the Creative Commons Attribution 4.0 License.
the Creative Commons Attribution 4.0 License.
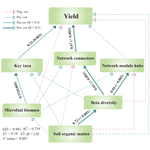
Soil bacterial communities triggered by organic matter inputs associates with a high-yielding pear production
Li Wang
Xiaomei Ye
Hangwei Hu
Jing Du
Yonglan Xi
Zongzhuan Shen
Jing Lin
Deli Chen
The roles of microorganisms in enhancing crop production have been demonstrated for a range of cropping systems. Most studies to date, however, have been confined to a limited number of locations, making it difficult to identify general soil biotic and abiotic characteristics underpinning the yield-promotion across various locations. This knowledge gap limits our capacity to harness soil microbiome to improve crop production. Here we used high-throughput amplicon sequencing to investigate the common features of bacterial community composition, ecological networks and physicochemical properties in six yield-invigorating and adjacent yield-debilitating orchards. We found that yield-invigorating soils exhibited higher contents of organic matter than yield-debilitating soils and harbored unique bacterial communities. Greater alpha diversity and higher relative abundances of Planctomycetota and Chloroflexota were observed in yield-debilitating soils. Co-occurrence network analysis revealed that yield-invigorating soils displayed a greater number of functionally interrelated modules (meta-modules) and a higher proportion of negative links to positive links. Chloroflexota was recognized as a keystone taxon in manipulating the interaction of bacterial communities in yield-invigorating soils. Altogether, we provide evidence that yield-invigorating soils across a range of locations appear to share common features, including accumulation of soil organic matter, higher microbial diversity, enrichment of key taxa like Chloroflexota and maintaining a competitive network. These findings have implications for science-based guidance for sustainable food production.
- Article
(4000 KB) - Full-text XML
-
Supplement
(753 KB) - BibTeX
- EndNote
Soils are essential to human well-being due to their great contributions to the production of food, fiber, feed and medicine (Raaijmakers and Mazzola, 2016). Soil organisms play critical roles in maintaining these ecosystem services, such as driving nutrient cycling, maintaining soil fertility, improving plant productivity and suppressing plant diseases (Bender et al., 2016; Barrios, 2007). Microorganisms participate in nearly all soil biological processes, and the microbial abundance, community composition and activity primarily determine the sustainable productivity of agricultural lands (Philippot et al., 2013). Fungi participate in decomposition of organic matter and deliver nutrients for plant growth (Frąc et al., 2018); however, considering that bacteria are the most diverse and abundant group of microorganisms in soil, bacterial communities and their functions can be pivotal indicators for crop production in agroecosystems (van der Heijden et al., 2008).
In general, an increase in microbial diversity is linked to a high-yielding crop production mainly through improving the host resilience to physical or chemical disturbances, modifying plant competition and facilitating plant access to nutrients (Chaer et al., 2009; Kennedy and Smith, 1995). Since individual organisms do not live in isolation but rather form a complex system of inter-species interactions in soil, interactions among community members were found to be related to crop production in the potato monoculture system (Lu et al., 2013). Enrichments of key functional microbes in soil were deemed to serve specific soil system functions, such as suppressing soil-borne pathogens and maintaining sustainable crop production (Banerjee et al., 2018). However, the relative contributions of microbial diversity, interactions among community members or enrichment of key taxa to crop production remain largely unknown. Therefore, it is highly desirable to identify pivotal indicators of bacterial community composition in response to high-yielding crop production.
Changes in composition of soil bacterial communities across space are often strongly correlated with soil pH (Fierer and Jackson, 2006). Soil pH has been recognized as a key driver in determining the assembly of bacterial communities in arable soils by field experiments (Rousk et al., 2010). However, recent studies have demonstrated that compositions of soil bacterial communities were driven by a myriad of soil abiotic traits, such as organic matter contents, forms and contents of soil nutrients (Tian et al., 2018; Wang et al., 2018). For example, soil bacterial community composition, which determines the ability of soil to suppress soil-borne pathogens, was found to be strongly correlated with soil organic matter (Shen et al., 2018). An imbalanced ratio of soil nutrients, i.e., the ratio of nitrogen to phosphorus or potassium, could be a driving force altering the bacterial community composition in long-term fertilized soils (Eo and Park, 2016). Key soil chemical properties identified in controlling the distribution and abundance of bacterial communities largely depends on the geographical distributions of soils. As a consequence, a better understanding of the relationship between soil edaphic properties and bacterial community composition is critical to develop targeted manipulation options to increase soil service provisions.
“Sucui No. 1” pear is an early-maturing variety bred by the Jiangsu Academy of Agricultural Sciences, China, and has been popularly cultivated in eastern and central China, due to certain advantages including being easy to produce, adaptable to the environment and having good quality and high economic benefits (Lin et al., 2013). With the increasing demand in China, sustainable production of high-quality pear is becoming increasingly important. Manipulation of soil microbiomes has shown to be an effective way to increase soil productivity (Chaparro et al., 2012). Considering that large-scale surveys could exhibit the diversity of soil microbial communities exceeds what is found in host-associated communities (Toju et al., 2018), it is necessary to explore the general microbial characteristics of multiple yield-invigorating soils and identify key environmental drivers in assembling bacterial communities.
In this study, orchards with higher pear yield production compared with local average yield was recognized as yield-invigorating (YI) orchards, while orchards having lower pear yield production in comparison with local average yield was regarded as yield-debilitating (YD) orchards. After field surveys accomplished in 2019, six separately located YI pear orchards and adjacent YD pear orchards were selected for further analysis of soil chemical properties and microbiome. We hypothesized that high input of organic fertilizer could improve soil structure and modify soil chemical properties, which leads to YI soils harboring unique bacterial communities associated with high-yielding pear production. To address this, soil bacterial communities and edaphic properties of the study sites were compared to (1) decipher the differences of taxonomic diversity and composition of the bacterial communities, and (2) determine the contributions of environmental variables to the changes in the structure of bacterial communities.
2.1 Study sites and experimental design
From July–August 2019, a field production survey of orchards cultivated with “Suci No. 1” pear was performed after pear fruits harvest to compare the differences of soil nutrients and microbiota between yield-invigorating with yield-debilitating orchards. The locations, planting density, cropping years, soil type and total yield were recorded. To minimize the effects of microclimate at each site, only pair-located pear orchards with invigorating and debilitating yield and at similar growth stage were selected for this research. In total, six pair-located yield-invigorating and yield-debilitating pear orchards distributed in four cities of Jiangsu province, China, were selected in the main pear production areas (Fig. 1; Table S1 in the Supplement). The yield per tree was obtained by dividing the total yield per hectare by plant density.
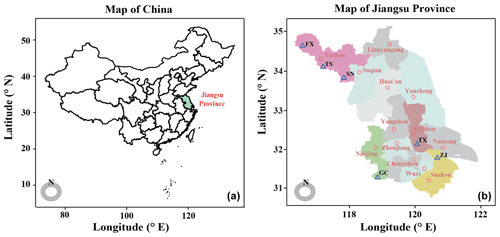
Figure 1Distribution of studied field sites. Map showing the sites of six pair-located orchards sampled in this study.
Paired yield-invigorating and yield-debilitating orchards from Fengxian (FX), Suining (SN) and Tongshan (TS) were maintained in the Xuzhou city under the warm temperate subhumid monsoon climate. This site has a mean annual temperature (MAT) of 14.5 ∘C and mean annual precipitation (MAP) of 847 mm. Orchards from Taixing (TX) were located in the Taizhou city under the humid southern subtropical climate with a MAT of 15.3 ∘C and MAP of 1055 mm. Orchards from Gaochun (GC) were located in the Nanjing city under the humid subtropical monsoon climate with a MAT of 15.4 ∘C and MAP of 1106 mm. Orchards from Zhangjiagang (ZJ) were located in the Suzhou city under the humid subtropical monsoon climate with a MAT of 15.7 ∘C and MAP of 1094 mm. For paired yield-invigorating and yield-debilitating orchards, the irrigation and pesticide management practices were similar according to farm records. However, the yield-invigorating orchard was usually amended with more organic fertilizer under integrated nutrient management, whereas the co-located yield-debilitating orchard received more chemical fertilizer under intensive management. Detailed information about fertilization regimes for each orchard is shown in Table S2 in the Supplement.
2.2 Soil sample collection and chemical properties determination
Along with the field survey, soil sampling campaigns were performed from July to August 2019 after pear fruits harvest. For each yield-invigorating or yield-debilitating orchard, four subplots with three pear trees in each subplot were randomly selected for soil sampling. Subsequently three soil cores (0–20 cm) under the trunk base for each tree were collected using a 25 mm soil auger. In total, nine soil cores for each subplot were pooled as a composite sample and finally four composite soil samples for each orchard were collected and promptly transported on ice to the laboratory. After sifting through a 2 mm sieve and thoroughly mixing, one portion of each soil sample was air-dried for chemical property analyses while the remainder was stored at −70 ∘C for DNA extraction.
Soil chemical properties, including soil pH, content of organic matter (OM), total nitrogen (TN), available phosphorus (AP), available potassium (AK), alkali-hydrolyzable nitrogen (N), exchangeable calcium (Ca), effective magnesium (Mg), effective iron (Fe), effective manganese (Mn), effective copper (Cu) and effective zinc (Zn), were measured according to methods described by Shen et al. (2018) and Huang et al. (2019). Briefly, soil pH was determined using a glass electrode meter in a suspension with a 1 : 5 soil water ratio (). Soil OM was determined using the potassium dichromate external heating method. The TN was determined using a dry combustion method on an Element Analyzer (Vario EL, Germany). The AP and AK were determined using the molybdenum blue method after soil was extracted with sodium bicarbonate and flame photometry after soil was extracted with ammonium acetate, respectively. Soil alkaline hydrolyzable nitrogen (N) was measured by the alkaline hydrolyzable diffusion method. Contents of soil Ca, Mg, Fe, Mn, Cu and Zn were determined by the atomic absorption spectroscopy method using ICE 3300 AAS Atomic Absorption Spectrometer (ThermoScientific, USA) after acid hydrolysis.
2.3 Soil DNA extraction and bacterial abundance quantification
Genomic DNA from 0.25 g soil for each sample was extracted by using the DNeasy® PowerSoil® Kit (QIAGEN GmbH, Germany) according to the manufacturer's instructions. The abundances of soil bacteria were determined with the Eub338F/Eub518R primer using a 7500 Real Time PCR System (Applied Biosystems, USA). Standard curves were generated by using 10-fold serial dilutions of a plasmid containing a full-length copy of the 16S rRNA gene from Escherichia coli. Quantitative PCR analysis was performed in 96-well plates with a 20 µL mixture for each reaction using SYBR® Premix Ex Taq™ (TaKaRa, Japan). Thermal cycling was conducted according to a standard procedure with three replicates, and the results were expressed as log copy numbers per gram of dry soil.
2.4 Sequencing library construction and sequencing
The gene-specific primers 515F/806R with 12 bp barcode were used to amplify the V4 region of bacterial 16S rRNA gene on the BioRad S1000 (Bio-Rad Laboratory, CA) according to the protocols described by Caporaso et al. (2011). All constructed libraries were sequenced using the Illumina NovaSeq 6000 at the Guangdong Magigene Biotechnology Co., Ltd. (Guangzhou, China).
2.5 Sequence processing
Quality filtering of the paired-end raw reads was performed to obtain the high-quality clean reads according to the Trimmomatic V0.33 (Bolger et al., 2014) quality control process. Sequences were assigned to each sample based on their unique barcode, after which the barcodes and primers were removed. Paired-end clean reads were merged using FLASH V1.2.11 (Magoč and Salzberg, 2011). Raw tags were processed to generate the final Amplicon Sequence Variant (ASV) table file at 97 % pairwise identity according to the QIIME2 pipeline (Bolyen et al., 2019). The nonbacterial and mitochondrial ASVs and extremely low frequency ASVs (relative abundance < 0.01 %) were removed. A representative sequence for each ASV was selected and classified using the RDP classifier against the RDP Bacterial 16S database (Wang et al., 2007).
2.6 Statistical analyses
Statistical analyses were performed using the software SPSS 20.0 (SPSS Technologies, Armonk, NY, USA) and R (http://www.R-project.org/, last access: November 2021). Non-normal data were square-root or log transformed when necessary. The significance of soil properties or microbial taxa in yield-invigorating or yield-debilitating orchards was determined based on the paired Wilcoxon rank sum test, and adjusted P values (<0.05) were obtained by the FDR method. Mantel tests were used to identify the correlations between microbial community composition and pear yield, and soil chemical properties using the “vegan” package (Oksanen et al., 2013) in R. The linear regression analyses relating yield to selected microbial taxa or soil chemical properties were conducted using the “basicTrendline” package (Mei et al., 2018) in R.
Principal Coordinate Analysis (PCoA) based on the Bray–Curtis distance was performed in MOTHUR V1.38.1 (Schloss et al., 2009) and visualized by the “ggplot2” package (Wickham and Chang, 2015) in R to explore the differences in microbial community composition. Permutational multivariate analysis of variance (PERMANOVA) was performed to evaluate the significant differences of microbial community composition according to sample locations and orchard yield using the “vegan” package in R. Microbial alpha diversity indexes (Chao, Shannon) were calculated based on randomly resampled ASV abundance matrices at the same depth (23 800 sequences) in MOTHUR. A Venn diagram was generated based on the final ASVs to compare microbial community composition between yield-invigorating and yield-debilitating orchard soils. The affiliations of unique and shared ASVs in yield-invigorating and yield-debilitating soils were compared to evaluate the differences in the bacterial community composition and plotted using the “pheatmap” package (https://cran.r-project.org/web/packages/pheatmap, last access: November 2021) in R. Fold changes (log2 transformed) of shared ASVs across yield-invigorating and yield-debilitating soils were calculated. The ASVs with fold change ratios >2 and unique ASVs in yield-invigorating soils were recognized as potential responders to yield promotion. In addition, to better understand the bacterial community composition, relative abundances at the genus level were compared by STAMP software v2.1.3 (Parks et al., 2014).
The phylogenetic molecular ecological networks (pMEN) were constructed using the random matrix theory-based approach to explore the organization of bacterial communities in yield-invigorating (YI) or yield-debilitating (YD) soil samples. Potential ecological interactions among bacteria were determined by modeling the microbial community using Molecular Ecological Network Analysis (http://ieg2.ou.edu/MENA, last access: November 2021) based on pear yield (Deng et al., 2012). Given the large number of rare taxa that are specific to certain locations, ASVs that occurred in less than half of soil samples and lower than 0.01 % were filtered, which resulted in 591 and 485 ASVs for YI and YD samples, respectively, before networks were constructed. The microbial network was constructed using random matrix theory based at 0.94 similarity threshold and visualized using Cytoscape 2.8.3 software (Smoot et al., 2011). Module clustering and composition in yield-invigorating and yield-debilitating networks were compared and plotted in R using the “pheatmap” and “ggplot2” packages, and the threshold values of Zi and Pi were 2.5 and 0.62, respectively, for topology analysis of the network. Redundancy analysis (RDA) was performed in the R “vegan” package to examine the relationship among frequencies of ASVs, samples and soil variables, which were selected using “stepAIC” in R. Variance partitioning analysis (VPA) was used to determine the contributions of soil properties, sample location and yield, as well as interactions among the variation in a microbial community with Hellinger-transformed data. The predictors of selected soil properties for explaining the pear yield were identified by random forest regression analysis (Boulesteix et al., 2012). The significance of each predictor in the response variables was assessed with the “rfPermute” package (Liaw and Wiener, 2002) with 1000 permutations based on 1000 trees.
3.1 Overview of sequencing data
In total, 1 622 858 16S rRNA sequences were retained after quality control and a total of 9394 ASVs were obtained for the 16S rRNA gene sequences based on 97 % similarity. Among the total 16S rRNA gene sequences, 159 ASVs with 74 372 sequences were classified as archaea while 9235 ASVs with 1 548 486 sequences were identified as bacteria. Among Bacteria, Acidobacteriota, Pseudomonadota, Chloroflexota, Planctomycetota and Actinomycetota were the most abundant phyla (Fig. S1 in the Supplement).
3.2 Soil chemical properties
Soil chemical properties differed among the locations and orchard yield types (Table S3 in the Supplement). On average, yield-invigorating orchards showed obviously higher contents of OM, AP, Mg and Fe, and a lower content of Mn, in comparison with those in yield-debilitating orchards. However, when taking all sites together, only a higher relative abundance of OM, on average, was observed in yield-invigorating orchards compared with that in yield-debilitating orchards based on the Wilcoxon test (P<0.05).
3.3 Bacterial abundances and community compositions
Yield-invigorating orchards together displayed significantly higher abundances of total bacteria than those in co-located yield-debilitating orchards based on real-time PCR results (Fig. 2a). Meanwhile, bacterial community compositions at the ASV level were significantly correlated to pear yield (r=0.460, p=0.001) (Fig. 2b).
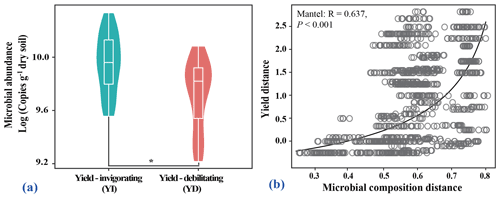
Figure 2Quantitation of the abundance of bacteria population, and linkage of microbial composition to pear yield. (a) Violin plot showing the abundance of total bacteria for all selected orchards. The asterisk indicates a significant difference between yield-invigorating (YI) and yield-debilitating (YD) orchards based on Wilcoxon tests (p<0.05). (b) Correlation plot showing the relationship of microbial composition and yield based on Bray–Curtis distances calculated by the Mantel test.
PCoA based on Bray–Curtis distance matrices clearly revealed location-based differences in bacterial community compositions (Fig. 3a). Six distinct groups representing samples from different locations (FX, GC, SN, TS, TX and ZJ) were obviously separated and confirmed by the PERMANOVA test (F=14.9, P=0.001). At each location, soil bacterial community composition in yield-invigorating orchards was significantly separated from that in co-located yield-debilitating orchards, which was also confirmed by the PERMANOVA test (F=3.6, P=0.001). Although only the Shannon diversity in yield-invigorating orchards from GC and ZJ was significantly higher than that in co-located yield-debilitating orchards (Fig. S2 in the Supplement), the mean alpha diversity indices of Chao and Shannon in all yield-invigorating orchards were significantly higher than those in all yield-debilitating orchards based on the paired Wilcoxon test (Fig. 3b).
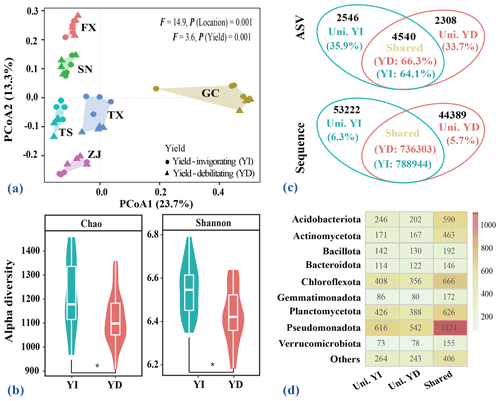
Figure 3Overview of bacterial composition and alpha diversity. (a) Principal Coordinates Analysis (PCoA) plot displaying the bacterial community composition calculated based on Bray–Curtis distances. (b) Violin plot showing the alpha diversity indices (Chao and Shannon) for all selected orchards. Asterisk indicates a significant difference between yield-invigorating (YI) and yield-debilitating (YD) orchards based on Wilcoxon tests (p<0.05). (c) Venn plot depicting the unique and shared bacterial ASVs between yield-invigorating (YI) and yield-debilitating (YD) orchards at ASV and sequence insights. Uni. YI and Uni. YD represent unique ASVs or sequences in the YI or YD soils while Shared represent shared ASVs or sequences between the YI and YD soils. (d) Heatmap displaying the composition of unique and shared ASVs at phylum level in YI and YD soils. Numbers in the cell represent the number of ASVs affiliated to that phylum.
The Venn diagram showed that 4540 ASVs occupying over 90 % of total sequences were shared between yield-invigorating and yield-debilitating orchards (Fig. 3c). Among these shared ASVs, the fold changes larger than 2 of ASVs in yield-invigorating compared with yield-debilitating orchards were recognized as potential responders linking to yield improvement. Surprisingly, none of these ASVs potentially linked to yield improvement were shared among six separated co-located orchards (Fig. S3 in the Supplement). A total of 2546 unique ASVs with 53 222 sequences were found in all yield-invigorating orchards while 2308 unique ASVs with 44 389 sequences were observed in all yield-invigorating orchards. Among these unique ASVs, almost 70 % of ASVs were shared between yield-invigorating orchards and yield-debilitating orchards; however, no shared unique ASVs were found among six separately located orchards. The affiliation of unique and shared ASVs at the phylum level exhibited that the Pseudomonadota, Planctomycetota, Chloroflexota, Acidobacteriota and Actinomycetota were the top five phyla (Fig. 3d).
At the phylum level, the relative abundances of bacterial dominant phyla varied across the location and orchard yield condition. Pseudomonadota, Acidobacteriota, Actinomycetota, Chloroflexota and Planctomycetota were the top five abundant phyla (Fig. 4). The mean abundance of Chloroflexota and Planctomycetota was significantly higher, while Firmicutes was significantly lower, in yield-invigorating orchards compared with yield-debilitating orchards based on the Wilcoxon test (Fig. S4 in the Supplement).
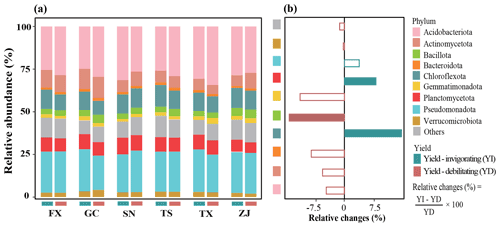
Figure 4Key taxonomic groups in distinguishing yield-invigorating (YI) and yield-debilitating (YD) orchards. (a) Stacked bar chart showing dominant phyla affiliation in YI and YD soils for six pair-located sites, and (b) horizontal histogram depicting relative changes of dominant phyla in YI soils compared with those in YD soils.
At a finer resolution, 967 genera were observed for all soil samples, among which 299 genera appeared in more than half of soil samples in yield-invigorating or yield-debilitating orchards; however, only 34 genera displayed significant differences between yield-invigorating or yield-debilitating orchard soils based on STAMP analysis (Fig. S5 in the Supplement). Interestingly, Ornatilinea, Ktedonobacter and Longilinea, belonging to Chloroflexota, were significantly enriched in yield-invigorating orchard soils. Gimesia in Planctomycetota and Arenimonas in Pseudomonadota showed significantly higher relative abundances in yield-invigorating orchard soils than in yield-debilitating orchard soils.
3.4 Co-occurrence patterns of bacterial communities
The yield-invigorating network contained 302 nodes, 448 edges and 11 larger modules (>5 nodes), with an average connectivity (avgK) of 2.967, average path distance of 5.494 and clustering coefficient (avgCC) of 0.152, while the values in the yield-debilitating network were 235, 334, 9, 2.843, 6.232 and 0.131, respectively (Fig. 5a; Table S4 in the Supplement). The module eigengene network analysis revealed a difference in the higher-order organization between the two networks. Notably, the node composition was substantially different between the two networks as the relative abundances of dominant phyla were obviously different among different modules (Fig. 5b and c). A higher proportion of nodes in the module of the yield-invigorating network was unique. The ASVs affiliated with Acidobacteriota, Chloroflexota, Pseudomonadota, Actinomycetota and Planctomycetota within the unique modules (M9, M10 and M11) were observed in the yield-invigorating versus yield-debilitating network.
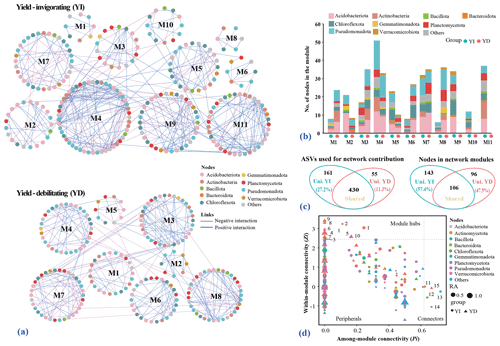
Figure 5Co-occurrence networks of bacterial communities and identified keystone taxa in distinguishing yield-invigorating (YI) and yield-debilitating (YD) orchards. (a) An overview of microbial phylogenetic molecular ecological networks constructed from YI and YD soils. Blue lines indicate positive correlations in each network, whereas pink lines signify negative correlations. Only modules containing more than five nodes in the networks are displayed in the network and labeled with corresponding letter followed by a number. Circular node colors indicate different bacterial phyla. (b) Stacked figure showing the relative abundance of nodes in each module within each network at the phylum level. (c) Venn plot depicting the unique and shared bacterial ASVs between two networks constructed from YI and YD soils. The left panel is plotted based on the original nodes used in building the network, while the right panel is plotted based on the nodes from modules. Uni. YI and Uni. YD represent unique ASVs in the YI or YD networks, while Shared represent shared ASVs between the YI and YD networks. (d) Zi–Pi plot showing the distribution of nodes based on their topological roles. The threshold values of Zi and Pi for categorizing OTUs were 2.5 and 0.62, respectively. Node colors indicate different bacterial phyla and node sizes represent the relative abundance in each network.
Analysis using the threshold values of Zi (within-module connectivity) and Pi (among-module connectivity) showed that the majority of nodes from both networks were categorized as peripherals that had only a few links and almost always linked to the nodes within their own modules (Fig. 5d). Although only three nodes affiliated with Acidobacteriota were categorized as module hubs in the yield-invigorating network, seven nodes belonging to Acidobacteriota, Actinomycetota and Pseudomonadota were categorized as module hubs in the yield-debilitating network. Interestingly, four nodes including Longilinea species from Chloroflexota in the yield-invigorating network, whereas only one node in the yield-debilitating network, were categorized as module connectors (Table S5 in the Supplement).
3.5 Relationships between soil chemical properties and microbial community composition
Soil chemical properties were significantly correlated to the bacterial community compositions (Mantel: r=0.803, p=0.001). Soil chemical properties, location and orchard explained 44.9 % of the observed variation, leaving 55.1 % of the variation unexplained for bacterial community composition based on VPA result (Fig. 6a). Variation in the community composition was largely explained by soil properties (42.3 %), and was also influenced by locations and orchard yield types.
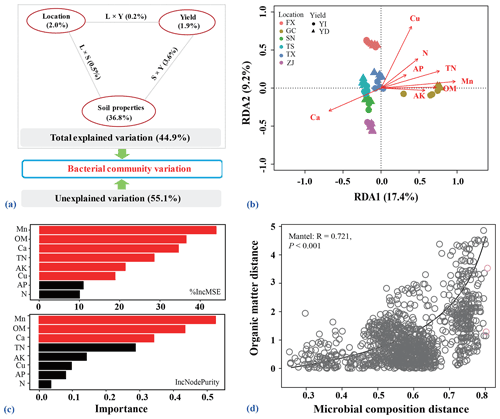
Figure 6Relationships among bacterial communities, soil edaphic factors and pear yield. (a) Variance partitioning analysis (VPA) map of the effects of soil edaphic properties, sample locations, pear yield and interactions of these factors on the bacterial communities. (b) Redundancy analysis (RDA) plot showing the relationships among all assigned bacterial ASVs and measured soil edaphic properties for all soils after stepwise selection. (c) Random forest mean predictor importance of selected soil edaphic properties used as drivers in predicting the pear yield. Red bar indicates that the given predictor is significant, while black bar indicates that the given predictor is non-significant. The %IncMSE in the upper panel means the increase in mean squared error, while IncNodePurity indicates the increase in node purity. The values of these two indices represent the importance of each variable to predict the module. A larger value indicates that the variable is more important. (d) Correlation plot showing the relationship of microbial composition and soil organic matter based on Bray–Curtis distances calculated by the Mantel test.
After forward stepwise selection, the module including soil OM, TN, alkaline N, AP and AK, available calcium (Ca), copper (Cu) and manganese (Mn) explained the majority of the variation in bacterial community composition (Fig. 6b). As evidenced by the RDA vectors, OM within the module was identified as the most important soil property that determines the composition of bacterial communities. Random forest analysis showed that contents of soil Mn, OM and Ca were the top parameters for predicting the orchard yield (Fig. 6c). Furthermore, soil OM was also significantly correlated with bacterial communities as revealed by the Mantel test (Fig. 6d; Table S6 in the Supplement).
Although pear is among the most important fruits worldwide, soil microbial communities in pear orchards have been largely under-investigated (Huang et al., 2019). The present study attempts to decipher the bacterial communities linked to high-yield production of pear. Our results based on Mantel analysis suggested significant correlations among bacterial communities, soil chemical properties and pear yield. Microbial characteristics responding to yield promotion have repeatedly been observed on several crops depending on single experimental site (Zhong et al., 2020; Qiao et al., 2019; Shen et al., 2013). It remained unclear, however, whether these distinctions are ubiquitous at a large-scale. By comparing multiple co-located yield-invigorating and yield-debilitating orchards, we demonstrate that high-yielding pear production soils exhibited high organic matter contents and harbored bacterial communities with high diversity, significantly enriched indigenous microbes and more interactive network, which was triggered by high inputs of soil organic fertilizer. Here we have discussed these main results and potential mechanisms in detail.
Microbial diversity is critical to soil ecosystems in maintaining the integrity, function and long-term sustainability (Kennedy and Smith, 1995). Higher soil biodiversity is considered to be linked to a more stable system and enhance the combination of vital microbial functions and processes (Cardinale et al., 2006; Bell et al., 2005). In line with a previous report that crop yield was correlated to the soil bacterial diversity (Zhao et al., 2014), greater diversity of bacterial communities in yield-invigorating soils was observed in the present study. Hence, we infer that higher microbial diversity may result in a more productive agroecosystem, contributing to sustainable pear production.
In this study, we found that Pseudomonadota, Acidobacteriota, Actinomycetota, Planctomycetota and Chloroflexota were the top abundant phyla. This result roughly agreed with previous studies showing that Pseudomonadota, Acidobacteriota and Actinomycetota are usually dominant bacterial taxa in agricultural soils (Xun et al., 2019; Dai et al., 2018), while Planctomycetota and Chloroflexota exhibit an unexpectedly high relative abundance in rice cropped soil (Edwards et al., 2015) and sandy loam soil (Pathan et al., 2021). The highest relative abundance of Pseudomonadota was probably explained by the fact that Pseudomonadota are considered as copiotrophic bacteria and flourish in soils with large amounts of available nutrients (Fierer et al., 2007).
Moreover, a significantly higher abundance of Planctomycetota and Chloroflexota was observed in yield-invigorating orchards, indicating that Planctomycetota and Chloroflexota may be associated with pear yield improvement. There is no direct evidence showing that Planctomycetota could improve plant growth. However, Planctomycetota has been reported to be involved in many soil biological processes such as ammoxidation as well as carbohydrate and polysaccharide metabolism (Fuerst, 2017). This implies that Planctomycetota may promote plant production through improving soil fertility. Chloroflexota is a facultative anaerobic phylum including autotrophic, heterotrophic and mixotrophic taxa (Speirs et al., 2019). Considering that soil amended with organic fertilizer may enhance the soil water holding capacity, the yield-invigorating soils with more organic material input have a higher soil moisture content, especially after irrigation, probably leading to the enrichment of Chloroflexota in soil.
Network analysis is a systems-level method to explore interactions within an ecosystem that cannot be directly observed through co-occurrence analysis (Fath et al., 2007). Similar to the food web network analyses in macro-ecosystems, microorganisms also form complex interactions with other species (Faust and Raes, 2012) and have been widely investigated to explore the linkage of microbial networks with soil function, such as nutrient supply (Fan et al., 2021) and disease suppression (Lu et al., 2013). Overall, in line with previous findings (Hu et al., 2020), the topological properties of the constructed networks, including connectivity, average clustering coefficients, average degree distance and modularity, indicate that these networks are scale-free, modular and small world. In short, a scale-free network represents a network whose connectivity follows a power law, and most of the nodes have only a few connections with other nodes, whereas a small-world network is a network in which most nodes are not neighbors but can be reached by a few paths. Modularity is a fundamental characteristic of biological network as a module in the network is a group of nodes that are highly connected within the group, but with very few connections outside the group (Deng et al., 2012). Our comparative network analysis indicated that microbial co-occurrence patterns in soils links to different pear production. As a meta-module is usually considered as a group of modules functionally interrelated (Langfelder and Horvath, 2007), a greater number of meta-modules were identified in the network constructed from yield-invigorating soils, suggesting that a greater number of network nodes in the yield-invigorating soils were functionally interrelated than those in the yield-debilitating soils. A majority of nodes in the meta-modules were not shared between yield-invigorating and yield-debilitating networks, indicating basal shifts in network architecture during pear production with contrasting yield performance.
Furthermore, a higher proportion of negative interactions to positive interactions were identified in the network constructed from the yield-invigorating network than the yield-debilitating network. Our results indicated stronger resource competitions in yield-invigorating soils, which means that the soil co-occurrence network was more stable to maintain soil ecosystem function (Coyte et al., 2015). In our study, three module connectors and three module hubs were identified as potentially key taxa in the yield-invigorating network. Interestingly, among those key species, ASV357 affiliated to Longilinea, belonging to the Chloroflexota, was recognized as a key phylum associated with higher pear yields. Similarly, Chloroflexota was reported to be keystone taxa in the constructed network from agricultural soils with 40 years of fertilization (Fan et al., 2021). Chloroflexota play key roles in connecting network nodes of soil microbiome probably because Chloroflexota could participate in degrading plant compounds to create more nutrients via pathways for the degradation of starch, cellulose and long-chain sugars, as it is positively correlated with genes for amino sugars, sugar alcohols and simple carbohydrate metabolic pathways (Hug et al., 2013).
In this study, a significantly higher content of soil organic matter was observed in yield-invigorating orchards, demonstrating that soil organic matter could drive the assembly of bacterial communities. Consensus is emerging that microbial residues are an important constituent of soil organic matter (Kallenbach et al., 2016), which participate in almost all soil biological processes (Fierer, 2017). Despite that the quality of soil organic matter was not evaluated in this study, the quality of soil organic matter was associated with the diversity of microbial community (Ding et al., 2015), which implies that in our future work more attention should be paid to illustrate the relationship between the quality of soil organic matter and microbial community.
In conclusion, yield-invigorating soils displayed a higher content of organic matter and harbored unique bacterial communities with greater diversity than yield-debilitating soils. Further Chloroflexota was significantly enriched and identified as a potential keystone taxon in manipulating the interaction of bacterial communities in yield-invigorating soils. These findings indicated that soil organic matter triggered the assembly of soil microbiome, which both participated in maintaining crop production. Such knowledge is a first step toward harnessing soil microbiome in support of sustainable agroecosystems.
Raw amplicon sequencing data for each sample used in this study was deposited at the National Center for Biotechnology Information (NCBI) in the FASTQ format and is available under the accession number PRJNA749397. Other data that support the findings of this study are available on request from the corresponding author (Xiaomei Ye).
The supplement related to this article is available online at: https://doi.org/10.5194/soil-8-337-2022-supplement.
LW: performed all experiments; LW, XY and ZS: designed the study and wrote the majority of the paper; LW and ZS: analyzed the data; HH, JD, YX, JL and DC: participated in the design of the study, provided comments and edited the paper. All authors read and approved the final paper.
The contact author has declared that neither they nor their co-authors have any competing interests.
Publisher’s note: Copernicus Publications remains neutral with regard to jurisdictional claims in published maps and institutional affiliations.
We sincerely thank all those who assisted with any part of this paper and all pear orchard owners for providing access to the soil sampling.
This research has been supported by the Jiangsu Agricultural Science and Technology Innovation Fund (grant no. CX(19)3094) and the National Natural Science Foundation of China (grant nos. 31801842 and 42090065).
This paper was edited by Axel Don and reviewed by two anonymous referees.
Banerjee, S., Schlaeppi, K., and van der Heijden, M. G. J.: Keystone taxa as drivers of microbiome structure and functioning, Nat. Rev. Microbiol., 16, 567–576, 2018.
Barrios, E.: Soil biota, ecosystem services and land productivity, Ecol. Econ., 64, 269–285, 2007.
Bell, T., Newman, J. A., Silverman, B. W., Turner, S. L., and Lilley, A. K.: The contribution of species richness and composition to bacterial services, Nature, 436, 1157–1160, 2005.
Bender, S. F., Wagg, C., and van der Heijden, M. G. A.: An underground revolution: biodiversity and soil ecological engineering for agricultural sustainability, Trend. Ecol. Evol., 31, 440–452, 2016.
Bolger, A. M., Lohse, M., and Usadel, M.: Trimmomatic: a flexible trimmer for Illumina sequence data, Bioinformatics, 30, 2114–2120, 2014.
Bolyen, E., Rideout, J. R., Dillon, M. R., Bokulich, N. A., Abnet, C. C., Al-Ghalith, G. A., Alexander, H., Alm, E. J., Arumugam, M., Asnicar, F., Bai, Y., Bisanz, J. E., Bittinger, K., Brejnrod, A., Brislawn, C. J., Brown, C. T., Callahan, B. J., Caraballo-Rodríguez, A. M., Chase, J., Cope, E. K., Silva, R. D., Diener, C., Dorrestein, P. C., Douglas, G. M., Durall, D. M., Duvallet, C., Edwardson, C. F., Ernst, M., Estaki, M., Fouquier, J., Gauglitz, J. M., Gibbons, S. M., Gibson, D. L., Gonzalez, A., Gorlick, K., Guo, J., Hillmann, B., Holmes, S., Holste, H., Huttenhower, C., Huttley, G. A., Janssen, S., Jarmusch, A. K., Jiang, L., Kaehler, B. D., Kang, K. B., Keefe, C. R., Keim P., Kelley, S. T., Knights, D., Koester, I., Kosciolek, T., Kreps, J., Langille, M. G. I., Lee, J., Ley, R., Liu, Y., Loftfield, E., Lozupone, C., Maher, M., Marotz, C., Martin, B. D., McDonald, D., McIver, L. J., Melnik, A. V., Metcalf, J. L., Morgan, S. C., Morton, J.T., Naimey, A. T., Navas-Molina, J. A., Nothias, L. F., Orchanian, S. B., Pearson, T., Peoples, S. L., Petras, D., Preuss, M. L., Pruesse, E., Rasmussen, L. B., Rivers, A., Robeson II, M. S., Rosenthal, P., Segata, N., Shaffer, M., Shiffer, A., Sinha, R., Song, S. J., Spear, J. R., Swafford, A., D., Thompson, L. R., Torres, P. J., Trinh, P., Tripathi, A., Turnbaugh, P. J., Ul-Hasan, S., van der Hooft, J. J. J., Vargas, F., Vázquez-Baeza, Y., Vogtmann, E., von Hippel, M., Walters, W., Wan, Y., Wang, M., Warren, J., Weber, K. C., Williamson, C. H. D., Willis, A. D., Xu, Z. Z., Zaneveld, J. R., Zhang, Y., Zhu, Q., Knight, R., and Caporaso, J. G.: Reproducible, interactive, scalable and extensible microbiome data science using QIIME 2, Nat. Biotechnol., 37, 852–857, 2019.
Boulesteix, A. L., Janitza, S., Kruppa, J., and König, I. R.: Overview of random forest methodology and practical guidance with emphasis on computational biology and bioinformatics, Wires Data Min. Knowl., 2, 493–507, 2012.
Caporaso, J. G., Lauber, C. L., Walters, W. A., Berg-Lyons, D., Lozupone, C. A., Turnbaugh, P. J., Fierer, N., and Knight, R.: Global patterns of 16S rRNA diversity at a depth of millions of sequences per sample, P. Natl. Acad. Sci. USA, 108, 4516–4522, 2011.
Cardinale, B. J., Srivastava, D. S., Duffy, J. E., Wright, J. P., Downing, A. L., Sankaran, M., and Jouseau, C.: Effects of biodiversity on the functioning of trophic groups and ecosystems, Nature, 443, 989–992, 2006.
Chaer, G., Fernandes, M., Myrold, D., and Bottomley, P.: Comparative resistance and resilience of soil microbial communities and enzyme activities in adjacent native forest and agricultural soils, Microb. Ecol., 58, 414–424, 2009.
Chaparro, J. M., Sheflin, A. M., Manter, D. K., and Vivanco, J. M.: Manipulating the soil microbiome to increase soil health and plant fertility, Biol. Fert. Soils, 48, 489–499, 2012.
Coyte, K. Z., Schluter, J., and Foster, K. R.: The ecology of the microbiome: networks, competition, and stability, Science, 350, 663–666, 2015.
Dai, Z., Su, W., Chen, H., Barberán, A., Zhao, H., Yu, M., Yu, L., Brookes, P. C., Schadt, C. W., Chang, S. X., and Xu, J.: Long-term nitrogen fertilization decreases bacterial diversity and favors the growth of Actinobacteria and Proteobacteria in agro-ecosystems across the globe, Glob. Change Biol., 24, 3452–3461, 2018.
Deng, Y., Jiang, Y., Yang, Y., He, Z., Luo, F., and Zhou, J.: Molecular ecological network analyses, BMC Bioinformatics, 13, 1–20, 2012.
Ding, J., Zhang, Y., Wang, M., Sun, X., Cong, J., Deng, Y., Lu, H., Yuan, T., van Nostrand, J. D., Li, D., Zhou, J., and Yang, Y.: Soil organic matter quantity and quality shape microbial community compositions of subtropical broadleaved forests, Mol. Ecol., 24, 5175–5185, 2015.
Edwards, J., Johnson, C., Santos-Medellín, C., Lurie, E., Podishetty, N. K., Bhatnagar, S., Eisen, J. A., and Sundaresan, V.: Structure, variation, and assembly of the root-associated microbiomes of rice, P. Natl. Acad. Sci. USA, 112, E911–E920, 2015.
Eo, J. and Park, K.: Long-term effects of imbalanced fertilization on the composition and diversity of soil bacterial community, Agr. Ecosyst. Environ., 231, 176–182, 2016.
Fan, K., Delgado-Baquerizo, M., Guo, X., Wang, D., Zhu, Y., and Chu, H.: Biodiversity of key-stone phylotypes determines crop production in a 4-decade fertilization experiment, ISME J., 15, 550–561, 2021.
Fath, B. D., Scharler, U. M., Ulanowicz, R. E., and Hannon, B.: Ecological network analysis: network construction, Ecol. Model., 208, 49–55, 2007.
Faust, K. and Raes, J.: Microbial interactions: from networks to models, Nat. Rev. Microbiol., 10, 538–550, 2012.
Fierer, N.: Embracing the unknown: disentangling the complexities of the soil microbiome, Nat. Rev. Microbiol., 15, 579–590, 2017.
Fierer, N. and Jackson, R. B. J.: The diversity and biogeography of soil bacterial communities, P. Natl. Acad. Sci. USA, 103, 626–631, 2006.
Fierer, N., Bradford, M. A., and Jackson, R. B. J.: Toward an ecological classification of soil bacteria, Ecology, 88, 1354–1364, 2007.
Frąc, M., Hannula, S. E., Bełka, M., and Jędryczka, M.: Fungal biodiversity and their role in soil health, Front. Microbiol., 9, 707, https://doi.org/10.3389/fmicb.2018.00707, 2018.
Fuerst, J. A.: Planctomycetes – new models for microbial cells and activities, edited by: Kurtböke, I., Microbial Resources, Elsevier/Academic Press, London, 1–27, https://doi.org/10.1016/B978-0-12-804765-1.00001-1, 2017.
Hu, Q., Tan, L., Gu, S., Xiao, Y., Xiong, X., Zeng, W., Feng, K., Wei, Z., and Deng, Y.: Network analysis infers the wilt pathogen invasion associated with non-detrimental bacteria, npj Biofilms Microb., 6, 1–8, 2020.
Huang, Z., Zhao, F., Wang, M., Qi, K., Wu, J., and Zhang, S.: Soil chemical properties and geographical distance exerted effects on arbuscular mycorrhizal fungal community composition in pear orchards in Jiangsu Province, China, Appl. Soil Ecol., 142, 18–24, 2019.
Hug, L. A., Castelle, C. J., Wrighton, K. C., Thomas, B. C., Sharon, I., Frischkorn, K. R., Williams, K. H., Tringe, S. G., and Banfield, J. F.: Community genomic analyses constrain the distribution of metabolic traits across the Chloroflexi phylum and indicate roles in sediment carbon cycling, Microbiome, 1, 1–17, 2013.
Kallenbach, C. M., Frey, S. D., and Grandy, A. S. J.: Direct evidence for microbial-derived soil organic matter formation and its ecophysiological controls, Nat. Commun., 7, 1–10, 2016.
Kennedy, A. C. and Smith, K. J.: Soil microbial diversity and the sustainability of agricultural soils, Plant Soil, 170, 75–86, 1995.
Langfelder, P. and Horvath, S. J.: Eigengene networks for studying the relationships between co-expression modules, BMC Syst. Biol., 1, 1–17, 2007.
Liaw, A. and Wiener, M.: Classification and regression by randomForest, R News, 2, 18–22, 2002.
Lin, J., Sheng, B., Li, X., Yang, Q., Wang, Z., Li, H., Wang, H., and Cheng, Y.: A new pyrus pyrifolia cultivar “Sucui 1”, Acta Hortic. Sin., 40, 1849–1850, 2013 (in Chinese)
Lu, L., Yin, S., Liu, X., Zhang, W., Gua, T., Shen, Q., and Qiu, H.: Fungal networks in yield-invigorating and-debilitating soils induced by prolonged potato monoculture, Soil Biol. Biochem., 65, 186–194, 2013.
Magoč, T. and Salzberg, S. L.: FLASH: fast length adjustment of short reads to improve genome assemblies, Bioinformatics, 27, 2957–2963, 2011.
Mei, W., Yu, G., Lai, J., Rao, Q., and Umezawa, Y.: basicTrendline: add trendline and confidence interval of basic regression models to plot, R package version 2.0.3, http://cran.r-project.org/package=basicTrendline, 2018.
Oksanen, J., Blanchet, F. G., Friendly, M., Kindt, R., Legendre, P., McGlinn, D., Minchin, P. R., O'Hara, R. B., Simpson, G. L., Solymos, P., Stevens, M. H. H., Szoecs, E., and Wagner, H.: Package “vegan”, Community ecology package, version 2, http://CRAN.R-project.org/package=vegan (last access: November 2021), 2013.
Parks, D. H., Tyson, G. W., Hugenholtz, P., and Beiko, R. G.: STAMP: statistical analysis of taxonomic and functional profiles, Bioinformatics, 30, 3123–3124, 2014.
Pathan, S. I., Roccotelli, A., Petrovičovà, B., Romeo, M., Badagliacca, G., Monti, M., and Gelsomino, A.: Temporal dynamics of total and active prokaryotic communities in two Mediterranean orchard soils treated with solid anaerobic digestate or managed under no-tillage, Biol. Fert. Soils, 57, 837–861, 2021.
Philippot, L., Raaijmakers, J. M., Lemanceau, P., and van der Putten, W. H.: Going back to the roots: the microbial ecology of the rhizosphere, Nat. Rev. Microbiol., 11, 789–799, 2013.
Qiao, C., Penton, C. R., Xiong, W., Liu, C., Wang, R., Liu, Z., Xu, X., Li, R., and Shen, Q.: Reshaping the rhizosphere microbiome by bio-organic amendment to enhance crop yield in a maize-cabbage rotation system, Appl. Soil Ecol., 142, 136–146, 2019.
Raaijmakers, J. M. and Mazzola, M. J.: Soil immune responses, Science, 352, 1392–1393, 2016.
Rosseel, Y.: Lavaan: An R package for structural equation modeling and more, J. Stat. Softw., 48, 1–36, 2012.
Rousk, J., Bååth, E., Brookes, P. C., Lauber, C. L., Lozupone, C., Caporaso, J. G., Knight, R., and Fierer, N.: Soil bacterial and fungal communities across a pH gradient in an arable soil, ISME J., 4, 1340–1351, 2010.
Schloss, P. D., Westcott, S. L., Ryabin, T., Hall, J. R., Hartmann, M., Hollister, E. B., Lesniewski, R. A., Oakley, B. B., Parks, D. H., Robinson, C. J., Sahl, J. W., Stres, B., Thallinger, G. G., Van Horn, D. J., and Weber, C. F.: Introducing mothur: open-source, platform-independent, community-supported software for describing and comparing microbial communities, Appl. Environ. Microb., 75, 7537–7541, 2009.
Shen, Z., Zhong, S., Wang, Y., Wang, B., Mei, X., Li, R., Ruan, Y., and Shen, Q.: Induced soil microbial suppression of banana fusarium wilt disease using compost and biofertilizers to improve yield and quality, Eur. J. Soil Biol., 57, 1–8, 2013.
Shen, Z., Penton, C. R., Lv, N., Xue, C., Yuan, X., Ruan, Y., Li, R., and Shen, Q.: Banana Fusarium wilt disease incidence is influenced by shifts of soil microbial communities under different monoculture spans, Microb. Ecol., 75, 739–750, 2018.
Smoot, M. E., Ono, K., Ruscheinski, J., Wang, P. L., and Ideker, T.: Cytoscape 2.8: new features for data integration and network visualization, Bioinformatics, 27, 431–432, 2011.
Speirs, L. B. M., Rice, D. T. F., Petrovski, S., and Seviour, R. J.: The phylogeny, biodiversity, and ecology of the Chloroflexi in activated sludge, Front Microbiol., 10, 2015, https://doi.org/10.3389/fmicb.2019.02015, 2019.
Tian, J., He, N., Hale, L., Niu, S., Yu, G., Liu, Y., Blagodatskaya, E., Kuzyakov, Y., Gao, Q., and Zhou, J.: Soil organic matter availability and climate drive latitudinal patterns in bacterial diversity from tropical to cold temperate forests, Funct. Ecol., 32, 61–70, 2018.
Toju, H., Peay, K. G., Yamamichi, M., Narisawa, K., Hiruma, K., Naito, K., Fukuda, S., Ushio, M., Nakaoka, S., Onoda, Y., Yoshida, K., Schlaeppi, K., Bai, Y., Sugiura, R., Ichihashi, Y., Minamisawa, K., and Kiers, E. T.: Core microbiomes for sustainable agroecosystems, Nat Plants, 4, 247–257, 2018.
van der Heijden, M. G., Bardgett, R. D., and van Straalen, N. M. J.: The unseen majority: soil microbes as drivers of plant diversity and productivity in terrestrial ecosystems, Ecol. Lett., 11, 296–310, 2008.
Wang, C., Liu, D., and Bai, E.: Decreasing soil microbial diversity is associated with decreasing microbial biomass under nitrogen addition, Soil Biol. Biochem., 120, 126–133, 2018.
Wang, Q., Garrity, G. M., Tiedje, J. M., and Cole, J. R.: Naive Bayesian classifier for rapid assignment of rRNA sequences into the new bacterial taxonomy, Appl. Environ. Microb., 73, 5261–5267, 2007.
Wickham, H. and Chang, W.: ggplot2: an implementation of the grammar of graphics, http://CRAN.R-project.org/package=ggplot2 (last access: November 2021), 2015.
Xun, W., Li, W., Xiong, W., Ren, Y., Liu, Y., Miao, Y., Xu, Z., Zhang, N., Shen, Q., and Zhang, R.: Diversity-triggered deterministic bacterial assembly constrains community functions, Nat. Commun., 10, 1–10, 2019.
Zhao, J., Ni, T., Li, Y., Xiong, W., Ran, W., Shen, B., Shen, Q., and Zhang, R.: Responses of bacterial communities in arable soils in a rice-wheat cropping system to different fertilizer regimes and sampling times, PloS One, 9, e85301, https://doi.org/10.1371/journal.pone.0085301, 2014.
Zhong, Y., Hu, J., Xi, Q., Zhang, S., Li, X., Pan, X., Zhao, R., Wang, R., Yang, W., Shangguan, Z., Hu, F., Yang, C., and Wang, W.: Soil microbial mechanisms promoting ultrahigh rice yield, Soil Biol. Biochem., 143, 107741, https://doi.org/10.1016/j.soilbio.2020.107741, 2020.