the Creative Commons Attribution 4.0 License.
the Creative Commons Attribution 4.0 License.
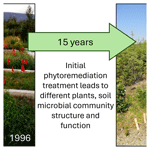
Long-term legacy of phytoremediation on plant succession and soil microbial communities in petroleum-contaminated sub-Arctic soils
Mary-Cathrine Leewis
Christopher Kasanke
Ondrej Uhlik
Mary Beth Leigh
Phytoremediation can be a cost-effective method of restoring contaminated soils using plants and associated microorganisms. Most studies follow the impacts of phytoremediation solely across the treatment period and have not explored long-term ecological effects. In 1995, a phytoremediation study was initiated near Fairbanks, Alaska, to determine how the introduction of annual grasses and/or fertilizer would influence degradation of petroleum hydrocarbons (PHCs). After 1 year, grass and/or fertilizer-treated soils showed greater decreases in PHC concentrations compared to untreated plots. The site was then left for 15 years with no active site management. In 2011, we re-examined the site to explore the legacy of phytoremediation on contaminant disappearance, as well as on plant and soil microbial ecology. We found that the recruited vegetation and the current bulk soil microbial community structure and functioning were all heavily influenced by initial phytoremediation treatment. The number of diesel-degrading microorganisms (DDMs) was positively correlated with the percentage cover of vegetation at the site, which was influenced by initial treatment. Even 15 years later, the initial use of fertilizer had significant effects on microbial biomass, community structure, and activity. We conclude that phytoremediation treatment has long-term, legacy effects on the plant community, which, in turn, impact microbial community structure and functioning. It is therefore important to consider phytoremediation strategies that not only influence site remediation rates in the short-term but also prime the site for the restoration of vegetation over the long-term.
- Article
(3761 KB) - Full-text XML
-
Supplement
(703 KB) - BibTeX
- EndNote
© His Majesty the King in right of Canada, as represented by the Minister of Agriculture and Agri-Food Canada, 2024.
There is a worldwide legacy of contamination from operational and abandoned mining and industrial sites, waste disposal sites, abandoned and active military installations, and other anthropogenic activities (Stow et al., 2005; Pier et al., 2002). In particular, petroleum hydrocarbons (PHCs) are widespread in the environment as a consequence of their broad use in society, posing serious human health hazards. In the state of Alaska, there are nearly 2000 documented sites with contaminated soil, many of which contain petroleum (Alaska Department of Environmental Conservation Spill Prevention and Response, 2024). The harsh climate and remote location of many communities at high latitudes pose unique challenges for contaminant remediation. Lack of road access can make traditional physical remediation methods difficult, cost-prohibitive, or even impossible (Kuiper et al., 2004). Bioremediation offers a potentially affordable and feasible solution for contaminant clean-up in remote communities which are especially dependent on petroleum products for heat and transportation. Soil amendments, such as fertilizer or plants, can be used to stimulate the degradation of contaminants by indigenous soil microorganisms and are often more cost effective in remote areas (Wenzel, 2009; Van Aken et al., 2010; Leewis et al., 2016b). The biodegradation of organic pollutants in plant-associated soils is hypothesized to be driven by the release of secondary plant metabolites (SPMEs) (Leewis et al., 2016a; Musilova et al., 2016). This is because soil bacterial communities that are plant-associated are able to use or transform SPMEs, such as phenols, through diverse groups of broadly specific enzymes such as aromatic ring-hydroxylating dioxygenases (Zubrova et al., 2021). These enzymes have also been implicated in the transformation and degradation of organic contaminants, such as PHCs, which are structurally similar to SPMEs (Leigh et al., 2002; Singer et al., 2003; Musilova et al., 2016; Zubrova et al., 2021).
The microbial and vegetative processes associated with biologically based remediation strategies, i.e., phytoremediation and rhizoremediation, have been a focus of study for decades (Fletcher and Hegde, 1995; Siciliano and Germida, 1998; Palmroth et al., 2002; Gerhardt et al., 2009; Macek et al., 2009; Glick, 2010). In many cases, remediation treatment results in decreases in soil contaminant concentrations and changes in the soil microbial community (Liu et al., 2015; Yergeau et al., 2015; Bell et al., 2013; Palmroth et al., 2005; Leewis et al., 2016a; Lopez-Echartea et al., 2020). Many of these studies, however, focus on the short-term effects of treatment on microbial and vegetative communities and contaminant transformation. Monitoring the long-term effects of contamination and subsequent treatment on soils beyond the first few years of active site management or experimentation is important in assessing the long-term impacts on ecological processes such as plant succession and microbial community functioning (Susarla et al., 2002; Mukherjee et al., 2014). Few studies have investigated the long-term effects of remediation treatments on PHC-contaminated soils; most focused on the effects of land-farming on contaminant concentrations (Loehr and Webster, 1996; Mills et al., 2006) without determining vegetation recruitment or the effects of treatment on microbial communities. Other studies have explored the long-term effects of treatment and soil chemistry on microbial communities (Sutton et al., 2013; Liang et al., 2016) but without determining the effects of treatment on vegetation recruitment. While these studies provide insight into the disappearance and biodegradation of legacy PHC contamination, even these comprehensive investigations stop short of fully characterizing the in situ degradation and plant-associated changes in microbial communities, which would be necessary for an integrative understanding of the effects of different remediation strategies on site ecology. More long-term studies of ecosystem recovery are essential to achieve an integrated understanding of how plant communities, remediation treatment, contaminant concentration, and microbial populations interact as sites change over time.
In a series of studies, we have sought to further understand the long-term (over-15-year-long) relationships between original phytoremediation treatment, colonizing vegetation, soil microbial communities, and the degradation of petroleum contaminants at an experimental site in Interior Alaska (Leewis et al., 2013; Reynolds et al., 1997a; Reynolds and Koenen, 1997). The original phytoremediation experiment by Reynolds et al. (1997a) was designed to investigate the effects of combinations of planting and fertilizer treatments on the remediation of soils contaminated with either crude oil or diesel fuel (Reynolds et al., 1997a; Reynolds and Koenen, 1997; Reynolds et al., 1999). In Leewis et al. (2013), we described changes in the soil chemistry, plant community composition, total petroleum hydrocarbon (TPH) concentrations, and bacterial community as determined by a molecular-fingerprinting technique (i.e., terminal restriction fragment length polymorphism – T-RFLP) after 15 years without active site management. Papik et al. (2023) further explore the legacy of original treatment on the bacterial rhizosphere and endosphere communities (Papik et al., 2023). In the current study, we build upon this previous work and aim to (i) evaluate the interactive effects of petroleum contamination and phytoremediation on site recovery over time and (ii) identify the factors that drive vegetation community structure, soil microbial community structure and functioning, and TPH loss. Here, we report additional analyses of the same soil samples from Leewis et al. (2013) to further characterize the microbial community using (A) culture-based techniques to determine the ability of the soil community to use TPH as a carbon source (MPNs), (B) phospholipid fatty acids (PLFAs) to fingerprint the entire soil community (including bacteria, fungi, and protozoa), and (C) 16S rRNA gene sequencing to determine the identity of bulk soil bacterial community members. We additionally incorporated the newly analyzed plant percent cover data and previously reported soil chemistry, petroleum hydrocarbon, and plant community count data into statistical analyses of the microbial community.
2.1 Legacy field study background and site description
In 1995, a phytoremediation field study was initiated by the U.S. Army Corps of Engineers Cold Regions Research and Engineering Laboratory (ACE CRREL) located in Fairbanks, Alaska (Reynolds et al., 1997b, 1999; Reynolds and Koenen, 1997). The study sought to compare the effects of different phytoremediation treatments on two soil and contamination types: (A) crude-oil-contaminated soils collected from a gravel pad or (B) diesel-contaminated soils collected during the removal of an underground storage tank. The experiment was initiated at the ACE CRREL Farmers Loop Facility, where soils were placed in piles in adjacent lined areas. Each of the separate soil piles was then divided into individual plots (Fig. 1) which represented different phytoremediation strategies. The original phytoremediation treatment plots were as follows: two plots not planted or fertilized (c1 and c2), one plot spread with fertilizer (f), one plot planted with annual ryegrass (Lolium multiflorum; p1), one plot planted with a mixture of annual ryegrass and Arctared fescue (Festuca rubra; 1 : 1 mixture, p2), one plot fertilized and planted with annual ryegrass (p1f), and one plot fertilized and planted with both ryegrass and fescue (p2f; Fig. 1c). Fertilizer was applied to soil surfaces by hand at approximately 620 g m−2 of N, P, and K using a commercially available fertilizer (granular 20-20-10). Seeds were spread by hand at approximately 10.8 g m−2.
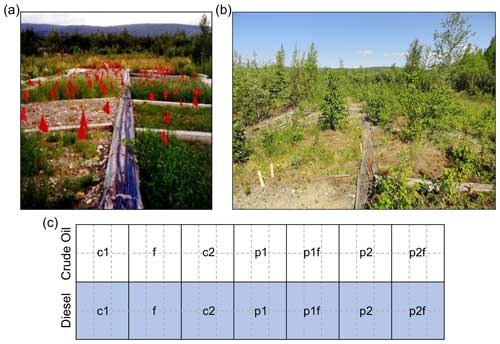
Figure 1Overview of site. Photos of study site in (a) 1996 (photo credit: C. Michael Reynolds) and (b) 2011 showing plant colonization of the different soil types and phytoremediation treatment plots. (c) Overview of the original phytoremediation treatment plots (outlined in thick black lines). Half of the plots are soils from a gravel pad originally contaminated with crude oil (white background), and half are soils from near a leaking diesel fuel storage tank that has been contaminated (grey background). The original treatments applied in 1995 were as follows: no treatment (c1, c2), planted with annual ryegrass (p1), a mix of annual ryegrass and Arctared fescue (p2), treated with fertilizer (f), and/or no added nutrients (no “f” indicated). For this follow-up study, original plots were each subdivided into six subsections (outlined in dashed grey lines) to allow for pseudo-replication.
2.2 Sample collection and vegetation characterization
In June 2011, we initiated a follow-up study of the initial phytoremediation experiment to determine the continuing influence of original treatment on current TPH concentrations, microbial community presence and abundance, and plant community composition. Due to the lack of replication in the original study design, we divided each of the original treatment plots into six 1×1.5 m2 subsections (Fig. 1c). From each subsection, we collected soil samples into both sterile plastic zip-top bags for microbial analyses and a glass jar with Teflon-lined lids for TPH analysis. Samples were sieved through a sterile 2.5 mm sieve and stored at (A) −80 °C for molecular and TPH analysis or (B) 4 °C for culture-based microbial assays (i.e., most-probable-number analyses – MPN) which took place within 1 week of collection. Due to the number of subsamples and to reduce the overall costs of analyses, we conducted analyses on the following number of subsections per plot: (A) culturable diesel-degrading microorganisms (DDM) – six subsections, (B) PLFAs – four subsections, and (C) bacterial community structure and TPH – three subsections. Reported values are averages with standard deviations.
At the time of soil sampling, we also photographed each individual subsection to estimate the percentage cover of vegetation. Vegetation coverage was classified into four types: grasses (including live and dead), forbs (herbaceous flowering plants; for example, fireweed, dandelions, vetch, Oxytropus), trees, and bare ground (including crusts and moss cover). Each photo was overlaid with a 5×5 grid, and if an individual block within the grid was more than 50 % covered by a vegetation type then that block was counted as positive for that vegetation type (Vanha-Majamaa et al., 2000; Chen et al., 2010).
2.3 Phospholipid fatty acid (PLFA) assay
PLFA extractions and analyses were performed following the methods of Wu et al. (2009) with minor modifications as follows. Each soil sample (ca. 4.8 g) was freeze-dried, and the weight after freeze-drying was recorded for data analysis. All drying steps were performed with N2 at a temperature below 40 °C. Phospholipids were extracted using 8 mL methanol (HPLC grade, Fisher Scientific, Pittsburgh, PA). Fatty acid methyl esters were extracted using hexane (3×1 mL) and then were dried under N2 (HLPC-grade hexane, EM, Germany). Prior to GC analysis, 80 µL of the internal standard, methyl nonadecanoate (0.737 nmol µL−1; Sigma-Aldrich, Switzerland), was added, and the samples were dried completely. The samples were then dissolved in 80 µL hexane and analyzed on an Agilent 6890 N Gas Chromatograph, using an Agilent 19091B-102 (25.0 m × 200 µm × 0.33 µm) capillary column and MIDI peak identification software using an internal standard (version, 6.1; MIDI Inc., Newark, DE). PLFA biomarkers and biomass were assigned to microbial groupings, as outlined in Table S1 in the Supplement (Song et al., 2008; Kaiser et al., 2010; Ngosong et al., 2012; Stella et al., 2015; Oburger et al., 2016; Polivkova et al., 2018).
2.4 Enumeration of diesel-degrading microorganisms
The abundance of culturable aerobic DDMs was determined by a 96 well plate most-probable-number (MPN) method adapted from Haines et al. (1996), following the modifications detailed in Leewis et al. (2016a). Soils were stored at 4 °C and processed within a week of collection. Briefly, microorganisms were extracted from triplicate soil samples in a solution of 1 % sodium pyrophosphate (Fisher Scientific, USA) with 3 g of sterile glass beads by shaking horizontally for 1 h, followed by 30 min of settling. This suspension was used to create a 10-fold serial dilution in a Bushnell Haas medium for each sample in triplicate, along with positive and negative controls. After inoculation, filter-sterilized diesel fuel oil no. 2 was added to each test well as the sole carbon source. Plates were then incubated in the dark at room temperature for 14 d, after which we added a sterile indicator dye (3 g L−1 p-iodonitrotetrazolium; MP Biomedicals, USA) to each well to indicate the presence of active respiring microorganisms. After 48 h, wells were scored according to the presence of a red or pink colour, and MPN values were calculated using previously published MPN tables (Man, 1983).
2.5 Amplicon preparation and sequencing
Soil DNA was extracted from a 0.5 g soil subsample using the FastDNA SPIN kit for soil (MP Biomedicals, Ohio, USA) following the manufacturer's instructions. DNA was eluted into 50 µL of water and stored at −20 °C until analysis. DNA concentrations were evaluated by measuring absorbance at 260 and 280 nm using a NanoDrop ND-1000 spectrophotometer (Thermo Fisher Scientific, USA). Regions V4–V5 of the 16S rRNA gene were amplified using primers 563-577F, 5′-AYTGGGYDTAAAGNG-3′, and 926-909R, 5′-CCGTCAATTCMTTTRAGT-3′ (Uhlik et al., 2012; Cole et al., 2009). Each of the primers was synthesized with sequencing adaptors (454 Sequencing Application Brief No. 001-2009, Roche), and the forward primer was also modified with different barcodes (454 Sequencing Technical Bulletin No. 005-2009, Roche) to allow for multiple samples to be pooled for sequencing. The PCR mixture (final volume: 25 µL) contained 1 µL of each primer (10 µM), 0.5 µL dNTP mix (10 mM), 2.5 µL FastStart 10× Buffer no. 2, 0.25 µL FastStart HiFi Polymerase (5 U µL−1), and 18.75 µL molecular-biology-grade water (Roche). The following thermal cycling scheme was used: initial denaturation at 95 °C for 3 min and 30 cycles of denaturation at 95 °C for 30 s, annealing for 1 min at 55 °C, and extension at 72 °C for 1.5 min, followed by a final extension period at 72 °C for 10 min. Each PCR product was obtained in three parallel reactions; the resulting preparations were mixed, purified using the PureLink PCR Purification Kit (Invitrogen, USA), and pooled for downstream sequencing. Roche 454 GS FLX Titanium sequencing (454 Life Sciences, USA) was performed on pooled reactions at the Utah State University Center for Integrated Biosystems, Logan, Utah, USA. It should be noted that, although older technologies, such as 454-pyrosequencing, are known to suffer from drawbacks such as limited read depth and higher error rates in repeated complex sequences (e.g., homopolymer regions), they can still provide a comparable and comprehensive overview of environmental communities and biodegradation processes as assessed by other sequencing technologies, including Illumia MiSeq (Lauber et al., 2009; Bell et al., 2011; Luo et al., 2012; Goordial et al., 2016; Akaçin et al., 2022).
2.6 Sequence analyses
Raw pyrosequencing data (*.sff files) were processed using the mothur software package version 1.45.3 and following the standardized operating procedure (Schloss et al., 2011), accessed July 2021, with minor modifications as described previously (Uhlik et al., 2012). Sequences with > 3 % sequence similarity were grouped into the same operational taxonomic units (OTUs). These were then classified by mothur-implemented RDP (Ribosomal Database Project) reference files (trainset18_062020; accessed July 2021) (Wang et al., 2007; Cole et al., 2014), and sequences associated with mitochondria, chloroplasts, archaea, eukaryote, and unknown kingdoms were removed. Data were then rarefied to the minimum library size of 3537 sequences. Pyrosequencing reads were deposited in NCBI Short Read Archive under bioproject no. PRJNA950456. All the coding for bioinformatics analyses is available at https://github.com/mcleewis/FarmersLoop (last access: 4 May 2024; https://doi.org/10.5281/zenodo.13288502, Leewis, 2024).
2.7 Statistical analyses
All statistical analyses were performed in R version 3.6.1 (R Core Team, 2019). To determine the difference in the means of variables, we used a Kruskal–Wallace (KW) rank sum test with the original treatment as the factors. We then used a pairwise Wilcox test with no assumption of equal variance and corrected for multiple comparisons using a Benjamini–Hochberg (BH) correction to further test differences between individual original treatments. Kendall's tau rank correlation tests (τ) were used to examine relationships between independent variables.
Alpha and beta diversity metrics were calculated using the phyloseq package (McMurdie and Holmes, 2013) on rarefied bacterial sequence data and were tested using the vegan package (Oksanen et al., 2017). The influence of treatment and soil properties on bacterial communities was visualized using non-metric multidimensional scaling (NMDS; metaMDS()
) with vector fitting of associated soil and plant data (envfit()
) and was tested using a permutational multivariate analysis of variance (PERMANOVA; adonis()
). For multivariate analyses, PFLA data were not aggregated as in Table S1 but instead were treated as individual biomarkers. Strongly autocorrelated soil chemical data were removed from the vector fitting prior to analysis (cor()
, Pearson's r>0.70), and P values were corrected using the p.adjust
function (method
= “BH”
). The relationship between microbial community structure and measured soil properties was assessed using a Mantel test (mantel()
). A multi-response permutation procedure (MRPP; mrpp()
) was used to test the influence of the original treatment or soil type on microbial community structure. Multivariate testing of the microbial community was conducted using the Bray–Curtis dissimilarity measure (distance
= “bray”
), and significance was quantified using permutation tests (permutations
= 999
). Major OTUs representing more than 2 % of the total communities were depicted in a heatmap using the heatmap2
function. Differential sequence analysis was conducted using the DESeq2 (Love et al., 2014) R package, with P values adjusted for multiple comparisons with the Benjamini–Hochberg (BH) correction. All the coding for statistical analyses is available at https://github.com/mcleewis/FarmersLoop (last access: 4 May 2024; https://doi.org/10.5281/zenodo.13288502, Leewis, 2024).
3.1 Prior findings on TPH, soil properties, and plant communities
The results detailing soil chemistry and TPH concentrations are previously described in detail in Leewis et al. (2013); we briefly summarize those findings here (Tables S2, S3). The initial TPH concentration measured in crude-oil-contaminated soils was 6070 ppm, and it was 8350 ppm in diesel-contaminated soils (Reynolds et al., 1997a, b). In 1996, after almost 1 year of treatment, TPH concentrations in crude-oil-contaminated soils were 27 %–47 % lower than the initially measured concentrations. In diesel-contaminated soils, soil TPH concentrations were 65 %–87 % lower than the initial measurements (Table S2).
After 238 d of treatment, TPH concentrations in soils treated with plants or fertilizer (p1, f, p2) and soils without any amendment (c1, c2) decreased relative to the initial (day 0) TPH measurements (Table S2). Soils treated with both plants and fertilizer (p1f, p2f) had significantly lower TPH concentrations than the untreated soils (c1, c2) after 238 d of treatment (Reynolds et al., 1997a; Reynolds and Koenen, 1997; Reynolds et al., 1999). After completion of the initial phytoremediation project in 1996, the site was not actively managed for approximately 15 years, with no nutrient or re-seeding amendments being made. As previously reported, we found that increased TPH disappearance was associated with increased numbers of native trees and shrubs, such as willow (Salix spp.), birch (Betula neoalaskana), white spruce (Simarouba glauca), and balsam poplar (Populus balsamifera) regardless of the original phytoremediation treatment (Leewis et al., 2013).
3.2 TPH and soil chemistry
In 2011, soil TPH concentrations were 80 %–95 % lower than the concentrations measured in 1996 (Table S2). Concentrations of the remaining TPH were higher in crude-oil-contaminated soils (ca. 631–760 ppm) compared to in diesel-contaminated soils (ca. 319–430 ppm). Differences in lingering TPH concentrations may be associated with the original soil contaminant as crude oil contains more recalcitrant compounds than refined diesel fuels, or they may be associated with the soil quality itself as the gravelly crude-oil-contaminated soils were more nutrient-poor compared to diesel-contaminated soils (Table S3). However, after 15 years of treatment, no one treatment resulted in a significantly greater reduction in TPH concentrations (P>0.05; Table S2).
Unfortunately, the initial 1995–1996 investigation did not include measurements of soil chemistry or texture; those data are only available for soils collected in 2011 (Table S3). Overall, diesel-contaminated soils were significantly higher in total carbon, NO3–N, and K, with finer soil texture than crude-oil-contaminated soils (KW P<0.01). Soils that had been originally fertilized remained significantly higher in total P and K compared to unfertilized soils (KW P<0.001), regardless of contaminant type.
3.3 Long-term responses of plant community to phytoremediation treatment
After 15 years with no active site management, the plant community within each treatment plot changed significantly from the first establishment of the site in 1995, and none of the originally planted grasses were present at the time of sample collection (Leewis et al., 2013). Plant communities differed according to soil type (MRPP A = 0.175, P=0.001): in the finer-textured diesel-contaminated soils, there were more – and larger – woody plants (e.g., Salix spp., Populus spp., Betula neoalaskana, and Picea glauca) present when compared to the crude-oil-contaminated soils (Table S4, Fig. S1 in the Supplement). The crude oil plots, with soils that originated from a gravel pad, were more heavily colonized by non-native plants from the family Fabaceae, such as Taraxacum officinale (dandelion), Vicia cracca (common vetch), and Trifolium hybridum (clover). Native plants including Ledum decumbens (Labrador Tea) and Epilobium angustifolium (fireweed), along with willow (Salix spp.), spruce (Picea spp.), and poplar seedlings (Populus spp.), were present throughout the site, regardless of soil type (i.e., crude-oil-contaminated gravelly soils or diesel-contaminated fine-textured soils). Further exploration found that original phytoremediation treatment explained 63 % of the variation in the current plant community structure (PERMANOVA CO: P=0.001, R2=0.630, F=3.978; DE: P=0.001, R2=0.635, F=4.077). In both soil types, plots originally fertilized had greater coverage with grasses and less bare ground than other plots; on average, 53 % and 38 % of the area was covered by grasses in crude oil and diesel plots, respectively (Figs. 2, S1). Crude-oil-contaminated soils had more bare ground than diesel-contaminated soils (39 % and 13 %, respectively). In crude-oil-contaminated soils, originally untreated plots had an average of 44 % bare ground, and those initially planted had 79 % bare ground. Plots not originally fertilized had more coverage of trees compared to other plots, with, respectively, on average, 16 % and 66 % tree coverage in crude-oil-contaminated and diesel-contaminated plots not originally fertilized (Figs. 2, S2).
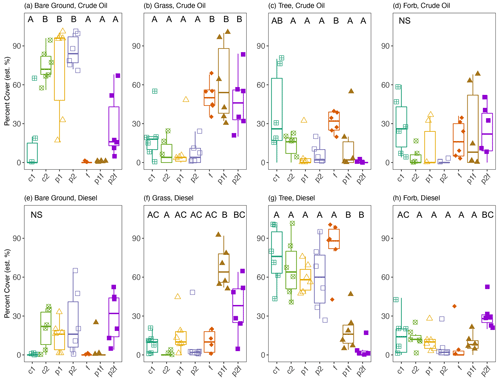
Figure 2Estimated percentage cover of vegetation groups in either crude oil (a–d) or diesel (e–h) plots. Measurements were based on visual estimates in each of the six sub-plots. The values shown are means with 95 % confidence intervals (N=6); note that the y axes have different scales. Treatments indicated are as follows: no treatment (c1, c2), planted with annual ryegrass (p1), a mix of annual ryegrass and Arctared fescue (p2), treated with fertilizer (f), and/or no added nutrients (no “f” indicated). Significant differences in percentage cover are indicated by different letters, where “NS” indicates that no significant differences were found.
3.4 Quantity of diesel-degrading microorganisms is associated with TPH and vegetation
To assess the ability of the soil community to use petroleum hydrocarbons (PHCs) as a carbon source, we measured the MPN of culturable DDMs across all the treatment plots (Fig. 3a). Overall, there were significantly more DDMs in the crude-oil-contaminated, gravely soil (average 5.57±0.71 log MPN g soil−1) than in diesel-contaminated soils (4.31±0.77 log MPN g soil−1; P<0.05 CO vs. DE; Fig. 3a). We also found differences in the amount of DDM associated with the original phytoremediation treatment: in the crude oil soils, an initially untreated plot (6.34±0.24 log MPN g soil−1) and the fertilizer-only plot (6.43±0.61 log MPN g soil−1) had the highest numbers of DDM (P<0.04, Kruskal–Wallis). In diesel soils, one initially untreated plot had significantly higher numbers of DDM (5.35±0.81 log MPN g soil−1) than the other treated plots (P<0.04, Kruskal–Wallis).
We next examined the relationship between DDM and other factors at the site at the time of collection, including TPH concentrations and current vegetation. There was a weak trend between counts of DDM and TPH concentrations in both soil types, in which increased counts were associated with decreased TPH concentrations, although this relationship was not significant in either crude-oil- or diesel-contaminated soils (Fig. S2a, b). In both crude oil and diesel soils, there was a positive relationship between DDM and the percent of vegetation coverage in all plots (i.e., no bare ground), although this relationship was only significant in crude-oil-contaminated soils (CO: Kendall's τ=0.3512, P=0.0025; DE: Kendall's , P=0.7623; Fig. S3a, b). We further explored this plant-contaminant relationship and found a significant positive relationship between DDM and the percentage cover of trees in the crude oil plots (Kendall's τ=0.3550, P=0.0026; Fig. S2c) but not in the diesel plots (Fig. S2d). In crude oil plots, there was also significant relationship between the amount of bare ground and a lower DDM (Kendall's , P=0.0025), but no similar relationship was found in the diesel-contaminated plots (Kendall's τ=0.0367, P=0.7623). There was no statistically significant relationship found between the percentage coverage of grasses or forbs and DDM in either contamination type (P>0.06).
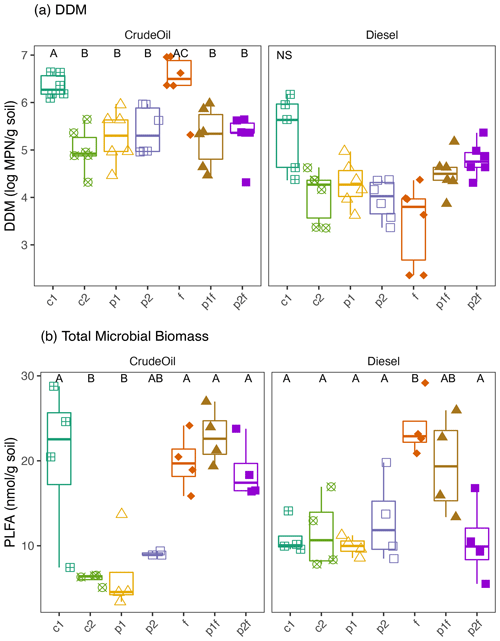
Figure 3Measures of (a) most probable number (MPN) of diesel-degrading microorganisms or (b) total microbial biomass as measured by PLFA biomarkers in either crude oil (dark grey) or diesel (light grey) soils. Measurements were based on 10 triplicate 1 g soil samples. The values shown are means with 95 % confidence intervals (N=6). Treatments indicated are as follows: no treatment (c1, c2), planted with annual ryegrass (p), a mix of annual ryegrass and Arctared fescue (p2), treated with fertilizer (f), and/or no added nutrients (no “f” indicated). Significant differences in percentage cover are indicated by different letters, where “NS” indicates that no significant differences were found.
3.5 Soil microbial communities are influenced by treatment and vegetation
Microbial biomass was significantly influenced by both the original phytoremediation treatment and soil type (i.e., crude-oil-contaminated soils or diesel-contaminated soils), as indicated by analysis of PLFAs. Total microbial biomass was generally higher in plots that had been fertilized compared to plots that had not received original fertilization (Fig. 3, P<0.05, Kruskal–Wallis), but there was no clear trend between grouped PLFA biomarkers and initial treatment (Fig. S4). However, we did find a significant association between total microbial biomass and the estimated total percent cover of vegetation in all plots (Fig. S3; CO: Kendall's τ=0.6101, P<0.0001; DE: Kendall's τ=0.3012, P=0.0374). We further explored the influence of original treatment and soil properties on microbial community structure as defined by PLFA biomarkers using PERMANOVA. Most of the variation in the composition of PFLA biomarkers was explained by original phytoremediation treatment (CO-contaminated soils: 82 %, DE-contaminated soils: 61 %) and by the presence of fertilizer in that treatment (CO-contaminated soils: 36 %, DE-contaminated soils: 24 %; Table 1). In diesel soils, the concentration of TPH and NO explained approximately 20 % variation in the composition of PLFA biomarkers (Table 1). In crude oil soils, other measured soil properties, such as percentage C, pH, NO, K, P, and CEC (cation exchange coefficient), also significantly explained the variation in the composition of PLFA biomarkers (Table 1). In crude-oil-contaminated soils, the coverage of grasses, the amount of bare ground, and (marginally) coverage of forbs were significantly associated with variation in the composition of PLFA biomarkers (Table 1).
Table 1Association of soil microbial community structure, as measured by PLFA biomarkers or 16S rRNA gene sequencing, with factors such as original treatment type, presence of fertilizer in original treatment, amount of vegetation present on the site at the time of sample collection, or soil nutrient and structure data. P values adjusted for multiple comparisons using BH. In the P (adj) column, bold values indicate P<0.05, and italicized values indicate P<0.1.
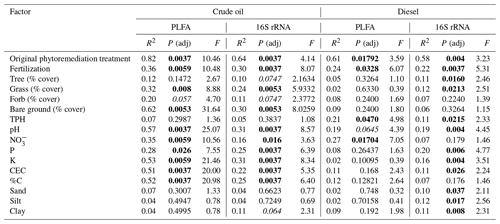
Ordination of individual PLFA biomarkers indicated that soil community structure was most strongly associated with soil type (Fig. S5a), and, when split by soil type, there was also an influence by the original phytoremediation treatment (Fig. 4a, b). We tested the strength of these associations and found that the relationship between original treatment and PLFA-derived biomarkers was stronger in the crude-oil-contaminated soils (MRPP A = 0.542, P=0.001) than in the diesel-contaminated soils (MRPP A = 0.268, P=0.005). A vector-fitting method used to interpret the ordinations using measured soil chemical properties indicated that, in the crude oil soils, several factors were significantly associated with the distribution of all PLFA biomarkers in ordination space, particularly in plots that were initially fertilized, which were associated with increased soil nutrients (e.g., P, K) and other metrics of soil health (e.g., near-neutral pH, CEC, and %C; Fig. 4a). In diesel-contaminated soils, however, only NO was associated with the distribution of PLFA biomarkers in ordination space (Fig. 4b).
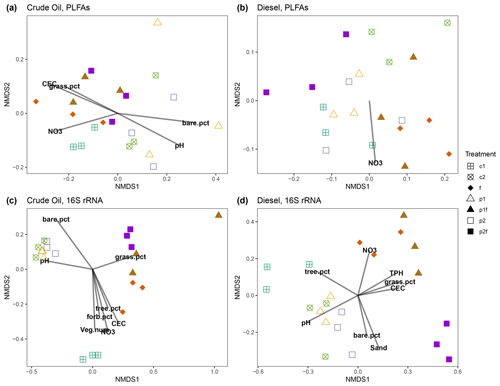
Figure 4Non-metric multidimensional scaling ordination analysis (NMDS) of soil PLFAs (a, b) or 16S rRNA genes (c, d) from crude-oil-contaminated (a, c) or diesel-contaminated (b, d) soils, with subsequent fitting of environmental vectors onto the ordination (P<0.05). The vectors are as follows: NO3 refers to nitrate, CEC refers to the cation exchange coefficient, veg.num refers to the total vegetation counts (excluding trees), tree.num refers to the total number of trees, TPH refers to the total petroleum hydrocarbons, and sand refers to the soil texture (i.e., % sand, % silt, % clay). Solid symbols indicate treatments originally fertilized. Treatments indicated are as follows: no treatment (c1, c2), planted with annual ryegrass (p1), a mix of annual ryegrass and Arctared fescue (p2), treated with fertilizer (f), and/or no added nutrients (no “f” indicated).
Analysis of the bacterial community structure using 16S rRNA gene sequencing revealed the strong influence of soil type (diesel-contaminated silty soil vs. crude-oil-contaminated gravelly soil) on the soil community (Fig. S5b). Alpha diversity of the bacterial community as measured by the Shannon index was higher in the crude oil soils when compared to the diesel-contaminated soils (Fig. S6; P=0.0005, Kruskal–Wallace). When separated by soil type, bacterial communities were significantly associated with original phytoremediation treatment; plots that were originally fertilized were grouped separately from other treatments (CO MRPP A = 0.214, P=0.013; DE MRPP A = 0.356, P=0.002; Fig. 4c, d). Vector-fitting analysis indicated that pH and soil chemical properties (e.g., NO, CEC, nutrients) heavily influenced the bacterial community structure in both soil types (Fig. 4). In both soil types, the number of trees present on each plot was associated with bacterial community structure in the originally unplanted or unfertilized plots.
We further examined the relationship between soil and phytoremediation treatment factors with bacterial community structure using a PERMANOVA test. In both soil types, the original treatment type explained more than half of the variation in the bacterial community structure (CO 64 %, DE 58 %), and the presence of fertilizer in the original treatment explained approximately one-quarter of the variation (CO 30 %, DE 22 %). Measures of soil health, such as near-neutral pH, nutrient concentrations (P, K), CEC, and soil texture, were also associated with the variation in bacterial community structure in both crude-oil- and diesel-contaminated soils (Table 1). The plant communities present also helped explain the variation in bacterial community structure. In diesel-contaminated soils, percent coverage of trees and grasses was associated with bacterial community structure (Table 1). In crude-oil-contaminated soils, the coverage of grasses, the amount of bare ground, and the (marginal) coverage of forbs were significantly associated with bacterial community structure (Table 1). We then used a Mantel test to further explore these relationships between the species composition of plant communities and the bacterial community structure. There was a strong relationship between the bacterial community and the vegetation present at the site in both crude-oil-dominated soils (Mantel r=0.2147, P=0.013) and diesel-contaminated soils (Mantel r=0.3568, P=0.002).
To determine differences among bacterial communities, we constructed a heatmap of families which were showed an abundance of at least 2 % in more than one sample (Fig. S7). The family Bradyrhizobiaceae dominated the bacterial community in diesel-contaminated soils (27.62 %) and was also abundant in the crude oil soils (8.89 %). The family Acidobacteria (subgroup Gp6) was also abundant across all the treatments and contaminant types, with a higher relative abundance in crude oil soils (11.60 %) than in diesel soils (8.36 %). Members of the families Gemmatimonadaceae (crude oil: 4.20 %, diesel: 7.63 %), Rhodospirillaceae (crude oil: 1.73 %, diesel: 2.66 %), Xanthomonadaceae (crude oil: 1.36 %, diesel: 1.08 %), and Caulobacteraceae (crude oil: 1.40 %, diesel: 1.69 %), among others, were present in all investigated soils (Fig. S7). At all sites, there was also a large percentage of bacteria unclassified at the family level (diesel, 9.81 %; crude oil, 13.29 %), suggesting that they may be novel sequences of uncharacterized bacteria (Fig. S7). To further explore the potential influence of original treatment on soil communities, we conducted differential sequence analysis (Fig. 5) to identify individual bacterial OTUs significantly associated with planting, fertilization, or a combination of planting and fertilization. Separately, for both crude-oil- and diesel-contaminated soils, we conducted three pairwise comparisons among the following treatments: (a) initially fertilized (f) versus planted (p1 and p2), (b) initially planted and fertilized (p1f and p2f) versus planted (p1 and p2), and initially fertilized (f) versus planted and fertilized (p1f and p2f). In general, similar taxa were identified in both the heatmap and differential abundance analysis, with many unclassified OTUs identified as being differentially abundant, along with members of the Bradyrhizobiaceae and Acidobacteria families.
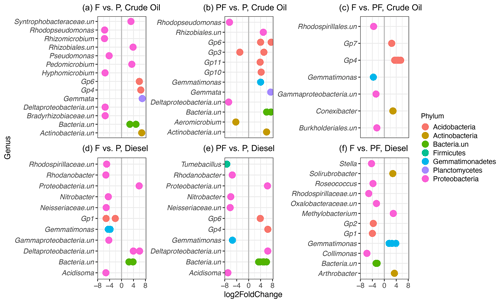
Figure 5Differential sequence analysis showing significantly enriched (Padj<0.001) bacterial OTUs from crude-oil-contaminated soils (a–c) and diesel-contaminated soils (d–f). Pairwise comparisons include initially fertilized (F) versus planted with one or two grasses (P), initially planted and fertilized (PF) versus planted only (P), and initially fertilized (F) versus planted and fertilized. Negative log2 fold change (left of vertical grey line) values represent OTUs significantly enriched in the treatment listed first, while positive log2 fold change (right of vertical grey line) represents OTUs significantly enriched in the second listed initial treatment. Only the top 15 differentially abundant OTUs with the highest log2 fold changes are represented. Multiple observations per row represent OTUs belonging to the same genus. Colours represent phyla, while genera ending with “.un” indicate unclassified taxa.
Here, we investigated the long-term influence of phytoremediation on recruited plant communities, contaminant disappearance, and soil microbial community structure and capacity for PHC degradation 15 years after phytoremediation treatment activities had stopped. We found that phytoremediation treatment had deterministic effects on the recruited vegetation (Figs. 2, S1) and associated bulk soil microbial communities (Table 1; Figs. 3, 4, S5). Together with the measurements of soil microbial community structure and PHC degradation capacity, these data provide a more holistic, long-term view of the impact of treatment on plants and soil microbial communities at this site.
Long after active phytoremediation ended, variation in plant communities across the site was most strongly shaped by the original treatment, although the study design did not allow us to fully determine the cause–effect nature of this relationship (Table 1; Figs. 2, S1). In particular, the initial use of fertilizer was strongly associated with more vegetation coverage, whereas plots without fertilizer application had more exposed bare ground. While the direct effects of fertilizer application may have been relatively transient, on the order of months, we did find that soil initially fertilized continued to have higher total P and K than other soils (Table S3). It is possible that the original fertilization acted to allow seeds to colonize and establish in treated plots, thereby priming plant succession. Many early successional plant species such as forbs, grasses, and deciduous trees produce litter that is of higher quality and that therefore decomposes more rapidly than secondary successional species such as coniferous trees (Allison and Treseder, 2011). The subsequent turnover of this nutrient-rich plant biomass may also have played a role in setting plant communities on different successional trajectories through longer-term enhancement of available nitrogen (Clark et al., 2007; Cleland and Harpole, 2010). Indeed, other studies in early successional boreal forests have shown that fertilization can shift the plant community toward species with higher-quality litter and increased litter decay rates compared to unfertilized plots (Ruess et al., 1996; Allison et al., 2010).
The continuing influence of the original phytoremediation treatment was also evident in the soil microbial community (Table 1; Figs. 3, 4, S5). In particular, the initial use of fertilizer had sustained effects on the bulk soil microbial community, accounting for differences in both total microbial biomass and bacterial community structure (Figs. 3, 5). The total microbial communities as measured by PLFAs and 16S rRNA amplicon sequencing were structured according to the original treatment and the use of fertilizer. This is in agreement with several long-term studies that have found that initial fertilization in unmanaged systems can lead to sustained differences in microbial communities (Talbot and Treseder, 2012; Geisseler and Scow, 2014; Lupwayi et al., 2021). These treatment-scale effects can extend beyond the bulk-soil community into the endosphere of the plants themselves. In a sister study, we also found that the choice of initial phytoremediation strategy drove the succession of endophytic bacteria associated with colonizing vegetation (Papik et al., 2023).
Soil physiochemical properties such as pH and texture are known to influence microbial communities (Sutton et al., 2013; Leewis et al., 2022). The two soils used in our study differed substantially, including different original contaminants, origins, textures, pH values, and nutrient statuses (Tables S2, S3). In our previous study of this site, Leewis et al. (2013), we reported that these dissimilarities led to distinctions in the plants recruited on the two soils, with more primary successional and invasive plants present in the coarse-textured crude-oil-contaminated soils. Here, we see how the soil physiochemical effects also influenced the microbial communities and their response to the initial treatments (Figs. 3, 4, S2, S3, S5). Total soil microbial communities and the bacterial community were all more strongly associated with initial phytoremediation treatment and fertilization in the crude-oil-contaminated soils than those in the diesel-contaminated soils (Table 1). Coarse-textured soils tend to have less water and nutrient retention, conditions that are less hospitable for plant and microbial growth. Treatment such as fertilization and planting can lead to major changes in soil physiochemical properties, such as increasing soil organic matter content and nutrient retention, which can, in turn, impact the soil community (Reeve et al., 2010; Blanchet et al., 2016). Therefore, the lingering effect of treatment would be more evident in soils that started out as being harsher for microbial and plant growth.
The original phytoremediation treatment strategy influenced the vegetation recruited, which, in turn, influenced the microbial community structure and microbial biomass. While it is well known that both plants and fertilizer can cause shifts in soil microbial community structure, here, it is almost impossible to tease apart their separate contributions in relation to the microbial community because the vegetation on each plot is driven by the initial phytoremediation treatment. Our results indicate that the percent coverage of vegetation rather than the individual plant species themselves was an important factor in driving soil community structure. Similarly Brown and Jumpponen (2014) found that the influence of vegetation composition on bacterial community structure was minor compared to the presence or absence of plants. We also found higher microbial biomass in plots with more vegetation cover, which may be a consequence of initial fertilization. In addition to shifting plant recruitment patterns, fertilization can increase plant biomass, which, in turn, can increase litter deposition and belowground organic carbon deposition (Geisseler and Scow, 2014), and these plant inputs to soil may have a particularly important influence in degraded soil (Knelman et al., 2012).
Microbial community functioning is also known to be influenced by the presence and diversity of plants (Leewis et al., 2016a; Musilova et al., 2016; Schmid et al., 2021). In our previous investigation, we found an inverse relationship between increasing counts of woody vegetation and decreasing TPH concentrations (Leewis et al., 2013). Here, we show increasing DDM with more tree coverage in crude-oil-contaminated soils and a trend towards increasing DDM associated with decreasing TPH concentrations (Fig. S2). Several of the enriched OTUs across all treatments belonged to the phyla Proteobacteria and Actinobacteria, members of which have been previously shown to degrade diesel in Arctic soils (Ferrera-Rodríguez et al., 2012). Additionally, taxa belonging to several Acidobacterial subgroups were found in high relative abundance in all plots (Fig. S7), and OTUs belonging to several Acidobacterial subgroups were also found to be differentially abundant in tested treatments (Fig. 5). Members of the phylum Acidobacteria have been previously found in association with willow trees (Salix spp.) in PHC-contaminated soils in the far north (Bell et al., 2014). Together, this may indicate that microbial biodegradation of PHCs is an ongoing process despite very low TPH concentrations and that the microbial functional potential is stimulated by, or at least associated with, the current vegetation of the site. It has been hypothesized that plants with higher amounts of some secondary plant metabolites, such as aromatics, may lead to increased biodegradation potential in contaminated soils (Fletcher and Hegde, 1995; Singer et al., 2004; Slater et al., 2011). At higher latitudes, trees have increased concentrations of secondary metabolites when compared to similar tree species growing at lower latitudes (Stark et al., 2008); this includes many species identified on the investigated sites such as willow (Salix spp.; Table S4). Our data indicate that the presence and coverage of trees, all of which found on the site were native Alaskan species (Leewis et al., 2013), are directly related to increased degradation potential and TPH disappearance in both crude-oil- and diesel-contaminated soils.
Phytoremediation is a common practice for the restoration of sites contaminated with organic pollutants, with most studies pursuing results over the short- to mid-term range (< 5 years; Palmroth et al., 2002; White et al., 2006; Siciliano et al., 2003; Elshamy et al., 2019). With phytoremediation treatment, contaminant concentrations have been shown to decrease to below regulatory clean-up limits, with similar percentage losses from initial TPH concentrations compared to those presented here, within a short- to mid-term time range in high-latitude soils (Robichaud et al., 2019; Lopez-Echartea et al., 2020). Even without active treatment, there can be significant decreases in original contaminant concentrations which, over long time frames, may result in a site reaching regulatory clean-up limits (Leewis et al., 2013; Robichaud et al., 2019; Lopez-Echartea et al., 2020). However, accelerating the rate of contaminant disappearance and therefore site remediation is essential in increasing the speed of ecosystem recovery and minimizing environmental impacts while still maintaining reasonable costs. Here, we show that minimal initial investment of plants and fertilizer can increase the initial TPH disappearance rates while also priming the site for long-term volunteer vegetation cover that supports continued TPH disappearance and, ultimately, remediation of the site. While the original grasses planted were associated with significant decreases in soil TPH concentrations, they required annual replanting. Our results suggest that initial planting with local plants, which are better adapted to prevailing environmental conditions, may provide a low-cost method to increase long-term phytoremediation potential (Slater et al., 2011; Robichaud et al., 2019).
In conclusion, our study adds to the growing body of knowledge that phytoremediation treatment appears to be an effective means of reducing petroleum hydrocarbon concentrations in soil and stimulating indigenous TPH-degrading microorganisms and that the effects of original treatment can be seen long after active site management has ceased. The data also indicate the importance of carefully choosing the initial phytoremediation treatments as these conditions will leave a lasting legacy on TPH transformation, vegetation recruitment, and soil microbial communities. More research is needed to identify local native vegetation that may be optimal for initiating plant establishment and microbial succession and accelerating in situ remediation processes, particularly in sub-Arctic regions.
Raw sequence data are available for download from the NCBI Short Read Archive under bioproject no. PRJNA950456 (https://www.ncbi.nlm.nih.gov/bioproject/PRJNA950456, last access: 9 August 2024). All the coding for the analyses is available at https://github.com/mcleewis/FarmersLoop and https://doi.org/10.5281/zenodo.13288502 (Leewis, 2024).
The supplement related to this article is available online at: https://doi.org/10.5194/soil-10-551-2024-supplement.
MCL analyzed the data and wrote the first draft of the manuscript with support from OU. MCL and MBL designed the experiment. MCL, MBL, and CK conducted experiments. MBL provided equipment, supplies, and feedback. All the authors provided revisions of the paper.
The contact author has declared that none of the authors has any competing interests.
The content is solely the responsibility of the authors and does not necessarily reflect the official views of National Institutes of Health (NIH).
Publisher’s note: Copernicus Publications remains neutral with regard to jurisdictional claims made in the text, published maps, institutional affiliations, or any other geographical representation in this paper. While Copernicus Publications makes every effort to include appropriate place names, the final responsibility lies with the authors.
The authors acknowledge and thank C. Michael Reynolds (US Army Corps of Engineers) for earlier site work and establishment and Jim Fish (Alaska Department of Environmental Conservation) for the introduction to the site. The authors thank Carl Richmond for the help with sample collection and processing and Taylor Gofstein for the analytical inspiration.
The research reported in this publication was supported by Institutional Development Awards (IDeA) from the National Institute of General Medical Sciences of the National Institutes of Health under grant no. P20GM103395 and from the National Centre for Research Resources (NCRR) under grant no. 5P20RR016466 and EPSCoR NSF award no. EPS-0701898. Ondrej Uhlik was supported by the Ministry of Education, Youth and Sports of the Czech Republic grant for Talking Microbes – understanding microbial interactions within One Health framework (grant no. CZ.02.01.01/00/22_008/0004597). Additional financial support was provided by NSF DEB-1257424 (Mary Beth Leigh and Mary-Cathrine Leewis) and the NSEP David L. Boren Fellowship (Mary-Cathrine Leewis).
This paper was edited by Rafael Clemente and reviewed by Eglantina Lopez Echartea and one anonymous referee.
Akaçin, İ., Ersoy, Ş., Doluca, O., and Güngörmüşler, M.: Comparing the significance of the utilization of next generation and third generation sequencing technologies in microbial metagenomics, Microbiol. Res., 264, 127154, https://doi.org/10.1016/j.micres.2022.127154, 2022.
Alaska Department of Environmental Conservation Spill Prevention and Response: Contaminated Sites Database, https://dec.alaska.gov/Applications/SPAR/PublicMVC/CSP/Search/, last access: 24 June 2024.
Allison, S. D. and Treseder, K. K.: Climate change feedbacks to microbial decomposition in boreal soils, Fungal Ecol., 4, 362–374, https://doi.org/10.1016/j.funeco.2011.01.003, 2011.
Allison, S. D., Gartner, T. B., Mack, M. C., McGuire, K., and Treseder, K.: Nitrogen alters carbon dynamics during early succession in boreal forest, Soil Biol. Biochem., 42, 1157–1164, https://doi.org/10.1016/j.soilbio.2010.03.026, 2010.
Bell, T. H., Yergeau, E., Martineau, C., Juck, D., Whyte, L. G., and Greer, C. W.: Identification of Nitrogen-Incorporating Bacteria in Petroleum-Contaminated Arctic Soils by Using [15N]DNA-Based Stable Isotope Probing and Pyrosequencing, Appl. Environ. Microb., 77, 4163–4171, https://doi.org/10.1128/AEM.00172-11, 2011.
Bell, T. H., Yergeau, E., Juck, D. F., Whyte, L. G., and Greer, C. W.: Alteration of microbial community structure affects diesel biodegradation in an Arctic soil, FEMS Microbiol. Ecol., 85, 51–61, https://doi.org/10.1111/1574-6941.12102, 2013.
Bell, T. H., El-Din Hassan, S., Lauron-Moreau, A., Al-Otaibi, F., Hijri, M., Yergeau, E., and St-Arnaud, M.: Linkage between bacterial and fungal rhizosphere communities in hydrocarbon-contaminated soils is related to plant phylogeny, ISME J., 8, 331–343, https://doi.org/10.1038/ismej.2013.149, 2014.
Blanchet, G., Gavazov, K., Bragazza, L., and Sinaj, S.: Responses of soil properties and crop yields to different inorganic and organic amendments in a Swiss conventional farming system, Agr. Ecosyst. Environ., 230, 116–126, https://doi.org/10.1016/j.agee.2016.05.032, 2016.
Brown, S. P. and Jumpponen, A.: Contrasting primary successional trajectories of fungi and bacteria in retreating glacier soils, Mol. Ecol., 23, 481–497, https://doi.org/10.1111/mec.12487, 2014.
Chen, Z., Chen, W., Leblanc, S. G., and Henry, G. H. R.: Digital Photograph Analysis for Measuring Percent Plant Cover in the Arctic, ARCTIC, 63, 261–379, https://doi.org/10.14430/arctic1495, 2010.
Clark, C. M., Cleland, E. E., Collins, S. L., Fargione, J. E., Gough, L., Gross, K. L., Pennings, S. C., Suding, K. N., and Grace, J. B.: Environmental and plant community determinants of species loss following nitrogen enrichment, Ecol. Lett., 10, 596–607, https://doi.org/10.1111/j.1461-0248.2007.01053.x, 2007.
Cleland, E. E. and Harpole, W. S.: Nitrogen enrichment and plant communities, Ann. NY Acad. Sci., 1195, 46–61, https://doi.org/10.1111/j.1749-6632.2010.05458.x, 2010.
Cole, J. R., Wang, Q., Cardenas, E., Fish, J., Chai, B., Farris, R. J., Kulam-Syed-Mohideen, A. S., McGarrell, D. M., Marsh, T., Garrity, G. M., and Tiedje, J. M.: The Ribosomal Database Project: Improved alignments and new tools for rRNA analysis, Nucleic Acids Res., 37, 141–145, https://doi.org/10.1093/nar/gkn879, 2009.
Cole, J. R., Wang, Q., Fish, J. A., Chai, B., McGarrell, D. M., Sun, Y., Brown, C. T., Porras-Alfaro, A., Kuske, C. R., and Tiedje, J. M.: Ribosomal Database Project: data and tools for high throughput rRNA analysis, Nucleic Acids Res., 42, D633–D642, https://doi.org/10.1093/nar/gkt1244, 2014.
Pier, M. D., Zeeb, B. a, and Reimer, K. J.: Patterns of contamination among vascular plants exposed to local sources of polychlorinated biphenyls in the Canadian Arctic and Subarctic, Sci. Total Environ., 297, 215–227, 2002.
Elshamy, M. M., Heikal, Y. M., and Bonanomi, G.: Phytoremediation efficiency of Portulaca oleracea L. naturally growing in some industrial sites, Dakahlia District, Egypt, Chemosphere, 225, 678–687, https://doi.org/10.1016/j.chemosphere.2019.03.099, 2019.
Ferrera-Rodríguez, O., Greer, C. W., Juck, D., Consaul, L. L., Martínez-Romero, E., Whyte, L. G., and Martı, E.: Hydrocarbon-degrading potential of microbial communities from Arctic plants, J. Appl. Microbiol., 114, 71–83, https://doi.org/10.1111/jam.12020, 2012.
Fletcher, J. S. and Hegde, R. S.: Release of phenols by perennial plant roots and their potential importance in bioremediation, Chemosphere, 31, 3009–3016, https://doi.org/10.1016/0045-6535(95)00161-Z, 1995.
Geisseler, D. and Scow, K. M.: Long-term effects of mineral fertilizers on soil microorganisms – A review, Soil Biol. Biochem., 75, 54–63, https://doi.org/10.1016/j.soilbio.2014.03.023, 2014.
Gerhardt, K. E., Huang, X.-D., Glick, B. R., and Greenberg, B. M.: Phytoremediation and rhizoremediation of organic soil contaminants: Potential and challenges, Plant Sci., 176, 20–30, https://doi.org/10.1016/j.plantsci.2008.09.014, 2009.
Glick, B. R.: Using soil bacteria to facilitate phytoremediation, Biotechnol. Adv., 28, 367–374, https://doi.org/10.1016/j.biotechadv.2010.02.001, 2010.
Goordial, J., Davila, A., Lacelle, D., Pollard, W., Marinova, M. M., Greer, C. W., Di Ruggiero, J., McKay, C. P., and Whyte, L. G.: Nearing the cold-arid limits of microbial life in permafrost of an upper dry valley, Antarctica, ISME J., 10, 1613–1624, https://doi.org/10.1038/ismej.2015.239, 2016.
Haines, J. R., Wrenn, B. A., Holder, E. L., Strohmeier, K. L., Herrington, R. T., and Venosa, A. D.: Measurement of hydrocarbon-degrading microbial populations by a 96-well plate most-probable-number procedure, J. Ind. Microbiol., 16, 36–41, https://doi.org/10.1007/BF01569919, 1996.
Kaiser, C., Frank, A., Wild, B., Koranda, M., and Richter, A.: Negligible contribution from roots to soil-borne phospholipid fatty acid fungal biomarkers 18 : 2ω6,9 and 18 : 1ω9, Soil Biol. Biochem., 42, 1650–1652, https://doi.org/10.1016/j.soilbio.2010.05.019, 2010.
Knelman, J. E., Legg, T. M., O'Neill, S. P., Washenberger, C. L., González, A., Cleveland, C. C., and Nemergut, D. R.: Bacterial community structure and function change in association with colonizer plants during early primary succession in a glacier forefield, Soil Biol. Biochem., 46, 172–180, https://doi.org/10.1016/j.soilbio.2011.12.001, 2012.
Kuiper, I., Lagendijk, E. L., Bloemberg, G. V., and Lugtenberg, B. J. J.: Rhizoremediation: A Beneficial Plant-Microbe Interaction, Mol. Plant-Microbe Interact., 17, 6–15, https://doi.org/10.1094/MPMI.2004.17.1.6, 2004.
Lauber, C. L., Hamady, M., Knight, R., and Fierer, N.: Pyrosequencing-based assessment of soil pH as a predictor of soil bacterial community structure at the continental scale, Appl. Environ. Microbiol., 75, 5111–5120, https://doi.org/10.1128/AEM.00335-09, 2009.
Leewis, M.-C.: mcleewis/FarmersLoop: FarmersLoop_SOILpub (FarmersLoop_SOILpub), Zenodo [code], https://doi.org/10.5281/zenodo.13288502, 2024.
Leewis, M.-C., Reynolds, C. M., and Leigh, M. B.: Long-term effects of nutrient addition and phytoremediation on diesel and crude oil contaminated soils in subarctic Alaska, Cold Reg. Sci. Technol., 96, 129–137, https://doi.org/10.1016/j.coldregions.2013.08.011, 2013.
Leewis, M.-C., Uhlik, O., Fraraccio, S., McFarlin, K., Kottara, A., Glover, C., Macek, T., and Leigh, M. B.: Differential Impacts of Willow and Mineral Fertilizer on Bacterial Communities and Biodegradation in Diesel Fuel Oil-Contaminated Soil, Front. Microbiol., 7, 837, https://doi.org/10.3389/fmicb.2016.00837, 2016a.
Leewis, M.-C., Uhlik, O., and Leigh, M. B.: Synergistic Processing of Biphenyl and Benzoate: Carbon Flow Through the Bacterial Community in Polychlorinated-Biphenyl-Contaminated Soil, Sci. Rep., 6, 22145, https://doi.org/10.1038/srep22145, 2016b.
Leewis, M.-C., Lawrence, C. R., Schulz, M. S., Tfaily, M. M., Ayala-Ortiz, C. O., Flores, G. E., Mackelprang, R., and McFarland, J. W.: The influence of soil development on the depth distribution and structure of soil microbial communities, Soil Biol. Biochem., 174, 108808, https://doi.org/10.1016/j.soilbio.2022.108808, 2022.
Leigh, M. B. M. B., Fletcher, J. S. J. S., Fu, X., and Schmitz, F. J. F. J.: Root turnover: an important source of microbial substrates in rhizosphere remediation of recalcitrant contaminants, Environ. Sci. Technol., 36, 1579–1583, https://doi.org/10.1021/es015702i, 2002.
Liang, Y., Zhao, H., Deng, Y., Zhou, J., Li, G., Sun, B., Hug, L. A., Flynn, T. M., Sun, B., Liang, Y., Zhao, H., Deng, Y., Zhou, J., Li, G., and Sun, B.: Long-term oil contamination alters the molecular ecological networks of soil microbial functional genes, Front. Microbiol., 7, 1–13, https://doi.org/10.3389/fmicb.2016.00060, 2016.
Liu, W., Hou, J., Wang, Q., Yang, H., Luo, Y., and Christie, P.: Collection and analysis of root exudates of Festuca arundinacea L. and their role in facilitating the phytoremediation of petroleum-contaminated soil, Plant Soil, 389, 109–119, https://doi.org/10.1007/s11104-014-2345-9, 2015.
Loehr, R. C. and Webster, M. T.: Performance of long-term, field-scale bioremediation processes, J. Hazard. Mater., 50, 105–128, https://doi.org/10.1016/0304-3894(96)01797-9, 1996.
Lopez-Echartea, E., Strejcek, M., Mukherjee, S., Uhlik, O., and Yrjälä, K.: Bacterial succession in oil-contaminated soil under phytoremediation with poplars, Chemosphere, 243, 125242, https://doi.org/10.1016/j.chemosphere.2019.125242, 2020.
Love, M. I., Huber, W., and Anders, S.: Moderated estimation of fold change and dispersion for RNA-seq data with DESeq2, Genome Biol., 15, 1–21, https://doi.org/10.1186/s13059-014-0550-8, 2014.
Luo, C., Tsementzi, D., Kyrpides, N., Read, T., and Konstantinidis, K. T.: Direct comparisons of Illumina vs. Roche 454 sequencing technologies on the same microbial community DNA sample, PLoS One, 7, e30087, https://doi.org/10.1371/journal.pone.0030087, 2012.
Lupwayi, N. Z., Janzen, H. H., Bremer, E., Smith, E. G., Kanashiro, D. A., Eastman, A. H., and Petri, R. M.: The core soil bacterial genera and enzyme activities in incubated soils from century-old wheat rotations, Geoderma, 404, 115275, https://doi.org/10.1016/j.geoderma.2021.115275, 2021.
Macek, T., Uhlík, O., Ječná, K., Nováková, M., Lovecká, P., Rezek, J., Dudková, V., Štursa, P., Vrchotová, B., Pavlíková, D., Demnerová, K., and Macková, M.: Advances in phytoremediation and rhizoremediation, in: Advances in Applied Bioremediation, edited by: Singh, A., Kuhad, R., and Ward, O., Springer, Berlin, 257–277, 2009.
Man, J. C.: MPN tables, corrected, Eur. J. Appl. Microbiol., 17, 301–305, https://doi.org/10.1007/BF00508025, 1983.
McMurdie, P. J. and Holmes, S.: Phyloseq: An R Package for Reproducible Interactive Analysis and Graphics of Microbiome Census Data, PLoS One, 8, e61217, https://doi.org/10.1371/journal.pone.0061217, 2013.
Mills, T., Arnold, B., Sivakumaran, S., Northcott, G., Vogeler, I., Robinson, B., Norling, C., and Leonil, D.: Phytoremediation and long-term site management of soil contaminated with pentachlorophenol (PCP) and heavy metals, J. Environ. Manage., 79, 232–241, https://doi.org/10.1016/j.jenvman.2005.07.005, 2006.
Mukherjee, S., Juottonen, H., Siivonen, P., Lloret Quesada, C., Tuomi, P., Pulkkinen, P., and Yrjälä, K.: Spatial patterns of microbial diversity and activity in an aged creosote-contaminated site, ISME J., 8, 2131–2142, https://doi.org/10.1038/ismej.2014.151, 2014.
Musilova, L., Ridl, J., Polivkova, M., Macek, T., and Uhlik, O.: Effects of Secondary Plant Metabolites on Microbial Populations: Changes in Community Structure and Metabolic Activity in Contaminated Environments, Int. J. Mol. Sci., 17, 1205, https://doi.org/10.3390/ijms17081205, 2016.
Ngosong, C., Gabriel, E., and Ruess, L.: Use of the Signature Fatty Acid 16 : 1ω5 as a Tool to Determine the Distribution of Arbuscular Mycorrhizal Fungi in Soil, J. Lipids, 2012, 1–8, https://doi.org/10.1155/2012/236807, 2012.
Oburger, E., Gruber, B., Wanek, W., Watzinger, A., Stanetty, C., Schindlegger, Y., Hann, S., Schenkeveld, W. D. C., Kraemer, S. M., and Puschenreiter, M.: Microbial decomposition of 13C-labeled phytosiderophores in the rhizosphere of wheat: Mineralization dynamics and key microbial groups involved, Soil Biol. Biochem., 98, 196–207, https://doi.org/10.1016/j.soilbio.2016.04.014, 2016.
Oksanen, J., Blanchet, F. G., Friendly, M., Kindt, R., Legendre, P., McGlinn, D., Minchin, P. R., O'Hara, R. B., Simpson, G. L., Solymos, P., Stevens, M. H. H., Szoecs, E., and Wagner, H.: vegan: Community Ecology Package, R package version 2.4-5, https://vegan.r-forge.r-project.org/ (last access: 9 August 2024), 2017.
Palmroth, M. R. T., Pichtel, J., and Puhakka, J. A.: Phytoremediation of subarctic soil contaminated with diesel fuel, Bioresource Technol., 84, 221–228, 2002.
Palmroth, M. R. T., Münster, U., Pichtel, J., and Puhakka, J. A.: Metabolic responses of microbiota to diesel fuel addition in vegetated soil, Biodegradation, 16, 91–101, 2005.
Papik, J., Strejcek, M., Musilova, L., Guritz, R., Leewis, M.-C., Leigh, M. B., and Uhlik, O.: Legacy Effects of Phytoremediation on Plant-Associated Prokaryotic Communities in Remediated Subarctic Soil Historically Contaminated with Petroleum Hydrocarbons, Microbiol. Spectr., 11, e04448-22, https://doi.org/10.1128/spectrum.04448-22, 2023.
Polivkova, M., Suman, J., Strejcek, M., Kracmarova, M., Hradilova, M., Filipova, A., Cajthaml, T., Macek, T., and Uhlik, O.: Diversity of root-associated microbial populations of Tamarix parviflora cultivated under various conditions, Appl. Soil Ecol., 125, 264–272, https://doi.org/10.1016/j.apsoil.2018.02.002, 2018.
R Core Team: R: A language and environment for statistical computing, R Foundation for Statistical Computing, Vienna, Austria, https://www.R-project.org/ (last access: 5 July 2019), 2019.
Reeve, J. R., Schadt, C. W., Carpenter-Boggs, L., Kang, S., Zhou, J., and Reganold, J. P.: Effects of soil type and farm management on soil ecological functional genes and microbial activities, ISME J., 4, 1099–1107, https://doi.org/10.1038/ismej.2010.42, 2010.
Reynolds, C. M. and Koenen, B. A.: Rhizosphere-Enhanced Bioremediation, Mil. Eng., 586, 32–33, 1997.
Reynolds, C. M., Koenen, B. A., Perry, L. B., and Pidgeon, C. S.: Initial Field Results for Rhizosphere Treatment of Contaminated Soils in Cold Regions, in: International Association of Cold Regions Development Studies, Anchorage, Alaska, USA, 1997, 143–146, 1997a
Reynolds, C. M., Bhunia, P., and Koenen, B. A.: Soil Remediation Demonstration Project: Biodegradation of Heavy Fuel Oils, ACE CRREL Special Report 97-20, 1997b.
Reynolds, C. M., Perry, L. B., Pidgeon, C. S., Koenen, B. A., Pelton, D. K., and Foley, K. L.: Plant-Based Treatment of Organic-Contaminated Soils in Cold Climates, in: Proceeding of Assessment and Remediation of Contaminated Sites in Arctic and Cold Climates, Edmonton, Canada, 3–4 May 1999, 166–172, 1999.
Robichaud, K., Girard, C., Dagher, D., Stewart, K., Labrecque, M., Hijri, M., and Amyot, M.: Local fungi, willow and municipal compost effectively remediate petroleum-contaminated soil in the Canadian North, Chemosphere, 220, 47–55, https://doi.org/10.1016/j.chemosphere.2018.12.108, 2019.
Ruess, R. R. W., Van Cleve, K., Yarie, J., and Viereck, L. A.: Contributions of fine root production and turnover to the carbon and nitrogen cycling in taiga forests of the Alaskan interior, Can. J. Forest Res., 26, 1326–1336, https://doi.org/10.1139/x26-148, 1996.
Schloss, P. D., Gevers, D., and Westcott, S. L.: Reducing the Effects of PCR Amplification and Sequencing Artifacts on 16S rRNA-Based Studies, PLoS One, 6, e27310, https://doi.org/10.1371/journal.pone.0027310, 2011.
Schmid, M. W., van Moorsel, S. J., Hahl, T., De Luca, E., De Deyn, G. B., Wagg, C., Niklaus, P. A., and Schmid, B.: Effects of plant community history, soil legacy and plant diversity on soil microbial communities, J. Ecol., 109, 3007–3023, https://doi.org/10.1111/1365-2745.13714, 2021.
Siciliano, S. D. and Germida, J. J.: Mechanisms of phytoremediation: biochemical and ecological interactions between plants and bacteria, Environ. Rev., 6, 65–79, 1998.
Siciliano, S. D. D., Germida, J. J. J., Banks, K., and Greer, C. W.: Changes in Microbial Community Composition and Function during a Polyaromatic Hydrocarbon Phytoremediation Field Trial, Appl. Environ. Microb., 69, 483–489, https://doi.org/10.1128/AEM.69.1.483, 2003.
Singer, A. C., Smith, D., Jury, W. A., Hathuc, K., and Crowley, D. E.: IMPACT OF THE PLANT RHIZOSPHERE AND AUGMENTATION ON REMEDIATION OF POLYCHLORINATED BIPHENYL CONTAMINATED SOIL, Environ. Toxicol. Chem., 22, 1998–2004, https://doi.org/10.1897/02-471, 2003.
Singer, A. C., Thompson, I. P., and Bailey, M. J.: The tritrophic trinity: A source of pollutant-degrading enzymes and its implications for phytoremediation, Curr. Opin. Microbiol., 7, 239–244, https://doi.org/10.1016/j.mib.2004.04.007, 2004.
Slater, H., Gouin, T., and Leigh, M. B.: Assessing the potential for rhizoremediation of PCB contaminated soils in northern regions using native tree species, Chemosphere, 84, 199–206, https://doi.org/10.1016/j.chemosphere.2011.04.058, 2011.
Song, Y., Deng, S. P., Acosta-Martínez, V., and Katsalirou, E.: Characterization of redox-related soil microbial communities along a river floodplain continuum by fatty acid methyl ester (FAME) and 16S rRNA genes, Appl. Soil Ecol., 40, 499–509, https://doi.org/10.1016/j.apsoil.2008.07.005, 2008.
Stark, S., Julkunen-Tiitto, R., Holappa, E., Mikkola, K., and Nikula, A.: Concentrations of Foliar Quercetin in Natural Populations of White Birch (Betula pubescens) Increase with Latitude, J. Chem. Ecol., 34, 1382–1391, https://doi.org/10.1007/s10886-008-9554-8, 2008.
Stella, T., Covino, S., Burianová, E., Filipová, A., Křesinová, Z., Voříšková, J., Větrovský, T., Baldrian, P., and Cajthaml, T.: Chemical and microbiological characterization of an aged PCB-contaminated soil, Sci. Total Environ., 533, 177–186, https://doi.org/10.1016/j.scitotenv.2015.06.019, 2015.
Stow, J. P., Sova, J., and Reimer, K. J.: The relative influence of distant and local (DEW-line) PCB sources in the Canadian Arctic, Sci. Total Environ., 342, 107–118, https://doi.org/10.1016/j.scitotenv.2004.12.028, 2005.
Susarla, S., Medina, V. F., and McCutcheon, S. C.: Phytoremediation: An ecological solution to organic chemical contamination, Ecol. Eng., 18, 647–658, https://doi.org/10.1016/S0925-8574(02)00026-5, 2002.
Sutton, N. B., Maphosa, F., Morillo, J. A., Abu Al-Soud, W., Langenhoff, A. a M., Grotenhuis, T., Rijnaarts, H. H. M., Smidt, H., Al-Soud, W. A., Langenhoff, A. a M., Grotenhuis, T., Rijnaarts, H. H. M., Smidt, H., Abu, W., Langenhoff, A. a M., Grotenhuis, T., Huub, H., Rijnaarts, M., and Smidt, H.: Impact of long-term diesel contamination on soil microbial community structure, Appl. Environ. Microb., 79, 619–630, https://doi.org/10.1128/AEM.02747-12, 2013.
Talbot, J. M. and Treseder, K. K.: Interactions among lignin, cellulose, and nitrogen drive litter chemistry-decay relationships, Ecology, 93, 345–354, https://doi.org/10.1890/11-0843.1, 2012.
Uhlik, O., Wald, J., Strejcek, M., Musilova, L., Ridl, J., Hroudova, M., Vlcek, C., Cardenas, E., Mackova, M., and Macek, T.: Identification of Bacteria Utilizing Biphenyl, Benzoate, and Naphthalene in Long-Term Contaminated Soil, PLoS One, 7, e40653, https://doi.org/10.1371/journal.pone.0040653, 2012.
Van Aken, B., Correa, P. A., Schnoor, J. L., Van Aken, B., Correa, P. A., and Schnoor, J. L.: Phytoremediation of Polychlorinated Biphenyls: New Trends and Promises, Environ. Sci. Technol., 44, 2767–2776, https://doi.org/10.1021/es902514d, 2010.
Vanha-Majamaa, I., Salemaa, M., Tuominen, S., and Mikkola, K.: Digitized photographs in vegetation analysis – a comparison of cover estimates, Appl. Veg. Sci., 3, 89–94, https://doi.org/10.2307/1478922, 2000.
Wang, Q., Garrity, G. M., Tiedje, J. M., and Cole, J. R.: Naive Bayesian classifier for rapid assignment of rRNA sequences into the new bacterial taxonomy, Appl. Environ. Microb., 73, 5261–5267, https://doi.org/10.1128/AEM.00062-07, 2007.
Wenzel, W. W.: Rhizosphere processes and management in plant-assisted bioremediation (phytoremediation) of soils, Plant Soil, 321, 385–408, https://doi.org/10.1007/s11104-008-9686-1, 2009.
White, P. M., Wolf, D. C., Thoma, G. J., and Reynolds, C. M.: Phytoremediation of Alkylated Polycyclic Aromatic Hydrocarbons in a Crude Oil-Contaminated Soil, Water, Air. Soil Pollut., 169, 207–220, https://doi.org/10.1007/s11270-006-2194-0, 2006.
Wu, Y., Ding, N., Wang, G., Xu, J., Wu, J., and Brookes, P. C.: Effects of different soil weights, storage times and extraction methods on soil phospholipid fatty acid analyses, Geoderma, 150, 171–178, https://doi.org/10.1016/j.geoderma.2009.02.003, 2009.
Yergeau, E., Bell, T., Champagne, J., Maynard, C., Tardif, S., Tremblay, J., and Greer, C.: Transplanting soil microbiomes leads to lasting effects on willow growth, but not on the rhizosphere microbiome, Front. Microbiol., 6, 1–14, https://doi.org/10.3389/fmicb.2015.01436, 2015.
Zubrova, A., Michalikova, K., Semerad, J., Strejcek, M., Cajthaml, T., Suman, J., and Uhlik, O.: Biphenyl 2,3-Dioxygenase in Pseudomonas alcaliphila JAB1 Is Both Induced by Phenolics and Monoterpenes and Involved in Their Transformation, Front. Microbiol., 12, 657311, https://doi.org/10.3389/fmicb.2021.657311, 2021.