-temporal resolution is the sEIT installation at the Selhausen minirhizotron facility in Selhausen, Germany (Weigand et al., 2022). The sEIT system there consisted of 40 electrodes (0.25 m electrode spacing) installed across three agricultural test plots (each of 3.75 m width). It does not have rainout shelters but did allow the testing and validation of many of the available techniques over several years of operation, yielding an impressive open subsoil data set (Lärm et al., 2023). In that installation, both electrical resistivity and chargeability properties are measured, which is a meaningful addition to the information which can be derived from resistivity. Chargeability was shown to be more directly linked to root biomass, which opens up new possibilities (Weigand and Kemna, 2017). However, accurately measuring these properties with sufficient data remains challenging. With HYDRAS, we take belowground field phenotyping one step further with the fully automated pipeline, derived phenotyping indicators and a field setup for breeding trials including rainout shelters.
Weigand et al. (2022) highlighted the need to further investigate innovative ways to assess uncertainties without compromising measurement time and, more importantly, ways to incorporate that information into the data processing and interpretation. This is also the case for the current data set, which exhibits error levels which change over time. We do take them into account in the inversion, letting the error model vary over time, but we did not yet assess how this affects the precision of our derived indicators. This would be an interesting topic for future research. Nevertheless, the automated, online monitoring also directly allows the user to spot and resolve inevitable field issues instantaneously. The SSI system provides email alerts for the operators using basic and more advanced warning thresholds based on system status (battery, temperature, connectivity) and measurement metrics (contact resistance, plausible values, etc.). This makes it possible to minimize error sources and data gaps.
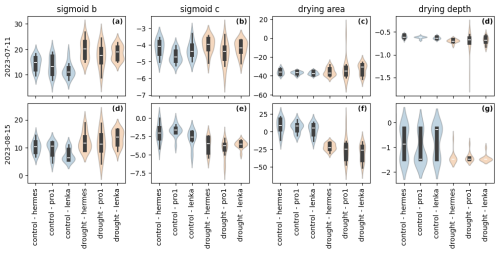
Figure 14Violin plots showing the distribution of the indicators calculated from the electrical conductivity profiles for the three genotypes in control and drought conditions.
The HYDRAS ERT setup has the advantage of having both a surface and a buried cable to enhance the sensitivity and resolution in the root zone. Based on this configuration, quadrupoles along the surface line, along the buried line, and between the surface and buried line were added. However, the combination of centimetre inaccuracies in the placement of the belowground cable with small electrode spacings resulted in inversion difficulties. Quadrupoles with injection electrodes in the surface line and potential electrodes in the buried line (or inversely) led to inversion artefacts close to the electrodes when inverted. We tested the approach of Wilkinson et al. (2015), including electrode location as part of the inversion, but this resulted in too many degrees of freedom in our case. However, simulating the data with slight electrode displacements (longitudinal or lateral) resulted in similar artefacts, which confirms the hypothesis. Further investigation is necessary to fully exploit the potential of the subsurface cable without compromising the inversion results.
The HYDRAS POC2023 data set is not only one of the rare freely available data sets resulting in defined belowground phenotyping indicators which can be related to crop traits but also the first to develop the belowground phenotyping data acquisition and processing pipeline in a fully automated way at field scale. This resulted in a standardized, reproducible, and high-quality data set and associated processing scripts. Although several attempts have been made to make geophysical data sets more FAIR (Findable, Accessible, Interoperable and Reproducible), the agrogeophysical community is far from reaching a community data model or reporting standard. Initiatives have been launched, such as CAGS (https://agrogeophy.github.io/catalog/, last access: 16 January 2025), but often become inactive or phase out after project funding stops. Others, such as the REDA package (https://geophysics-ubonn.github.io/reda/, last access: 16 January 2025), remain but are not widely adopted. In addition, these existing initiatives do not yet seek much compatibility with data standards from the research communities which seek to use the data, such as the phenotyping or precision agriculture communities. The POC2023 and following open data sets from HYDRAS seek to bridge that gap and open up the discussion on the specific needs of users in distinct use cases. For the HYDRAS pipeline, we explored several data models from different scientific communities and came up with a data model proposal largely compatible with the eLTER and the MIAPPE standards.
The infrastructure is not only a crucial asset for the phenotyping and breeding community; 2-D and 3-D, high-resolution, automated monitoring of water flow, solute, and heat transport processes in the undisturbed soil–plant–atmosphere continuum under agricultural land use provides several exciting opportunities, especially since the mobile shelters provide a crucial control on the top boundary condition of the soil. One of the opportunities is improving the understanding of how soil health and its management drive plant performance in agroecosystems. As Carminati and Javaux (2020) and Abdalla et al. (2021) have highlighted, soil – and, more particularly, the rhizosphere – is a major driver of the plants response to drought. However, studying this in field conditions remains challenging. The HYDRAS infrastructure, complemented with ground-truthing data of small-scale processes in the rhizosphere or at the level of plant organs, could help better understand how agricultural practices can optimize the soil–plant interactions. Without aspiring to be exhaustive, potential fields of research tackled in this infrastructure could be the investigation of the (preferential) flow and transport of agro-chemicals, the impact of agricultural management practices on soil health in its various dimensions and on the water use efficiency of crops, the effects of irrigation with different types of water sources on soil salinization, and so on. In addition, this infrastructure enables us to further explore the drivers and multiscale nature of the pedophysical relationship and the uncertainties associated with it. Taking into account the heterogeneities in the pedophysical relationships can improve our ability to detect differences between genotypes (e.g. Blanchy et al., 2020b). A full analysis of the uncertainties associated with the pedophysics is out of the scope of this paper but will be actively investigated in future work. In short, this infrastructure provides new and exciting opportunities for the broad soil science community.
The HYDRAS POC2023 experiment has shown that ERT monitoring at a high spatio-temporal resolution offers unprecedented capabilities for reproducible and accurate belowground field phenotyping. The raw apparent conductivity data clearly show differences between treatments (drought or control) and soybean genotypes, highlighting the information content in the data. Inversion allows us to further fine-tune the information in relation to depth-specific data, which is then used to develop phenotyping indicators related to specific plant traits of interest. Further improvement is desirable to quantify the uncertainties added in each step and to show how these propagate through the entire acquisition and processing pipeline to the final indicators since this influences the interpretation of the data and the power of statistical tests. Various approaches are present in the literature, including Bayesian inversion techniques, joint inversion, and coupled inversion using soil–plant models, which can now be tested based on the big phenotyping data set.
The sequence of quadrupoles used is composed of a “plot sequence” that is moved along the surface and a buried line of a transect. The plot sequence includes 12 surface electrodes spaced 0.3 m apart (electrodes 1 to 12) and 6 buried electrodes spaced 0.6 m apart (electrodes 13 to 18). The plot sequence contains one injection in the surface electrodes, with potential readings between the injection dipole (gradient), outside on the surface, and in the buried lines (dipole–dipole). Another injection is done with a buried pair of electrodes, and similar potentials are collected. The plot sequence is repeated at every fourth surface electrode (two buried electrodes) along the transects to form the final sequence used. This measurement sequence was chosen as a compromise between the spatial and temporal resolution. The sequence includes all reciprocal quadrupoles.
Table B1Plot sequence repeated along each transect. A and B are injection electrodes, and M and N are potential electrodes used to measure voltage. Electrodes 1 to 12 are surface electrodes with 0.3 m spacing. Electrodes 13 to 16 are buried electrodes with 0.6 m spacing. Electrode 1 is on top of electrode 13. K is the geometric factor computed.
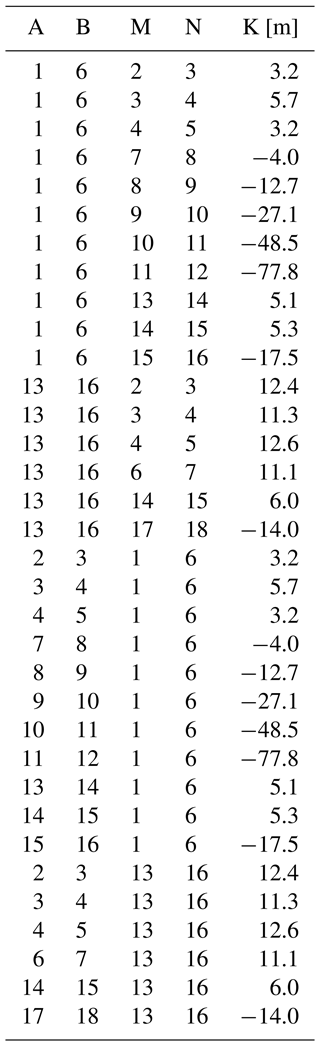
The notebooks and data used to generate the figures in this paper can be found at https://zenodo.org/records/14673647 (Blanchy, G. and Garré, 2025). The data set of POC2023 can be found here: https://doi.org/10.5281/zenodo.14175354 (De Swaef et al., 2024).
Conceptualization: GB, SG, WD, TDS. Formal analysis: GB, SG. Data curation: GB, SG, WD. Writing (original draft): SG, GB. Writing (review and editing): all authors. Project administration: SG, IRR, PL.
The contact author has declared that none of the authors has any competing interests.
Publisher’s note: Copernicus Publications remains neutral with regard to jurisdictional claims made in the text, published maps, institutional affiliations, or any other geographical representation in this paper. While Copernicus Publications makes every effort to include appropriate place names, the final responsibility lies with the authors.
This article is part of the special issue “Agrogeophysics: illuminating soil's hidden dimensions”. It is not associated with a conference.
The HYDRAS open-access infrastructure is a joint effort. We would like to thank colleague Filip De Brouwer and the entire O&I field team of ILVO for the planning and execution of all the necessary field operations, as well as the aboveground high-throughput field phenotyping using UAVs equipped with different sensors. Thanks are given to Jonas Aper from Protealis, who provided the Pro-1 seeds. We also thank FWO for funding this research infrastructure through their “International Research Infrastructure (IRI) call” under the project Emphasis-Belgium: phenotyping the future crops (I002121N) and the Flemish government for their additional support under the project FutureAdapt (project no. VV028). We thank the reviewer Luca Peruzzo and an anonymous reviewer for their feedback and suggestions on the article.
This research has been supported by the Fonds Wetenschappelijk Onderzoek (grant no. I002121N). Guillaume Blanchy is a research fellow of the Fonds de la Recherche Scientifique – FNRS (grant no. CR: 1.B.044.22F).
This paper was edited by Yuxin Wu and reviewed by Luca Peruzzo and one anonymous referee.
Abdalla, M., Ahmed, M. A., Cai, G., Wankmüller, F., Schwartz, N., Litig, O., Javaux, M., and Carminati, A.: Stomatal closure during water deficit is controlled by below-ground hydraulics, Ann. Botany, 129, 161–170, https://doi.org/10.1093/aob/mcab141, 2021. a
Amato, M., Bitella, G., Rossi, R., Gómez, J. A., Lovelli, S., and Gomes, J. J. F.: Multi-electrode 3D resistivity imaging of alfalfa root zone, Eur. J. Agro., 31, 213–222, https://doi.org/10.1016/j.eja.2009.08.005, 2009. a
Araus, J. L. and Cairns, J. E.: Field high-throughput phenotyping: the new crop breeding frontier, Trend. Plant Sci., 19, 52–61, https://doi.org/10.1016/j.tplants.2013.09.008, 2014. a
Atkinson, J. A., Pound, M. P., Bennett, M. J., and Wells, D. M.: Uncovering the hidden half of plants using new advances in root phenotyping, Curr. Op. Biotechnol., 55, 1–8, https://doi.org/10.1016/j.copbio.2018.06.002, 2019. a
Binley, A.: 11.08 – Tools and Techniques: Electrical Methods, pp. 233–259, Elsevier, Oxford, https://doi.org/10.1016/B978-0-444-53802-4.00192-5, 2015. a
Binley, A. and Slater, L.: Resistivity and Induced Polarization: Theory and Applications to the Near-Surface Earth, Cambridge University Press, Cambridge, https://doi.org/10.1017/9781108685955, 2020. a, b
Blanchy, G. and Garré, S.: “Closing the Phenotyping Gap with Non-Invasive Belowground Field Phenotyping”, January, Zenodo [code], https://zenodo.org/records/14673647 (last access: 21 January 2025), 2025. a
Blanchy, G., Saneiyan, S., Boyd, J., McLachlan, P., and Binley, A.: ResIPy, an intuitive open source software for complex geoelectrical inversion/modeling, Comput. Geosci., 137, 104423, https://doi.org/10.1016/j.cageo.2020.104423, 2020a. a
Blanchy, G., Watts, C. W., Ashton, R. W., Webster, C. P., Hawkesford, M. J., Whalley, W. R., and Binley, A.: Accounting for heterogeneity in the θ−σ relationship: Application to wheat phenotyping using EMI, Vadose Zone J., 19, e20037, https://doi.org/10.1002/vzj2.20037, 2020b. a
Blanchy, G., Watts, C. W., Richards, J., Bussell, J., Huntenburg, K., Sparkes, D. L., Stalham, M., Hawkesford, M. J., Whalley, W. R., and Binley, A.: Timelapse geophysical assessment of agricultural practices on soil moisture dynamics, Vadose Zone J., 19, e20080, https://doi.org/10.1002/vzj2.20080, 2020c. a
Borra-Serrano, I., De Swaef, T., Quataert, P., Aper, J., Saleem, A., Saeys, W., Somers, B., Roldán-Ruiz, I., and Lootens, P.: Closing the Phenotyping Gap: High Resolution UAV Time Series for Soybean Growth Analysis Provides Objective Data from Field Trials, Remote Sens., 12, 1644, https://doi.org/10.3390/rs12101644, 2020. a
Campbell, R. B., Bower, C. A., and Richards, L. A.: Change of Electrical Conductivity With Temperature and the Relation of Osmotic Pressure to Electrical Conductivity and Ion Concentration for Soil Extracts, Soil Sci. Soc. Am. J., 13, 66–69, https://doi.org/10.2136/sssaj1949.036159950013000c0010x, 1949. a
Carminati, A. and Javaux, M.: Soil Rather Than Xylem Vulnerability Controls Stomatal Response to Drought, Trend. Plant Sci., 25, 868–880, https://doi.org/10.1016/j.tplants.2020.04.003, 2020. a
Cassiani, G., Ursino, N., Deiana, R., Vignoli, G., Boaga, J., Rossi, M., Perri, M. T., Blaschek, M., Duttmann, R., Meyer, S., Ludwig, R., Soddu, A., Dietrich, P., and Werban, U.: Noninvasive Monitoring of Soil Static Characteristics and Dynamic States: A Case Study Highlighting Vegetation Effects on Agricultural Land, Vadose Zone J., 11, vzj2011.0195, https://doi.org/10.2136/vzj2011.0195, 2012. a
Das, A., Schneider, H., Burridge, J., Ascanio, A. K. M., Wojciechowski, T., Topp, C. N., Lynch, J. P., Weitz, J. S., and Bucksch, A.: Digital imaging of root traits (DIRT): a high-throughput computing and collaboration platform for field-based root phenomics, Plant Method., 11, 51, https://doi.org/10.1186/s13007-015-0093-3, 2015. a
De Swaef, T., Deroo, W., Blanchy, G., Garré, S., Instituut voor Landbouw en Visserijonderzoek, and Lootens, P.: 2023 HYDRAS Proof of concept experiment with soybean genotypes, In SOIL, Zenodo [data set], https://doi.org/10.5281/zenodo.14175354, 2024. a
Ehosioke, S., Nguyen, F., Rao, S., Kremer, T., PlacenciaGomez, E., Huisman, J. A., Kemna, A., Javaux, M., and Garré, S.: Sensing the electrical properties of roots: A review, Vadose Zone J., 19, e20082, https://doi.org/10.1002/vzj2.20082, 2020. a, b
Garré, S., Coteur, I., Wongleecharoen, C., Kongkaew, T., Diels, J., and Vanderborght, J.: Noninvasive Monitoring of Soil Water Dynamics in Mixed Cropping Systems: A Case Study in Ratchaburi Province, Thailand, Vadose Zone J., 12, 1–12, https://doi.org/10.2136/vzj2012.0129, 2013. a, b
Garre, S., Deswaef, T., Borra-Serrano, I., Lootens, P., and Blanchy, G.: The potential of electrical imaging for field root zone phenotyping, in: NSG2021 27th European Meeting of Environmental and Engineering Geophysics, Eur. Assoc. Geosci. Eng., 1–5, https://doi.org/10.3997/2214-4609.202120221, 2021. a
Koestel, J., Kemna, A., Javaux, M., Binley, A., and Vereecken, H.: Quantitative imaging of solute transport in an unsaturated and undisturbed soil monolith with 3D ERT and TDR, Water Resour. Res., 44, W12411, https://doi.org/10.1029/2007wr006755, 2008. a
LaBrecque, D. J. and Yang, X.: Difference Inversion of ERT Data: a Fast Inversion Method for 3-D In Situ Monitoring, J. Environ. Eng. Geophys., 6, 83–89, https://doi.org/10.4133/jeeg6.2.83, 2001. a
Langstroff, A., Heuermann, M. C., Stahl, A., and Junker, A.: Opportunities and limits of controlled-environment plant phenotyping for climate response traits, Theor. Appl. Genet., 135, 1–16, https://doi.org/10.1007/s00122-021-03892-1, 2021. a
Lärm, L., Bauer, F. M., Hermes, N., van der Kruk, J., Vereecken, H., Vanderborght, J., Nguyen, T. H., Lopez, G., Seidel, S. J., Ewert, F., Schnepf, A., and Klotzsche, A.: Multi-year belowground data of minirhizotron facilities in Selhausen, Sci. Data, 10, 672, https://doi.org/10.1038/s41597-023-02570-9, 2023. a
Linde, N., Ginsbourger, D., Irving, J., Nobile, F., and Doucet, A.: On uncertainty quantification in hydrogeology and hydrogeophysics, Adv. Water Resour., 110, 166–181, https://doi.org/10.1016/j.advwatres.2017.10.014, 2017. a
Ma, R., McBratney, A., Whelan, B., Minasny, B., and Short, M.: Comparing temperature correction models for soil electrical conductivity measurement, Prec. Agr., 12, 55–66, https://doi.org/10.1007/s11119-009-9156-7, 2010. a
McGrail, R., Van Sanford, D., and McNear, D.: Trait-Based Root Phenotyping as a Necessary Tool for Crop Selection and Improvement, Agronomy, 10, 1328, https://doi.org/10.3390/agronomy10091328, 2020. a
Michot, D., Benderitter, Y., Dorigny, A., Nicoullaud, B., King, D., and Tabbagh, A.: Spatial and temporal monitoring of soil water content with an irrigated corn crop cover using surface electrical resistivity tomography, Water Resour. Res., 39, 1138, https://doi.org/10.1029/2002wr001581, 2003. a
Ochs, J., Klitzsch, N., and Wagner, F. M.: Mitigation of installation-related effects for small-scale borehole-to-surface ERT, J. Appl. Geophys., 197, 104530, https://doi.org/10.1016/j.jappgeo.2022.104530, 2022. a
Oldenborger, G. A., Routh, P. S., and Knoll, M. D.: Sensitivity of electrical resistivity tomography data to electrode position errors, Geophys. J. Int., 163, 1–9, https://doi.org/10.1111/j.1365-246X.2005.02714.x, 2005. a
Pranga, J., Borra-Serrano, I., Aper, J., De Swaef, T., Ghesquiere, A., Quataert, P., Roldán-Ruiz, I., Janssens, I. A., Ruysschaert, G., and Lootens, P.: Improving Accuracy of Herbage Yield Predictions in Perennial Ryegrass with UAV-Based Structural and Spectral Data Fusion and Machine Learning, Remote Sens., 13, 3459, https://doi.org/10.3390/rs13173459, 2021. a
Rajurkar, A. B., McCoy, S. M., Ruhter, J., Mulcrone, J., Freyfogle, L., and Leakey, A. D. B.: Installation and imaging of thousands of minirhizotrons to phenotype root systems of field-grown plants, Plant Methods, 18, 39, https://doi.org/10.1186/s13007-022-00874-2, 2022. a
Seabold, S. and Perktold, J.: statsmodels: Econometric and statistical modeling with python, in: 9th Python in Science Conference, 92–96, https://doi.org/10.25080/Majora-92bf1922-011, 2010. a
Shanahan, P. W., Binley, A., Whalley, W. R., and Watts, C. W.: The Use of Electromagnetic Induction to Monitor Changes in Soil Moisture Profiles beneath Different Wheat Genotypes, Soil Sci. Soc. Am. J., 79, 459–466, https://doi.org/10.2136/sssaj2014.09.0360, 2015. a
Snowdon, R. J., Wittkop, B., Chen, T.-W., and Stahl, A.: Crop adaptation to climate change as a consequence of long-term breeding, Theor. Appl. Genet., 134, 1613–1623, https://doi.org/10.1007/s00122-020-03729-3, 2020. a
Srayeddin, I. and Doussan, C.: Estimation of the spatial variability of root water uptake of maize and sorghum at the field scale by electrical resistivity tomography, Plant Soil, 319, 185–207, https://doi.org/10.1007/s11104-008-9860-5, 2009. a
Svane, S. F., Jensen, C. S., and Thorup-Kristensen, K.: Construction of a large-scale semi-field facility to study genotypic differences in deep root growth and resources acquisition, Plant Method., 15, 26, https://doi.org/10.1186/s13007-019-0409-9, 2019. a
Trachsel, S., Kaeppler, S. M., Brown, K. M., and Lynch, J. P.: Shovelomics: high throughput phenotyping of maize (Zea mays L.) root architecture in the field, Plant Soil, 341, 75–87, https://doi.org/10.1007/s11104-010-0623-8, 2010. a
Tso, C.-H. M., Iglesias, M., Wilkinson, P., Kuras, O., Chambers, J., and Binley, A.: Efficient multiscale imaging of subsurface resistivity with uncertainty quantification using ensemble Kalman inversion, Geophys. J. Int., 225, 887–905, https://doi.org/10.1093/gji/ggab013, 2021. a
Uhlemann, S., Wilkinson, P. B., Maurer, H., Wagner, F. M., Johnson, T. C., and Chambers, J. E.: Optimized survey design for electrical resistivity tomography: combined optimization of measurement configuration and electrode placement, Geophys. J. Int., 214, 108–121, https://doi.org/10.1093/gji/ggy128, 2018. a, b
Vamerali, T., Bandiera, M., and Mosca, G.: Minirhizotrons in Modern Root Studies, 341–361 pp., Springer Berlin Heidelberg, https://doi.org/10.1007/978-3-642-22067-8_17, 2011. a
Voss-Fels, K. P., Stahl, A., and Hickey, L. T.: Q–A: modern crop breeding for future food security, BMC Biology, 17, 18, https://doi.org/10.1186/s12915-019-0638-4, 2019. a
Wagner, F. M. and Uhlemann, S.: Chapter One – An overview of multimethod imaging approaches in environmental geophysics, in: Inversion of Geophysical Data, edited by Schmelzbach, C., Vol. 62, Adv. Geophys., 1–72 pp., Elsevier, https://doi.org/10.1016/bs.agph.2021.06.001, 2021. a
Weigand, M. and Kemna, A.: Multi-frequency electrical impedance tomography as a non-invasive tool to characterize and monitor crop root systems, Biogeosciences, 14, 921–939, https://doi.org/10.5194/bg-14-921-2017, 2017. a
Weigand, M., Zimmermann, E., Michels, V., Huisman, J. A., and Kemna, A.: Design and operation of a long-term monitoring system for spectral electrical impedance tomography (sEIT), Geosci. Instrum. Method. Data Syst., 11, 413–433, https://doi.org/10.5194/gi-11-413-2022, 2022. a, b
Whalley, W., Binley, A., Watts, C., Shanahan, P., Dodd, I., Ober, E., Ashton, R., Webster, C., White, R., and Hawkesford, M. J.: Methods to estimate changes in soil water for phenotyping root activity in the field, Plant Soil, 415, 407–422, https://doi.org/10.1007/s11104-016-3161-1, 2017. a
Wilkinson, P. B., Chambers, J. E., Lelliott, M., Wealthall, G. P., and Ogilvy, R. D.: Extreme sensitivity of crosshole electrical resistivity tomography measurements to geometric errors, Geophys. J. Int., 173, 49–62, https://doi.org/10.1111/j.1365-246X.2008.03725.x, 2008. a
Wilkinson, P. B., Chambers, J. E., Meldrum, P. I., Gunn, D. A., Ogilvy, R. D., and Kuras, O.: Predicting the movements of permanently installed electrodes on an active landslide using time-lapse geoelectrical resistivity data only, Geophys. J. Int., 183, 543–556, https://doi.org/10.1111/j.1365-246X.2010.04760.x, 2010. a
Wilkinson, P. B., Uhlemann, S., Chambers, J. E., Meldrum, P. I., and Loke, M. H.: Development and testing of displacement inversion to track electrode movements on 3-D electrical resistivity tomography monitoring grids, Geophys. J. Int., 200, 1566–1581, https://doi.org/10.1093/gji/ggu483, 2015. a
Wohner, C., Peterseil, J., and Klug, H.: Designing and implementing a data model for describing environmental monitoring and research sites, Ecol. Inform., 70, 101708, https://doi.org/10.1016/j.ecoinf.2022.101708, 2022. a
Ye, H., Song, L., Schapaugh, W. T., Ali, M. L., Sinclair, T. R., Riar, M. K., Mutava, R. N., Li, Y., Vuong, T., Valliyodan, B., Pizolato Neto, A., Klepadlo, M., Song, Q., Shannon, J. G., Chen, P., and Nguyen, H. T.: The importance of slow canopy wilting in drought tolerance in soybean, J. Experiment. Botany, 71, 642–652, https://doi.org/10.1093/jxb/erz150, 2019. a
Zhao, P.-F., Wang, Y.-Q., Yan, S.-X., Fan, L.-F., Wang, Z.-Y., Zhou, Q., Yao, J.-P., Cheng, Q., Wang, Z.-Y., and Huang, L.: Electrical imaging of plant root zone: A review, Comput. Electro. Agr., 167, 105058, https://doi.org/10.1016/j.compag.2019.105058, 2019. a
- Abstract
- Introduction
- Methodology
- Results
- Discussion
- Conclusions
- Appendix A: Field management activities
- Appendix B: Measurement sequence
- Appendix C: Overview of inversion quality indicators
- Code and data availability
- Author contributions
- Competing interests
- Disclaimer
- Special issue statement
- Acknowledgements
- Financial support
- Review statement
- References
- Abstract
- Introduction
- Methodology
- Results
- Discussion
- Conclusions
- Appendix A: Field management activities
- Appendix B: Measurement sequence
- Appendix C: Overview of inversion quality indicators
- Code and data availability
- Author contributions
- Competing interests
- Disclaimer
- Special issue statement
- Acknowledgements
- Financial support
- Review statement
- References