the Creative Commons Attribution 4.0 License.
the Creative Commons Attribution 4.0 License.
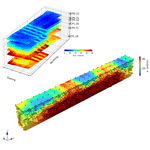
Uncovering soil compaction: performance of electrical and electromagnetic geophysical methods
Luca Peruzzo
Matteo Longo
Giorgio Cassiani
Francesco Morari
Monitoring soil structure is of paramount importance due to its key role in the critical zone as the foundation of terrestrial life. Variations in the arrangement of soil components significantly influence its hydro-mechanical properties and therefore its impact on the surrounding ecosystem. In this context, soil compaction resulting from inappropriate agricultural practices not only affects soil ecological functions, but also decreases the water-use efficiency of plants by reducing porosity and increasing water loss through superficial runoff and enhanced evaporation.
In this study, we compared the ability of electric and electromagnetic geophysical methods, i.e., electrical resistivity tomography (ERT) and frequency-domain electromagnetic (FDEM) method, to assess the effects caused by both heavy plastic soil deformations generated by a super-heavy vehicle and the more common tractor tramlines on silty-loam soils. We then tested correlations between geophysical response and soil variables (i.e., penetration resistance, bulk density, and volumetric water content on collected samples) at different homogeneous areas defined by k-means clustering.
This work is intended to be a contribution to clarify expectations about the use of geophysical techniques to rapidly investigate soil compaction at various spatial scales, dissecting their suitability and limitations. It also aims to contribute to the methodological optimization of agrogeophysical acquisitions and data processing in order to obtain accurate soil models through a non-invasive approach. Electrical prospecting has finer spatial resolution and allows a tomographic approach, requiring higher logistic demands and the need for ground galvanic contact. On the other hand, contactless electromagnetic induction methods can be quickly used to define the distribution of electrical conductivity in the shallow subsoil in an easier way. In general, compacted soil portions are imaged as high-electrical-conductivity anomalies relative to the context. Results, validated with traditional soil characterization, show the pros and cons of both techniques and how differences in their spatial resolution heavily influence the ability to characterize compacted areas with good confidence.
- Article
(7831 KB) - Full-text XML
- BibTeX
- EndNote
Soil is the foundation of terrestrial life, and its structure and dynamics result from the intertwining of biotic and abiotic factors, as well as the preponderance of human action. Recent developments in sensing technology, analysis methods, and data interpretation have paved the way for innovative approaches aimed at characterizing and safeguarding a wide spectrum of soil-based ecosystem services. Over the past decades, digital soil mapping has emerged as a transformative approach in soil science (McBratney et al., 2003), with the goal of enhancing our understanding of soil properties, state variables, agricultural processes, and moisture dynamics. The accessibility and affordability of ground-based and aerial sensor instruments have markedly improved, bringing high-resolution spatial–temporal data in support of traditional labor-intensive sampling techniques. Proximal and remote sensing techniques commonly rely on the use of instruments which can measure different portions of the electromagnetic spectrum, improving the understanding of processes governing the soil–plant–atmosphere continuum (Viscarra Rossel et al., 2011; Mulder et al., 2011). Software and hardware, mostly of a non-invasive nature, are continuously optimized for agronomic applications and also progressively deployed through airborne and uncrewed vehicles (von Hebel et al., 2021).
Applied geophysics plays a key role in this context, and its use has become increasingly assiduous (Romero-Ruiz et al., 2018; Garré et al., 2021). The preponderant methods employed here are based on the electrical properties of soil materials, which manifest main concomitant variations as the volumetric content and salinity of porous fluids change (Vereecken et al., 2007; Binley et al., 2015).
The frequency-domain electromagnetic (FDEM) method can be considered the cornerstone of electromagnetic sensors optimized for soil applications due to their fast logistics and user-friendliness (Doolittle and Brevik, 2014; Hanssens et al., 2019). By inducing electromagnetic fields underground and analyzing their interaction with the soil, this technique allows the electrical conductivity (EC) of large areas to be mapped non-invasively in short times (Boaga, 2017). This approach, especially if repeated over time, offers multifaceted advantages in the agronomic world, empowering farmers with information critical for precision agriculture practices (Corwin and Lesch, 2003; Lück et al., 2009). High-resolution soil variability and EC-derived moisture content and dynamics across the field allow us to implement precision irrigation strategies, tailoring water application to the specific moisture needs of different areas (Fortes et al., 2015; Serrano et al., 2020). However, while its application is relatively simple and quick, the FDEM method suffers from lower resolution than an in situ tomographic approach (e.g., electrical resistivity tomography, ERT; Lavoué et al., 2010; Von Hebel et al., 2014; Busato et al., 2019; Bernatek-Jakiel and Kondracka, 2022), and therefore detailed and more imperceptible spatial heterogeneities can escape at both the areal scale and especially the depth scale.
Electrical resistivity tomography has become a ubiquitous instrument in agricultural research due to its inherent robustness and demonstrated adaptability across a spectrum of applications and spatial scales (Garré et al., 2012; Cassiani et al., 2015; Mary et al., 2018; Blanchy et al., 2020b; Carrera et al., 2022). Surveys are conducted through multi-electrode devices to capture the spatial distribution of electrical resistivity in the subsurface, thereby facilitating the generation of comprehensive 2D and 3D models.
Soil EC is often used in the characterization of soil properties such as water content and salinity (Friedman, 2005a; Huang et al., 2017; Brevik et al., 2006), texture (Morari et al., 2009; Hanssens et al., 2019; Hubbard et al., 2021), and soil nutrients and organic content (Heiniger et al., 2003; Martinez et al., 2009) but also direct targeted sampling for detailed studies (Longo et al., 2020). Correlations between EC and soil properties, such as bulk density, porosity, and shear strength, are also used to identify soil compaction at the laboratory (Seladji et al., 2010) and field scales (Pentoś et al., 2021; Ren et al., 2022).
Soil compaction is a tangible manifestation of soil degradation. Heavy field traffic breaks down soil aggregates, altering the structure, limiting water and air infiltration, and reducing root penetration (Berisso et al., 2012; Schjønning et al., 2019). In recent years, modern agricultural machinery has increased considerably in size, and with it so has the compaction phenomenon (Raper, 2005; Nawaz et al., 2012). Its side effects have a significant impact on the soil ecosystem, particularly on hydrological regulation (i.e., surface runoff and reduced infiltration) and agronomic production (i.e., decreased yields), resulting in significant ecological and economic damage to the entire society (Bronick and Lal, 2004; Hamza and Anderson, 2005). Therefore, a correct understanding of the processes involved in soil compaction, as well as identification, and characterization, is necessary for prevention and to address future global challenges of sustainability and food security. This raises questions about the ability of geophysical methods to quantify the soil structure dynamics – including compaction – over space and time. Soil compaction exhibits highly spatial–temporal variability, depending on factors such as intensity and distribution of machinery traffic and/or the implementation of tillage practices (Alaoui and Diserens, 2018; Piccoli et al., 2022). Consequently, studying and mapping soil compaction with geophysical techniques also remains a challenge. Field evidence (Garciá-Tomillo et al., 2018; Mansourian et al., 2023) and modeling approaches (Romero-Ruiz et al., 2022) identify the compaction signature with increased EC. However, there is little exploration of resolution and sensitivity aspects of the techniques used, which in fact form the basis of all subsequent studies dealing with the acquired data.
In this work, we present the application of electrical (ERT) and electromagnetic (FDEM) geophysics, quantitatively integrated with traditional soil characterization techniques (i.e., penetration resistance, bulk density, and volumetric water content on collected samples) for the assessment of soil surface compaction (up to 0.4 m). The survey was conducted both at the areal level, covering 1.5 ha, and on detailed transects. This combination of measurements explores the importance of the survey design on the sensitivity of the method to soil compaction, as well as the 2D and 3D spatial heterogeneity that is often difficult to image using punctual information only. The study aims at (a) comparing the ability of ERT and FDEM to identify soil compaction, (b) quantifying the degree of compaction and its hydro-geophysical consequences caused by a common tractor and a hyper-heavy machine in a typical clayey soil, and (c) contributing towards the methodological optimization of agrogeophysical acquisitions and data processing, in order to obtain accurate soil models through a non-invasive approach. Results, validated with direct information, show the different ability of FDEM and ERT techniques to characterize compacted areas with good confidence, focusing on technical aspects and their spatial resolution.
2.1 Site description
The experiment was conducted in 2021 at the University of Padua's experimental farm “Lucio Toniolo”, located in Legnaro, northeastern Italy (45°21′ N, 11°57′ E; Fig. 1a). The investigation area presents a Fluvi-Calcaric Cambisol soil type (Anjos et al., 2014), characterized as a silt-loam soil with poor stratification and modest inherent fertility due to its limited organic carbon content (about 8–10 g kg−1 within the 0–0.2 m layer, declining to 0.5 g kg−1 at 0.6–0.9 m) and low cation exchange capacity (<20 cmol kg−1).
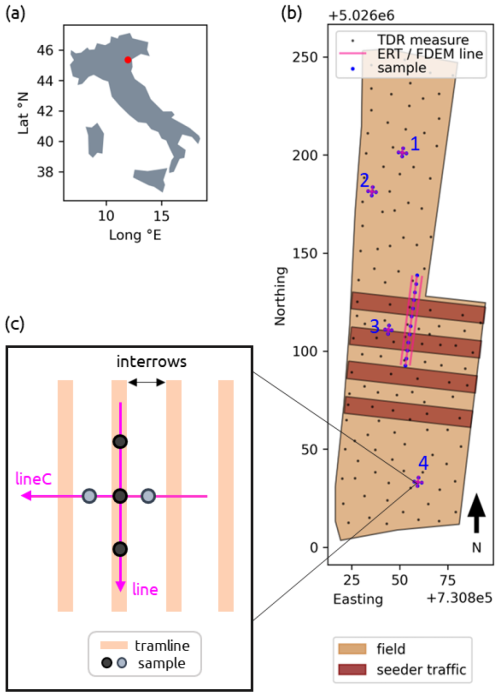
Figure 1(a) Site location and (b) experimental field with traffic pattern and survey design (small black dots: TDR (time-domain reflectometry) measurement; blue dots: samples; bright purple lines: ERT and FDEM transects). (c) Zoomed inset of transect orientation and sample position for all four detailed surveys.
The experiment was carried out in a bare soil area that is 60 m wide and 240 m long, for a total of 1500 m2 (Fig. 1b). In the past 60 years the field was used for arable cropping following conventional agricultural practices, which involved a moldboard plow at 0.3 m depth and disk harrowing before seeding. The main crops were maize (Zea mays L.), winter wheat (Triticum aestivum L.), soybean (Glycine max (L.) Merr.), sugar beet (Beta vulgaris L.), and Italian ryegrass (Lolium multiflorum Lam.). The soil was tilled as described above and then seeded (22 and 23 April 2021), either conventionally or by precision seeding. The vehicle used for common field works was a Fiat 680H of 2.8 t, while a precision seeding trial was performed with a Lemken Azurit 10 seed drill of 1.5 t mounted on a Fendt 718 tractor of around 8 t with frontally attached a localized fertilization system, totaling about 11 t. Each precision sowing event was performed with a single passage through the sowing steps highlighted in Fig. 1b. Data acquisition (fall season 2021) is described in detail in the following sections.
2.2 Frequency-domain electromagnetic method
The frequency-domain electromagnetic method utilizes time variations in electromagnetic fields at relatively low frequencies (∼1–100 kHz), and its functioning principle relies upon classical electromagnetic induction theory (McNeill, 1980; Deidda et al., 2014). Electromagnetic induction (EMI) instruments measure the interaction between an induced primary electromagnetic field and the resultant secondary electromagnetic field. During the measurement process, the transmitter coil emits a primary time-varying electromagnetic field (Hp) that induces eddy currents increasing with increasing ground EC. This complex network of eddy currents induces a secondary electromagnetic field (Hs), which is jointly sensed by the receiver coils. From these measurements, an apparent electrical conductivity (ECa) can be derived. Raw electrical conductivity values acquired through EMI surveys are apparent since they represent integrated values over depth. By varying the coil spacing or orientation, various subsurface depths can be obtained (Blanchy et al., 2024). Inverse methods need to be used to convert the ECa (as a function of either frequency or coil setup) to a depth profile of true EC (Von Hebel et al., 2019; McLachlan et al., 2021).
In this study, we adopted a CMD Mini-Explorer (GF Instruments), which contains three receiver coils with transmitter–receiver separation distances of 0.32, 0.71, and 1.18 m. The CMD was used in horizontal co-planar (HCP) and vertical co-planar (VCP) orientation, with respect to the ground, meaning that in total six depth-averaged readings were obtained for each measurement point (corresponding to the center of the instrument).
For an extensive survey, the device was pulled by a tractor using a 4 m long rope, placing the instrument on a dedicated wood sled at the soil surface. In this manner, no interaction with metallic (conductive) parts of the tractor or the sled was ensured. The travel speed was approximately 6 km h−1, with 0.5 s of sampling rate, ensuring a spatial sampling density of approximately 0.8 m. The parallel transects were set about 4 m apart from each other, covering an area of about 1.5 ha. For the high-resolution 2D transects, the device was hand-carried through its holding system at the soil surface, and the travel speed was that of a slow walk (approximately 3.5 km h−1). Measurements were logged every 0.5 s and paired with coordinates obtained from the ProXT GPS receiver (Trimble, USA). In this case, spatial sampling was approximately 0.5 m.
The measured data were filtered to remove outliers (values outside the mean ±2 standard deviations). Furthermore, a smoothing window was applied, replacing each data point with the average of its neighbors (number =5) to favor a smoother inversion process. Finally, to define the maximum depth of the models, sensitivity profiles for each survey have been calculated: all approximate zero toward 1.4 m below the surface. We set soil profiles composed of 24 layers with a thickness of 0.05 m each and initial EC of 10 mS m−1. Afterwards, the datasets were inverted using EMagPy (McLachlan et al., 2021), with the full Maxwell (FS) forward model (Wait, 1982) and the Gauss–Newton optimization method (McLachlan et al., 2021) in order to minimize the total misfit between observed values and predicted values from the forward model solution. The choice of the FS forward operator, instead of the more frequently adopted cumulative sensitivity (CS; McNeill, 1980), allows for the calculation of a non-simplified response of the ground. EMagPy has the capability to perform quasi-2D inversions, generating inverted EC depth profiles for each point of measurement, holding an average final root mean square percentage error (RMSPE) of 6.1 % for the eight cross transects and of 9.7 % for the three longer lines.
2.3 Electrical resistivity tomography
Electrical resistivity tomography is a well-established imaging technique and also long used in soil science (e.g., Samouëlian et al., 2005). ERT exploits multiple electrodes to measure the distribution of the electrical resistivity of the subsurface. Surveys are conducted through a quadrupole electrode arrangement: current is injected between a pair of electrodes, and the difference in electrical potential is measured between the other pair. From each measurement, an apparent resistivity value is derived, representing the equivalent resistivity of a homogenous subsurface. Given multiple combinations of current and potential electrodes along a transect, inverse modeling is then used to reconstruct a two- or three-dimensional image of the actual resistivity (Binley, 2015).
In this study, surveys were collected using a Syscal Pro 72 resistivimeter (Iris Instruments, Orleans, France) with an optimized dipole–dipole skip 0 scheme applied at 24 surface electrodes, acquiring both direct and reciprocal measurements, i.e., exchanging current and potentiometric electrodes for each quadrupole of measurement to get a statistical estimate of the experimental error (Binley et al., 1995; Cassiani et al., 2006). Two sets of ERT transects were acquired at different scales to enhance the comparison with the EM data (Fig. 1). A first set of three longer lines (2 m electrode spacing, above the seeder passages) was acquired to compare against plot-scale FDEM mapping. The second set of eight short cross transects (0.25 m electrode spacing) was acquired to match and compare with the high-resolution EMI transects. Stainless-steel electrodes were hammered into the first few centimeters (0.05 m) of the soil to achieve the best compromise that would ensure electrical contact and still abide by the general assumption of point-current injection. Contact resistances were checked before each acquisition, with very satisfactory values in the range 10−1–100 kΩ.
Along each line, 477 quadrupoles were acquired, adopting a current injection time of 250 ms per cycle, with minimum and maximum stack numbers (number of cycles per quadrupole) of 3 and 6, and a quality factor (acceptable difference between cycle results) Q=2 %. With these parameters, the total acquisition time for each line lasted around 8 min.
Datasets were analyzed in terms of direct-reciprocal deviation, removing the quadrupoles with discrepancy larger than 5 %, thus losing only a few dozen quadrupoles per line. The inversion process of the acquired datasets was performed adopting the same error threshold within the ResIPy software (Blanchy et al., 2020a), based on the R2 and R3t codes based on Occam's inversion method (Binley, 2015). All models converged within a maximum of two iterations, with a final RMS misfit of 1.0 each, thus confirming the excellent quality of data.
2.4 Soil sampling, penetration resistance, and TDR measurements
Both electrical and electromagnetic surveys were acquired with the intent to map but also to characterize in detail portions of the field: an initial areal FDEM acquisition was followed by three additional lines to intercept seeder heavy passages, as well as eight detailed transects, for both FDEM and ERT (four along and four across normal tractor tramlines; Fig. 1c).
Survey positions were identified according to the FDEM spatial variability of the soil. The areal FDEM survey was used to provide ancillary data that help identify homogenous areas. Transect data were spatially interpolated using an ordinary kriging approach, and a k-means clustering algorithm was used to identify homogenous areas on the FDEM kriged maps. The k-means algorithm divides M points in N dimensions into K clusters to minimize the within-cluster sum of squares (Hartigan and Wong, 1979). Both spatial interpolation and cluster analysis were performed using ArcGIS Pro (ESRI, Redlands, CA). The objective function is calculated using Eq. (1) (Gore, 2008),
where dih is the component of a distance matrix, obtained from Eq. (2),
where ch is the centroid of class h and C−1 is the inverse of covariance matrix of the independent variables, called the Mahalanobis distance (Varmuza and Filzmoser, 2016). The use of the Mahalanobis distance is justified by the fact that the six FDEM variables are correlated. The Calinski–Harabasz index (Caliñski and Harabasz, 1974) was used to select the optimal number of homogeneous areas, resulting in four clusters (Fig. 2). For each area, two geophysical detailed surveys (i.e., ERT and FDEM) were performed. Pairs of profiles were acquired, specifically four “lines” superimposed on the tramlines and four “lines C” crossing them orthogonally (Fig. 1c).
On top of each geophysical transect, three equally spaced penetration resistance (PR) sampling zones were selected, for a total of 24 in-depth profiles. Penetration resistance was measured at such points using a hydraulic-driven penetrologger (Eijkelkamp, Netherland), throughout the 0–0.8 m soil layer, with a 30°, 2 cm2 cone. Undisturbed 0.072 m diameter soil samples were collected down to 0.7 m at the corresponding PR locations (24 in total) using a hydraulic sampler. Undisturbed soil samples were weighed, and a fraction (two-thirds) was oven-dried at 105 °C for 24 h, to compute the volumetric water content (VWC) and bulk density (BD). The remaining soil fraction (one-third) was air-dried and sieved at 2 mm for texture analysis. Soil BD was estimated using the core method (Grossman and Reinsch, 2018), while soil texture was determined by laser diffraction (Mastersizer 2000; Malvern Panalytical Ltd, Malvern, UK), as described, e.g., in Bittelli et al. (2019). In addition, along the longer ERT midline, 12 PR depth profiles were acquired every 4 m. During the same day and just after the geophysical survey, the volumetric surface water content in the entire field was measured using 128 geolocated spatially distributed TDR measurements (FieldScout TDR 350 soil moisture meter, Spectrum Technologies, Inc.) equipped with 22 cm long spikes.
2.5 Statistical analysis
The level of dependency between soil electrical properties (i.e., ECFDEM, ECERT) and basic physical soil properties (i.e., soil moisture, texture, bulk density, and penetration resistance) was calculated by the non-parametric Spearman coefficients (rs). Indeed, EC soil properties showed a non-normal distribution, possibly implying non-linear relationships between the variables. This dynamic would not have been appreciable with Pearson's coefficient, thus motivating our choice. Depths down to 0.3 m of the simultaneously collected parameters were considered, given the predominant compaction of interest observed in the shallowest layer and a general homogenization of trends at depth. The statistical analysis was performed using a Python routine based on SciPy (Virtanen et al., 2020).
3.1 Areal FDEM survey and soil characterization
The initial areal FDEM survey clearly shows the presence of systematic conductive anomalies in the center of the field (Fig. 2a), i.e., four 70 m long corridors parallel to each other and approximately 8 m wide, with ECa values exceeding 30 mS m−1. In the rest of the field, ECa values are generally lower, around 6–10 mS m−1, with slightly more conductive portions (20–25 mS m−1) at the northern and southern extremes, where the field borders irrigation channels. The spatial variability just described in the shallowest layer (VCP0.32) propagates along the investigation depth with a very similar pattern, with conductivity ranges gradually increasing with depth. In the bottom layer (HCP1.18), a maximum increase of approximately 15 mS m−1 is observed, with values exceeding 40 mS m−1 in the most conductive zones and around 20–25 mS m−1 elsewhere. This lateral and anomalous heterogeneity motivated the clustering process that led to the identification of the four distinct homogeneous areas to investigate further and carry out soil sampling (Fig. 3). Specifically, parallel patterns were grouped in the center of the monitored field. Less regular patterns were identified at the northern and southern parts of the field. Area no. 3 is the largest one (4745 m2), while area no. 4 is the smallest one (1876 m2).
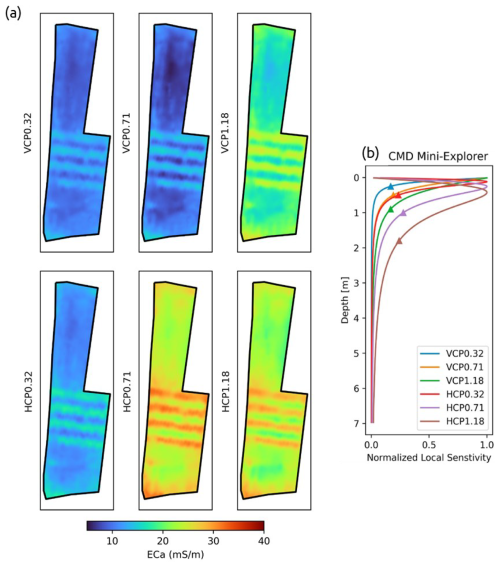
Figure 2(a) Apparent electrical conductivity (ECa) field maps obtained from the initial areal FDEM survey through the CMD Mini-Explorer, showing the systematic conductive seeder anomalies for each coil configuration (VCP and HCP probe orientation and Tx–Rx coil separation) and (b) their normalized local sensitivity pattern (from McLachlan et al., 2021).
Figure 4 shows the VWC map, obtained from kriging the point values measured over the entire field using the portable TDR instrument. We can clearly observe a spatial pattern very similar to ECa (Fig. 2a), characterized by the presence of systematic anomalies in the center of the field. In this case, these are portions with high water content (>30 %), arranged on parallel bands 8 m wide and the length of the field. A further area with fairly large water content (>25 %) is located in the lower-left corner, while the remaining field portions settle at values between 5 % and 20 %, with an average of 16.4 %.
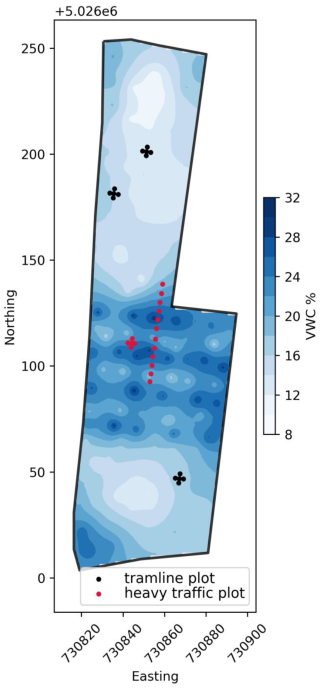
Figure 4VWC map obtained by kriging of punctual TDR measurements. Samples are indicated with different colors according to the treatment.
Seeder heavy traffic determined significantly different soil bulk density values (significance level p<0.05) compared to the rest of the field in the upper soil layers (0–0.2 m). This treatment exhibits increased BD, with average values of 1.53 g cm−3, respectively, compared to the rest of the field that averages 1.41 g cm−3 (Fig. 5a). Along the 0.2–0.7 m depth profile, bulk density becomes similar between treatments, within the range of 1.56–1.58 g cm−3. The VWC shows slight differences among the treatments within the shallowest 0.2 m. The average values in this depth range are 0.26 m3 m−3 for heavy-traffic regions and 0.24 m3 m−3 for uncompacted regions, whereas below 0.2 m depth the profiles become similar, with values averaging 0.25 m3 m−3. In terms of penetration resistance, the heavy-traffic regions show significantly higher resistances than the uncompacted regions throughout the depth range. Down to a depth of 0.3 m, the average penetration resistance for the non-compacted soil is 1.26 MPa, while it increased to an average of 4.9 MPa in compacted areas. Even at greater depths, beyond 0.4 m, seeder traffic shows significantly higher values, with average PR values more than 2.7 MPa higher than in the uncompacted area.
From the analyses of clusters 1, 2, and 4 (Fig. 5b), a difference is shown between the interrow and the tramline in the shallowest 0.2 m. Both PR and BD measured on the tramlines are higher (mean values of 1.6 MPa and 1.49 g cm−3, respectively) than those on the rows (mean values of 1.0 MPa and 1.41 g cm−3). Deeper than the topsoil (>0.3 m), the profiles tend to become uniform, with monotonous growth downward. VWC profile shows higher values for the tramline than for the interrows in the shallowest 0.1 m (0.25 kg kg−1 for tramline and 0.23 kg kg−1 for interrows), while below that the behavior changes with a kind of slightly reversed trend.
3.2 Electromagnetic and DC-current geophysics
The three inverted transects, both FDEM and ERT, acquired across the heavy seeder traffic passages (Fig. 6), show a common pattern, consistent with the geophysical aerial survey. Two highly conductive anomalies (>30 mS m−1) are clearly visible in the uppermost portion of the subsoil (down to 0.5 m), located between 16 and 24 m and between 29 and 38 m along the transect direction. In the same depth range, the remaining portions of the investigated profile have an average EC value of about 9 mS m−1. Moving to greater depths (>0.8 m), conductivity values increase and become laterally uniform, around 40 mS m−1. To note, the ERT transects were inverted together to generate a pseudo-3D model (Fig. 6b). In this way, the spatial extent of the systematic conductive anomalies described above could be better imaged. In addition, the 2 m electrode spacing of the ERT lines made it possible to extend the depth of investigation to approximately 6 m. However, beyond 2 m from ground level, the tomograms become uniform at values greater than 50 mS m−1, as already found in the FDEM models (Fig. 6a).
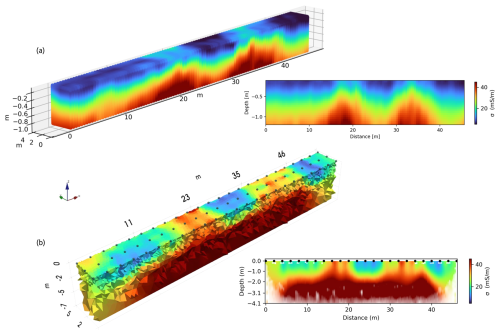
Figure 6Pseudo-3D inverted models obtained from the inversion of the three single lines, (a) FDEM and (b) ERT, over the heavy-traffic area.
As for the detailed survey, carried out in the four cluster areas (Fig. 3), pairs of profiles were acquired: four “lines” were superimposed on the tramlines and four “lines C” crossed them orthogonally (Figs. 7 and 8). Figure 7 shows relatively homogeneous FDEM models, with conductivities in the range of (1–50) mS m−1. Except for cluster 3, the remaining models agree in showing lower conductivity values (1–20 mS m−1) in the shallow topsoil (0–0.5 m), gradually increasing with depth (>30 mS m−1) down to approximately 1.4 m. Lines 3 and 3C, acquired close to heavy-traffic pathways (see Fig. 1b), deviate from the monotonic trend just described, showing more pronounced conductivity values (>40 mS m−1) at shallower depths than the previous lines. In particular, high ECs are observed as shallow as 0.3 m depth in the second portion of line3, close to the seeder passage.
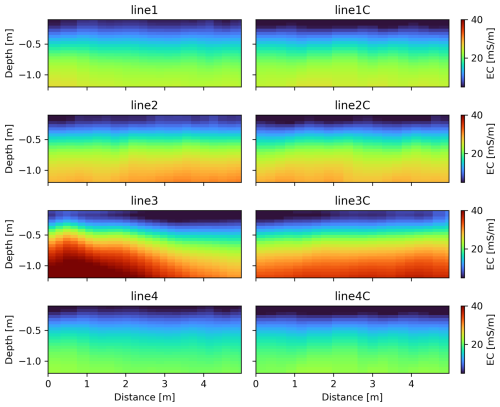
Figure 7Electrical conductivity models after FSlin inversion of FDEM transects. The numbering is relative to the related cluster; “line” is superimposed on the tramline and “line C” crosses it orthogonally.
ERT inverted models reflect the similarity between clusters 1, 2, and 4 and the deviation of cluster 3 (Fig. 8). As regards the former ensemble, a horizontal layer with higher conductivity (>40 mS m−1) with a rather homogeneous thickness is found in the uppermost portion (0–0.1 m). Below this, the EC values decrease (10–20 mS m−1), showing basically uniform models. The reduced spacing between the electrodes, their small number, and the adopted dipole–dipole skip 0 sequence contributes to increase the resolution but limits the investigation depth to only 1 m. In the transversal lines (Fig. 1), the same degree of EC homogeneity is found at depth. However, circumscribed conductive bulbs (>40 mS m−1) can be observed at the surface (0–0.1 m). They are placed at progressive distances of approximately 1, 3, and 4.5 m, coinciding with the three tramlines intersected by these cross profiles.
3.3 Primary soil properties and electromagnetic behavior
When analyzing the relationships between primary soil parameters, a significant, though weak, correlation was found between PR and BD (rs=0.23), and a moderate correlation was found between VWC and BD (rs=0.43, p<0.01), while no dependency was found between the texture and other physical properties (Fig. 9a). VWC was also correlated with electrical conductivity in topsoil, weakly with ECFDEM (rs=0.28) and moderately with ECERT (rs=0.32, Fig. 9a). Similarly, conductivity demonstrated moderate significant correlations with PR (rs=0.41 and 0.39 for ECFDEM and ECERT, respectively), whereas only ECERT exhibits a positive correlation with BD (rs=0.32), differing from ECFDEM in this case.
An EC dependency from VWC was also found when the moisture content of the field topsoil (down to 0.2 m) was considered. The VWC measured with portable TDR in 128 randomly distributed samples (Fig. 1c) strongly influenced the areal electromagnetic response (rs=0.78), as reported in Fig. 9b.
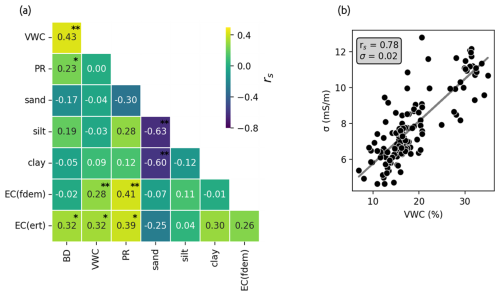
Figure 9(a) Heatmap displaying the Spearman correlation coefficients (rs) between the measured variables (BD: bulk density; VWC: volumetric water content; PR: penetration resistance, sand, silt, and clay; EC(fdem): electrical conductivity from FDEM; EC(ert): electrical conductivity from ERT). The asterisks identify significant relationships, * at p<0.05 and at p<0.01. (b) Spearman's correlation between volumetric moisture content (measured with TDR) and ECFDEM from the areal survey.
This work explores the capabilities of ERT and FDEM to discriminate soil compaction in the field, at both aerially extended and detailed scales, and provides some methodological insights to optimize geophysical acquisition for this specific goal. Since EC is a parameter influenced by multiple soil properties (Friedman, 2005a; Doolittle and Brevik, 2014), it is useful to explore its correlation with soil bulk density, penetration resistance, and volumetric water content in order to improve soil management and monitoring of agricultural practices.
As a starting point, we utilized BD and PR as indicators of the state of compaction. Soil BD is the mass of dry soil per unit volume obtained from a sample, while PR is measured in the field as the result of the cohesive forces between the individual soil particles and the frictional resistance encountered by the particles sliding over each other (Marshall and Holmes, 1980). Although often affected by punctual heterogeneity when measured in the field, PR remains an effective indicator of soil compaction (Benevenute et al., 2020). A poorly structured soil is characterized by high BD values (Håkansson and Lipiec, 2000), and this often happens with the repeated passage of heavy vehicles in unfavorable field conditions. As in our case, the portions of soil heavily compacted on the surface for heavy seeder passes show very high BD (>1.55 g cm−3) and PR (>4.5 MPa). Even when considering the case of common tramline compaction, the values are higher than uncompacted regions by roughly 1.5 g cm−3 and 2.2 MPa, respectively, in line with findings of Reintam et al. (2009) and Elaoud and Chehaibi (2011) for this type of soil.
Taking VWC into consideration as well, an increase in moisture is observed in the compacted subsurface portions. The behavior is definitely evident in the areal mapping performed with TDR (Fig. 4), but it is also observed from the samples collected from the 0–0.3 m depth layer (rs=0.43 with BD, Fig. 9b). This phenomenon is generated where the percolation rate of rainwater or irrigation water through the soil is reduced by a compacted layer, thus developing localized saturated zones close to the soil surface (Batey and McKenzie, 2006).
This aspect is of paramount importance when evaluating the geophysical response, particularly considering the electromagnetic nature of the methods here (and commonly) adopted. Indeed, they rely on electromagnetic properties of the subsurface, which change dramatically with water content (Binley et al., 2015; Vereecken et al., 2007). In fact, a positive correlation was observed between electrical conductivity and soil moisture in the areal survey (rs=0.78 for ECFDEM, Fig. 9a) but also a significant relationship by examining soil samples (rs=0.28 and 0.32 for ECFDEM and ECERT respectively, Fig. 9b). In both areal and detailed surveys, the highly compacted portions of the soil are characterized by high-EC anomalies relative to the context, likely caused by their low permeability and surface saturation. This aspect may therefore be indicative of soil compaction if electromagnetic surveys are used as an initial monitoring tool. In general, the conductivities found are in the range below 50 mS m−1, in good agreement with the agricultural context of the area. FDEM models agree in showing lower values (below 10 mS m−1) in the shallowest soil layer (0–0.1 m), likely due to drier conditions, with a gradual increase with depth (>30 mS m−1), related to an increase in water content (Fig. 7). This dynamic is also evident in the pseudo-3D models in Fig. 6, for both ERT and FDEM results. Note that the circumscribed and systematic surface conductive anomalies present here (>40 mS m−1) are precisely placed on the seeding corridors that compacted the first subsurface.
The high-resolution ERT transects are informative down to depths of about 1 m (Fig. 8), as they are designed to achieve a high resolution close to the surface, where they show localized conductive anomalies (about 0.3 m wide, 0.15 m thick) in correspondence with the compaction generated on the tramlines. This resolving ability is not found in FDEM transects, and this is motivated by the very nature of the techniques: the electromagnetic induction approach generates vertical soundings with integrated ECa values over depth (McNeill, 1980), and the output models are strictly dependent on the instrument footprint, the spatial density of the measurements, and the need to smooth the measured data during processing and inversion. Given the adopted instrument, it is expected that its footprint be no smaller than a square meter. On the contrary, ERT can be adapted to the scale and resolution needed to optimally investigate the phenomenon of interest with a tomographic approach. The different surface sensitivity of the two methods to compaction is also confirmed by statistical analysis: ECERT shows a significant correlation with BD (rs=0.32), whereas ECFDEM is even slightly negative (). One last thing to consider, in this specific field of study concerned with the most proximal portion of the subsurface (down to 1–2 m), is the critical effect of a correct placing of the electrodes: they should not penetrate too far into the soil to ensure that the assumption of punctual current injection is satisfied, given the commonly small spacings; however, at the same time, good coupling with the plowed and aerated soil must be ensured, minimizing contact resistances.
The advantages of FDEM instruments are considerable – ease of use and speed of acquisition first and foremost. The equipment is commonly lightweight (a CMD Mini-Explorer probe weighs 2 kg), and measurements are collected in a contactless manner by simply carrying the device over the target area, walking, or dragging it. With little effort and in less than 2 h, the ECa distribution in the shallow subsurface can be acquired over about 2 ha, thus highlighting portions with high compaction and surface moisture. However, as shown here but also noted by Blanchy et al. (2024), detailed spatial heterogeneities at both areal and depth scales can be missed by FDEM instruments. Other important aspects during acquisition are (a) avoiding the nearby presence of metal objects that can produce spurious results and (b) checking the potential instrumental drift (De Smedt et al., 2016). In addition, it is crucial to remember that in the presence of conductive soil, most of the signal at higher frequencies is conveyed, via electromagnetic induction, into the topmost layer and does not diffuse into the subsoil, thus decreasing the depth of investigation. Therefore, although ERT requires more logistical effort and the need for galvanic contact with the soil, it remains a technique of fundamental application for obtaining a more accurate subsurface model with sufficient spatial resolution. In this respect, the existence of new georesistivimeters (i.e., Syscal Terra, Iris Instruments) capable of collecting datasets with moving streamer systems could generate a breakthrough to obtain truly 3D field electrical conductivity models.
Our results show a good correlation between FDEM and ERT in highlighting the compacted and saturated portions of the soil, with some differences. Despite its potential and widespread application, the use of electromagnetic geophysics in agriculture presents challenges, primarily related to the complex and site-specific influences on soil EC (Friedman, 2005b; Carroll and Oliver, 2005; Kühn et al., 2009). Survey resolution, adaptability, and ease of use are all paramount. Also, soil electrical conductivity depends on soil type, moisture content, and texture, highlighting the complexity inherent in understanding which factor is predominant in a site-specific situation. Identifying compaction through EC measurements, therefore, is not a trivial task. Variability in field conditions, sensor calibration, and data interpretation are critical considerations. This requires multi-parameter approaches that incorporate direct point measurements (e.g., bulk density, porosity and permeability, soil penetration resistance; Johnson and Bailey, 2002; Keller et al., 2021) and auxiliary data like historical land use. However, the spatial extent and resolution permitted by non-invasive geophysical methods is a great advantage over other local measurement methods, and increased efforts shall be devoted towards improving the accuracy of such techniques in identifying soil compaction, as recently done with shallow seismic methods (Carrera et al., 2024), along with other parameters important in soil management.
In this work, we compare the efficiency of the two geophysical techniques most used in agronomy, i.e., ERT and FDEM, and specifically for the characterization of soil compaction. We specifically explore the sensitivity and resolution of these methods in assessing shallow soil compaction in the field, at both the plot scale and the submeter-tramline scale.
FDEM allows rapid acquisition of measurements that can define spatial variability at the ground surface, which motivates its appreciation in soil science and agronomy. Nevertheless, it must be noted that a rigorous acquisition protocol must be applied in order to avoid potential instrumental drift and other issues, e.g., related to local strong conductors, and, therefore, scattered or negative values and local conductivity anomalies unrelated to soil structure. Moreover, FDEM inversion is for now a purely one-dimensional process with depth, with lower spatial resolution as compared to ERT. In addition, FDEM has an intrinsic spatial scale linked to the coil distance and used frequency, which can hardly achieve a resolution finer than the meter scale.
Due to its accuracy and flexibility of application, the ERT method is well established and widespread as well in agronomy. However, it also requires a rigorous approach to ensure the desired resolution and reliability of results. The type of measurement sequence, site- and target-specific, is known to be critical, and reciprocal data acquisition is strongly recommended since it allows an assessment of the quality of the collected dataset and therefore provides tools to minimize possible artifacts in the reconstructed subsurface models.
Future challenges must address the increase in spatial resolution and sampling potential of electromagnetic induction instruments, as well as the development of algorithms that could allow a true 3D inversion of the spatially measured data. At the same time, the use of next-generation georesistivimeters capable of collecting datasets with moving streamer systems represents a great opportunity to be tested in the field. Technology advances are visible in this direction, and this will foster precision agriculture practices but also a broader understanding of soil–plant–water interactions and ecosystem dynamics.
Data used to obtain the results presented in this work can be accessed on the Zenodo open-source repository (https://doi.org/10.5281/zenodo.11356672, Carrera, 2024).
Conceptualization: AC. Methodology: AC, GC, and FM. Validation: GC. Investigation: AC. Data curation: AC, ML, and LP. Writing (original draft preparation): AC. Writing (review and editing): AC, ML, LP, GC, and FM. Funding acquisition: FM.
The contact author has declared that none of the authors has any competing interests.
This paper reflects only the authors' views and opinions; neither the European Union nor the European Commission can be considered responsible for them.
Publisher’s note: Copernicus Publications remains neutral with regard to jurisdictional claims made in the text, published maps, institutional affiliations, or any other geographical representation in this paper. While Copernicus Publications makes every effort to include appropriate place names, the final responsibility lies with the authors.
This article is part of the special issue “Agrogeophysics: illuminating soil's hidden dimensions”. It is not associated with a conference.
The authors thank Franco Gasparini, Riccardo Polese, and Ilaria Piccoli for their valuable support and fieldwork assistance. Moreover, we are very grateful to editors Alejandro Romero-Ruiz and Kristof Van Oost as well as the three anonymous reviewers for their valuable comments, which improved the original manuscript.
The research that led to these results received funding from the European Union's Horizon 2020 and the MIPAAF (Ministry of Agricultural Food and Forestry Policies) research and innovation program (grant agreement no. 862665 ICTAGRI-FOOD, SoCoRisk project), the Agritech National Research Center (Spoke 4, WP4.3), the European Union Next Generation EU (Piano Nazionale di Ripresa e Resilienza (PNRR) – Missione 4 Componente 2, Investimento 1.4 – D.D. 1032 17/06/2022, CN00000022), and the Geosciences for Sustainable Development project (Budget Ministero dell'Università e della Ricerca-Dipartimenti di Eccellenza 2023–2027 C93C23002690001).
This paper was edited by Alejandro Romero-Ruiz and reviewed by Sashini Pathirana and two anonymous referees.
Alaoui, A. and Diserens, E.: Mapping soil compaction – A review, Curr. Opin. Environ. Sci. Heal., 5, 60–66, https://doi.org/10.1016/j.coesh.2018.05.003, 2018.
Anjos, L., Gaistardo, C., Deckers, J., Dondeyne, S., Eberhardt, E., Gerasimova, M., Harms, B., Jones, A., Krasilnikov, P., Reinsch, T., Vargas, R., and Zhang, G.: World reference base for soil resources 2014 International soil classification system for naming soils and creating legends for soil maps, edited by: Schad, P., Van Huyssteen, C., and Micheli, E., Rome (Italy), FAO, 2015, JRC91947, https://publications.jrc.ec.europa.eu/repository/handle/JRC91947, 2014.
Batey, T. and McKenzie, D. C.: Soil compaction: Identification directly in the field, Soil Use Manag., 22, 123–131, https://doi.org/10.1111/j.1475-2743.2006.00017.x, 2006.
Benevenute, P. A. N., de Morais, E. G., Souza, A. A., Vasques, I. C. F., Cardoso, D. P., Sales, F. R., Severiano, E. C., Homem, B. G. C., Casagrande, D. R., and Silva, B. M.: Penetration resistance: An effective indicator for monitoring soil compaction in pastures, Ecol. Indic., 117, 106647, https://doi.org/10.1016/j.ecolind.2020.106647, 2020.
Berisso, F. E., Schjønning, P., Keller, T., Lamandé, M., Etana, A., De Jonge, L. W., Iversen, B. V, Arvidsson, J., and Forkman, J.: Persistent effects of subsoil compaction on pore size distribution and gas transport in a loamy soil, Soil Till. Res., 122, 42–51, https://doi.org/10.1016/j.still.2012.02.005, 2012.
Bernatek-Jakiel, A. and Kondracka, M.: Detection of soil pipe network by geophysical approach: Electromagnetic induction (EMI) and electrical resistivity tomography (ERT), Land Degrad. Dev., 33, 1002–1014, https://doi.org/10.1002/ldr.4205, 2022.
Binley, A.: Tools and Techniques: Electrical Methods, Elsevier B.V., 233–259, https://doi.org/10.1016/B978-0-444-53802-4.00192-5, 2015.
Binley, A., Ramirez, A., and Daily, W.: Regularised image reconstruction of noisy electrical resistance tomography data, in: Process Tomography, 401–410, 1995.
Binley, A., Hubbard, S. S., Huisman, J. A., Revil, A., Robinson, D. A., Singha, K., and Slater, L. D.: The emergence of hydrogeophysics for improved understanding of subsurface processes over multiple scales, 51, 3837–3866, https://doi.org/10.1002/2015WR017016, 2015.
Bittelli, M., Andrenelli, M. C. C., Simonetti, G., Pellegrini, S., Artioli, G., Piccoli, I., and Morari, F.: Shall we abandon sedimentation methods for particle size analysis in soils?, Soil Till. Res., 185, 36–46, https://doi.org/10.1016/j.still.2018.08.018, 2019.
Blanchy, G., Saneiyan, S., Boyd, J., McLachlan, P., and Binley, A.: ResIPy, an intuitive open source software for complex geoelectrical inversion/modeling, Comput. Geosci., 137, 104423, https://doi.org/10.1016/j.cageo.2020.104423, 2020a.
Blanchy, G., Virlet, N., Sadeghi-Tehran, P., Watts, C. W., Hawkesford, M. J., Whalley, W. R., and Binley, A.: Time-intensive geoelectrical monitoring under winter wheat, Near Surf. Geophys., 18, 413–425, https://doi.org/10.1002/nsg.12107, 2020b.
Blanchy, G., Mclachlan, P., Mary, B., Censini, M., Boaga, J., and Cassiani, G.: Comparison of multi-coil and multi-frequency frequency domain electromagnetic induction instruments, Front. Soil Sci., 4, 1239497, https://doi.org/10.3389/fsoil.2024.1239497, 2024.
Boaga, J.: The use of FDEM in hydrogeophysics: A review, J. Appl. Geophys., 139, 36–46, https://doi.org/10.1016/j.jappgeo.2017.02.011, 2017.
Brevik, E. C., Fenton, T. E., and Lazari, A.: Soil electrical conductivity as a function of soil water content and implications for soil mapping, Precis. Agric., 7, 393–404, https://doi.org/10.1007/s11119-006-9021-x, 2006.
Bronick, C. J. and Lal, R.: Soil structure and management: a review, 124, 3–22, https://doi.org/10.1016/j.geoderma.2004.03.005, 2004.
Caliñski, T. and Harabasz, J.: A Dendrite Method Foe Cluster Analysis, Commun. Stat., 3, 1–27, https://doi.org/10.1080/03610927408827101, 1974.
Carrera, A.: AlbCa/Electrocomp_SOIL: v1.0.0 (v1.0.0), Zenodo [data set], https://doi.org/10.5281/zenodo.11356672, 2024.
Carrera, A., Longo, M., Piccoli, I., Mary, B., Cassiani, G., and Morari, F.: Electro-Magnetic Geophysical Dynamics under Conservation and Conventional Farming, Remote Sens., 14, 6243, https://doi.org/10.3390/rs14246243, 2022.
Carrera, A., Pavoni, M., Barone, I., Boaga, J., Ferro, N. D., Cassiani, G., and Morari, F.: Assessment of different agricultural soil compaction levels using shallow seismic geophysical methods, Geoderma, 447, 116914, https://doi.org/10.1016/J.GEODERMA.2024.116914, 2024.
Carroll, Z. L. and Oliver, M. A.: Exploring the spatial relations between soil physical properties and apparent electrical conductivity, Geoderma, 128, 354–374, https://doi.org/10.1016/J.GEODERMA.2005.03.008, 2005.
Cassiani, G., Bruno, V., Villa, A., Fusi, N., and Binley, A. M.: A saline trace test monitored via time-lapse surface electrical resistivity tomography, J. Appl. Geophys., 59, 244–259, https://doi.org/10.1016/j.jappgeo.2005.10.007, 2006.
Cassiani, G., Boaga, J., Vanella, D., Perri, M. T., and Consoli, S.: Monitoring and modelling of soil–plant interactions: the joint use of ERT, sap flow and eddy covariance data to characterize the volume of an orange tree root zone, Hydrol. Earth Syst. Sci., 19, 2213–2225, https://doi.org/10.5194/hess-19-2213-2015, 2015.
Corwin, D. L. and Lesch, S. M.: Application of soil electrical conductivity to precision agriculture: Theory, principles, and guidelines, in: Agronomy Journal, 455–471, https://doi.org/10.2134/agronj2003.4550, 2003.
Deidda, G. P., Fenu, C., and Rodriguez, G.: Regularized solution of a nonlinear problem in electromagnetic sounding, Inverse Probl., 30, 125014, https://doi.org/10.1088/0266-5611/30/12/125014, 2014.
De Smedt, P., Delefortrie, S., and Wyffels, F.: Identifying and removing micro-drift in ground-based electromagnetic induction data, J. Appl. Geophys., 131, 14–22, https://doi.org/10.1016/J.JAPPGEO.2016.05.004, 2016.
Doolittle, J. A. and Brevik, E. C.: The use of electromagnetic induction techniques in soils studies, 223–225, 33–45, https://doi.org/10.1016/j.geoderma.2014.01.027, 2014.
Elaoud, A. and Chehaibi, S.: Soil compaction due to tractor traffic, 11, 539–545, https://doi.org/10.1007/s11668-011-9479-3, 2011.
Fortes, R., Millán, S., Prieto, M. H., and Campillo, C.: A methodology based on apparent electrical conductivity and guided soil samples to improve irrigation zoning, Precis. Agric., 16, 441–454, https://doi.org/10.1007/s11119-015-9388-7, 2015.
Friedman, S. P.: Soil properties influencing apparent electrical conductivity: A review, Comput. Electron. Agric., 46, 45–70, https://doi.org/10.1016/j.compag.2004.11.001, 2005a.
Friedman, S. P.: Soil properties influencing apparent electrical conductivity: A review, Comput. Electron. Agric., 46, 45–70, https://doi.org/10.1016/j.compag.2004.11.001, 2005b.
Garciá-Tomillo, A., Figueiredo, T. De, Dafonte, J. D., Almeida, A., and Paz-González, A.: Effects of machinery trafficking in an agricultural soil assessed by Electrical Resistivity Tomography (ERT), Open Agric., 3, 378–385, https://doi.org/10.1515/opag-2018-0042, 2018.
Garré, S., Günther, T., Diels, J., and Vanderborght, J.: Evaluating Experimental Design of ERT for Soil Moisture Monitoring in Contour Hedgerow Intercropping Systems, Vadose Zone J., 11, vzj2011.0186, https://doi.org/10.2136/vzj2011.0186, 2012.
Garré, S., Hyndman, D., Mary, B., and Werban, U.: Geophysics conquering new territories: The rise of “agrogeophysics,” Vadose Zone J., 20, e20115, https://doi.org/10.1002/VZJ2.20115, 2021.
Gore, S. D.: Sampling for Natural Resource Monitoring, J. Am. Stat. Assoc., 103, 889–890, https://doi.org/10.1198/jasa.2008.s240, 2008.
Grossman, R. B. and Reinsch, T. G.: The Solid Phase: Bulk Density and Linear Extensibility, Methods Soil Anal. Part 4 Phys. Methods, 201–228, https://doi.org/10.2136/SSSABOOKSER5.4.C9, 2018.
Håkansson, I. and Lipiec, J.: A review of the usefulness of relative bulk density values in studies of soil structure and compaction, Soil Till. Res., 53, 71–85, https://doi.org/10.1016/S0167-1987(99)00095-1, 2000.
Hamza, M. A. and Anderson, W. K.: Soil compaction in cropping systems: A review of the nature, causes and possible solutions, Soil Till. Res., 82, 121–145, https://doi.org/10.1016/J.STILL.2004.08.009, 2005.
Hanssens, D., Delefortrie, S., Bobe, C., Hermans, T., and De Smedt, P.: Improving the reliability of soil EC-mapping: Robust apparent electrical conductivity (rECa) estimation in ground-based frequency domain electromagnetics, Geoderma, 337, 1155–1163, https://doi.org/10.1016/j.geoderma.2018.11.030, 2019.
Hartigan, J. A. and Wong, M. A.: Algorithm AS 136: A K-Means Clustering Algorithm, Appl. Stat., 28, 100, https://doi.org/10.2307/2346830, 1979.
Heiniger, R. W., McBride, R. G., and Clay, D. E.: Using soil electrical conductivity to improve nutrient management, Agron. J., 508–519, https://doi.org/10.2134/agronj2003.5080, 2003.
Huang, J., Kilminster, T., Barrett-Lennard, E. G., and Triantafilis, J.: Characterization of field-scale dryland salinity with depth by quasi-3d inversion of DUALEM-1 data, Soil Use Manage., 33. 205–215, https://doi.org/10.1111/sum.12345, 2017.
Hubbard, S. S., Schmutz, M., Balde, A., Falco, N., Peruzzo, L., Dafflon, B., Léger, E., and Wu, Y.: Estimation of soil classes and their relationship to grapevine vigor in a Bordeaux vineyard: advancing the practical joint use of electromagnetic induction (EMI) and NDVI datasets for precision viticulture, Precis. Agric., 22, 1353–1376, https://doi.org/10.1007/s11119-021-09788-w, 2021.
Johnson, E. C. and Bailey, A. C.: Soil Compaction, Adv. Soil Dyn., 2, 155, https://doi.org/10.13031/2013.9452, 2002.
Keller, T., Lamandé, M., Naderi-Boldaji, M., and de Lima, R. P.: Soil Compaction Due to Agricultural Field Traffic: An Overview of Current Knowledge and Techniques for Compaction Quantification and Mapping, in: Advances in Understanding Soil Degradation, Springer, Cham, 287–312, https://doi.org/10.1007/978-3-030-85682-3_13, 2021.
Kühn, J., Brenning, A., Wehrhan, M., Koszinski, S., and Sommer, M.: Interpretation of electrical conductivity patterns by soil properties and geological maps for precision agriculture, Precis. Agric., 10, 490–507, https://doi.org/10.1007/s11119-008-9103-z, 2009.
Lavoué, F., Van Der Kruk, J., Rings, J., André, F., Moghadas, D., Huisman, J. A., Lambot, S., LWeihermüller, Vanderborght, J., and Vereecken, H.: Electromagnetic induction calibration using apparent electrical conductivity modelling based on electrical resistivity tomography, Near Surf. Geophys., 8, 553–561, https://doi.org/10.3997/1873-0604.2010037, 2010.
Longo, M., Piccoli, I., Minasny, B., and Morari, F.: Soil apparent electrical conductivity-directed sampling design for advancing soil characterization in agricultural fields, Vadose Zone J., 19, 1–14, https://doi.org/10.1002/vzj2.20060, 2020.
Lück, E., Gebbers, R., Ruehlmann, J., and Spangenberg, U.: Electrical conductivity mapping for precision farming, Near Surf. Geophys., 7, 15–25, https://doi.org/10.3997/1873-0604.2008031, 2009.
Mansourian, D., Vanderhasselt, A., Cornelis, W., and Hermans, T.: Response to soil compaction of the electrical resistivity tomography, induced polarisation, and electromagnetic induction methods: a case study in Belgium, Soil Res., 62, SR22260, https://doi.org/10.1071/sr22260, 2023.
Marshall, T. J. and Holmes, J. W.: Soil physics, Soil Phys., Cambridge University Press, 1980.
Martinez, G., Vanderlinden, K., Ordóñez, R., and Muriel, J. L.: Can Apparent Electrical Conductivity Improve the Spatial Characterization of Soil Organic Carbon?, Vadose Zone J., 8, 586–593, https://doi.org/10.2136/vzj2008.0123, 2009.
Mary, B., Peruzzo, L., Boaga, J., Schmutz, M., Wu, Y., Hubbard, S. S., and Cassiani, G.: Small-scale characterization of vine plant root water uptake via 3-D electrical resistivity tomography and mise-à-la-masse method, Hydrol. Earth Syst. Sci., 22, 5427–5444, https://doi.org/10.5194/hess-22-5427-2018, 2018.
McBratney, A. B., Mendonça Santos, M. L., and Minasny, B.: On digital soil mapping, Geoderma, 117, 3–52, https://doi.org/10.1016/S0016-7061(03)00223-4, 2003.
McLachlan, P., Blanchy, G., and Binley, A.: EMagPy: Open-source standalone software for processing, forward modeling and inversion of electromagnetic induction data, Comput. Geosci., 146, 104561, https://doi.org/10.1016/j.cageo.2020.104561, 2021.
McNeill, J.: Electromagnetic terrain conductivity measurement at low induction numbers, Geonics Ltd., Technical Note TN-6, 1980.
Morari, F., Castrignanò, A., and Pagliarin, C.: Application of multivariate geostatistics in delineating management zones within a gravelly vineyard using geo-electrical sensors, Comput. Electron. Agr., 68, 97–107, https://doi.org/10.1016/j.compag.2009.05.003, 2009.
Mulder, V. L., de Bruin, S., Schaepman, M. E., and Mayr, T. R.: The use of remote sensing in soil and terrain mapping – A review, 162, 1–19, https://doi.org/10.1016/j.geoderma.2010.12.018, 2011.
Nawaz F., M., Bourrié, G., and Trolard, F.: Soil compaction impact and modelling. A review, Agron. Sustain. Dev., 33, 291–309, https://doi.org/10.1007/s13593-011-0071-8, 2012.
Pentoś, K., Pieczarka, K., and Serwata, K.: The Relationship between Soil Electrical Parameters and Compaction of Sandy Clay Loam Soil, Agriculture, 11, 114, https://doi.org/10.3390/agriculture11020114, 2021.
Piccoli, I., Seehusen, T., Bussell, J., Vizitu, O., Calciu, I., Berti, A., Börjesson, G., Kirchmann, H., Kätterer, T., Sartori, F., Stoate, C., Crotty, F., Panagea, I. S., Alaoui, A., and Bolinder, M. A.: Opportunities for Mitigating Soil Compaction in Europe–Case Studies from the SoilCare Project Using Soil-Improving Cropping Systems, Land, 11, 223, https://doi.org/10.3390/land11020223, 2022.
Raper, R. L.: Agricultural traffic impacts on soil, J. Terramechanics, 42, 259–280, https://doi.org/10.1016/J.JTERRA.2004.10.010, 2005.
Reintam, E., TrüKmann, K., Kuht, J., Nugis, E., Edesi, L., Astover, A., Noormets, M., Kauer, K., Krebstein, K., and Rannik, K.: Soil compaction effects on soil bulk density and penetration resistance and growth of spring barley (Hordeum vulgare L.), Acta Agr. Scand. B.-S. P., 59, 265–272, https://doi.org/10.1080/09064710802030070, 2009.
Ren, L., D'Hose, T., Borra-Serrano, I., Lootens, P., Hanssens, D., De Smedt, P., Cornelis, W. M., and Ruysschaert, G.: Detecting spatial variability of soil compaction using soil apparent electrical conductivity and maize traits, Soil Use Manag., 38, 1749–1760, https://doi.org/10.1111/SUM.12812, 2022.
Romero-Ruiz, A., Linde, N., Keller, T., and Or, D.: A Review of Geophysical Methods for Soil Structure Characterization, Rev. Geophys., 56, 672–697, https://doi.org/10.1029/2018RG000611, 2018.
Romero-Ruiz, A., Linde, N., Baron, L., Breitenstein, D., Keller, T., and Or, D.: Lasting Effects of Soil Compaction on Soil Water Regime Confirmed by Geoelectrical Monitoring, Water Resour. Res., 58, e2021WR030696, https://doi.org/10.1029/2021WR030696, 2022.
Samouëlian, A., Cousin, I., Tabbagh, A., Bruand, A., and Richard, G.: Electrical resistivity survey in soil science: A review, Soil Till. Res., 83, 173–193, https://doi.org/10.1016/j.still.2004.10.004, 2005.
Schjønning, P., Lamandé, M., and Thorsøe, M. H.: Soil compaction – drivers, pressures, state, impacts and responses, DCA Rep., ISBN 978-87-93787-51-3, 2019.
Seladji, S., Cosenza, P., Tabbagh, A., Ranger, J., and Richard, G.: The effect of compaction on soil electrical resistivity: A laboratory investigation, Eur. J. Soil Sci., 61, 1043–1055, https://doi.org/10.1111/j.1365-2389.2010.01309.x, 2010.
Serrano, J., Shahidian, S., da Silva, J. M., Paixão, L., Moral, F., Carmona-Cabezas, R., Garcia, S., Palha, J., and Noéme, J.: Mapping management zones based on soil apparent electrical conductivity and remote sensing for implementation of variable rate irrigation–case study of corn under a center pivot, Water, 12, 1–17, https://doi.org/10.3390/w12123427, 2020.
Varmuza, K. and Filzmoser, P.: Introduction to Multivariate Statistical Analysis in Chemometrics, CRC Press, 46 pp., https://doi.org/10.1201/9781420059496, 2016.
Vereecken, H., Binley, A., Cassiani, G., Revil, A., and Titov, K.: Applied Hydrogeophysics, in: Applied Hydrogeophysics, Springer Netherlands, 1–8, https://doi.org/10.1007/978-1-4020-4912-5_1, 2007.
Von Hebel, C., Rudolph, S., Mester, A., Huisman, J. A., Kumbhar, P., Vereecken, H., and Van Der Kruk, J.: Three-dimensional imaging of subsurface structural patterns using quantitative large-scale multiconfiguration electromagnetic induction data, Water Resour. Res., 50, 2732–2748, https://doi.org/10.1002/2013WR014864, 2014.
Von Hebel, C., Van Der Kruk, J., Huisman, J. A., Mester, A., Altdorff, D., Endres, A. L., Zimmermann, E., Garré, S., and Vereecken, H.: Calibration, conversion, and quantitative multi-layer inversion of multi-coil rigid-boom electromagnetic induction data, Sensors (Switzerland), 19, 4753, https://doi.org/10.3390/s19214753, 2019.
von Hebel, C., Reynaert, S., Pauly, K., Janssens, P., Piccard, I., Vanderborght, J., van der Kruk, J., Vereecken, H., and Garré S.: Toward high-resolution agronomic soil information and management zones delineated by ground-based electromagnetic induction and aerial drone data, Vadose Zone J., 20, 1539–1663, https://doi.org/10.1002/vzj2.20099, 2021.
Virtanen, P., Gommers, R., Oliphant, T. E., Haberland, M., Reddy, T., Cournapeau, D., Burovski, E., Peterson, P., Weckesser, W., Bright, J., van der Walt, S. J., Brett, M., Wilson, J., Millman, K. J., Mayorov, N., Nelson, A. R. J., Jones, E., Kern, R., Larson, E., Carey, C. J., Polat, İ., Feng, Y., Moore, E. W., VanderPlas, J., Laxalde, D., Perktold, J., Cimrman, R., Henriksen, I., Quintero, E. A., Harris, C. R., Archibald, A. M., Ribeiro, A. H., Pedregosa, F., van Mulbregt, P., Vijaykumar, A., Bardelli, A. Pietro, Rothberg, A., Hilboll, A., Kloeckner, A., Scopatz, A., Lee, A., Rokem, A., Woods, C. N., Fulton, C., Masson, C., Häggström, C., Fitzgerald, C., Nicholson, D. A., Hagen, D. R., Pasechnik, D. V., Olivetti, E., Martin, E., Wieser, E., Silva, F., Lenders, F., Wilhelm, F., Young, G., Price, G. A., Ingold, G. L., Allen, G. E., Lee, G. R., Audren, H., Probst, I., Dietrich, J. P., Silterra, J., Webber, J. T., Slavič, J., Nothman, J., Buchner, J., Kulick, J., Schönberger, J. L., de Miranda Cardoso, J. V., Reimer, J., Harrington, J., Rodríguez, J. L. C., Nunez-Iglesias, J., Kuczynski, J., Tritz, K., Thoma, M., Newville, M., Kümmerer, M., Bolingbroke, M., Tartre, M., Pak, M., Smith, N. J., Nowaczyk, N., Shebanov, N., Pavlyk, O., Brodtkorb, P. A., Lee, P., McGibbon, R. T., Feldbauer, R., Lewis, S., Tygier, S., Sievert, S., Vigna, S., Peterson, S., More, S., Pudlik, T., et al.: SciPy 1.0: fundamental algorithms for scientific computing in Python, Nat. Methods, 17, 261–272, https://doi.org/10.1038/s41592-019-0686-2, 2020.
Viscarra Rossel, R. A., Adamchuk, V. I., Sudduth, K. A., McKenzie, N. J., and Lobsey, C.: Proximal Soil Sensing: An Effective Approach for Soil Measurements in Space and Time, in: Advances in Agronomy, vol. 113, Academic Press, 243–291, https://doi.org/10.1016/B978-0-12-386473-4.00005-1, 2011.
Wait, J.: Geo-electromagnetism, New York, https://doi.org/10.1016/B978-0-12-730880-7.X5001-7, 1982.